39 Neuroprosthetics
Abstract
Can a computer infer human intention or perception? It is now possible that it can.
Devices that convert brain signals, reflect its intentions, and then control an external device are known as neuroprosthetics. Their future development will have significant implications for the neurologically disabled. The greater our understanding of the cortical physiology underlying human intentions, the closer we will get to understanding the complexity of brain-derived control. This chapter summarizes the current status of the field of brain computer interface (BCI) and trends that may improve future clinical applications.
39.1 Introduction
The expanding field of neuroprosthetics will substantially impact the future practice of neurosurgery. A neuroprosthetic device supplants or supplements the input and/or output of the nervous system. Neuroprosthetics can now bypass a deficit caused by disease or augment function to improve performance. Understand that there is varied terminology used to describe the development of this technology. Terms in use include brain computer interface (BCI), brain machine interface (BMI), neural interface system (NIS), direct neural interface (DNI), and mind-machine interface (MMI). These terms and others all describe the interface of the nervous system and external devices.
The field of neuroprosthetics integrates numerous disciplines. These include neuroscience, computer science, and engineering. Brain-machine interfacing research was actually conceived long before the advent of it as an organized academic field of study. Early questions were raised by Vidal, who in 1973 asked when studying electroencephalograms, “Can these observable electrical brain signals be put to work as carriers of information in man-computer communication or for the purpose of controlling such external apparatus as prosthetic devices or spaceships?” While his grander visions have not come to fruition, brain-driven operation of a prosthetic device is becoming commonplace in research labs across the world.
The field was stymied by the slow computation speeds of existing computers and software systems. Advances in microprocessor design/speeds and digital signal analysis enable computers to go far beyond current neuroprosthetic requirements. Computational speed is no longer a limiting factor. Technological advances in other relevant fields such as virtual reality, robotics, haptics, advanced imaging, and biomaterials also have provided the necessary tools for device development. This allows innovative introduction of motor, sensory, visual, auditory, speech, and other applications to the field. In this chapter we will review these applications and emphasize the role of neurosurgeons in its translation from fundamental research to clinical application.
39.2 Interface Modalities
The goal of a neuroprosthetic device is to replace or augment an individual’s function by interfacing an external device with the nervous system. In the early phase of this field this was understood to be a device that detects brain signals to infer intentions, and then transforms those intentions to an external effector. Since the field’s inception there have been many different types of signal inputs that have been used to achieve this goal. Each of these has distinct clinical considerations. The sources of signal input range from individual neuronal spiking, to field potential from cortical ensembles, or action potentials conducted by peripheral nerves. Output effectors are also wide-ranging. Classic and current examples include computer cursor control, robotic arm movement, and re-animation of paretic limbs. Neuroprosthetics are not unidirectional output devices. By reversing the direction of information transfer, an input device can provide simulated perceptions. Here the device records signals from the external environment and converts those signals into an appropriate stimulus delivered to the nervous system. These devices may thus use sensory input to provide tactile or proprioceptive feedback to assist with the control of robotic arm movements. It may also be an independent application. Cochlear implants record sounds and translate them into electrical stimuli. These are then delivered to the acoustic nerve and provide auditory perception to an otherwise deaf patient. In the broadest sense, neuroprosthetics can be categorized as “output neural interfaces” that convert the brain’s intentions to external actions, or as “input neural interfaces” which take information from the environment and convert them into the brain’s perceptions.
Neuroprosthetics may also be characterized by their mode of operation be it motor, somatosensory, speech, auditory and the source of neural interface (single unit neuron, cortical local field potential, peripheral nerve). The most successful neuroprosthesis to date is the cochlear implant. For neurosurgery the neuroprosthetics that are poised to impact the field are those that provide motor output. The goal of a motor output prosthesis is to be a device capable of interpreting an individual’s movement intentions in real-time and direct the output to an effector such as a robotic limb. With these examples in mind, the various means of interfacing with the nervous system and their clinical implications will be explored.
39.3 Electroencephalography
Because it is non-invasive, electroencephalography (EEG) is still the most common means of studying cerebral electrophysiology. Electrodes are placed on a subject’s scalp and electrical potentials from large cortical populations are recorded. Changes in neural rhythms are measured to evaluate various types of cognitive processes. The EEG, represents the summated electrical potentials from the electrochemical interaction of a vast number of cells, both neuronal and glial in origin. This necessarily limits the complexity and precision of the information it provides.
Amplifiers and converters digitize the electrical signals that have been derived from the EEG. By sampling a continuous signal in brief, regular intervals, discrete values are obtained and stored in series, thereby converting the signal from an analog to a digital domain. The time-varying signal is then analyzed as the sum of multiple sinusoidal signals of varying frequency and amplitude. This process, using the Fourier transform, converts signals from a time domain to a frequency domain. In the frequency domain one can observe the change in power at a given frequency over time. This is known as time-frequency analysis. The distribution of signal power across a range of frequencies is the signal spectrum and its plot over time is the spectrogram. By time-locking the sampled signal to measured subject data, correlations are made between cortical activity and task performance. One such observation in signals measured over the motor homunculus is the reliably reproducible decrease in spectral power in the 8 to 13 Hz range. This occurs with overt or imagined movement of the hand contralateral to the side of the cortical recording.
Relevant physiologic signals in the EEG in humans is segmented into distinct components in the frequency domain and named by Greek alphabet characters. These are classically defined as the delta band below 4 Hz, theta band from 4 to 8 Hz, mu band from 8 to 13 Hz (also known as alpha), beta band from 13 to 30 Hz, and gamma band beyond 30 Hz. The mu rhythm is associated with motor electrophysiology and the Rolandic cortex (▶ Fig. 39.1a). Decreases in mu band spectral power with activity (▶ Fig. 39.1b) are thought to represent event related desynchronization. This release of synchronized cortical rhythms is thought to involve thalamocortical circuits modulating motor cortical systems (▶ Fig. 39.1c). Real-time measurements of power fluctuations in this frequency range provide a signal that represents motor movement intentions. Wolpaw and colleagues showed that these mu motor signals could be used to control a virtual cursor displayed on a computer screen using either real or imagined motor movements. In effect, the velocity of cursor movement was derived in real-time from changes in the mu spectral power volitionally modulated by the user. Similar modulations of EEG are observed in imagined motor actions. This allows control without any peripheral activation of motor units.

EEG correlates of imagined motor movements are critical to the clinical application of BCIs in motor impaired patients. Real-world translation is impractical if overt motor function is required for the production of an adequate source control signal. Simply said, those who would need brain controlled prosthesis should not be required to move a limb to generate a control signal. It is thus essential that individuals affected by neurologic disorders such as amyotrophic lateral sclerosis (ALS), locked-in syndrome, or spinal cord injury (SCI) be capable of modulating cortical rhythms in the absence of end organ effect. Fortunately, preservation of this capability has been found in subjects with long-term deficits. Volitional modulation of stable electrophysiologic structures has been maintained.
To date, EEG-based brain computer interfaces have led the field in human neuroprosthetic research. One limitation of EEG methodologies is the physical separation of the cortical source signal and the scalp based recording electrodes. Intervening meninges, bone, and scalp limits spectral and spatial resolution of the signal associated with specific cognitive processes. For an EEG electrode to record a measurable signal from the cortex, electrical potentials must be summated across an area of cortex approximately 6 cm2. The spatial resolution of independent signals is thereby limited. Similarly, destructive summation of temporally overlapping signals results in cancelation of higher frequencies in EEG recordings (i.e gamma rhythms which carry substantial information on cognitive processes). These limitations may be mitigated in part by intracranial placement of recording electrodes, but at the cost of increased invasiveness (▶ Fig. 39.2).

39.4 Electrocorticography
Signals from non-penetrating electrodes on the cortical surface have features that may be optimal for clinical application. The means to obtain such signals is known as electrocorticography (ECoG). While placement of the electrodes requires surgery, such signals balance clinical risk with acceptable signal quality, durability, and reliability. They can enable a neuroprosthetic solution for the near future. Because ECoG electrodes record directly from the cortical surface (▶ Fig. 39.3b), the signals have excellent spatial and spectral resolution when compared to EEG. The higher signal-to-noise ratio is due in part to physical proximity, and to the reduced shielding effects of the skull (reducing ambient muscle and environmental noise). Because these electrodes do not penetrate cortex, the immunologic response to them is lessened. This improves long-term signal quality. ECoG has a long history of use in clinical neurosurgery. It was the initial application used by Penfield and Jasper in epilepsy surgery. Such a background better enables a straightforward technical transition from current methods to neuroprosthetic applications in the future.

There has been a substantial increase in the use of the ECoG approach for neuroprosthetic research since its initial demonstration of closed loop BCI control by patients undergoing invasive intracranial monitoring for epilepsy surgery. There have been numerous studies examining motor intentions to enable control of external devices. Furthermore, studies have shown that the motor physiology features that enable neuroprosthetic applications remain consistent from a pediatric age to advanced age. An additional benefit of the use of human subjects in research is that BCI research can expand beyond classic motor physiology as a signal source. While animal models have several distinct advantages in research design, higher cognitive functions are inaccessible in non-human subjects. One example of human-specific cognitive operations used for BCI applications is the use of speech cortex and speech intentions for device control. ECoG signals over speech cortex can be modulated by imagined and overt speech production by the monitored subject. The user is then able to control the direction of a virtual cursor by saying out loud, or imagining saying covertly, various discriminating words pre-assigned to opposing direction.
ECoG also has several signal analysis advantages over noninvasive electroencephalography. Spectral resolution with EEG is limited to frequencies less than approximately 30–40 Hz (▶ Fig. 39.1b). This occurs because of the nature of the conductive properties of the skull and scalp, signal deterioration because of the increased distance between source and electrode, and the logarithmically decreasing signal power that occurs with frequency increases. These EEG limitations are overcome by intracranial electrode placement. Frequencies greater than 40 Hz, known as gamma rhythms, have been used to decode cognitive intentions associated with auditory processing, speech production, and higher cognitive functions. All of these have been used to demonstrate BCI control with ECoG in humans. Furthermore, Gaona et al., found sub-band selectivity in the high gamma range for behavior and location dependent activation during the performance of certain cognitive tasks. This is a feature not observed in the EEG frequency range.
Spatial resolution is improved by electrode placement directly onto the cortical surface. This higher anatomic fidelity translates into improved discrimination of cognitive tasks. Examples include the accurate decoding of individual finger movement (versus just hand movement) and phoneme articulation (versus just general speaking). Decreasing the size and spacing of electrode arrays increases spatial resolution. Common clinical ECoG arrays use inter electrode spacing of one centimeter. Experimental evidence suggests that less than 5 mm is plausible with microwires and continues to provide meaningful signals. Another advantage of signal source proximity is the magnitude of the voltage. While both ECoG and EEG require amplification of signals for sufficient signal analysis, scalp-based measurements are in the range of 10–100 µV. Cortical surface measurements reach up to 10–20 mV. This is several degrees of magnitude higher than with EEG. Higher voltages make it easier to filter noise and ambient signal from the environment and to reach a signal-to-noise ratio adequate for neuroprosthetic applications.
These advantages are being translated into early clinical trials for motor impaired patients. Wang et al. used a temporary ECoG implant for BCI control in a tetraplegic patient with a high cervical spinal cord injury. Their group implanted a custom designed high-density ECoG array over primary motor cortex that had been identified by fMRI. The subject had a complete C4 level spinal cord injury that occurred seven years earlier. He had no motor control of his upper extremities. Over the course of a four week implant period, the user was able to progress through a series of experiments sequentially achieving two-dimensional cursor, three-dimensional cursor, and ultimately three-dimensional robotic arm control. Vansteensel et al. implanted a chronic ECoG BCI in a patient with late-stage amyotrophic lateral sclerosis (ALS). This provided a brain–computer interface of subdural electrodes located over the motor cortex and connected to a transmitter placed subcutaneously in the left thorax. When the patient attempted to move the hand opposite to the side of the implanted electrodes, he was able to accurately and independently control a computer-typing program. The task performance required 28 weeks of training and he could type two letters per minute. The brain–computer interface offered autonomous communication that supplemented and at times supplanted the patient’s eye-tracking device.
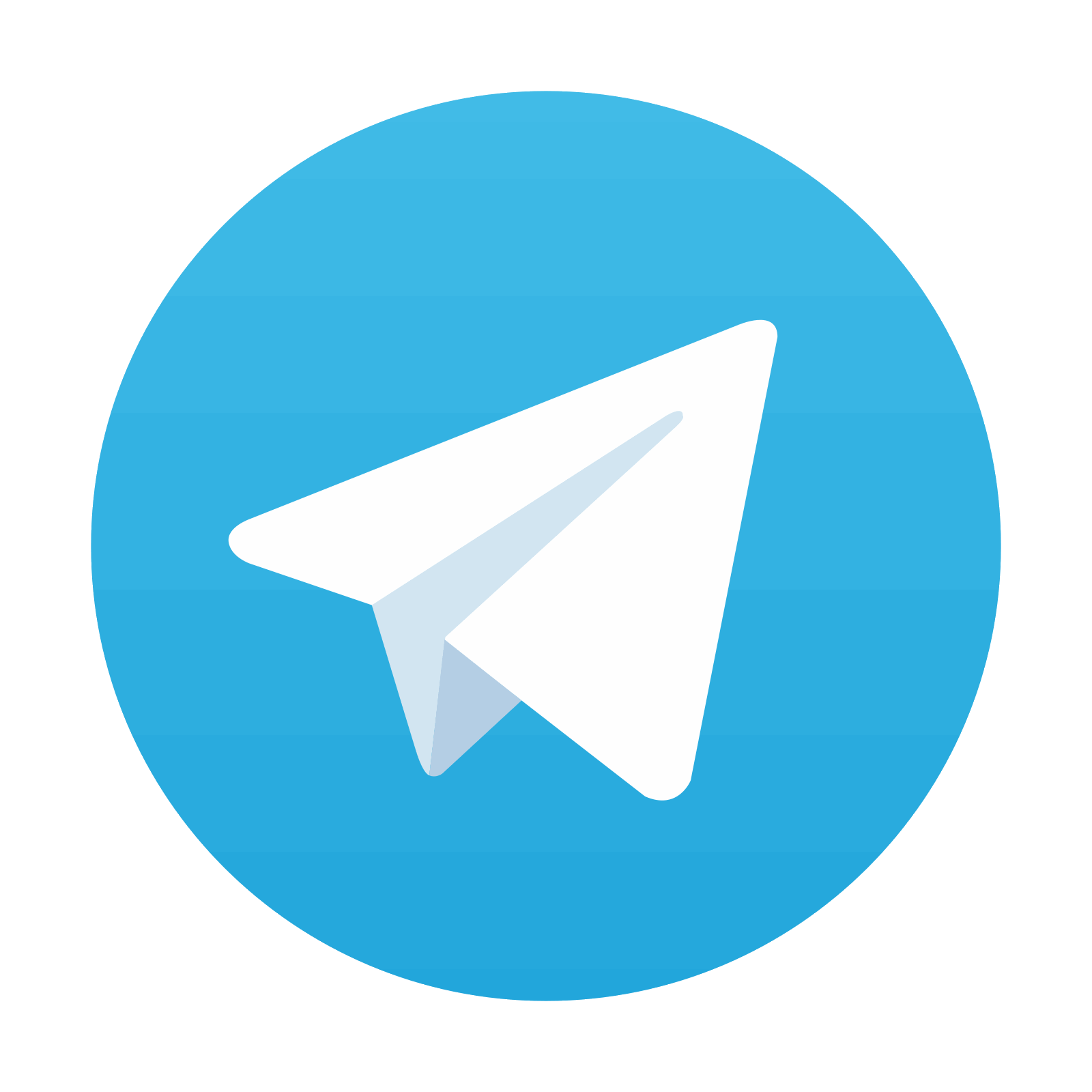
Stay updated, free articles. Join our Telegram channel

Full access? Get Clinical Tree
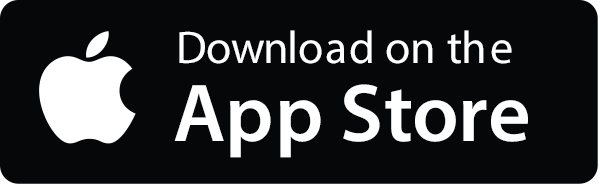
