4 An Introduction to Macroconnectomic Networks in Cerebral Surgery
4.1 Introduction
Macroconnectomics and functional network based thinking are the foundation of rational decision making in glioma surgery and this book and philosophy are based on them. So it is probably worth defining the terms early.
The term connectomics refers to efforts to create a connectional map of the human cerebrum. Such efforts are ambitious and likely will yield key insights into how human cognition works. However, they are of limited clinical utility at present. Beyond just the fact that they are not done, they provide a level of detail and complexity that we cannot really harness with our hands or our minds to drive surgical efforts. Until we have better lasers or better brains, we cannot use this much information to make the kind of decisions we need to make in a pinch.
Macroconnectomics is a term we coined at our center to describe the kind of connectomics we were studying in our efforts to do better brain surgery, which is connectional anatomy involving bulk information flow though large connections. In other words, we were not aiming to know every single neuronal connection, but rather the big connections and the important ones. The rationale for the macroconnectomic approach is threefold. First, the practical ability to utilize a brain map with billions of mapped connections is limited without technological advances in other areas to work at that scale. Second, it will likely be some time before a map of that complexity will be complete and annotated, and in the meantime we have a very poor understanding of even major brain connections underlying elemental brain functions such as speech, vision, and attention, so it is probably best to first work on the lower parts of Maslow’s hierarchy of needs and fix the massive knowledge gaps necessary for daily practice of brain surgery prior to aiming for perfect models. Finally, it seems unlikely to me that there is a single neuron in any place in the brain that can be cut to cause a deficit, especially one that cannot recover. In other words, clinically relevant deficits generally require a significant number of neurons to be taken offline in a network before they become evident.
In short, during a macroconnectomic glioma surgery, our goal is to understand a functional network and its major connections, and to perform resections in a way that the network maintains its function.
Thinking in terms of functional networks in brain surgery means planning and executing brain surgery with the idea that higher cognitive functions require the coordination of several brain structures, each of which contribute a subprocessing component to the overall successful end output, and that functional preservation requires anatomic preservation of these structures and their interconnections.
The idea that certain complex brain functions are located in a single place or two places, is contradicted by functional imaging data which shows multiple co-activated areas during most higher tasks, by neuro-psychological data showing complex and subtle variations of neuropsychological syndromes (i.e., aphasia, apraxia, etc.) with varying injuries of often distant parts of the brain, and by the experience of most of us who have noted neuro-psychologic consequences of our surgery that “don’t make sense.” I would suggest that when a patient has a neuro-psychologic deficit that “doesn’t make sense,” that often an explanation can be found by thinking of the function in terms of a network, as opposed to thinking of brain functions being localized to a single part of the brain, or a specific gyrus.
4.2 An Introduction to Complexity and Cerebral Networks
For over a century, neurosurgery has been working in the cerebrum with a model of the brain that not only is incorrect, but I would argue cannot possibly be a basically correct view of the brain. Localizationism, the idea that certain functions are performed by specific parts of the brain, like many outdated models, does lead to some often good predictions, for example primary motor functions are usually found in the precentral gyrus in most people. However, such a model will fail one if followed blindly off the cliff, especially in patients who reorganize, and it has minimal ability to work towards a world where we do not unintentionally damage higher cognitive functions, which do not fit the ideas put forward by this model. I put forward the idea that for neurosurgery to improve our outcomes, we need to embrace “complexity” and begin to restructure our ideas around the idea that we are cutting around functional networks.
The term “complex” does not merely signify that a system has a lot of parts, that it is difficult to study, or that it is poorly understood. A cell phone is complicated; it is not “complex.” Formally defined, the term “complex systems” refers to large networks of diverse, interconnected, interdependent and adaptive parts. In other words, to be “complex” a system needs to have a network of interconnected entities which react to each other’s activity, usually in nonlinear ways. Most of the persistent problems we face as a society: the behavior of the stock market; economics; global terrorism; neuroscience; the proteomics of cancer; and so on, have complexity as a predominant issue. In other words, we will have trouble improving our nonlinear complex problems with linear thinking. Of note, many of the earliest seminal papers in studying complexity are less than 20 years old, and presently the mathematics are in evolution.
“Complex” systems display some unique properties not seen in linear, randomly connected, or regularly interconnected systems. Most notable, complex systems demonstrate a property called emergence, which is when a “complex” network and its nonlinear dynamics, demonstrate behavior on the macro scale which cannot possibly be predicted a priori by the simple sum of the parts and their individual interactions. Higher cognitive functions, even consciousness itself, are emergent phenomenon which cannot be predicted simply by looking at the binary fire/don’t fire nature of individual neuron action potentials.
Another feature of complex networks is the presence of power law mathematical distributions within their static and dynamic structures. While most variables which are influenced by multiple independent variables vary in normal or near normal distribution (i.e., a bell curve), complex systems often vary in a power law fashion, meaning that the distribution curve has a very long broad tail. Height is a variable influenced by numerous mostly independent influences, and it is roughly normally distributed. This means that as we move several standard deviations away from mean height, that the probability of finding extreme outliers approaches zero. For example, there has never been a 10-foot-tall person in recorded history, and a 20-foot or 100-foot-tall person would be unthinkable. If height were distributed as a power law, then 100-foot-tall people would be uncommon, but occasionally we would see one. This means that very extreme outliers are uncommon but possible.
The degree of connectedness of brain nodes (i.e., the number of other areas an area is connected to), doesn’t perfectly conform to a power law distribution, but it is closer to a broad tailed distribution than a normal distribution. This means that while most areas of the brain are mainly connected to immediate neighbors, a few areas (so called hubs) are extremely interconnected to a large number of areas.
Final observations about complex networks are that they are robust. Deletion studies of brain networks, where nodes are removed from a network to determine the effect on the network, have shown that power law distributed networks are highly resilient to random deletions, meaning that the path length is relatively unchanged due to the loss of individual nodes. However, targeted attack on the most highly connected nodes cause the network to fragment at relatively low numbers of nodes deleted. The parallels between the eloquence versus non-eloquence binary which has long dominated our thinking is striking, and it provides a better model I think for thinking through the problem.
Robustness may also explain the early recovery of some neurologic problems (in addition to brains swelling resolution), as the network might figure a way to perform a cognitive task without the injured area. It might also be a key way gliomas cause the brain to reorganize.
4.3 Small World Networks
One of the seminal papers of the past 20 years in science was published by Watts and Strogatz in 1998 in a paper cited over 32,000 times at the time of this book’s writing. In this simple, elegant study, they studied completely regular, lattice graphs (graphs where every node was initially only connected to its neighbors), and used computer simulation to randomly rewire one connection at a time. They found that the path length (meaning the mean number of connections distance between any two nodes in the graph) decreased in a steep nonlinear fashion dropping substantially when only a small fraction of the connections were rewired. They termed this the small world phenomenon, and it has been shown to be a common organization in diverse complex networks including the internet, social networks, and the brain. In other words, complexity is governed by common mathematical principles regardless of the area of study, and that mathematics is heavily driven by small world topology.
There is abundant evidence that brain networks follow small world patterns of organization. This means that the dominant mode of connectivity is modular, meaning most areas are most connected to their neighbors, with interconnections between modules principally linked through the highly connected nodes, occasionally with linker nodes whose main function is to ensure no lag synchronization between two distant areas which need to synchronize their activity.
Complex networks have been frequently shown to be self-organizing, meaning that they usually do not have a dominant area which drives the activity or static organization of the network. For example, who really can control the global activity of the stock market. The small world organization is a logical effect of the need for the brain to achieve its static and dynamic goals with a minimal consumption of energy. Statically, it is most efficient for areas to make the shortest axons possible, and most areas should thus be mainly connected to their neighbors. However, as Watts and Strogatz demonstrated, path length drops substantially with making a few long range connections. Path length in the brain equals the number of synapses, and the increasing synapse number needed for two distant areas to communicate, slows long range conduction, increases noise, and increases energy costs. Small world networks are the result of self-organization driven around these competing demands.
There are many ways to describe brain networks; however, the one presently most accessible to us for surgery, is to focus on major white matter pathways, as they are demonstrable and disproportionately central to the proper functioning of wide-scale brain networks. Given that these tracts are the principle method by which two modules of nodes talk to each other, it is reasonable to conclude that they play a disproportionate role per mL of tissue in cognitive function.
4.4 Static versus Dynamic Connectivity
It is tempting to look at brain networks and their architecture and try to deduce a direction of information flow, and to determine that this is showing us the master blueprint of how the brain works. The reality, as usual, is far more complex. Structural connections are meaningless without understanding function, and studies of functional connectivity has taught us that the functional connections of the brain are changing constantly. For example, standard resting state fMRI provides statistical evidence that two areas coactivate with each other more than chance, and this is the basis of determining the location of functional networks. However, more nuanced analyses of so-called dynamic functional connectivity, have shown that this approach is akin to opening the camera shutter at an auto race for a few minutes and then pointing to the strongest blurs. The brain is constantly altering its activity pattern depending on context and cognitive tasks, and while areas A and B may coactivate for some tasks, areas A, C, and D may need to co-activate for others.
Obviously, sorting out an already complex static network is hard enough without accounting for the thousands of possible metastable states for all situations. This is a work for supercomputers, and the mathematical tools for working this out are in evolution. It is critical to note that the dynamic configuration of brain activities is constrained by the possibilities of the static brain network. In other words, areas A, B, and C cannot talk to each other if they are not connected in some way, either directly, or indirectly. Until dynamic connectivity is better understood, it is at least a good idea not to disconnect key parts of the network from itself. However, we should always look towards the future that to truly understand how to improve our outcomes, we need to continuously improve our models of the brain. Further, it highlights the drastic need to abandon our old models.
4.5 Nodes, Edges, Hubs and Rich Clubs
Graph theoretical metrics are not presently clinically accessible to brain surgeons; however, I have long believed that eventually they will be as they have the promise to answer questions. Thus, a short digression to define terms is worth a few paragraphs of space.
A brain graph analysis reduces the connections of the brain to a ball and stick type graphical format comprised of nodes, which signify specific brain regions, and edges, which are the lines signifying the connections between them. It does not take a high level of exposure to the area to recognize that nodes are grey matter structures and edges are axons/white matter. The concept of how to define the best way to split the brain into nodes exactly is a contentious one, but in almost all systems, most of the nodes are parts of the cerebral cortex, with nodes in thalamic and other subcortical nuclei included in some graphs. Either way, while a graph does not necessarily reflect the nature of the transformations enacted by various nodes, it does allow some basic hypothesis testing on the overall organization of the connectome.
A key question in graph theory is the definition of a hub, in other words a node which is extensively connected to many other areas, and thus assumed to be the pathway involved in numerous systems. There are several ways to define such a hub. Degree centrality refers to the number of other nodes which connect to a node. This is simple, but ignores the fact that a connection to a highly connected node should count more than a connection to a peripheral low degree node. This is corrected by measures such as eigenvector centrality and page rank centrality which take connectivity of neighbors into account. Closeness and betweenness centrality consider the number of shortest paths through the graph which traverse a node. Further, highly connected nodes which are highly connected to each other comprise a so called “rich club.”
Does “hubness” indicate parts of the brain we should avoid destroying and if so what measure is best? These are unknown and in need of study. Of note some of the more well known primary cortices, such as the primary motor cortex and primary visual area, are neither hubs nor in rich clubs. Others, like area 44, known for its role in language, definitely are. Based on mathematical measurements of random versus targeted attacks on broad scale networks (such as the brain) it is likely that preserving hubs are critical in preventing cognitive networks from collapsing; however, it is unclear which hubs, and what happens if you don’t achieve this.
4.6 What Parts of the Brain are Truly Eloquent
For decades, neurosurgeons have divided the cerebral world into “eloquent” brain and “non-eloquent brain” with the dividing line being whether the brain was felt to do something clinically evident when you cut into, removed or damaged it. In this conceptual model of how cerebral functions are organized in the brain, areas such as the motor strip, where an injury would cause a motor deficit, are “eloquent” and thus best avoided, while other areas of the brain, such as the anterior right frontal lobe were more “silent” and safe to cut into.
This is a system for organizing brain function which is simple, easy to teach residents, and completely inconsistent with common sense and scientific studies. I would argue that there are probably no legitimately “silent” areas of the brain. All parts of the brain probably did not evolve as a decoration, a CSF sponge, or a shock absorber, but rather evolved to perform some neurologic function. Thus, all parts of the brain are eloquent in some way and are doing something. If you are skeptical of this, spend some time talking to a family member of someone who has had a large amount of right frontal lobe damage, and you will probably become convinced that there are consequences to losing this part of your brain, they just are not glaringly obvious immediately after surgery in the recovering room.
This is not to argue that brain function is randomly distributed, or that some parts of the cortex are not more tolerant of injury than others. No inaccurate scientific model persists for years without at least some explanatory power. It’s just that the model we work under presently fails to prevent neurologic problems, often especially with higher brain functions, and often with systems like motor and language.
A better explanation, again comes from network and complexity science. Studies of small world networks suggest that the problem is just more complex than that will break under our efforts to think through the problem, but rather requires the work of computers to deal with the massive parallel nature and complexity of the brain. Likely different nodes need to coordinate with different networks under different contexts in order to achieve specific goals.
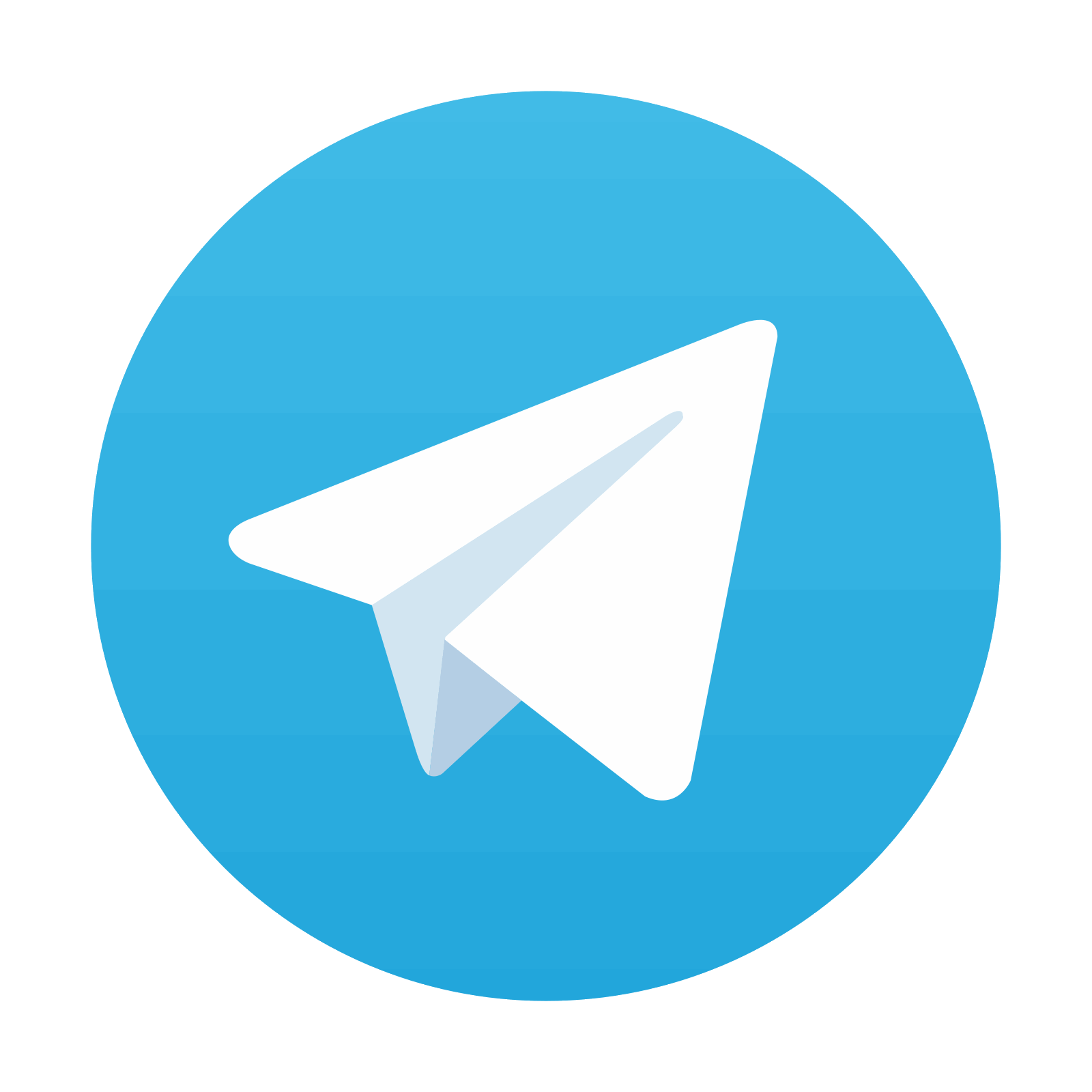
Stay updated, free articles. Join our Telegram channel

Full access? Get Clinical Tree
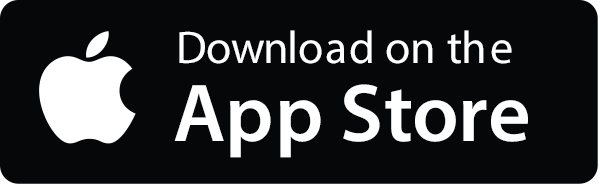
