(p.172) For the end positions, a slightly different equation is used, which takes into account the limit that perturbation can occur in only one direction. For position 1,


Recall begins with the cue in primary memory that has the highest probability of being the cue for the first item. The probability of sampling (P s) a particular secondary memory item, SM j, given a particular primary memory cue, PM i, depends of the ratio of the similarity of the cue and target relative to the similarity of the cue to all other possible responses:

In this equation, n is the number of potential responses and the similarity between the cue and the secondary memory item, s(i,j), is given by

In Equation 5, distance, d, is based on the number of mismatching features, M, divided by the number of compared features, N:

The value M k is the number of times the feature at position k in the cue does not match the feature at position k in the secondary memory item. The parameter a is a scaling parameter that can be mapped onto the overall level of attention or available resources.
After an item has been sampled, it still might not be output. The probability of outputting an item, P o, depends on the number of times it has been previously recalled, r, as well as a scale constant, c

Simulations
Given the relative lack of data, some simplifying assumptions will be made for the purpose of fitting the data. First, all simulations will use the same list length rather than simulating spanadjusted lengths. Secondly, it is assumed that older subjects will always recall slightly less in the ‘control’ condition (i.e., short words, dissimilar letters, quiet condition) than younger subjects. Third, the key measure will be relative difference, given the built-in absolute difference in the (p.173) control conditions. Thus, the five results to be simulated are the following: Relative to younger adults, older adults show (1) an equivalent effect of irrelevant speech; (2) an equivalent effect of concurrent articulation; (3) a larger acoustic confusion effect for auditory items but (4) a smaller acoustic confusion effect for visual items; and (5) an equivalent effect of word length for auditory items (the effect for visual items is unknown).
The basic strategy, as in other simulations with the Feature Model, is to begin with a standard set of parameters (see Table 10.2) to produce the simulated data for the young subjects, and then change only those parameters directly tied to the hypothesized difference between younger and older subjects to fit the data of the older adults. Thus, we start by simulating immediate serial recall of six item lists by younger subjects. To fit the data of the older subjects, we change two parameters. First, the probability of correctly encoding a particular modality-independent feature is reduced from 1.0 (for young adults) to 0.66 (for older adults). The idea is that the representations of older adults are noisier or less clear. Second, when auditory presentation is used, the probability of encoding a particular modality-dependent feature is reduced from 1.0 (for young adults) to 0.66 (for older adults). This reflects the common finding that most older adults have some degree of hearing loss relative to young adults. When visual presentation is being modeled, the probability of encoding a particular modality-dependent feature remains at 1.0, as most older adults have similar visual abilities as younger adults. Note that there is an overall difference in recall in all of the simulations below, and that this is due to the assumed worse modality-independent representation in the older subjects.
Irrelevant speech effect
The Feature Model offers a different explanation than that given by working memory for the four phenomena that are the focus of this chapter. The effects of irrelevant speech and concurrent
Table 10.2 Standard parameters for the simulations.
Young |
Old | |
---|---|---|
List length |
6 |
6 |
Probability of encoding each MI feature |
1.0 |
0.66 |
Probability of encoding each visual MD feature |
1.0 |
1.0 |
Probability of encoding each auditory MD feature |
1.0 |
0.66 |
Number of MI features |
20 |
20 |
Number of MD features: visual |
2 |
2 |
Number of MD features: auditory |
20 |
20 |
Number of similar MI features |
0 |
0 |
Number of similar MD features |
0 |
0 |
Attention (a) |
12.0 |
12.0 |
Probability of overwriting (F) |
1.0 |
1.0 |
Recovery constant (c) |
2.0 |
2.0 |
Number of perturbations |
3 |
3 |
Perturbation probability (theta) |
0.05 |
0.05 |
Number of simulations |
5000 |
5000 |
Table 10.3 Relative effect (Control-Experimental)/Control, for young and old subjects and simulation results from the feature model.
Source |
Young |
Old |
---|---|---|
Irrelevant speech effect |
||
Belleville et al. (2003) |
0.13 |
0.12 |
Model |
0.222 |
0.215 |
Concurrent articulation effect |
||
Rouleau and Belleville (1996) |
0.47 |
0.47 |
Model |
0.450 |
0.461 |
Acoustic confusion effect (auditory) |
||
Belleville et al. (1996) |
0.45 |
0.80 |
Model |
0.397 |
0.559 |
Acoustic confusion effect (visual) |
||
Belleville et al. (1996) |
0.42 |
0.34 |
Model |
0.414 |
0.419 |
Word length effect (auditory) |
||
Belleville et al. (1996) |
0.44 |
0.43 |
Model |
0.095 |
0.086 |
Word length effect (visual) |
||
Model |
0.196 |
0.181 |
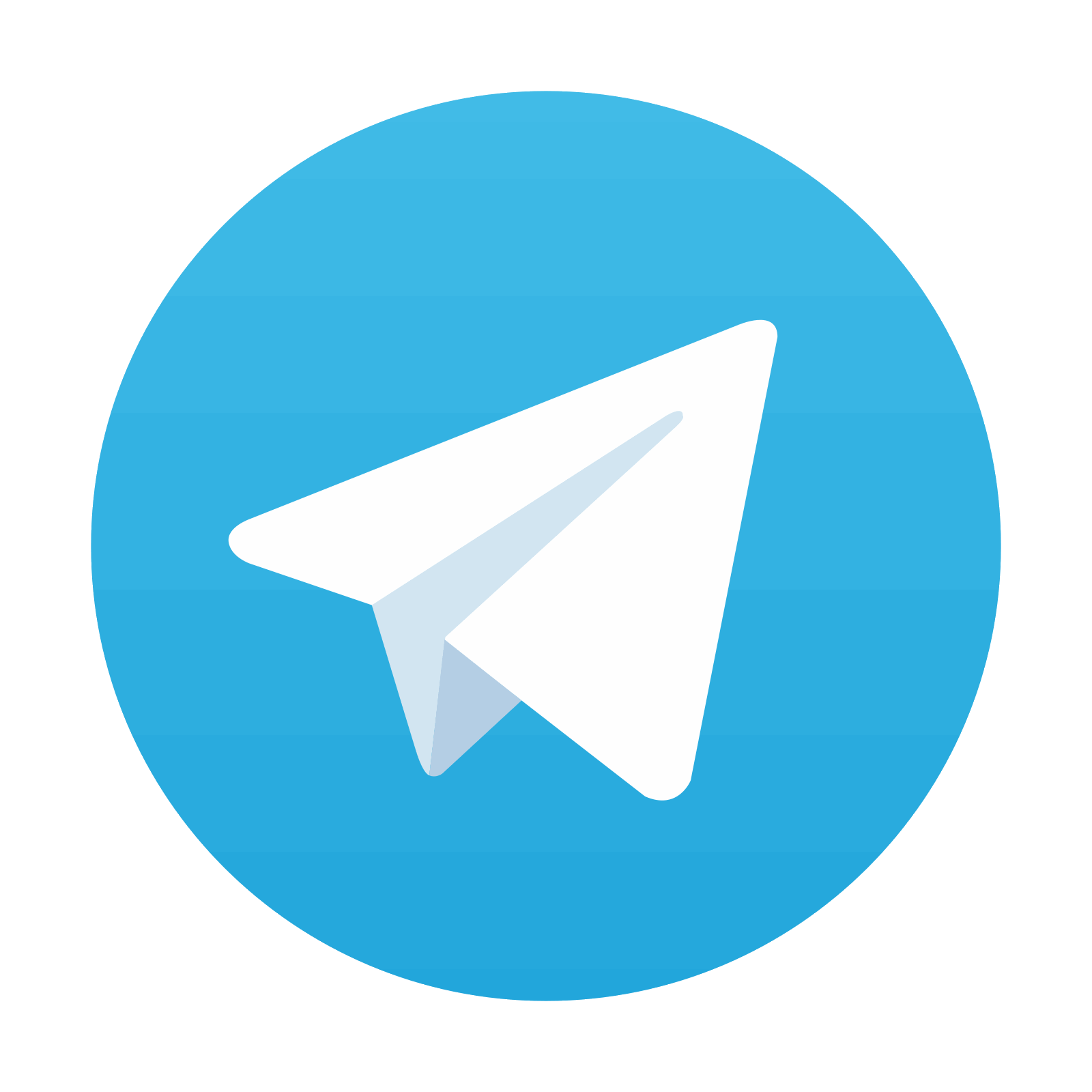
Stay updated, free articles. Join our Telegram channel

Full access? Get Clinical Tree
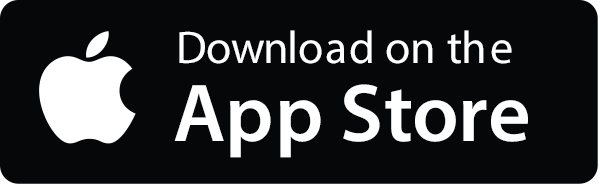
