Fig. 7.1
Hierarchical structure in the world is represented in a cortical hierarchy
This figure describes the predictive coding scheme associated with a simple hierarchical model shown on the left. In this model each node has a single parent. The ensuing inversion or generalised predictive coding scheme is shown on the right. The key quantities in this scheme are (conditional) expectations of the hidden states and causes and their associated prediction errors. The basic architecture – implied by the inversion of the graphical (hierarchical) model – suggests that prediction errors (caused by unpredicted fluctuations in hidden variables) are passed up the hierarchy to update conditional expectations. These conditional expectations now provide predictions that are passed down the hierarchy to form prediction errors. We presume that the forward and backward message passing between hierarchical levels is mediated by extrinsic (feedforward and feedback) connections. Neuronal populations encoding conditional expectations and prediction errors now have to be deployed in a canonical microcircuit to understand the computational logic of intrinsic connections – within each level of the hierarchy – as shown in Fig. 7.4 (From Bastos et al. 2012)
Conditional dependencies among hidden states and causes are responsible for generating sensory input. These dependencies mean that we can interpret neuronal activity as message passing among the nodes of a generative model, wherein each node contains a canonical microcircuit that represents expectations about hidden states and causes (see Sect. 7.3). We now look at how perception or model inversion – recovering the hidden states and causes of this model given sensory data – might be implemented at the level of a microcircuit:
In predictive coding, representations (or conditional expectations) generate top-down predictions to produce prediction errors. These prediction errors are then passed up the hierarchy in the reverse direction, to update conditional expectations. This ensures an accurate prediction of sensory input and all its intermediate representations. This hierarchical message passing can be expressed mathematically as a gradient descent on the (sum of squared) prediction errors
, where the prediction errors are weighted by their precision (inverse variance).



(7.1)
The first pair of equalities just says that conditional expectations about hidden causes and states
are updated based upon the way we would predict them to change – the first term – and subsequent terms that minimise prediction error. The second pair of equations simply expresses prediction error (ξ v (i), ξ x (i)) as the difference between conditional expectations about hidden causes and (the changes in) hidden states and their predicted values, weighed by their precisions (Π v (i), Π x (i)). These predictions are nonlinear functions of conditional expectations (g (i), f (i)) at each level of the hierarchy and the level above (levels denoted by the superscript ‘i’).

In neuronal network terms, Eq. (7.1) says that prediction error units receive messages from the same level and the level above. This is because the hierarchical form of the model only requires conditional expectations from neighbouring levels to form prediction errors, as can be seen schematically in Fig. 7.1. Conversely, expectations are driven by prediction error from the same level and the level below – updating expectations about hidden states and causes respectively. These constitute the bottom-up and lateral messages that drive conditional expectations to provide better predictions – or representations – that suppress prediction error. This updating corresponds to an accumulation of prediction errors – in that the rate of change of conditional expectations is proportional to prediction error. This is the essence of recurrent message passing between hierarchical levels to suppress prediction error (see Friston (2008) for a more detailed discussion).
7.2 Predictive Coding and Extrinsic Connection Properties in Sensory and Motor Areas
The brain can minimise prediction error in one of two ways. It can either change its predictions to better cohere with sensory input, or change the sampling of the environment such that sensory samples conform to predictions. The former process corresponds to perceptual inference – discussed in the previous section as predictive coding – the latter to action: together, they constitute ‘active inference’ (Friston et al. 2010). The free energy principle thus dictates that the perceptual and motor systems should not be regarded as separate but instead as a single active inference machine that tries to predict its sensory input in all domains: visual, auditory, somatosensory, interoceptive and, in the case of the motor system, proprioceptive.
Descending messages in the somatomotor system might therefore be best characterized as predictions of proprioceptive input and not motor commands. These proprioceptive predictions should not be corrected but fulfilled, by the automatic peripheral transformation of proprioceptive prediction errors into movement, by classical reflex arcs (see also Fig. 7.2a). If both systems are minimising prediction error, descending hierarchical projections in the motor cortex should share the laminar, topographic and physiological characteristics of backward connections in exteroceptive (sensory) systems.




Fig. 7.2
Connections in the somatomotor hierarchy: functional and laminar characteristics
(a) This simplified schematic ignores the contributions of spinal circuits and subcortical structures; and omits many hierarchical levels (especially on the sensory side). M1, S1, M2 and S2 signify primary and secondary motor and sensory cortex, while As signifies prefrontal association cortex. Dashed arrows denote driving ‘forward’ projections, and unbroken arrows modulatory ‘backward’ projections. Afferent somatosensory projections are dotted. α-MN and γ-MN signify alpha- and gamma motor neuron output (From Adams et al. 2013)
(b) The diagram shows patterns of extrinsic projection terminations in selected areas comprising the somatomotor hierarchy. Not all connections are shown, only those for which an adequate indication of laminar characteristics is obtainable. The relevant citations (corresponding to the bracketed numbers) and a diagram of the patterns of projection origins have been omitted due to space constraints: they can be found in Adams et al. (2013). In order to compile data across studies with variable terminology and placement of injected tracers, or with similar outcomes, some areas are combined into single blocks; the ‘&’ symbol should be interpreted as ‘and/or’. The diagrams are intended to give an indication of forward or backward relationships, but not the precise number of pathways or levels involved. The sensory tiers, for instance, are compressed into a single level: S1, shown as a single block, comprises four separate areas (3a, 3b, 1 and 2) that precede higher order parietal areas in a sensory hierarchy. The figure depicts schematic illustrations of terminal patterns – forward (2, 3, 13 & 20); intermediate (4, 5, 6, 11 & 21); and backward (1, 7–10, 12 & 14–19). Forward patterns have a concentration in layer 3. Intermediate patterns are described as columnar, with little or no laminar differentiation. Backward patterns are concentrated in layers 1 (and 6) and/or tend to avoid the lower part of layer 3. Feedback from M1 to S1 tends to avoid layer 4 (This figure is updated from Shipp (2005) and taken from Adams et al. (2013))
A brain implementing predictive coding ought to have particular attributes (Friston 2005). These include: (i) a hierarchical organization with reciprocal connections between areas (conveying predictions and prediction errors) that are (ii) topographically asymmetrical (predictive connections being more divergent because a high level cause has multiple sensory consequences) and (iii) functionally asymmetrical. The functional asymmetry is important because descending predictions have to embody nonlinearities in the generative model (e.g., to model visual occlusion) that require them to interact or modulate each other, whereas ascending connections that drive higher representations do not.
We now review these attributes of the architecture of extrinsic connections in sensory and motor systems in the brain.
7.2.1 Hierarchical (Laminar) Characteristics
The cerebral neocortex consists of six layers of neurons, defined by differences in neuronal composition (pyramidal or stellate excitatory neurons, and numerous inhibitory classes) and packing density (Shipp 2007). Layer 4 is known as the ‘granular layer’ (due to its appearance), and the layers above and below it are known as ‘supragranular’ and ‘infragranular’ respectively.
Felleman and Van Essen (1991) surveyed 156 corticocortical pathways and specified criteria by which projections could be classified as forward, backward or lateral. They defined forward projections as originating predominantly (i.e. >70 % cells of origin) in supragranular layers, or occasionally with a bilaminar pattern (meaning <70 % either supra- or infragranular, but excluding layer 4 itself). Forward projections terminate preferentially in layer 4. Backward projections are predominantly infragranular or bilaminar in origin with terminations in layers 1 and 6 (especially the former), and always evading layer 4.
Felleman and Van Essen’s (1991) analysis included some somatomotor areas but concentrated on sensory – especially visual – hierarchies. Continuing work by Shipp (2005), we have recently performed a more fine grained analysis of projections in somatomotor areas (Adams et al. 2013), of which the major findings are as follows:
(a)
The terminations of projections ascending the somatomotor hierarchy are intermediate in character (terminate in all layers) apart from those originating in the sensory areas of parietal cortex, which have the characteristics of forward projections.
(b)
The terminations of projections descending the somatomotor hierarchy have an overall backward character. The pattern is notably more distinct for terminations within postcentral granular areas, but the available evidence leans toward a backward pattern in the precentral agranular areas (i.e. from secondary to primary motor cortices) as well. Projection termination patterns are illustrated in Fig. 7.2b.
(c)
The origins of projections ascending or descending the somatomotor hierarchy are qualitatively similar to each other; the projecting neurons are typically described as bilaminar and equally dense in layers 3 and 5, or as predominating in layer 3. However, the origin of ascending projections within the somatomotor hierarchy may be characterised by a higher superficial : deep ratio than the origin of descending connections, even if both ratios are above one (Shipp 2005). This is true for (i) projections to M1 from S1 versus premotor cortex (PMd), and (ii) projections to PMd from M1 versus rostral frontal cortex. The ratio of ratios device may depart from the original test criteria but as Felleman and Van Essen (1991) point out: “the key issue is whether a consistent hierarchical scheme can be identified using a modified set of criteria”.
In short, there is clear hierarchical organisation in both sensory and motor systems, with descending (predictive) connections in particular in both sensory and motor systems sharing laminar properties.
7.2.2 Topographic Characteristics
Salin and Bullier (1995) reviewed a large body of evidence concerning the microscopic and macroscopic topography of corticocortical connections, and how these structural properties contribute to their function; e.g. their receptive fields. In cat area 17, for example, <3 % of forward projecting neurons have axons which bifurcate to separate cortical destinations. Conversely, backward projections to areas 17 and 18 include as much as 30 % bifurcating axons (Bullier et al. 1984; Ferrer et al. 1992). A similar relationship exists in visual areas in the monkey (Salin and Bullier 1995).
Rockland and Drash (1996) contrasted a subset of backward connections from late visual areas (TE and TF) to primary visual cortex with typical forward connections in the macaque. The forward connections concentrated their synaptic terminals in 1–3 arbours of around 0.25 mm in diameter, whilst backward connections were distributed over a “wand-like array” of neurons, with numerous terminal fields stretching over 4–10 mm, and in one case, 21 mm (Fig. 7.3a). This very diffuse pattern was only found in around 10 % of backward projections, but it was not found in any forward projection.


Fig. 7.3
Topographic characteristics of forward and backward projections
(a) This schematic is adapted from Rockland and Drash (1996), and illustrates the terminal fields of ‘typical’ forward (axon FF) and backward (axon FB) connections in the visual system. IG represents infragranular collaterals of a backward connection, and ‘ad’ an apical dendrite; cortical layers are labelled on the left. Note the few delimited arbours of terminals on the forward connection, and the widely distributed “wand-like array” of backward connection terminals
(b) This schematic illustrates projections to and from a lower and higher level in the visual hierarchy (Adapted from Zeki and Shipp 1988). Dashed arrows signify forward connections and continuous arrows backward connections. Note that there is a much greater convergence (from the point of view of neurons receiving projections) and divergence (from the point of view of neurons sending projections) in backward relative to forward connections (From Adams et al. 2013)
These microscopic properties of backward connections reflect their greater macroscopic divergence. Zeki and Shipp (1988) reviewed forward and backward connections between areas V1, V2 and V5 in macaques, and concluded that backward connections showed much greater convergence and divergence than their forward counterparts (Fig. 7.3b). This means that cells in higher visual areas project back to a wider area than that which projects to them, and cells in lower visual areas receive projections from a wider area than they project to. Whereas forward connections are typically patchy in nature, backward connections are more diffuse (Salin and Bullier 1995; Shipp and Zeki 1989a, b). These attributes mean that visuotopy preserved in the forward direction is eroded in the backward direction, allowing backward projections to contribute significantly to the extra classical receptive field of a cell (Angelucci and Bullier 2003).
Salin and Bullier (1995) also noted that in the macaque ventral occipitotemporal pathway (devoted to object recognition), backward connections outnumber forward connections. Forward projections from the lateral geniculate nucleus (LGN) to V1 are outnumbered 20 to 1 by those returning in the opposite direction; and backward projections outweigh forward projections linking central V1 to V4, TEO to TE, and TEO and TE to parahippocampal and hippocampal areas.
Now let us examine corticocortical connections within the sensorimotor system. Studies using dual retrograde tracers have examined the sources of input to the parts of M1 innervating the distal and proximal parts of the monkey forelimb (Tokuno and Tanji 1993) and hindlimb (Hatanaka et al. 2001), and showed that divergence in the descending (motor) input to M1 exceeds divergence in the ascending (sensory) input to M1.
In a later study, Dancause et al. (2006) found that: (a) the distal forelimb part of premotor (PMv) connects with both distal and proximal forelimb parts of M1, demonstrating descending divergence similar to the other motor areas noted above; (b) the termination of the descending projection to M1, while patchy, was broader than the territory occupied by source neurons for the ascending projection, thus replicating the kind of pattern noted previously in visual cortex (Fig. 7.3b).
7.2.3 Physiological Characteristics
Forward and backward connections in sensory systems have typically been associated with ‘driving’ and ‘modulatory’ characteristics, respectively, though the latter physiological duality has lacked the empirical clarity of its anatomical counterpart, particularly for cortical interactions.
Recent evidence, however, suggests that feedback connections do more than modulate lower level responses: Sherman and colleagues recorded cells in mouse area V1/V2 and A1/A2, while stimulating feedforward or feedback afferents. In both cases, driving-like responses as well as modulatory-like responses were observed (Covic and Sherman 2011; De Pasquale and Sherman 2011). This indicates that – for these hierarchically proximate areas – feedback connections can drive their targets just as strongly as feedforward connections. Imaging studies of top-down influences acting on area V1 imply that backward connections can sustain or even initiate activity, in the absence of a retinal signal (e.g. Harrison and Tong 2009; Muckli et al. 2005). In summary, backward connections can mediate modulatory and driving effects. This is important from the point of view of predictive coding because, as noted above, top-down predictions have to drive cells that explain away prediction error. From a computational perspective, the key role of modulatory effects is to model the context-sensitive and nonlinear way in which causes interact to produce sensory consequences.
We now consider evidence linking nonlinear (modulatory) effects to backward connections in both sensory and motor systems, much of which depends on a closer consideration of the roles played by the different types of postsynaptic glutamate receptors:
Glutamate is the principal excitatory neurotransmitter in the cortex and activates both ionotropic and metabotropic receptors. Metabotropic receptor binding is clearly modulatory in action (Pin and Duvoisin 1995). Ionotropic glutamate receptors are classified according to their AMPA, Kainate and NMDA agonists (Traynelis et al. 2010). AMPA activation is fast and stereotyped, with onset times <1 msec, and deactivation within 3 msec; recombinant Kainate receptors have AMPA receptor-like kinetics, although they can be slower in vivo. NMDA currents, by contrast, are smaller but more prolonged: the onset and deactivation are one and two orders of magnitude slower, respectively.
Unlike non-NMDA receptors, NMDA receptors (NMDA-Rs) are both ligand-gated and voltage-dependent – to open their channel they require both glutamate binding and membrane depolarisation to displace the blocking Mg2+ ion. The voltage dependence makes NMDA transmission non-linear and the receptors function, in effect, as postsynaptic coincidence detectors. These properties may be particularly important in governing the temporal patterning of network activity (Durstewitz 2009). Once activated, NMDA-Rs play a critical role in changing long term synaptic plasticity (via Ca2+ influx) and increase the short-term gain of AMPA/Kainate receptors (Larkum et al. 2004). In summary, NMDA-Rs are nonlinear and modulatory in character, whereas non- NMDA-Rs have more phasic, driving properties.
NMDA-Rs are ubiquitous in distribution, and clearly participate in forward, intrinsic and backward signal processing. Their subunit composition differs, however, and there is evidence in the macaque sensory cortex that the most non-linear variants are densest in the layers receiving backward connections (Muñoz et al. 1999). Predictive coding requires descending non-linear predictions to negate ascending prediction errors, and interestingly it seems that the inhibitory effects of backward projections to macaque V1 are mediated by NR2B-containing NMDA-R’s (Self et al. 2012). By contrast, the least non-linear NMDA-R variants are found in layer 4 of macaque area 3B (Muñoz et al. 1999) and of rodent S1 (Binshtok et al. 2006).
Studies with pharmacological manipulation of NMDA-R in vivo are rare. However application of an NMDA-R agonist to cat V1 raised the gain of response to stimulus contrast (Fox et al. 1990). The effect was observed in all layers, except layer 4. Application of an NMDA-R antagonist had the reverse effect, however the gain-reduction effect was only observed in layers 2 and 3. To interpret these results, the NMDA-R agonist may have simulated a recurrent enhancement of responses in the layers exposed to backward connections (i.e. all layers save layer 4). The restriction of the antagonist effect to layer 2/3 could indicate that NMDA-R plays a more significant role in nonlinear intrinsic processing in these layers.
NMDA-Rs in motor cortices have similar anatomical and functional properties. Zilles et al. (1995) demonstrated that human motor cortex has the same distribution of NMDA and non- NMDA-Rs as is found elsewhere in the brain: the former are concentrated in supragranular layers, whereas the latter have a uniform (AMPA-R) or infragranular (KA-R) distribution.
Ghosh et al. (1987) counted the relative numbers of neurons projecting to monkey forelimb M1. In the 3 animals they examined, 11–31 % of neurons projecting to M1 came from premotor cortex (lateral area 6), whereas 1–17 % of neurons originated in area 5 (higher sensory cortex). Ghosh and Porter (1988) then stimulated these two cortical areas using surface electrodes, and recorded EPSP’s and IPSP’s in M1. They found that despite the bias in numbers towards descending projections, stimulation of area 5 neurons elicited responses in 90 % of recorded M1 neurons, whereas the same stimulation of premotor cortex caused only 30 % of recorded M1 neurons to respond. One can infer from this that ascending projections from sensory cortex are the more driving in character, despite their lesser number. Likewise, it is known that inactivation of M1 has a more significant effect on the activity in PMv and SMA than vice versa (Schmidlin et al. 2008), which one would expect if descending connections to M1 were more modulatory, and ascending connections from M1 more driving in character.
What of the receptor types mediating these connections? By administering receptor antagonists, Shima and Tanji (1998) showed that the influence of SMA over M1 depends to a much greater extent upon NMDA-R transmission, whilst the ascending connections from S1 to M1 rely more heavily on AMPA or Kainate receptors.
Shima and Tanji (1998) speculated that SMA – via NMDA-R’s – might modulate the gain of driving S1 inputs to M1. Evidence for higher motor areas modulating the gain of M1 neurons has actually been provided by Shimazu et al. (2004), who recorded corticospinal outputs following stimulation of the ventral premotor area (F5) and/or M1. M1 stimulation alone evoked several corticospinal volleys, whereas F5 stimulation alone evoked minimal output. If F5 stimulation directly preceded that of M1, however, the later corticospinal volleys were powerfully facilitated, as were the resulting EPSE’s in 92 % of intrinsic hand motor neurons. Similarly, Zagha et al. (2013) have shown in the mouse that descending connection from primary motor cortex can modulate responses in primary sensory cortex according to context.
In summary, predictive coding requires forward (prediction error) connections to be driving and backward (predictive) connections to have both driving and non-linear modulatory properties. This pattern is seen in sensory and motor cortices in both the anatomical distribution of non-linear NMDA-Rs (which are particularly present in lamina to which backward connections project), and in the responses of neurons to electrophysiological stimulation.
7.3 Section 3: Predictive Coding and Intrinsic Connections of the ‘Canonical Microcircuit’
In this section, we show how the equations describing hierarchical message passing within a predictive coding scheme in Sect. 7.1 can be mapped on to key features of intrinsic connections, namely the canonical cortical microcircuit.
7.3.1 Microcircuits in the Visual Cortex
The seminal work of Douglas and Martin (1991), in the cat visual system, produced a model of how information flows through the cortical column. Their model contained superficial and deep pyramidal cells with a common pool of inhibitory cells, and was able to explain how recurrent connections between the recorded cell populations gave rise to their observed data, in terms of intracellular membrane potentials. Their circuit, although based on recordings from cat visual cortex, was also proposed as a basic theme that might be present and replicated, with minor variations, throughout the cortical sheet (Douglas et al. 1989).
Subsequent studies have used intracellular recordings and histology to measure spikes (and intracellular membrane potentials) in pre and post-synaptic cells, whose cellular morphology can be determined. This approach quantifies both the connection probability – defined as the number of observed connections divided by total number of pairs recorded – and connection strength – defined in terms of post-synaptic responses. Thomson et al. (2002) used these techniques to study layers 2 to 5 (L2 to L5) of the cat and rat visual systems. The most frequently connected cells were located in the same cortical layer, where the largest interlaminar projections were the ‘feedforward’ connections from L4 to L3 and from L3 to L5. Excitatory reciprocal ‘feedback’ connections were not observed (L3 to L4) or less commonly observed (L5 to L3), suggesting that excitation spreads within the column in a feedforward fashion. Feedback connections were typically seen when pyramidal cells in one layer targeted inhibitory cells in another (see Thomson and Bannister 2003 for a review).
Recent advances in optogenetics have also made it possible to more easily study inhibitory cells: Kätzel et al. (2011) combined optogenetics and whole-cell recording to investigate the intrinsic connectivity of inhibitory cells in mouse cortical areas M1, S1, and V1. They transgenically expressed channelrhodopsin in inhibitory neurons and activated them, while recording from pyramidal cells. This allowed them to assess the effect of inhibition as a function of laminar position relative to the recorded neuron.
Several conclusions can be drawn from this approach (Kätzel et al. 2011): first, L4 inhibitory connections are more restricted in their lateral extent, relative to other layers. This supports the notion that L4 responses are dominated by thalamic inputs, while the remaining laminae integrate afferents from a wider cortical patch. Second, the primary source of inhibition originates from cells in the same layer, reflecting the prevalence of inhibitory intralaminar connections. Third, several interlaminar motifs appeared to be general – at least in granular cortex: principally, a strong inhibitory connection from L4 onto supragranular L2/3 and from infragranular layers onto L4. Figure 7.4 provides a summary of key excitatory and inhibitory intralaminar connections.
7.3.2 Microcircuits in the Sensorimotor Cortex
Do the features of visual microcircuits generalize to other cortical areas? Recently, two studies have mapped the intrinsic connectivity of mouse sensory and motor cortices: Lefort et al. (2009) used multiple whole-cell recordings in mouse barrel cortex to determine the probability of monosynaptic connections – and the corresponding connection strength. As in visual cortex, the strongest connections were intralaminar and the strongest interlaminar connections were the ascending L4 to L2, and descending L3 to L5.
One puzzle about canonical microcircuits is whether motor cortex has a local circuitry that is qualitatively similar to sensory cortex. This question is important because motor cortex lacks a clearly defined granular L4. Weiler et al. (2008) combined whole-cell recordings in mouse motor cortex with photo-stimulation to uncage Glutamate, and mapped the excitatory influence that each layer exerts over the others. They found that the L2/3 to L5A/B was the strongest connection – accounting for one-third of the total synaptic current in the circuit. The second strongest interlaminar connection was the reciprocal L5A to L2/3 connection. This pathway may be homologous to the prominent L4/5A to L2/3 pathway in sensory cortex. Also – as in sensory cortex – recurrent (intralaminar) connections were prominent, particularly in L2, L5A/B and L6. The largest fraction of synaptic input arrived in L5A/B, consistent with its key role in accumulating information from a wide range of afferents – before sending its output to the corticospinal tract. In summary, strong input-layer to superficial, and superficial to deep connectivity, together with strong intralaminar connectivity, suggests that the intrinsic circuitry of motor cortex is similar to other cortical areas.
7.3.3 Predictive Coding in the Canonical Microcircuit
Recently, we showed that the variables shown in Eq. (7.1) (Sect. 7.1) can be associated with specific populations in the laminar circuit (Bastos et al. 2012). The proposed mapping in Fig. 7.4 illustrates a remarkable correspondence between the form of Eq. (7.1) and the connectivity of the canonical microcircuit. Furthermore, the resulting scheme corresponds almost exactly to the computational architecture proposed by Mumford (1992).


Fig. 7.4
A canonical microcircuit for predictive coding
The upper panel is the canonical microcircuit based on Haeusler and Maass (2007), where we have removed inhibitory cells from the deep layers – because they have very little interlaminar connectivity. The numbers denote connection strengths (mean amplitude of PSPs measured at soma in mV) and connection probabilities (in parentheses) according to Thomson et al. (2002). Excitatory and inhibitory populations are indicated as E and I, respectively. The lower panel shows the proposed cortical microcircuit for predictive coding, where the quantities from Fig. 7.1 have been associated with various cell types. Here, extrinsic connections are shown in thick lines. Intrinsic connections are shown in thinner lines. Prediction error populations are the populations encoded by ξ v (i), ξ x (i), and populations encoding conditional expectations are encoded by
. Intrinsic inhibitory populations are shown in light gray, while excitatory populations are shown in white. The dotted lines refer to connections that are not present in the microcircuit on the left. In this scheme, expectations (about causes and states) are assigned to (excitatory and inhibitory) interneurons in the supragranular layers, which are passed to infragranular layers. The corresponding prediction errors occupy granular layers, while superficial pyramidal cells encode prediction errors that are sent forward to the next hierarchical level. Conditional expectations and prediction errors on hidden causes are associated with excitatory cell types, while the corresponding quantities for hidden states are assigned to inhibitory cells. Circles with dark outlines indicate pyramidal cells. Finally, we have placed the precision of the feedforward prediction errors against the superficial pyramidal cells. This quantity controls the postsynaptic sensitivity or gain to (intrinsic and top-down) pre-synaptic inputs. We have previously discussed this in terms of attentional modulation, which may be intimately linked to the synchronisation of pre-synaptic inputs and ensuing postsynaptic responses (Fries et al. 2001; Feldman and Friston 2010) (From Bastos et al. 2012)

The architecture bears a striking correspondence to the microcircuit in Haeusler and Maass (2007) in the left panel of Fig. 7.4 – in the sense that nearly every connection required by the predictive coding scheme appears to be present in terms of quantitative measures of intrinsic connectivity. However, there are two exceptions that both involve connections to the inhibitory cells in the granular layer (shown as dotted lines in Fig. 7.4). Predictive coding requires that these cells (that encode prediction errors on hidden states) compare the expected changes in hidden states with the actual changes. This suggests that there should be interlaminar projections from supragranular (inhibitory) and infragranular (excitatory) cells. In terms of their synaptic characteristics, one would predict that these intrinsic connections would be of a feedback sort – in the sense that they convey predictions. Although the infragranular to granular connection was not included in the Haeusler and Maass circuit, this is likely due to the omission of layer 6 from their analysis, as feedback connections from layer 6 to layer 4 are an established component of the cortical microcircuit (e.g., Usrey and Fitzpatrick 1996; Olsen et al. 2012).
7.3.4 Functional Asymmetries in the Microcircuit and Oscillatory Dynamics
The circuitry in Fig. 7.4 appears consistent with the broad scheme of ascending (feedforward) and descending (feedback) intrinsic connections: feedforward prediction errors from a lower cortical level arrive at granular layers and are passed forward to excitatory and inhibitory interneurons in supragranular layers, encoding expectations. Strong and reciprocal intralaminar connections couple superficial excitatory interneurons and pyramidal cells. Excitatory and inhibitory interneurons in supragranular layers then send strong feedforward connections to the infragranular layer. These connections enable deep pyramidal cells and excitatory interneurons to produce (feedback) predictions, which ascend back to L4 or descend to a lower hierarchical level. This arrangement recapitulates the functional asymmetries between extrinsic feedforward and feedback connections and is consistent with the empirical characteristics of intrinsic connections. Note, however, that deviations from this circuitry (e.g., activation of the cortical column independent of layer 4: Constantinople and Bruno 2013) do exist and may play important roles in predictive coding and canonical cortical computations, but it is beyond the scope of this chapter to review all such exceptions. What we wish to highlight here is the remarkable correspondence between the theoretically predicted microcircuit and the currently established models of intrinsic cortical processing.
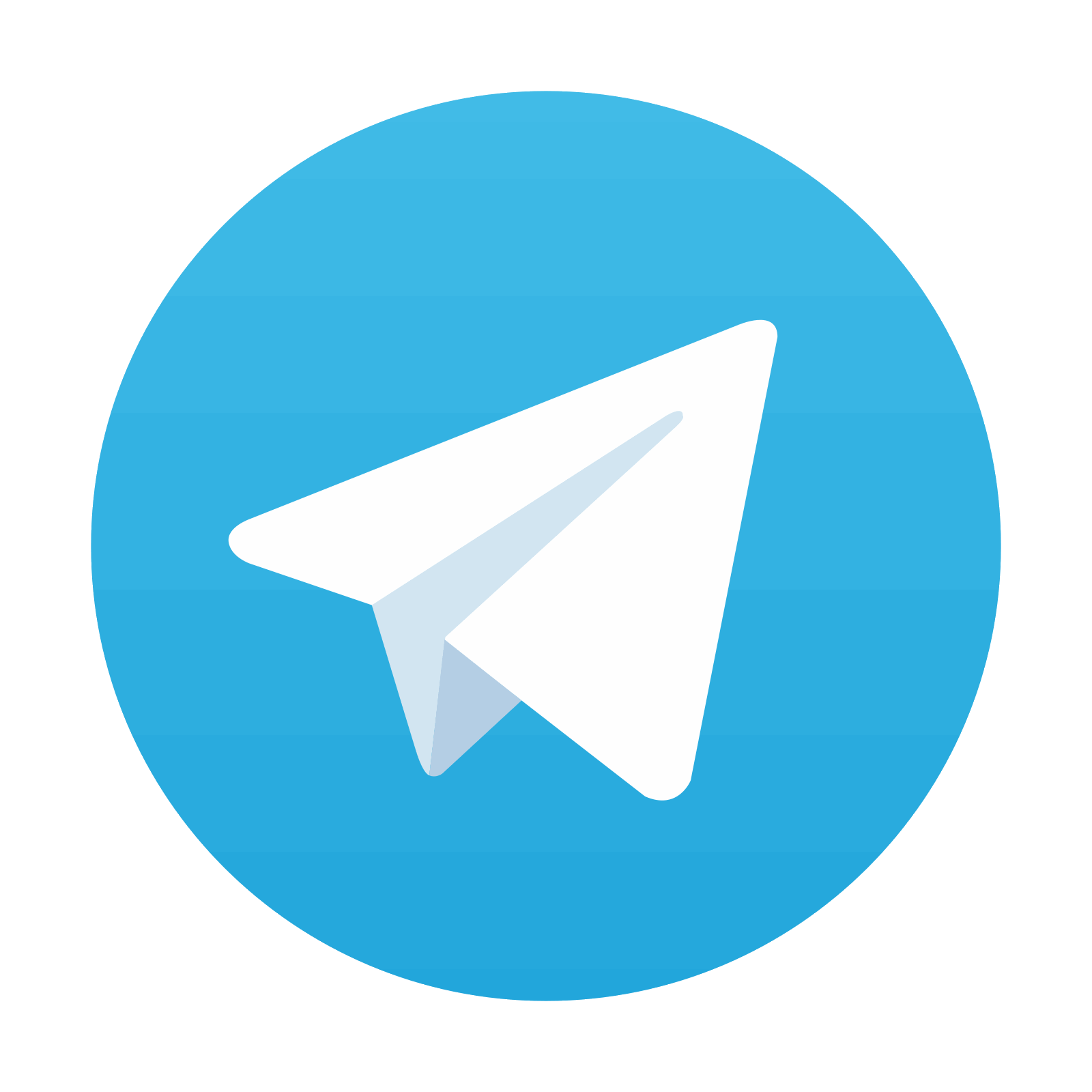
Stay updated, free articles. Join our Telegram channel

Full access? Get Clinical Tree
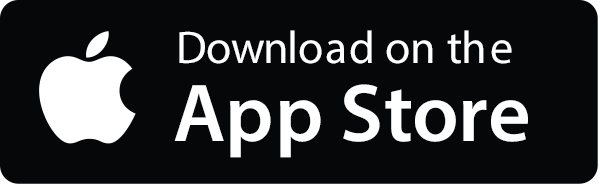
