Fig. 16.1
Rapid adaptation across cortical layers in V1. (a) Schematic description of the orientation adaptation protocol. (b) Multi-contact laminar electrodes were used to record neuronal activity across cortical depth (left). Current-source density analysis (based on the second spatial derivate of the LFP time-series) was used to identify the polarity inversion accompanied by the sink-source configuration at the base of the granular layer. The CSD traces represent the average of those contacts assigned to a given layer. (c) Receptive fields across contacts were mapped using oriented stimuli presented in random patches. Firing rates for each neuron are calculated independently at 5 ms intervals and the maximum firing rates (shown as red) were used to computed the centroid for each time delay
We observed that cells recorded on laminar probes had highly overlapping receptive fields (Fig. 16.1c) and highly similar preferred orientations (PO) (e.g., the difference in PO for over 58 % of the pairs of neurons was within 0°–10° range, p < 0.01, Wilcoxon signed-rank test).
16.2.2 Measures of Neuronal Synchronization
We used multi-taper spectral analysis to compute spike-field coherence (SFC), which measures the degree of synchronization between individual neurons and local population activity (LFPs) for each specific frequency band. In general, the coherence between two signals (x and y) recorded at different sites is a complex quantity whose magnitude is a measure of the phase synchrony for frequency f. Coherence is an absolute value that varies between 0 and 1 (e.g., a value of 1 indicates a perfect phase relationship between the firing of the spikes to the fluctuations of the LFP). Coherence is defined as
where S x (f) and S y (f) represent the auto-spectra and S yx (f) the cross-spectrum of the two signals x and y. Auto-spectra and cross-spectra are averaged across trials before the coherency calculation (Womelsdorf et al. 2006; Gregoriou et al. 2009). In an attempt to eliminate any bias from differing sample sizes, the same number of trials for each condition (adaptation and control) was used for the calculation of coherence for a given pair. Importantly, the length of temporal window included in each condition was also constant. Specifically, we utilized the Chronux function coherencycpt which computes the multi-taper spike-field coherence for a continuous signal (LFP) and point process data (spike-train) according to an optimal family of orthogonal tapers derived from Slepian functions (Mitra and Pesaran 1999; Pesaran et al. 2002). The number of tapers was calculated according to the formula:
where K is the highest number of tapers that can be used while preserving optimal time-frequency concentration of the data windowing available from the Slepian taper sequences, T is the length of the temporal window in seconds, and W is the half-bandwidth of the multi-taper filter. For our analysis we applied spectral smoothing of ±10 Hz for frequencies greater than 30 Hz and ±4 Hz for lower frequencies.


16.2.3 Correlated Variability
Multiple single-unit recordings were performed from V1 of two fixating monkeys (Macaca mulatta). Stimuli were presented such as to cover the center of the neurons’ receptive fields. In control trials, movie strips were presented for ~1.86 s (16 orientations × 7 repeats at 60 Hz; random spatial phase). In adaptation trials, movies were preceded by a 400-ms grating of fixed orientation. The Pearson correlation coefficient of spike counts, R sc , of two cells is defined as:
where N is the number of trials, r j i is the firing rate of cell j in trial i averaged over the entire stimulus sequence, and σ is standard deviation of the responses. Correlation coefficients after adaptation depend on three variables: the adapting orientation, ϕ a , and the preferred orientation of the cells in a pair, θ 1 and θ 2. To ensure that the parameter space is adequately sampled, the distance between the adapting orientation and the preferred orientation of one of the cells was held constant while varying the relative difference between the two cells’ preferred orientations (Δθ).

16.3 Results
16.3.1 Adaptation Increases Spike-LFP Gamma Synchronization
We used multi-contact laminar electrodes (Plextrode® U-Probe, Plexon Inc.) to record neuronal activity at 20 V1 recording sites, each measured at 16 different depths, while two monkeys (W: 13; P: 7) performed a rapid adaptation fixation task (Fig. 16.1a). While animals fixated a white dot at the center of a screen, an adapting stimulus was flashed for 300 ms in the center of the neurons’ receptive field. After a 100 ms blank, a test stimulus of random orientation (8 equally spaced orientations spanning 0–180°) was presented for 300 ms. The adapting stimulus was either a random dot patch (control condition) or a sine-wave grating with spatial characteristics identical to those of the test stimulus, but fixed in orientation within 45° of each cell’s preferred orientation (adaptation condition).
In control trials, the presentation of the test stimulus led to pronounced synchronous activity across all cortical layers, with the most significant increase in gamma synchronization in the granular layers (Fig. 16.2a). However, after adaptation, there was a significant increase in gamma synchronization specifically in the supragranular layers (Fig. 16.2b). Our population analysis confirms these results – before adaptation we found spike-LFP gamma synchronization across all layers (mean + S.E.M; SG: 0.08 + 0.002; G: 0.12 + 0.007; IG: 0.08 + 0.006) with the largest SFC level in the granular layers (one-way ANOVA, F (2, 74) = 8.75, P = 0.0004; post-hoc multi-comparison, Tukey’s Least Significant Difference).


Fig. 16.2
Example of synchronization, measured as spike-field coherence as a function of frequency, across cortical layers during control and adaptation across cortical depth during control (left) and adaptation (right). During the presentation of the control stimuli there is an increase in gamma activity in the granular layer (Adaptation increases SFC across cortical layers with the largest increase in the supragranular layer. Dashed lines equal the granular layer)
We also calculated the percentage change in gamma SFC between adaptation and control (ΔSFC) across the entire frequency range and found a significant increase in synchronization for the supragranular layer (68.91 % + 6.75 %; Fig. 16.3a, b; one-way ANOVA, F (2, 74) = 35.24, P = 1.77 10−11; post-hoc multi-comparison, Tukey’s Least Significant Difference). The post-adaptation increase in gamma SFC in the supragranular layers was observed only when both recording sites were stimulated with test stimuli within 45° of the cells’ preferred orientation. Non-optimal test orientations reduced spike rates, LFP amplitudes, and SFC in the control condition; adaptation at these orientations did not result in a significant increase in gamma SFC (P = 0.20, Wilcoxon signed-rank test).


Fig. 16.3
Adaptation influences synchronization between individual neurons and local populations in a layer-specific manner. (a, top) Population analysis during the presentation of the control stimulus results in a significant increase in SFC between 30 and 80 Hz in the granular layer. (a, bottom) Adaptation increases SFC in the supragranular layer for all frequency bands between 0 and 80 Hz, with the largest increase in the gamma-band (30–80 Hz; shaded regions represent S.E.M.) (b) We calculated the percentage change between adaptation and control across the entire frequency range and observed a significant increase in gamma-band spike-field coherence for the supragranular layer (shaded regions represent S.E. for percentage change). (c) Scatter plot showing the effects of adaptation on neuronal discrimination performance (d′) at the population level across cortical layers. Each dot represents the mean d′ during control and adaptation, while the different colors indicate the layer in which the neuron was isolated. Across the total population of cells (n = 77), adaptation significantly increases orientation discriminability. Inset: Although adaptation significantly increases d′ across all cortical layers, the largest increase occurred in the supragranular layer. (d) There is a significant and positive correlation between the gamma-band spike-field coherence after adaptation and the change in d′ that is specific to the supragranular layers. The analysis of lower frequency bands (<30 Hz) does not reveal a statistically significant correlation between the post-adaptation change in SFC and the change in d′
16.3.2 Relationship Between Gamma Synchronization and Neuronal Discrimination
We further investigated whether the ability of neurons in different cortical layers to discriminate stimulus orientation (Fig. 16.3c) is influenced by the post-adaptation change in synchronization between individual cells and their local population (Fig. 16.3d). We addressed this issue by examining the relationship between the post-adaptation change in gamma-band spike-field coherence and the change in neurons’ capacity (d′) (Green and Swets 1966; Macmillan and Creelman 2005) to discriminate nearby orientations (22.5° apart). We found a significant correlation between the post-adaptation change in d′ and the corresponding change in SFC only for the recording sites in the supragranular layers (Fig. 16.2d; r = 0.38, P = 0.02, Pearson correlation). In contrast, neurons in granular and infragranular layers exhibited post-adaptation changes in discriminability that were independent of the changes in gamma spike-field coherence (G: r = 0.04, P = 0.84; IG: r = 0.08, P = 0.70).
16.3.3 Adaptation Reduces Noise Correlations in V1
Responses to dynamic test stimuli in V1 of fixating monkey were recorded before and after brief (400 ms) adaptation to a sine-wave grating of fixed orientation (Fig 16.4a). We used a movie sequence as test stimulus, in which each frame was a sine-wave grating of pseudorandom orientation flashed at 60 Hz. We measured noise correlations between pairs of nearby neurons (n = 423 pairs). We confirmed previous results (Kohn and Smith 2005) that noise correlations are independent of stimulus orientation (only 5 % of the pairs exhibited a significant relationship between the correlation coefficient and stimulus orientation).


Fig. 16.4
Adaptation-induced response decorrelation in V1. (a) Schematic representation of the stimulus sequence: an adapting stimulus of fixed orientation was presented for 400 ms and was followed by a 60-Hz test stimulus of random orientation presented for 1.86 s. (b) Scatter plot showing the trial-by-trial responses of two cells recorded simultaneously. Each dot represents the firing rates of both cells in a given trial. The dotted ellipses represent the two-dimensional gaussian fits of the firing rate distributions during control and adaptation (crosses represent the means). ‘r’ represents the correlation coefficient. (c) The reduction in the mean correlation coefficients after adaptation depends on the adapting orientation (for pairs for which Δφ < 30°). All panels are based on the correlation analysis of n = 423 cell pairs (Δθ < 30°, 223 pairs; Δθ > 30°, 200 pairs). Error bars represent s.e.m. (*P < 0.005; **P < 0.0005)
Figure 16.4b shows an example of a pair of cells preferring nearby orientations that exhibit a strong reduction in correlations after adaptation (the pre-adaptation condition is labeled ‘control’). Across the population, we found an overall post-adaptation decrease in the absolute correlation coefficients that was significant both for positive (mean reduction 22 %, P < 10−8, Wilcoxon signed rank test) and negative (mean reduction 74 %, P < 10−5) coefficients. This reduction in correlation strength is also found in cells exhibiting positive correlations before adaptation and negative correlations after adaptation (mean reduction 73 %, P < 10−6) and negative correlations before adaptation and positive correlations after adaptation (mean reduction 42 %, P < 0.005). Overall, correlation coefficients decayed exponentially with the difference (Δθ in the cells’ preferred orientation (Ts’o et al. 1986). We further examined whether the decrease in correlations after adaptation could be due to the small (5.8 %), but significant (P < 10−6), reduction in mean firing rates. However, we found no relationship between the mean changes in firing rates after adaptation (P > 0.3, Pearson correlation) and the changes in correlation coefficients (de la Rocha et al. 2007).
Since adaptation is an orientation-specific phenomenon (Dragoi et al. 2000, 2002; Felsen et al. 2002), we reasoned that the degree of decorrelation would depend on the relationship between the adapting stimulus and the preferred orientation of the cells in a pair. We thus selected the cell pairs preferring nearby orientations (Δθ < 30°), and compared the mean correlation coefficients before and after adaptation to stimuli of different orientation (we defined as the minimum difference between the adapting stimulus and the preferred orientation of each cell in a pair. There is a strong reduction in correlations (Fig. 16.4c) for adapting stimuli near (Δϕ ≤ 30°, 31 %, P < 0.005; Wilcoxon sum rank test) and far (Δϕ > 60°, 43 %, P < 0.0005) relative to the orientation of the cell pair, but intermediate adaptation (30° < Δϕ ≤ 60°) was ineffective in reducing correlations (19 %; P > 0.1).
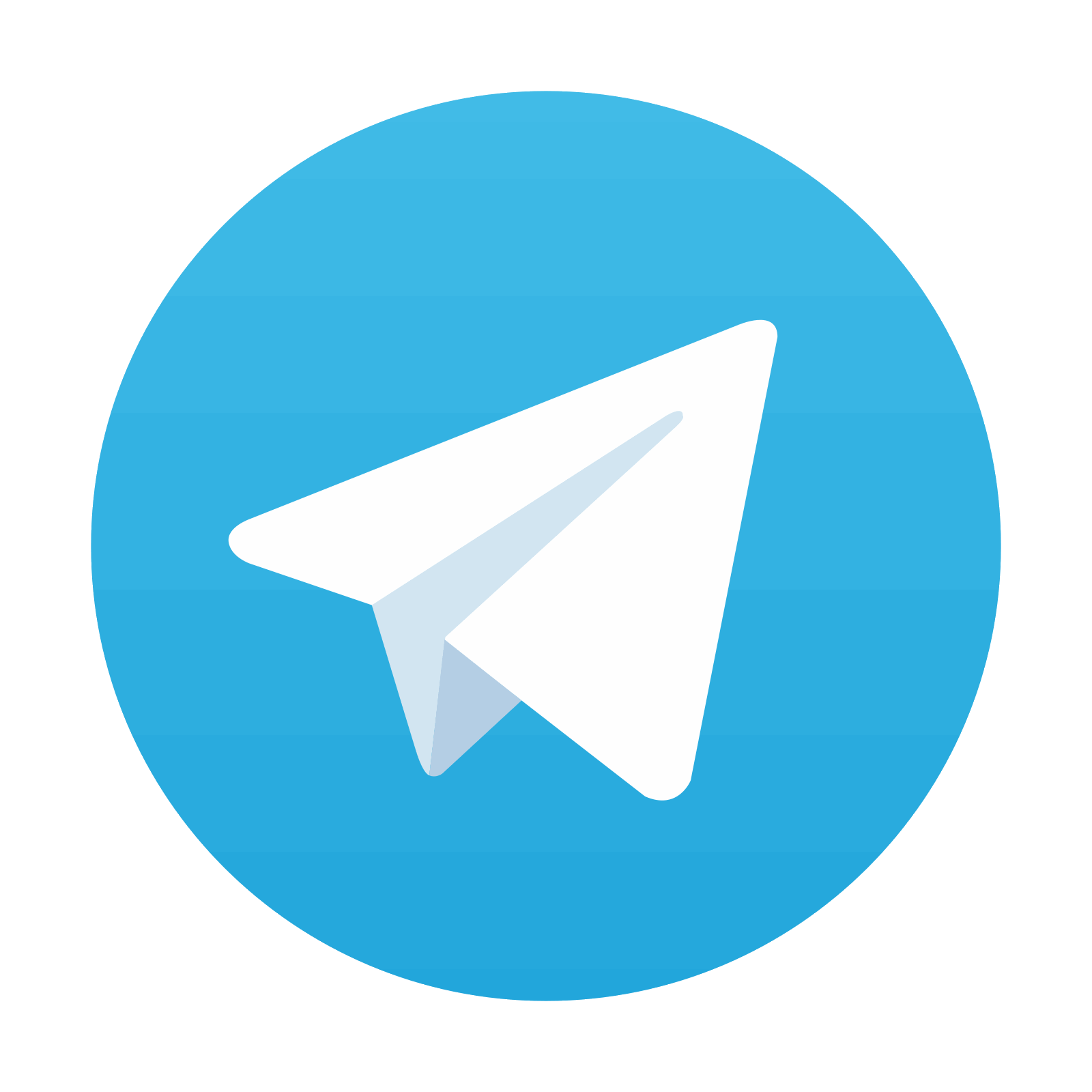
Stay updated, free articles. Join our Telegram channel

Full access? Get Clinical Tree
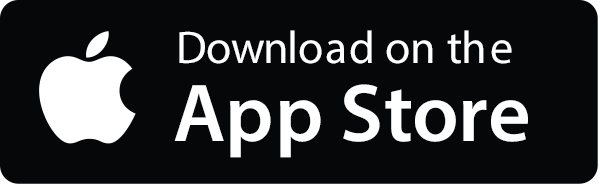
