Diffusion tensor magnetic resonance imaging (DTI) is a relatively new technology that is popular for imaging the white matter of the brain. This article provides a basic and broad overview of DTI to enable the reader to develop an intuitive understanding of these types of data, and an awareness of their strengths and weaknesses.
Diffusion tensor magnetic resonance imaging (DTI) is a relatively new technology that is popular for imaging the white matter of the brain. This article provides a basic and broad overview of DTI to enable the reader to develop an intuitive understanding of these types of data, and an awareness of their strengths and weaknesses. The authors have tried to include equations for completeness, but these are not necessary for understanding the content. Wherever possible, more in-depth technical articles or books are suggested for further reading. The authors especially recommend the new diffusion MRI textbook, the introductory paper on fiber tracts and tumors, the white matter atlas book, and the review of potential pitfalls in DTI analysis. The remainder of this article addresses basic questions about DTI (the what, why, and how of DTI), followed by a discussion of issues in interpretation of DTI, and finally an overview of more advanced diffusion imaging methods and future directions.
Why DTI? A brief history of DTI and its impact on clinical research
The diffusion tensor was originally proposed for use in MRI by Peter Basser in 1994. Before DTI, diffusion MRI had developed from research in diffusion nuclear magnetic resonance. Before the diffusion tensor model was introduced, the orientation of the axons in a tissue sample had to be known to measure anisotropic diffusion, and therefore only fixed samples could be scanned, such as the axon of the giant squid. The introduction of the diffusion tensor model allowed, for the first time, a rotationally invariant description of the shape of water diffusion. The invariance to rotation was crucial because it enabled application of the DTI method to the complex anatomy of the fiber tracts in the human brain. However, the diffusion tensor is not able to fully describe crossing of the fiber tracts.
The popularity of DTI has been enormous. It has been applied to a tremendous variety of neuroscientific studies, including schizophrenia, traumatic brain injury, multiple sclerosis, autism, and aging. Anatomic investigations have been undertaken regarding the structure of the language network, the asymmetry of the white matter in twins and siblings, and the location, asymmetry, and variability of the fiber tracts. Recent investigations have attempted to model the human “connectome” by analyzing structural versus functional brain connectivity as measured with DTI and functional MRI. DTI has also been applied for neurosurgical planning and navigation. A large prospective study showed that addition of preoperative DTI to neuronavigation increased tumor resection and survival and decreased neurologic morbidity.
What is DTI?
DTI is a sensitive probe of cellular structure that measures the diffusion of water molecules. The measured quantity is the diffusivity or diffusion coefficient, a proportionality constant that relates diffusive flux to a concentration gradient and has units of m m 2 s . Unlike the diffusion in a glass of pure water, which would be the same in all directions (isotropic), the diffusion measured in tissue varies with direction (is anisotropic). The measured macroscopic diffusion anisotropy is the result of microscopic tissue heterogeneity. In the white matter of the brain, diffusion anisotropy is primarily caused by cellular membranes, with some contribution from myelination and the packing of the axons. Anisotropic diffusion can indicate the underlying tissue orientation ( Fig. 1 ).

The diffusion tensor describes the diffusion of water molecules using a Gaussian model. Technically, it is proportional to the covariance matrix of a three-dimensional Gaussian distribution that models the displacements of the molecules. The tensor is a 3 × 3 symmetric, positive-definite matrix, and these matrix properties mean that it has three orthogonal (mutually perpendicular) eigenvectors and three positive eigenvalues. The major eigenvector of the diffusion tensor points in the principal diffusion direction (the direction of the fastest diffusion). In anisotropic fibrous tissues, the major eigenvector also defines the fiber tract axis of the tissue, and thus the three orthogonal eigenvectors can be considered a local fiber coordinate system. (This interpretation is only strictly true in regions where fiber tracts do not cross, fan, or branch.) The three positive eigenvalues of the tensor ( λ 1 , λ 2 , λ 3 ) give the diffusivity in the direction of each eigenvector. Together, the eigenvectors and eigenvalues define an ellipsoid that represents an isosurface of (Gaussian) diffusion probability: the axes of the ellipsoid are aligned with the eigenvectors and their lengths are 2 τλ i . Fig. 2 shows three diffusion tensors chosen from different regions of the human brain to illustrate possible shapes of the ellipsoid.
What is DTI?
DTI is a sensitive probe of cellular structure that measures the diffusion of water molecules. The measured quantity is the diffusivity or diffusion coefficient, a proportionality constant that relates diffusive flux to a concentration gradient and has units of m m 2 s . Unlike the diffusion in a glass of pure water, which would be the same in all directions (isotropic), the diffusion measured in tissue varies with direction (is anisotropic). The measured macroscopic diffusion anisotropy is the result of microscopic tissue heterogeneity. In the white matter of the brain, diffusion anisotropy is primarily caused by cellular membranes, with some contribution from myelination and the packing of the axons. Anisotropic diffusion can indicate the underlying tissue orientation ( Fig. 1 ).
The diffusion tensor describes the diffusion of water molecules using a Gaussian model. Technically, it is proportional to the covariance matrix of a three-dimensional Gaussian distribution that models the displacements of the molecules. The tensor is a 3 × 3 symmetric, positive-definite matrix, and these matrix properties mean that it has three orthogonal (mutually perpendicular) eigenvectors and three positive eigenvalues. The major eigenvector of the diffusion tensor points in the principal diffusion direction (the direction of the fastest diffusion). In anisotropic fibrous tissues, the major eigenvector also defines the fiber tract axis of the tissue, and thus the three orthogonal eigenvectors can be considered a local fiber coordinate system. (This interpretation is only strictly true in regions where fiber tracts do not cross, fan, or branch.) The three positive eigenvalues of the tensor ( λ 1 , λ 2 , λ 3 ) give the diffusivity in the direction of each eigenvector. Together, the eigenvectors and eigenvalues define an ellipsoid that represents an isosurface of (Gaussian) diffusion probability: the axes of the ellipsoid are aligned with the eigenvectors and their lengths are 2 τλ i . Fig. 2 shows three diffusion tensors chosen from different regions of the human brain to illustrate possible shapes of the ellipsoid.
How is DTI measured?
To measure diffusion using MRI, magnetic field gradients are used to create an image that is sensitized to diffusion in a particular direction. Through repeating this process of diffusion weighting in multiple directions, a three-dimensional diffusion model (the tensor) can be estimated. In simplified terms, diffusion imaging introduces extra gradient pulses whose effect “cancels out” for stationary water molecules, and causes a random phase shift for molecules that diffuse. Because of their random phase, signal from diffusing molecules is lost. This loss of signal creates darker voxels (volumetric pixels), meaning that white matter fiber tracts parallel to the gradient direction will appear dark in the diffusion-weighted image for that direction ( Fig. 3 ).
Next, the decreased signal ( S k ) is compared with the original signal ( S 0 ) to calculate the diffusion tensor (D) by solving the Stejskal-Tanner Equation (1) . This equation describes how the signal intensity at each voxel decreases in the presence of Gaussian diffusion:
In this equation, S 0 is the original image intensity at the voxel (measured with no diffusion-sensitizing gradient) and S k is the intensity measured after the application of the k th diffusion-sensitizing gradient in the (unit) direction g ˆ k . The product g ˆ k T D g ˆ k represents the diffusion coefficient (diffusivity) in direction g ˆ k . Because the entire set of diffusion-weighted images is used (giving many values for S k and g ˆ k ), this is actually a system of equations that is solved for D, the diffusion tensor. To calculate the six independent numbers in the 3 × 3 symmetric matrix D, at least seven images are needed: six diffusion-weighted images from six gradient directions (giving six values for S k ) plus one baseline image (giving S 0 ). However, in clinical research today, a higher number of images are almost always used. The above system of equations can be solved via the least squares method at each voxel.
Equation (1) also contains b , LeBihan’s factor describing the pulse sequence, gradient strength, and physical constants. The b-factor is near 0 s m m 2 for the image S 0 , which is T2-weighted, and the b-factor is near 1000 s m m 2 for the diffusion-weighted images S k in DTI. For rectangular gradient pulses, the b-factor is defined by b = γ 2 δ 2 ( Δ − δ 3 ) | g | 2 , where γ is the proton gyromagnetic ratio (42 MHz/Tesla), | g | is the strength of the diffusion sensitizing gradient pulses, δ is the duration of the diffusion gradient pulses, and Δ is the time between diffusion gradient RF pulses.
Information on the MR physics of DTI and more information on the tensor calculation process are provided elsewhere. Goodlett and colleagues provide a comparison of tensor calculation methods (including least squares and weighted least squares) in the presence of noise.
How is DTI displayed?
DTI is usually displayed through condensing the information contained in the tensor ( Fig. 4 ) into either one number (a scalar), or four (to give an RGB color and a brightness value). The diffusion tensor can also be viewed using glyphs, which are small three-dimensional representations of the major eigenvector or whole tensor. Finally, DTI is often viewed through estimating the course of white matter tracts through the brain using a process called tractography .
Scalars Derived from DTI
This section describes commonly used scalar quantities, which can be divided into two categories: diffusion magnitude measures and anisotropy measures. The eigenvalues of the symmetric, positive-definite diffusion tensor D are referred to using λ 1 ≥ λ 2 ≥ λ 3 ≥ 0 . Pierpaoli and colleagues originally measured and compared several scalar measures, and the eigenvalues, in different regions of the human brain.
Measures of diffusion magnitude
The simplest and possibly most useful scalar is the average of the tensor’s eigenvalues. This average may be referred to as the mean diffusivity (MD) ; the bulk mean diffusivity ( 〈 D 〉 ) ; or the apparent diffusion coefficient (ADC) map. In clinical imaging, ADC maps may be measured using fewer diffusion gradients than needed for the tensor. A similar quantity to the MD is the sum of the eigenvalues, called the trace of the tensor.
The trace and MD relate to the total amount of diffusion in a voxel, which is related to the amount of water in the extracellular space. The trace is clinically useful in early stroke detection because it is sensitive to the initial cellular swelling (cytotoxic edema) that restricts diffusion. In the normal human brain, the trace is high in cerebrospinal fluid, around 9.6 × 10 − 3 m m 2 s , and relatively constant in normal brain parenchyma (white and gray matter), between 1.95 × 10 − 3 m m 2 s and 2.2 × 10 − 3 m m 2 s . For comparison, the self-diffusion coefficient of water (the diffusivity measured in pure water without any tissue) at a body temperature of 37°C is 3 × 10 − 3 m m 2 s , which would give a trace of 9 × 10 − 3 m m 2 s . The MD and trace measured in ventricles or in edema can be higher than in water because of fluid flow or enhanced perfusion, respectively.
Measures of diffusion anisotropy
Tensor anisotropy measures are ratios of the eigenvalues that are used to quantify the shape of the diffusion. These measures are useful for describing the amount of tissue organization and for locating voxels likely to contain a single white matter tract (without crossing or fanning). The following measures are normalized and all range from 0 to 1, except for the mode, which ranges from –1 to +1.
The fractional anisotropy (FA) is the most widely used anisotropy measure. Its name comes from the fact that it measures the fraction of the diffusion that is anisotropic. This measure can be considered the difference of the tensor ellipsoid’s shape from that of a perfect sphere. FA is basically a normalized variance of the eigenvalues:
where ˆλ is the mean diffusivity. FA is often considered a measure of white matter integrity, although changes in FA may be caused by many factors.
Three intuitive measures are C L , C P , and C S , which are the linear, planar, and spherical shape measures. They describe whether the shape of diffusion is similar to a cigar (linear), pancake (planar), or sphere (spherical).
In voxels with high planar or spherical measure, the principal eigenvector will not always match an underlying fiber tract direction (where tracts cross the eigenvector may point to neither one). However, if the largest eigenvalue is much larger than the other two eigenvalues, the linear measure will be large, indicating the presence of a single fiber tract. These measures can be normalized by λ 1 , by the trace, or by λ 1 2 + λ 2 2 + λ 3 2 .
Although FA measures how far the tensor is from a sphere, another complementary measure discriminates between linear and planar anisotropy. This information is given by the mode, a quantity that is mathematically orthogonal to the FA measure and relates to the skewness of the eigenvalues.
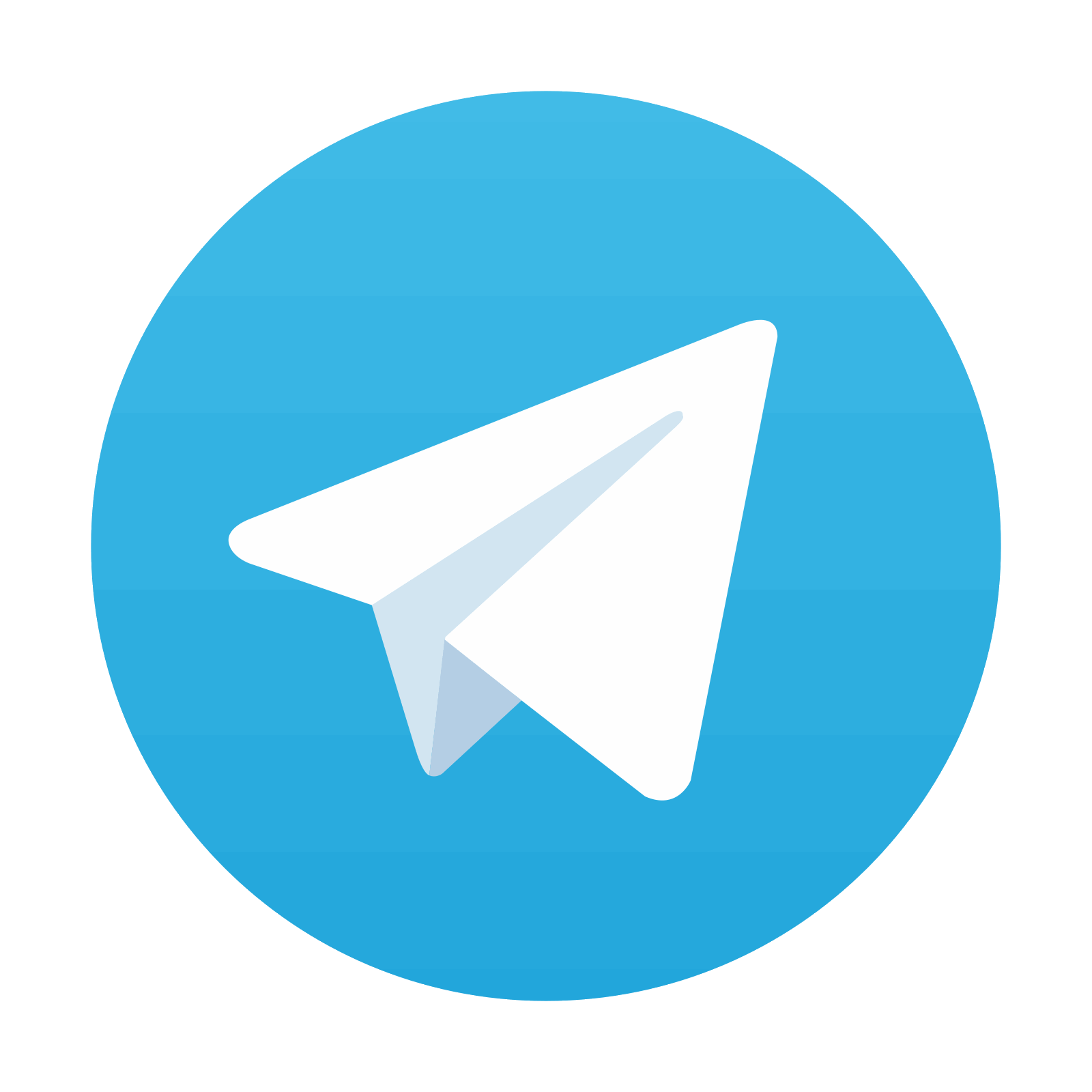
Stay updated, free articles. Join our Telegram channel

Full access? Get Clinical Tree
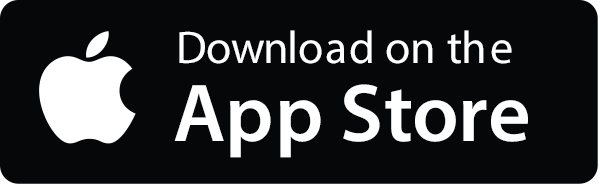
