Fig. 1
a Twenty four hour EEG power spectra in NREM sleep, REM sleep and waking in one representative adult male rat (Wistar Kyoto strain). b EEG signals and corresponding cortical multiunit activity (raster plots below; each bar is a spike) representative of the three vigilance states. Note the fast irregular pattern of cortical firing in waking and REM sleep, and regular occurrence of generalized neuronal silence, corresponding to EEG slow waves, in NREM sleep
Conversely, cortical activity during NREM sleep is generally slower compared to both wakefulness and REM sleep (Hobson and McCarley 1971; Steriade et al. 2001; Vyazovskiy et al. 2004b, 2009b). Early studies showed that, upon falling asleep, the quasi-independent firing of neurons typical of the awake state is replaced by regular synchronous bursts of action potentials (Noda and Adey 1970; Verzeano and Negishi 1960). In more recent studies, intracellular single-cell recordings have shown that, during NREM sleep, virtually all cortical neurons engage in the so-called slow (<1 Hz) oscillation, consisting of a depolarized up state, when neurons show sustained firing, and a hyperpolarized down state, characterized by neuronal silence (Destexhe et al. 1999; Steriade et al. 1993c). During the up state, both pyramidal cells and interneurons are active, and excitation and inhibition are balanced at the synaptic level (Haider et al. 2006; Okun and Lampl 2008). Simultaneous EEG and intracellular or extracellular recordings have shown that slow waves are associated with transitions between up and down states or on and off periods in large populations of cortical neurons (Ji and Wilson 2007; Steriade et al. 2001), and high amplitude slow waves are associated with longer periods of neuronal silence (Fig. 2) and tighter synchrony at on–off and off–on transitions (Vyazovskiy and Harris 2013; Vyazovskiy et al. 2009b).
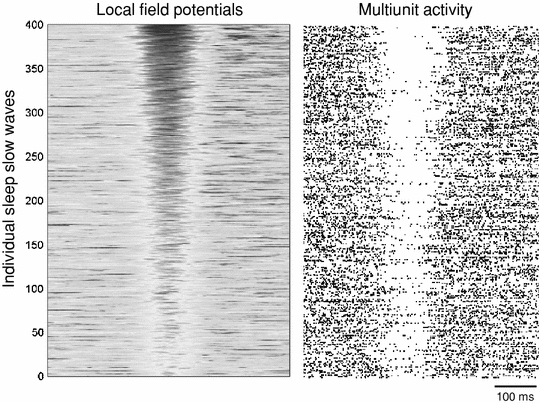
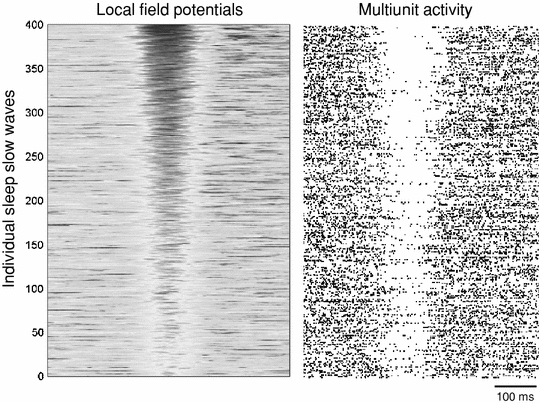
Fig. 2
Left panel individual local field potential slow waves during spontaneous NREM sleep in one individual rat. Four hundred slow waves were detected, and are sorted as a function of their amplitude (gray intensity coded, highest amplitude slow waves are on the top). Right panel corresponding raster plots of cortical multiunit activity. Note that high amplitude slow waves (top) are associated with longer silent periods across the recorded neuronal population
Surprisingly, 20 years after the slow oscillation was first described in the seminal triad of papers by Steriade et al. (1993a, b, c), its functional role remains unclear. At the macroscopic EEG level, the cellular slow oscillation is reflected in the Slow Wave Activity (SWA, EEG power between 0.5 and 4.0 Hz) during NREM sleep (Borbely 1982), which is the best characterized physiological marker of sleep intensity. One of the main defining features of sleep is that it is homeostatically regulated (Cirelli and Tononi 2008; Tobler 2005). The main postulate of sleep homeostasis is “the longer we are active (and, perhaps, the more we are active), the deeper our subsequent sleep” (Daan et al. 1984). In other words, this implies that sleep need (or “sleep pressure”) increases in proportion to the preceding duration of waking, and then dissipates during the ensuing sleep in proportion to its duration and intensity. In mammals and birds, sleep SWA is high in early sleep and decreases progressively within sleep to reach low levels several hours later (Jones et al. 2008; Tobler and Borbely 1986). This dynamics of sleep slow wave activity suggests that sleep is associated with a recovery process. However, neither the nature of the putative recovery processes, nor its relationship with the homeostatic changes in SWA are clear (Vyazovskiy and Harris 2013).
3 Global and Local Sleep Regulation
The first and perhaps most essential question that needs to be addressed is, what is “recovered” during sleep after wakefulness? This question implies that being awake is associated with certain molecular, structural, or functional changes in the brain, which occur progressively and can only be reversed by sleep. The term “recovery” could either apply to specific measurable variables (from metabolic ones, such as cerebral glycogen stores, to cognitive ones, such as reaction times in the psychomotor vigilance task) or, in a broader sense, refer to an overall optimal waking function, which consists of many components and is determined by a variety of factors. The original notion that sleep has a homeostatic recovery function was proposed based on the observation that the levels of EEG slow wave activity during sleep increase in proportion to preceding waking duration and decrease during subsequent sleep. This relationship was mathematically formalized by using a simulation approach, where the temporal evolution of sleep–wake dependent process (so-called Process S) was modeled with a set of exponential functions using such parameters as time constants of an increase and a decrease, the lower and higher asymptote, and others (Borbély and Achermann 2005; Franken et al. 2001; Huber et al. 2000a; Rusterholz et al. 2010; Vyazovskiy et al. 2007a; Zavada et al. 2009). According to this model, sleep pressure, as reflected in SWA, increases during waking and decreases during sleep (Deboer 2013). Notably, NREM SWA increases after wakefulness not only in mammals, but also in birds (Jones et al. 2008; Martinez-Gonzalez et al. 2008).
It is now widely accepted that not only waking duration, but also waking quality is essential, such that the same duration of wake period can lead to markedly different sleep patterns if wake experience is qualitatively different. Important insights have been obtained from studies in which the content of waking experience was manipulated by inducing specific behaviors. For example, in one study rats were subjected to a stressful social defeat, which resulted in a substantial increase of subsequent EEG slow wave activity (Meerlo et al. 1997). More recently, it was found that the same amount of waking in the light and in the darkness appeared to lead to markedly different levels of SWA, which correlated with the amount of preceding exploratory behavior (Huber et al. 2007). Moreover, EEG theta activity, which is a marker of behavioral arousal and active locomotion, predicted the levels of SWA in subsequent sleep in rats subjected to chronic sleep restriction (Leemburg et al. 2010).
Notably, the association between the type of preceding activity and subsequent sleep is also apparent at a local level. Specifically, this was shown by performing finer topographic analyses, in experiments using selective local or peripheral stimulation, or in studies which recorded the activity of individual neurons (Krueger and Tononi 2011). Almost half a century ago, Giuseppe Moruzzi proposed that sleep might entail a cellular dimension: “sleep concerns primarily not the whole cerebrum, nor even the entire neocortex, but only those neurons or synapses, and possibly glia cells, which during wakefulness are responsible for the brain functions concerned with conscious behavior” (Moruzzi 1972). The local, use-dependent facet of sleep regulation had been suggested about 20 years ago, and since then it has received substantial experimental support (Krueger and Obal 1993; Krueger et al. 2008). The most appealing evidence for the local occurrence of sleep has been obtained from marine mammals, such as dolphins and seals, which exhibit unihemispheric slow wave activity while in water (Lyamin et al. 2012; Mukhametov et al. 1977). Such asymmetries appear to be homeostatically regulated, as manifested in a unilateral increase of SWA after selective suppression confined to one hemisphere (Oleksenko et al. 1992). Spontaneous interhemispheric asymmetries of the EEG during sleep have also been found , albeit to a much lesser extent, in birds (Rattenborg et al. 2001, 2012), humans, (Achermann et al. 2001; Nir et al. 2011) and rodents (Vyazovskiy et al. 2002a). In addition, strong anterior–posterior gradients in the expression of SWA have been shown in rodents (Huber et al. 2000b; Vyazovskiy et al. 2002a, b, 2004c, 2007c, 2008b; Vyazovskiy and Tobler 2005) and humans (Cajochen et al. 1999; Marzano et al. 2009; Massimini et al. 2004; Werth et al. 1996).
The evidence for a local, use- or activity- dependent increase in sleep slow wave activity has been obtained from several species and many experimental paradigms. For example, in both rats and mice, spontaneous unilateral whisker stimulation led to a significant increase in SWA over the stimulated somatosensory cortex during subsequent sleep (Vyazovskiy et al. 2000, 2004c). Rats trained to perform a skilled reaching task exhibit locally increased SWA in the primary motor cortex during post-training NREM sleep (Hanlon et al. 2009; Vyazovskiy and Tobler 2008). In humans, unilateral vibratory stimulation of the right hand led to an increase in SWA over the left somatosensory area during subsequent sleep (Kattler et al. 1994). Moreover, a visuomotor learning task led to a local modulation in SWA during subsequent sleep that was significantly increased over the right parietal cortex (Huber et al. 2004). Conversely, following immobilization of one arm during the day, NREM sleep SWA was reduced over the corresponding contralateral somatosensory cortex (Huber et al. 2006). Finally, in rodents, local pharmacological manipulations of neuronal activity during waking altered the expression of sleep-like activity in the corresponding cortical areas (Churchill et al. 2008; Faraguna et al. 2008, 2010). Notably, signs of “local sleep ” have been documented in behaviorally active animals (Pigarev et al. 1997; Vyazovskiy et al. 2011b; Vyazovskiy and Tobler 2008), suggesting that wakefulness is associated with an increasing drive to engage in a sleep-like mode of activity within specific neuronal circuits (Fig. 3). This notion was supported by a recent human study showing local, task-related changes in slow frequency EEG activity in awake subjects (Hung et al. 2013). The underlying mechanism of increased propensity to engage in down-states at a single neuron level is unknown but may be related to the growing need to obtain metabolic recovery or perform necessary cellular maintenance processes after sustained waking activity (Vyazovskiy and Harris 2013). Alternatively, or in addition, local changes in sleep-related activities may reflect synaptic changes.
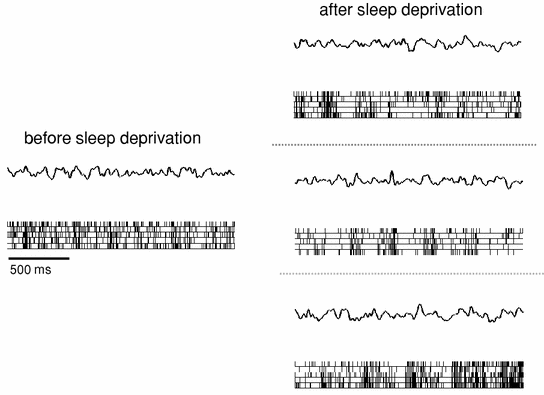
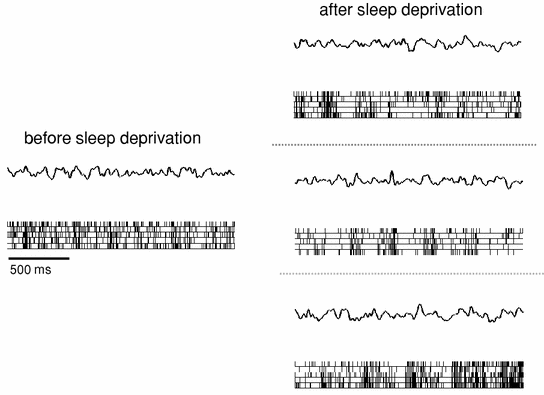
Fig. 3
Left a typical 2-s EEG trace and corresponding multiunit activity (MUA) recorded from the frontal motor cortex in one individual rat during waking at the beginning of sleep deprivation. Right three representative examples of EEG and MUA in an awake rat at the end of 4-h sleep deprivation. Note that despite wake-like EEG signals, the neuronal population often shows generalized quasi-synchronous cessation of neuronal firing, as during NREM sleep
4 Sleep, Neuroplasticity, and Synaptic Homeostasis
The ultimate function of neural or synaptic plasticity is to provide greater flexibility in the interaction of the organism with the constantly changing environment. It is not surprising, therefore, that several forms of neuroplasticity are highly conserved across evolution (Ebendal 1992; Qiu et al. 2006). It still remains to be established when neuronal plasticity first emerged, but it is undoubtedly present already in invertebrates (Bailey and Kandel 2008). Ultimately synaptic plasticity concerns the interaction between individual neurons. Within the brain, neurons integrate thousands of synaptic inputs from local and distant neurons at a timescale of milliseconds, but usually respond with only a few spikes per second. There are many intrinsic and extrinsic factors which influence neuronal spiking activity on a moment to moment basis (Fig. 4). However, even if all these factors remain stable, the capacity to respond to incoming inputs appears to be ultimately determined by the total synaptic weight impinging on a particular neuron, which must therefore be strictly regulated. Indeed, as the brain is constantly receiving sensory information, it must be continuously performing complex computational tasks, such as extracting relevant patterns of information from the multisensory world outside, determined by the context, future goals and preceding experience.
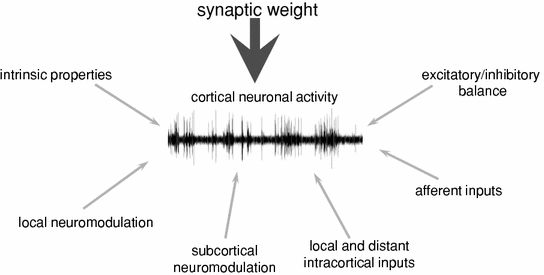
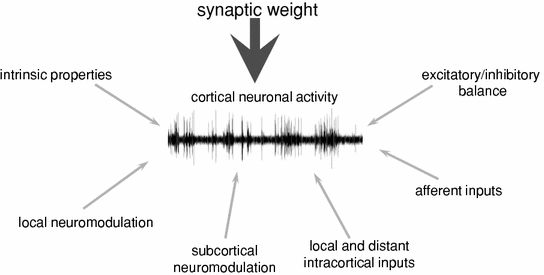
Fig. 4
Some of the essential factors that determine the occurrence of individual spikes, instantaneous changes in spiking activity, and long-term changes in neuronal responsiveness
For synaptic plasticity to be beneficial, it must be regulated to prevent it from taking an aberrant form (Parent et al. 1997). Moreover, the regulation of net synaptic strength is vital for survival, inasmuch as it allows re-allocation of the existing, limited amount of resources in a most efficient way, e.g., toward those neurons or local or distributed neuronal networks, which are most relevant for the ongoing behavior, or those, which were most affected by preceding activity. Dynamic adjustments of spiking outputs depending on the input can occur both on a fast scale, such as spike-frequency adaptation of individual neurons (Fuhrmann et al. 2002) and on a slower timescale, corresponding to homeostatic scaling (Turrigiano 1999) or Hebbian plasticity (Abbott and Nelson 2000). Homeostatic scaling refers to a mechanism, by which firing activity of individual neurons and neuronal circuits is maintained within a specific range, and has been demonstrated both in vitro and in vivo (Hengen et al. 2013; Ibata et al. 2008; Turrigiano 1999). In contrast, Hebbian mechanisms refer to a bidirectional form of plasticity, whereby the weight of synaptic connections between two neurons is increased or decreased depending on precise timing of their respective activities (Abbott and Nelson 2000; Caporale and Dan 2008). As the mechanisms of synaptic plasticity are highly elaborate (Malenka and Bear 2004; Turrigiano 2008), there is a great potential for malfunctioning (Nava and Roder 2011) and a breakdown in some key elements, such as signaling pathways (Lockett et al. 2010), or specific synaptic proteins (Bhakar et al. 2012) is detrimental. This may be manifested primarily in pathologic forms of activity incompatible with normal behaviors, and lead to neuronal damage, severe disease, or death.
Intriguingly, the general features aforementioned about plasticity largely apply to sleep as well. It is conserved across evolution (Cirelli and Tononi 2008; Tobler 2005), it is considered to be vitally important (Rechtschaffen et al. 1999), its mechanisms are highly complex (Brown et al. 2012), and it is prone to various disturbances (Porkka-Heiskanen et al. 2013). Finally, both neural plasticity and sleep are activity-dependent processes. As synaptic activity invariably leads to modifications of individual synapses potentially affecting neuronal spiking output, sleep invariably occurs after a certain amount of (waking) activity, and its major defining electrical event in mammals—EEG slow wave activity—is determined by preceding local neuronal activity. In fact, activity dependence of both plasticity and sleep are their most essential, if not defining features. On one hand, sustained synaptic activity invariably leads to some form of plastic changes. On the other hand, continuous physiological waking cannot be sustained for longer than a few hours or days and is consistently followed by local and global sleep (Cirelli and Tononi 2008).
5 Experimental Observations that can be Explained by Synaptic Homeostasis
The SHY reconciles many empirical observations concerning sleep and plasticity in a comprehensive theoretical framework. In the original formulation of the SHY proposed by Tononi and Cirelli in 2003, it had the following tenets: “(1) Wakefulness is associated with synaptic potentiation in several cortical circuits; (2) Synaptic potentiation is tied to the homeostatic regulation of NREM sleep EEG slow wave activity; (3) Slow wave activity is associated with synaptic downscaling; (4) Synaptic downscaling is tied to the beneficial effects of sleep on neural function and, indirectly, on performance” (Tononi and Cirelli 2003). According to this hypothesis, a key variable that changes systematically during wakefulness, and must be restored back to baseline levels during sleep, is net synaptic weight. It is further suggested that since synaptic strength is tightly linked to energy needs, space, metabolic supplies, and, importantly, to the capacity for further plasticity, restoration of net synaptic strength by sleep would ultimately lead to renormalization of all these variables. The SHY has emerged as a conceptual shell based on a large body of disparate or unexplained findings accumulated over the last decades, and subsequently triggered intensive efforts in many laboratories around the world. Various models and experimental paradigms have been exploited in this framework—from single-cell recordings to complex cognitive tasks, and empirical observations have been obtained at several different levels: molecular, cellular, network, structural, functional, and behavioral. Each of these levels will be considered below, in an attempt to integrate them into a consistent picture.
5.1 Molecular Markers of Plasticity, Cellular Stress, and Sleep
Waking and sleep are associated with systematic changes in the expression and/or release of a variety of molecules, directly or indirectly involved in synaptic plasticity as well as in many other essential cellular functions. After the pioneering experiments by Giuditta et al., in the 1970s and 1980s, showing that overall RNA synthesis was increased during NREM sleep (Giuditta et al. 1980), a series of approaches including PCR, in situ hybridization, mRNA differential display and microarray have shown that several clusters of transcripts known to directly or indirectly influence plasticity are modulated across the sleep-wake cycle and after sleep deprivation. Among the established sleep-promoting substances is the tumor necrosis factor (TNF), which under physiological conditions increases during synaptic activity and can promote sleep when administered externally (Churchill et al. 2008; Krueger et al. 2001; Obal and Krueger 2003). While it remains to be determined to what extent TNF is essential for sleep regulation in physiological conditions, the involvement of TNF in the modulation of synaptic plasticity has been described (Stellwagen and Malenka 2006). Among the effects of TNF on plastic processes, a regulation of glutamate signaling by increasing expression of AMPA receptors has been reported (Pickering et al. 2005). Notably, in the rat cerebral cortex, the expression of GluR1-containing AMPA receptors were found to increase after waking (Vyazovskiy et al. 2008a). On the other hand, TNF-alpha administration led to a decrease in brain-derived neurotrophic factor (BDNF) mRNA in several brain regions (Churchill et al. 2006). Several studies have shown that BDNF, which has been implicated in various forms of synaptic plasticity (Gomez-Palacio-Schjetnan and Escobar 2013), is expressed after sleep deprivation (Cirelli and Tononi 2000a), and especially after active exploratory waking (Huber et al. 2007), while its intracortical infusion enhances local sleep SWA (Faraguna et al. 2008). Also, the cortical expression of BDNF seems to parallel the homeostatic response to sleep deprivation in neonatal rats (Hairston et al. 2004). It should be noted, however, that another study showed that in the neocortex, neither 8 nor 48 h SD had significant effects on BDNF, Synapsin I or CAMKII mRNA levels (Guzman-Marin et al. 2006). In the hippocampus, the picture seems to also be complex: according to some reports BDNF appears to be down-regulated by 8 h of sleep deprivation (Alhaider et al. 2011; Guzman-Marin et al. 2006), while others detected an increase within both the cerebral cortex and the hippocampus (Cirelli et al. 2006; Cirelli and Tononi 2000a; Fujihara et al. 2003). Furthermore, one study found that 12 h SD significantly reduced hippocampal AMPA receptor phosphorylation at the GluR1-S845 site (Hagewoud et al. 2010). Together with TNF-alpha and BDNF, the list of molecules both involved in neuroplasticity and fluctuating across the sleep–wake cycle includes but is not limited to: Homer1a (Maret et al. 2007), Zif-268 (Ribeiro et al. 2002) and Arc (Ribeiro et al. 2007; Seibt et al. 2012). However, it remains to be determined whether the changes in these molecules are related to the proposed increase in synaptic strength or have other roles.
According to SHY, prolonged waking activity leads to increased synaptic strength and, as a result, increased spiking and synaptic activity (Vyazovskiy et al. 2009b). In order to sustain neuronal function at a higher level of excitability, cellular synthetic processes are likely to be intensified to provide additional receptors and synaptic vesicles components (Mackiewicz et al. 2007). Such changes could not only lead to plastic changes but may also be associated with cellular stress responses, and there is an intriguing possibility that some of their underlying mechanisms might overlap. Specifically, one of the well established effects of physiological waking or sleep deprivation is an up-regulation of molecules involved in the unfolded protein response (UPR) (Cirelli 2002; Cirelli and Tononi 2000b; Naidoo et al. 2005; Terao et al. 2003), which is an adaptive mechanism of cellular stress triggered when misfolded proteins accumulate in the lumen of the endoplasmic reticulum (Sidrauski et al. 1998; Vyazovskiy and Harris 2013; Walter and Ron 2011). The endoplasmic reticulum is an important organelle involved in neurotransmission and synaptic plasticity, which are regulated by Ca+2 release (Verkhratsky and Petersen 2002). Paracrine signaling mediated by activity-dependent release of molecules such as TNF may be directly involved in triggering UPR (Xue et al. 2005), and promote the occurrence of local sleep-like activity (Churchill et al. 2008). It is possible that local neuronal down-states may prevent synaptic strength from increasing further, thereby protecting neurons from pathological hyperexcitability. It was proposed recently that local sleep-like activity may enable cellular maintenance processes (Vyazovskiy and Harris 2013), which could involve removal of AMPA receptors leading to a decrease in synaptic strength (Vyazovskiy et al. 2008a). The link between the changes at the level of protein synthesis and synaptic plasticity during waking has also been suggested by an increase in amyloid-beta during waking or sleep deprivation (Kang et al. 2009). It is possible that molecular chaperones, which constitute the primary biological defense against protein misfolding, and increase after waking (Terao et al. 2003) serve to protect the neurons from amyloid-beta aggregation as a result of intense synaptic activity during waking (Narayan et al. 2012). This notion is consistent with the hypothesis that increased neuronal activity during waking may lead to accumulation of protein fragments, which is reversed by sleep (Varshavsky 2012). Simpler preparations, such as in vitro neuronal cultures, which are capable of generating sustained sleep-like activity, appear to be a powerful model to address the link between specific patterns of network activity typical for waking and sleep and their corresponding molecular changes (Hinard et al. 2012).
5.2 Cellular Physiology and Sleep Homeostasis
In the last decades, much effort has been devoted to investigating state-dependent changes in the activity of individual cortical and subcortical neurons in vivo, in vitro, and under anesthesia. However, little has been done to address the effects of preceding history on the electrophysiological properties of individual neurons. More research is necessary since the effects observed may either arise from changes in (a) local or global neuromodulation, (b) neuronal excitability, (c) balance between excitation and inhibition, many other factors or their combined effects (Fig. 4). One possibility is that the effects of sleep–wake history on the electrophysiological properties of individual neurons could be mediated through voltage-dependent changes in the conformation of membrane-bound receptors (Rinne et al. 2013), which can be regulated by subcortical and cortical neuromodulation (McCormick 1992). Indeed, one study found that after 2 hours of sleep deprivation in young rats, the action of noradrenaline on the wake-promoting hypocretin/orexin (HCRT) neurons changed from excitation (75 % of cells) to inhibition (90 % of cells), and this effect was postsynaptic, as both excitatory and inhibitory responses persisted in tetrodotoxin or high magnesium/low calcium solutions (Grivel et al. 2005). This is an interesting observation, which could suggest that synaptic homeostasis in the neocortex may be regulated, at least in part, by functional changes controlled by subcortical neuromodulatory areas. A follow up study from the same group reported that sleep deprivation leads to an involvement of α2-adrenoreceptors, which are responsible for hyperpolarization of the orexinergic neurons (Uschakov et al. 2011). The authors suggested that this mechanism could lead to a decrease of their firing activity, thereby indirectly promoting sleep. There is also evidence that direct measures of synaptic strength are affected by preceding sleep–wake history. For example, another recent study found that waking is associated with an increase in the strength of glutamatergic synapses onto orexinergic neurons, as measured by an increase in amplitude and frequency of miniature excitatory postsynaptic currents (mEPSCs, “minis”) (Rao et al. 2007). These results can suggest that sleep–wake history is an important determinant for the activity of various neuromodulatory subcortical systems, which are likely to be involved (directly or indirectly) in the plastic processes occurring during waking and sleep.
The SHY was addressed by several further studies, which attempted to directly assess various markers of synaptic strength at a single-cell level in the neocortex. Specifically, in one study, recordings from pyramidal cortical neurons in brain slices taken from sleeping and sleep-deprived mice and rats were performed. In all cases both frequency and amplitude of mEPSCs increased after waking and decreased after sleep, suggesting that a net increase in cortical synaptic strength occurs during waking both pre- and post-synaptically, while opposite changes occur during sleep (Liu et al. 2010). Interestingly, another study in mice found that after 8 h of partial sleep deprivation, achieved by a modified pedestal (flowerpot) over water method, the amplitude of miniature excitatory postsynaptic currents, recorded in layer V/VI pyramidal cells of the medial prefrontal cortex, were slightly reduced, while miniature inhibitory postsynaptic currents were unaffected, and intrinsic membrane excitability was increased (Winters et al. 2011). Thus, cortical areas and layers, neuronal subtypes, and their specific electrophysiological properties may be differentially affected by preceding waking activity. This is expected given the differences in the involvement of different neurons in specific activities, different patterns in their connectivity and other factors (Beltramo et al. 2013; Harris et al. 2011; Lapray et al. 2012; McCormick et al. 1985; Sakata and Harris 2009).
Unfortunately, existing patch clamp techniques (cell-attached or whole cell patch) do not allow continuous recording from single neurons across several hours—the timescale of the slow sleep-dependent homeostatic process. Performing long-term juxtacellular recordings is more feasible, but it has been mostly used to investigate state-dependent, rather than history-dependent changes in neuronal activity. For example, it was recently found in rats that parvalbumin-expressing basket GABA-ergic interneurons in the hippocampus change their firing consistent to changes in behavioral state (Lapray et al. 2012). Another rat study found that the activity (measured as c-Fos expression) of individual neurons located in the preoptic and lateral hypothalamic areas, was dependent on preceding sleep deprivation or time spent in deep NREM sleep (Hsieh et al. 2011). Specifically, the percentage of activated cells in Median Preoptic Nucleus (MnPN) and ventrolateral preoptic area (VLPO) correlated significantly with time spent in deep NREM sleep during the 1 h prior to sacrifice. This observation is interesting, as it was shown earlier that bilateral lesions of the VLPO in rats resulted in a decrease of sleep time and sleep EEG SWA, which was proportional to the area that was lesioned (Lu et al. 2000). Moreover, it was shown recently, also in rats, that infusion of A2A adenosine receptor antagonist into the VLPO attenuated the increase in neuronal discharge induced by sleep deprivation (Alam et al. 2014). Although specific mechanisms of these changes remain to be established, since the hypothalamus is implicated in the regulation of state transitions (Saper et al. 2010), it is plausible that preceding history might affect both the strength of connectivity between cortical and subcortical neurons as well as the cortical neuromodulatory milieu, which could in turn promote sleep initiation, sleep maintenance, and sleep intensity.
5.3 Network Homeostasis
One of the most consistent observations in adult mammals is that sleep deprivation and prolonged physiological waking lead to increased EEG power in the slow wave range during subsequent sleep, which results from an increased incidence of high amplitude slow waves (Borbely et al. 1984; Huber et al. 2000b; Riedner et al. 2007; Vyazovskiy et al. 2006, 2007b; Vyazovskiy and Tobler 2005). Surprisingly, while increased SWA as a measure of preceding waking is well established, neither mechanistic explanation for it, nor its functional significance is clear.
As has been shown, EEG slow waves reflect the synchronous occurrence of down-states or off periods across large cortical populations (Buzsaki et al. 2012; Vyazovskiy et al. 2009b) (Figs. 1, 2). Anatomical studies suggest that the amplitude of slow wave activity can be accounted for by stronger connectivity between local and distant cortical regions (Piantoni et al. 2013; Vyazovskiy and Tobler 2005). Notably, it has been found that sleep with high SWA, such as under high sleep pressure, is characterized by increased local and global synchronization of cortical neuronal activity (Destexhe et al. 1999; Nir et al. 2011; Riedner et al. 2007; Vyazovskiy et al. 2004a, 2009b, 2011a), suggesting that either functional and/or anatomical connectivity is affected by preceding waking. It is important to emphasize that the slow oscillation is a network event, and for its expression it is essential that large, distributed interconnected neuronal populations engage in down-states near-simultaneously (Volgushev et al. 2006; Vyazovskiy and Harris 2013). The SHY provides a plausible mechanistic explanation for increased slow wave activity during early sleep after extended wakefulness (Esser et al. 2007; Hill and Tononi 2005; Riedner et al. 2007; Tononi and Cirelli 2006; Vyazovskiy et al. 2009a). Specifically, if cortical synaptic strength is affected by preceding waking or sleep, it would almost inevitably affect the local and global cortical dynamics, that would especially be apparent during spontaneous network activity during sleep (Vyazovskiy 2013; Vyazovskiy et al. 2011a).
Changes in the slope of slow waves usually parallel changes in slow wave amplitude. However, while slow wave slopes correlate with neuronal synchrony, the mechanisms underlying the changes in slow wave amplitude are less clear, and may be related to the duration of the population off periods (Vyazovskiy et al. 2009b). Indeed, although the higher amplitude of slow waves was often associated with steeper slope, this was not always the case, and several studies found steeper slopes after waking even after slow waves were matched by their amplitude (Esser et al. 2007; Riedner et al. 2007; Vyazovskiy et al. 2007b). While an LFP slope is an indirect measure of underlying neuronal synchrony, we also found that the slopes calculated based on the changes in cortical neuronal activity at on–off transitions also appear to be steeper in early sleep (Fig. 5).
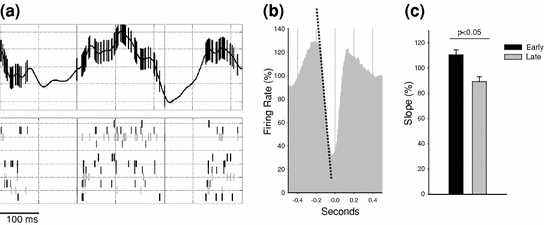
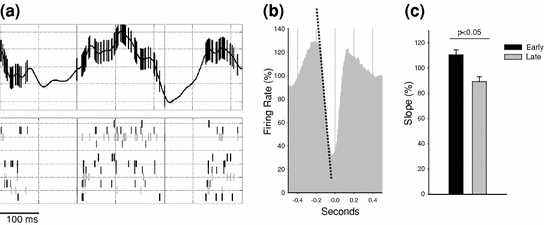
Fig. 5
a Top panel 600-ms record from the parietal EEG recorded in an individual rat during NREM sleep. Two individual slow waves (negativity downward) are apparent in this example. Vertical bars overlaid on the EEG trace denote the occurrences of neuronal spikes recorded concomitantly in the contralateral parietal cortex. Bottom panel raster plot of spiking activity of individual neurons shown on the top panel. Note that all neurons are silent during most of the downward deflection of the EEG signal, corresponding to slow waves. b An average profile of cortical multiunit activity (MUA) triggered by an occurrence of spontaneous EEG slow waves (time 0). Note that on average spiking activity during a slow wave is substantially decreased. Dotted line depicts the slope of the change in MUA corresponding to the downward slope of EEG slow wave. c Mean values (n = 4 rats) of the MUA slope (shown as dotted line on panel B) in early sleep (first 3 h period after light onset) and late sleep (last 3 h period of the same light period). Note that the MUA slope is more shallow in late sleep suggesting a decreased neuronal synchrony during the transition from network activity to network silence
It remains to be determined how the cortical networks self-organize in time and space to engage in synchronized slow wave activity, and what are the molecular mechanisms involved in synaptic plasticity, which are involved in this process. It was proposed recently that nitric oxide (NO), which is implicated strongly in synaptic plasticity in the cerebral cortex (Hardingham et al. 2013), may appear to be ideally placed to link homeostatic sleep drive with the observed changes in EEG SWA (Gerashchenko et al. 2008; Kilduff et al. 2011). Specifically, a novel sub-population of cortical inhibitory interneurons immunoreactive for neuronal nitric oxide synthase (nNOS) was found, which were activated after sleep deprivation in relation to cortical SWA (Morairty et al. 2013).
An essential step in the generation of slow wave activity is the initiation of an up state at the level of individual cortical neurons and its subsequent propagation across large cortical assemblies. It has been suggested that the onset of the up state is caused by a gradual membrane depolarization that builds up due to the summation of subthreshold events, which occur as a result of spontaneous neurotransmitter release (Chauvette et al. 2010; Timofeev et al. 2000). Once a subset of neurons enter an up state, they may be capable of recruiting other neurons, primarily those that receive the most dense or strongest projections from other neurons (Vyazovskiy et al. 2011b). Similarly, in humans and rats, a volley of locally applied electrical or magnetic activity, sufficiently strong to excite and recruit a large cortical neuronal population, is capable of inducing full-fledged EEG slow waves during natural sleep (Massimini et al. 2007; Vyazovskiy et al. 2009a). Notably, individual slow waves appear to have a unique site of origin and a distinct route of propagation, originating more frequently in the anterior cortical areas both in humans and in animals (Leemburg et al. 2010; Massimini et al. 2004; Murphy et al. 2009; Nir et al. 2011; Riedner et al. 2011; Vyazovskiy et al. 2009a). Consistently, the frontal areas of the neocortex have been described as more vulnerable to sleep loss (Anderson and Horne 2003; Horne 1993). Moreover, during sleep deprivation the frontal derivations show a more pronounced increase in slow EEG frequencies, which are considered a waking counterpart of “sleep pressure” (Finelli et al. 2000). Interestingly, spontaneous sleep slow waves appear to propagate from the site of origin to distant cortical areas faster in early sleep as compared to late sleep (Fig. 6), supporting the notion of stronger (or more efficacious) network connectivity after a period of waking. In addition to intracortical mechanisms, there is strong evidence for an involvement of the thalamus in generating cortical slow waves (Crunelli and Hughes 2009; David et al. 2013). It remains to be determined whether the strength of thalamocortical connections is also modified by preceding waking duration or sensory experience.
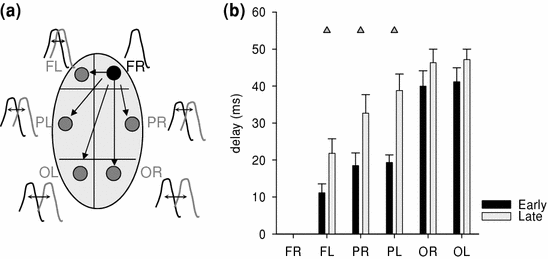
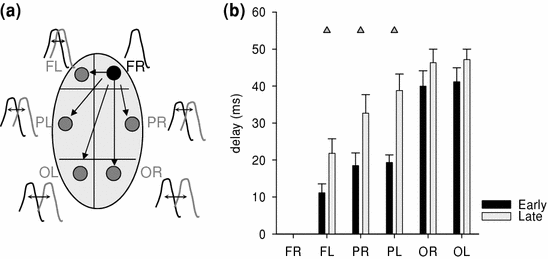
Fig. 6
a Schematic depiction of the position of six LFP bipolar concentric electrodes on the skull surface of a rat (FR, FL = frontal right, frontal left; PR, PL = parietal right, parietal left; OR, OL = occipital right, occipital left). Right frontal derivation was considered “origin” in this example, and sleep slow waves were detected in all six derivations. b The average delay was computed between the peaks of slow waves in FR and the peak of the next slow wave within the first 50 ms in the other five cortical derivations. Mean values ±SEM, n = 6 rats. Note that the delay was shortest for the slow wave in the contralateral frontal cortex, and longest for the most posterior occipital derivations. However, in late sleep (last 3 h period of the light period) the delay was significantly longer as compared to early sleep (first 3 h period of the light period)
5.4 Neuronal Structural Modifications Across the Sleep–Wake Cycle
The cortical activity during sleep ultimately arises from the anatomical patterns of cortical connectivity (Kurth et al. 2010; Murphy et al. 2009; Piantoni et al. 2013; Rattenborg 2006; Vyazovskiy et al. 2004a; Vyazovskiy and Tobler 2005). While the changes in network activity after waking and sleep, as a result of plastic changes, can be partly accounted for by an alteration of functional connectivity or subtle modifications in molecular machinery, the question remains whether different behavioral states are also associated with dynamic anatomical changes. Indeed, while functional modifications of synapses can confer flexibility to cortical circuitries, morphological changes could provide greater stability. For example, given a powerful role of subcortical neuromodulation in voltage-dependent properties of postsynaptic receptors (McCormick 1992), subtle functional modifications may be vulnerable to unspecific generalized changes in cortical excitability. On the other hand, structural changes present a significant demand in terms of biosynthetic work and energy requirements necessary to maintain newly formed or existing spines, and therefore must be subject to regulation. Indeed, unlike other tissues, the space within the cranium is limited, precluding unrestricted increase in the number of synaptic spines as a result of waking experience. Do waking and sleep lead to structural changes at the level of individual neurons?
The first evidence for sleep–wake dependent structural changes in the central nervous system came from a confocal microscopic study in Drosophila, in which it was found that protein levels of several key components of central synapses, such as bruchpilot, cysteine string protein, disks-large, dynapsin, and syntaxin were high after waking and low after sleep (Gilestro et al. 2009). These changes were related to the preceding behavioral state rather than the time of day, and occurred in all major areas of the Drosophila brain. The decrease of synaptic markers during sleep was progressive, and sleep was necessary for their decline. Simultaneously, in a similar fruit fly model, another study showed that social experience did not only increase sleep, but was also associated with an increase in the number of synaptic terminals. Moreover, the number of synaptic terminals was reduced during sleep and this decline was prevented by sleep deprivation (Donlea et al. 2009). More recently Bushey et al. described, in three different Drosophila neuronal circuits, that synapse size or number increased after a few hours of waking and decreased only if flies were allowed to sleep, while rich wake experience resulted in both larger synaptic growth and greater sleep need (Bushey et al. 2011). Finally, using time-lapse two-photon imaging of the presynaptic marker synaptophysin in zebrafish larvae HCRT neurons, it was recently shown that synapse number is affected not only by the time of day but also by preceding sleep–wake history (Appelbaum et al. 2010).
Similar changes in structural synaptic plasticity were also obtained in rodents. Specifically, in adolescent mice, using two-photon microscopy, it was found that waking results in a net increase in cortical spines, whereas sleep is associated with net spine loss (Maret et al. 2011; Yang and Gan 2012). Interestingly, the number of cortical spines did not change significantly across the sleep–wake cycle in adults, but only in adolescent mice, in which the turnover of spines is greater (Maret et al. 2011). This result indicates that, at least as far as superficial cortical layers are concerned, preceding history does not seem to lead to noticeable structural changes, and it may be that stronger stimulation or more intense waking experience is necessary for structural changes to be observed in adults animals. Moreover, the resolution of in vivo imaging techniques has so far prevented a systematic investigation of the effects of sleep and waking on synaptic volume, which could be modulated in the adult brain, similar to that seen for the number of synapses during adolescence. Interestingly, in the mouse olfactory bulb Yokoyama et al. found that elimination of adult-born granule cells occurs during postprandial behaviors, including sleep (Yokoyama et al. 2011), further suggesting that sleep may contribute to structural reorganization within the central nervous system. On the other hand, in the hippocampus prolonged sleep deprivation seems to reduce hippocampal cell proliferation, differentiation, and survival [for review see (Meerlo et al. 2009)].
5.5 Functional Implications
What are the functional consequences of plastic changes occurring during waking and sleep? Maintaining plastic changes within strictly controlled limits is not only important to assure sustainability of cortical circuits in terms of levels of excitability, availability of resources and brain “real estate” (Tononi and Cirelli 2003, 2006), but also in terms of functional consequences. While plastic changes may affect various aspects of brain physiology, the most important feature—the capacity for further change—deserves special attention.
Neuronal plasticity is manifested in several forms including potentiation and depression of synaptic transmission, which involve rapid adjustments in the strengths of individual synapses in response to specific patterns of correlated synaptic activity (Stellwagen and Malenka 2006), or in vivo experience (Rioult-Pedotti et al. 2000; Whitlock et al. 2006). An important property underlying long-term potentiation (LTP) is saturation, that is the relative inability to further enhance the amplitude of synaptic currents in response to a stimulus of increasing intensity or frequency (Heynen and Bear 2001). Saturation of both LTP and long-term depression (LTD) after repeated electrical or pharmacological stimulation was found in different species and preparations (Doyere et al. 1997; Frey et al. 1995; Heynen and Bear 2001; Lante et al. 2006; Moser et al. 1998).
A crucial observation was that the ability to learn and to induce LTP interact in a way suggestive of common substrates (Castro et al. 1989; Moser et al. 1998; Stefan et al. 2006; Ziemann 2004). For instance, after acquisition of a motor learning task both slope and amplitude of evoked potentials in the trained motor cortex increased relative to the contralateral, untrained one, suggesting the occurrence of LTP (Rioult-Pedotti et al. 1998). To confirm this point, the authors showed that the ability to electrically induce LTP was reduced in the trained motor cortex, while LTD was enhanced. Similar results were obtained in the hippocampus (Whitlock et al. 2006). Notably, there is also evidence suggesting that LTP-like plasticity may be partially saturated after wakefulness even without any specific learning paradigm. Thus, in vitro studies in the hippocampus showed that insufficient or fragmented sleep impairs the induction of LTP but favors the induction of LTD [e.g., (Campbell et al. 2002; Kopp et al. 2006; McDermott et al. 2003; Tartar et al. 2006)]. An alternative explanation could be that other, yet unknown, processes at the molecular level interfere with or inhibit further synaptic strengthening after prolonged wakefulness.
It has been proposed that one of the functions for synaptic renormalization during sleep is to prevent LTP saturation, thereby allowing further plastic changes during subsequent wakefulness. This notion is consistent with the hypothesis that LTD may prime a synapse in preparation for the expression of LTP (Braunewell and Manahan-Vaughan 2001). Interestingly, several studies in humans showed an impairment of episodic memory after total sleep deprivation, suggesting that sleep before learning is critical in preparing the brain for the acquisition of new memories (Curcio et al. 2006; Huber 2007; Turner et al. 2007; Yoo et al. 2007). Consistently, recent studies in rats demonstrated that LTP was successfully induced after a period of sleep, but it was partially occluded after a period of wakefulness (Vyazovskiy et al. 2008a, 2011a). Thus, the partial inability for further plastic changes after waking and its restoration after sleep can be explained in the framework of the SHY. Although several results are functionally consistent with the SHY main claim, a set of data seems to be in open contradiction with it. For instance, Chauvette et al. showed that cortical somatosensory evoked potentials induced in cats by electrical stimulation of the medial lemniscus were enhanced after a brief period of sleep (Chauvette et al. 2012), leading the authors to conclude that sleep is associated with synaptic potentiation. An alternative interpretation could be that enhanced somatosensory responses after a “power nap” reflect temporarily, improved signal-to-noise ratio and increased fidelity in the transmission of sensory information from the periphery. More studies are necessary to address this inconsistency and to investigate the role of other possibly confounding factors, such as sleep inertia, brain temperature, or glucocorticoids.
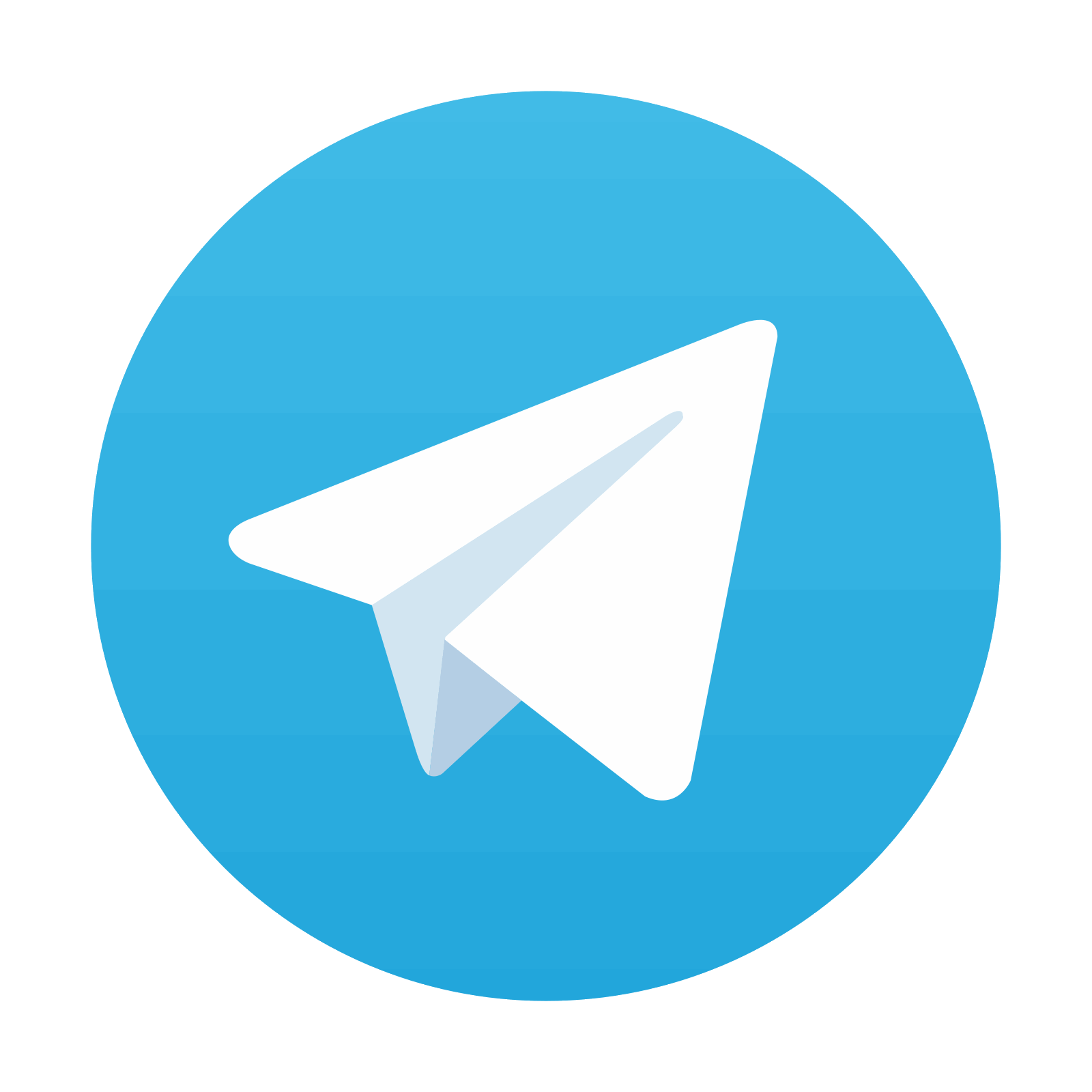
Stay updated, free articles. Join our Telegram channel

Full access? Get Clinical Tree
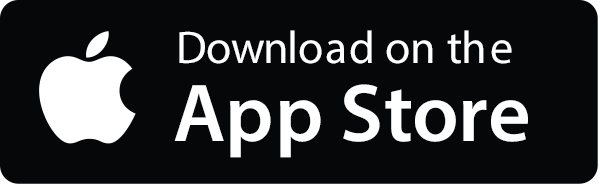
