Fig. 1
Ocular dominance plasticity in the cat requires protein synthesis during sleep. a In developing cats with normal vision, most neurons in the primary visual cortex (V1) are binocular (i.e., equally responsive to inputs from either eye, represented as the yellow area). b When animals are deprived of patterned visual input in one eye (i.e., monocular deprivation) most neurons in V1 become responsive only to stimulation of the nondeprived eye (NDE). This canonical form of physiological plasticity is known as ocular dominance plasticity (ODP). It is induced very rapidly in awake cats (6 h) and is enhanced/consolidated by subsequent sleep (6 h). To test the role of protein synthesis in sleep-dependent ODP, visual cortices are infused with vehicle or the selective mammalian target of rapamycin (mTOR) inhibitor during the post-MD sleep period. c Sleep-dependent ODP is intact in the vehicle infused hemispheres and includes a maintenance of depression of the DE visual input (dotted red line) and potentiation of the NDE input (thick red line). d Inhibition of protein synthesis in V1 with rapamycin during post-MD sleep blocks sleep-dependent ODP. This essentially halts plastic changes at a stage induced by waking experience alone. Reproduced with permission from (Seibt and Frank 2012)
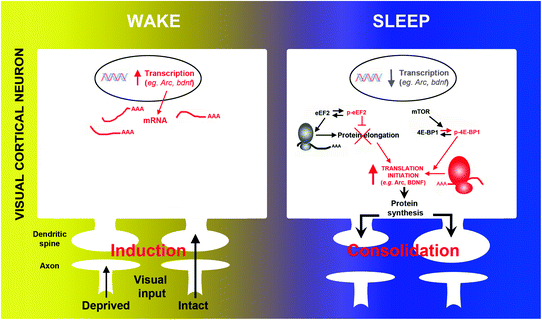
Fig. 2
The transcription and translation of plasticity-related mRNAs are divided across wake and sleep. During wake, monocular deprivation triggers activity-dependent transcription of immediate-early and neurotrophin genes (e.g., arc, bdnf, c–fos) in V1. Subsequent sleep activates a cascade of translational events (increased translation initiation via 4E-BP1 phosphorylation and reduced global elongation via eEF2 phosphorylation) leading to a net increase in translation initiation of subsets of mRNA. Arc and bdnf are two examples of important plasticity-related genes where transcription is decreased and translation is increased during sleep. Reproduced with permission from (Seibt et al. 2012)
A two-stage process in ODP is further demonstrated by a recent study of single neuron activity in freely behaving animals. Aton et al. (2013) used chronic, stereotrode recording of single visual cortical neurons to track their activity and interactions before, during, and after a period of MD. In contrast to previous studies employing similar longitudinal recording (Mioche and Singer 1989), neuronal activity was also recorded across the sleep-wake cycle. MD in the awake animal caused a profound reduction in firing rate in fast-spiking neurons (i.e., putative GABAergic cells) in the visual cortex. This decrease in activity was maintained during the first 6 h of post-MD sleep and accompanied by an increase in firing in regular-spiking neurons (i.e., putative excitatory neurons). The decrease in fast-spiking activity was also proportional to plastic changes in regular-spiking neurons observed after sleep. This suggests that in addition to changes in the deprived eye pathway, MD alters intracortical inhibition which contributes to sleep-dependent changes in excitatory circuits. Together, these results clearly demonstrate that sleep promotes cortical potentiation in the developing cat cortex.
The above results cannot be ascribed to nonphysiological processes resulting from MD as recently asserted (Tononi and Cirelli 2014). For example, it was claimed that the effect of short-term MD used in these studies (6 h) is nonphysiological because “wake is accompanied not by the usual net potentiation but by massive synaptic depression” (Tononi and Cirelli 2014). This is incorrect in three aspects. First, there is no “massive” synaptic depression after only 6 h of MD (Aton et al. 2009; Dumoulin et al. 2013; Frank et al. 2001; Seibt et al. 2012). Several days of MD in cats are required to produce what might constitute “massive” (i.e., saturating) weakening effects in deprived eye pathways (Crair et al. 1997; Olson and Freeman 1975, 1980). However, 6 h of MD in the awake cat only produces a small change in these circuits. Second, there are many examples of waking experience that do not produce “net potentiation”, but instead synaptic weakening [for discussion, see (Frank 2012)]. While it is true that motor learning commonly leads to potentiation in motor cortex, this is not universally true in other parts of the brain or with other types of experience (Frank 2012). Therefore, the mere direction of plastic change does not determine whether the underlying plasticity is “nonphysiological”. Third, although MD eventually leads to large changes in thalamocortical circuitry, other manipulations of experience in early life do so as well (de Villers-Sidani and Merzenich 2011; Foeller and Feldman 2004). The fact that the developing brain is more plastic than the adult brain does not mean that developmental plasticity is “nonphysiological”. It was further claimed that short-term MD is “followed by a 40 % decrease in slow waves during subsequent sleep” (Miyamoto et al. 2003). The 40 % reduction in NREM slow wave activity (SWA) in the Miyamoto study was not induced by MD, but by months of dark rearing from birth (Miyamoto et al. 2003), which is a completely different experimental paradigm with very different effects on developing circuits (Buisseret and Imbert 1976; Fagiolini et al. 1994). In fact, short-term MD used in studies from the Stryker and Frank laboratories does not significantly reduce visual cortical SWA (Seibt et al. 2012) or alter in any way basic visual processing in cortical neurons, except the expected loss of response to the deprived eye. More specifically, visual responses in the intact visual pathway are completely normal, which is to be expected if the underlying processes are physiological (Frank et al. 2001). In addition, the blockade of ODP by sleep deprivation is unlikely explained by increases in stress hormone concentration. This is because ODP is remarkably resistant to the effects of the principal stress hormone corticosterone (Daw et al. 1991), and corticosterone levels after MD (or sleep deprivation) are tenfold lower than those reported to reduce ODP (unpublished observations).
3 Sleep and Plasticity in the Adult Brain
3.1 LTP and LTD: In Vitro and In Vivo Studies in Adult Animals
The role of sleep in adult synaptic plasticity has historically been investigated using classic Hebbian LTP and LTD. Beginning in the late 1980s, several investigators showed that sleep states influence tetany-induced LTP in animal models [reviewed in (Benington and Frank 2003; Hennevin et al. 2007)]. Overall, it appears that hippocampal LTP can be induced during REM sleep, whereas similar stimulus protocols during NREM sleep have no effect or produce LTD. Subsequent investigations have shown that sleep and sleep loss can affect the induction or maintenance of LTP in vivo and in vitro. Romcy-Pereira and Pavlides (Romcy-Pereira and Pavlides 2004) found that REM sleep deprivation and total sleep deprivation impair the maintenance of LTP in the dentate gyrus, but enhance this process in the medial prefrontal cortex (mPFC). Marks and Wayner found that sleep disruption also reduces hippocampal LTP in anesthetized rats (Marks and Wayner 2005). Kim et al., also employed the “flower pot” REM sleep deprivation technique for 5 days in rats, after which tetany was applied to the hippocampus while the animals were awake (Kim et al. 2005). In contrast to Marks and Wayner, these investigators report a delayed effect of REM sleep deprivation on LTP; reductions in LTP were observed 24 h after the termination of REM sleep deprivation. A large number of studies also show that in vitro hippocampal LTP (either the incidence or maintenance) is reduced in rodents that undergo varying amounts of REM sleep deprivation, total sleep deprivation, or sleep restriction (Arrigoni et al. 2009; Campbell et al. 2002; Chen et al. 2006; Davis et al. 2003; Florian et al. 2011; Ishikawa et al. 2006; Kopp et al. 2006; McDermott et al. 2003, 2006; Ravassard et al. 2006, 2009; Tartar et al. 2006; Vecsey et al. 2009).
The underlying mechanisms mediating the effects of sleep loss on LTP and LTD are not well understood. However, they do not appear to be simply due to indirect effects of the sleep deprivation procedures. For example, these deficits can be dissociated from changes in stress hormones (Kopp et al. 2006; Ravassard et al. 2009). Diminished plasticity may instead be linked to decrements in hippocampal NMDA receptor function (Chen et al. 2006; Kopp et al. 2006; Longordo et al. 2009; McDermott et al. 2005) and ERK/MAPK activation (Ravassard et al. 2009) combined with reductions in plasticity-related mRNAs or proteins (Davis et al. 2006; Guzman-Marin et al. 2006), and elevated concentrations of PDE4 (Vecsey et al. 2009) and extracellular adenosine (Arrigoni et al. 2009; Florian et al. 2011).
Most studies of LTP and sleep have focused on the adult hippocampus. Consequently, very little is known about how sleep and sleep loss impact adult cortical plasticity. Aton et al., provide some of the first unequivocal evidence that sleep directly promotes naturally occurring LTP in the adult cortex in vivo (Aton et al. 2014). In adult nonanesthetized mice, brief exposure to a visual stimulus (phase-reversing, oriented gratings) results in an enhancement of cortical responses to stimuli of the same orientation (orientation-specific response potentiation: OSRP). OSRP is considered an in vivo form of LTP because it involves many of the same mechanisms governing Hebbian LTP in vitro (Cooke and Bear 2010). For example, tetany-induced cortical LTP occludes subsequent OSRP (and vice versa). Occlusion is a classic means of determining if two processes share the same intracellular mechanisms. Occlusion occurs because one process depletes substrates (e.g., phosphorylated proteins) that are also used for the second process. The role of sleep in adult OSRP was investigated by examining the effects of experience alone, or in combination with a subsequent period of sleep, or sleep deprivation. Chronic stereotrodes were used to chronically record the activity of single cortical neurons in the visual cortex in unanesthetized adult mice before, during, and after presentation of a specifically orientated grating (the training stimulus). OSRP developed several hours after the stimulus presentation, but only after the mice slept. OSRP was prevented when the animals were instead kept awake after the stimulus (Aton et al. 2014).
3.2 Replay-Reactivation of Waking Experience in the Sleeping Adult Brain
In the mid-1990s, Matt Wilson and Bruce McNaughton demonstrated that hippocampal place cells in rats replay patterns of activity during sleep originally present during prior waking experience (Wilson and McNaughton 1994). This work extended previous findings from Pavlides and Winson (1989), who found that place cells active during exploration showed increased activity in subsequent sleep. Collectively, these findings led to the theory that replay may be a means of transferring information (or memories) from the hippocampus to the neocortex (Buzsaki 1996; Diekelmann and Born 2010). On a synaptic level, this transfer likely involves LTP as it occurs during rapid bursts of hippocampal activity among specific sets of circuits [ripples and sharp waves] (Buzsaki 1996; Schwindel and McNaughton 2011). Evidence of replay has been found in an impressive number of animal species, ranging from birds (Dave and Margoliash 2000) to primates(Hoffman and McNaughton 2002) and based on brain imaging, humans (Deuker et al. 2013; Maquet et al. 2000; Peigneux et al. 2004). The animal studies are also embedded in well-established paradigms of behavior, cellular physiology, and plasticity [reviewed in (Girardeau and Zugaro 2011; Schwindel and McNaughton 2011)].
The phenomenon appears quite robust, as variants have been found in the rodent hippocampus, ventral striatum, and cortex (Ji and Wilson 2007; Kudrimoti et al. 1999; Lee and Wilson 2002; Louie and Wilson 2001; Pennartz et al. 2004; Skaggs and McNaughton 1996; Wilson and McNaughton 1994). Although there is some evidence that forms of replay occur during REM sleep (Louie and Wilson 2001; Poe et al. 2000), communication between the hippocampus and cortex is generally conjectured to occur during NREM sleep. This is because during this state activity in the hippocampus is consistent with outflow, rather than inflow (Buzsaki 1996; Diekelmann and Born 2010; Graves et al. 2001; Hasselmo 1999). There are indeed interesting correlations between ripples and sharp waves (hippocampal events when replay is reported) and thalamocortical spindles and delta waves consistent with this hypothesis (Battaglia et al. 2004; Siapas and Wilson 1998; Sirota et al. 2003). In addition, though quite rare, there are instances when hippocampal and cortical replay occur simultaneously (Ji and Wilson 2007; Qin et al. 1997).
3.3 Re-examining Replay-Reactivation
To summarize, there is little doubt about the presence of neuronal replay during sleep. The basic findings of Wilson and McNaughton have been replicated and extended in the rodent model and similar forms of reactivation are reported in other vertebrates including, possibly, humans. Historically, however, there has been some doubt about the significance of neuronal replay.
First, replay is not unique to sleep. Replay can be detected during periods of waking immobility and even during active exploration (Foster and Wilson 2006; Kudrimoti et al. 1999; O’Neill et al. 2006). This in turn suggests that replay may have little to do with central functions of sleep and is instead one of many phenomena that are peripherally modulated by sleep. While it remains possible that replay in sleep is qualitatively different than replay in wake, this has yet to be fully determined. Therefore sleep is sufficient but not necessary for replay.
Second, replay in sleep is generally not detected during learning but well after the animal learns the task. For example, most studies require that the animals be pretrained on a maze for several days to weeks before replay can be detected (Frank 2007; Peyrache et al. 2009). The slow appearance of replay might reflect a gradually developing engram that appears after initial learning and contributes to the transfer of memories from short-term stores (hippocampus) to long-term stores (neocortex). However, it could also indicate that replay is only a decaying reverberation of a very well-ingrained pattern of neural activity present during wakefulness. This may explain the ephemeral nature of replay. It is typically detectable only within the first 20–30 min of sleep and then fades away. In some measures, it also accounts for only a fraction of total variance in neuronal activity [reviewed in (Frank 2007)].
Unfortunately, very little data exist on the effects of novel experience on replay which might distinguish between these two possibilities. Some studies do show that neuronal activity patterns associated with novel maze running or motor behavior can be detected in sleep within a few days (as opposed to weeks for familiar mazes), but the novel tasks are often very similar to familiar tasks. For example, in one study there was substantial overlap in cells active in the familiar versus novel maze configurations [between 70 and 77 % (Kudrimoti et al. 1999)]. This issue seemed to be resolved by studies reporting novelty induced reactivation of waking activity patterns in the sleeping rat forebrain (Ribeiro 2004, 2007), but these findings have been challenged on technical and methodological grounds (Tatsuno et al. 2006). Recent findings from Peyrache et al., however, provide more compelling evidence that replay can occur following novel experience. In this study, rats were exposed to novel learning rules, and medial prefrontal cortex ensemble recordings showed that patterns of activity induced by learning “replayed” in subsequent NREM sleep (Peyrache et al. 2009).
A final consideration is that until quite recently, there has been no convincing evidence that replay in sleep has any function . Two independent studies in rodents provide evidence that interrupting the hippocampal bursts that convey replay impairs critically important behavior [learning and memory] (Ego-Stengel and Wilson 2010; Girardeau et al. 2009). These studies must be cautiously interpreted because they involved disruption of the hippocampal ripples and sharp waves, and not replay per se. It is also not clear if similar results would obtain if disruption were restricted to replay in wakefulness versus sleep. More recently, it has been shown that hippocampal replay during sleep can be triggered by presentation of auditory tones present during experience—which suggests that replay represents a memory trace (Bendor and Wilson 2012). Interestingly, similar experiments in humans lead to greater performance on memory tasks (Schönauer et al. 2013), and spontaneous replay can predict future performance (Deuker et al. 2013). These results strongly suggest that replay induces adaptive, functional plastic changes in the brain.
3.4 The Synaptic Homeostasis Hypothesis
Numerous theories have been proposed to explain how sleep may influence synaptic plasticity in the adult brain. In the last decade, SHY has received particular attention. Its hypothesized roles for sleep are not unique. Several theories proposing a synaptic weakening effect in either NREM or REM sleep predate SHY (Crick and Mitchison 1983, 1995; Giuditta 1995). Other scientists have also proposed mechanisms by which synapses might be weakened in sleep (Benington and Frank 2003; Poe et al. 2000). SHY, however, encompasses a number of perennial ideas about sleep function in one theory (e.g., metabolism, plasticity and homeostasis ). It also attempts to integrate Hebbian and non-Hebbian forms of plasticity across the sleep-wake cycle.
In the original description of SHY, learning occurs during wakefulness through synaptic potentiation which in turn drives sleep need. This form of synaptic potentiation appeared to be Hebbian LTP. First of all, it was described interchangeably as “long-term potentiation,” “LTP,” and “LTP-like” (Tononi and Cirelli 2003, 2006). The former terms classically refer to Hebbian mechanisms (Markram et al. 2011). Second, the synaptic potentiation of wakefulness involved “strong presynaptic firing…accompanied by postsynaptic depolarization” (Tononi and Cirelli 2006), which is a hallmark of correlation-based, or Hebbian LTP (Markram et al. 2011).
According to SHY, sleep weakens synapses through a process originally called downscaling (Tononi and Cirelli 2003, 2006), and more recently renamed as “synaptic renormalization” (Tononi and Cirelli 2012). The mechanisms governing renormalization are unspecified. However, the core features of synaptic renormalization are very similar to forms of non-Hebbian plasticity identified in the late 1990s. As originally described by Turrigiano et al. (1998), synaptic scaling (or homeostasis) is characterized by a global adjustment of synaptic weights in a neuron or network which is proportional to the strength at each synapse. Synaptic downscaling is proposed to offset Hebbian LTP, which if left unchecked would result in run-away synaptic strengthening that would saturate a neuron or neuronal network’s ability to form new synapses, or further strengthen existing ones (Turrigiano 1999, 2007; Turrigiano et al. 1998; Turrigiano and Nelson 2000). Therefore, the key concept of synaptic scaling is a global adjustment of synaptic weights that allows the network to retain past information, make new connections, and avoid network instability (i.e., a saturation of synaptic strength).
Synaptic renormalization has the same basic properties. In SHY, downscaling (or “renormalization”), affects all or most synapses, it offsets LTP (or LTP-like plasticity), and thereby allows more potentiation to occur (i.e., more learning). It involves a form of synaptic weakening (originally called “downscaling”) that is also proportional at each synapse (Tononi and Cirelli 2003, 2006). The consequences of unchecked synaptic potentiation in SHY are also similar to the network instability described in synaptic scaling , as sleep prevents “synaptic overload” (Tononi and Cirelli 2006). There appear to be only two principle differences between synaptic scaling proper and synaptic renormalization. First, in SHY LTP (or LTP-like plasticity) and downscaling are divided across wakefulness and sleep, respectively. Second, the cellular mechanisms governing synaptic scaling are not integrated into SHY. There are additional components to SHY not explicitly discussed in the original descriptions of synaptic scaling (e.g., metabolism, “synaptic space”). These, however, represent relatively minor differences or an emphasis on different outcomes of the same basic process.
3.5 Re-examining SHY
The theoretical underpinnings and evidence for SHY have been extensively reviewed elsewhere (Frank 2012; Tononi and Cirelli 2014). There is little doubt that under certain conditions and within some brain regions, synapses appear to be weaker after prolonged sleep periods. These findings are consistent with SHY and other theories that posit a similar synaptic weakening effect of sleep (Crick and Mitchison 1983; Giuditta 1995). Very little is known, however, about the mechanisms that drive this weakening process and whether they truly require sleep (Frank 2012, 2013).
For example, a central role was originally given to NREM slow wave activity (SWA), which was proposed to directly downscale synapses (Tononi and Cirelli 2003, 2006). This role has become obscure over the years, as SWA is sometimes also considered an “index” (Tononi 2009) or “sensor” of synaptic potentiation (Tononi and Cirelli 2012). Regardless of the particular role ascribed to SWA in SHY, there is currently no direct evidence that SWA in vivo weakens synapses (Frank 2012; Steriade and Timofeev 2003), and some findings indicating quite the opposite. For example, Tsanov and Manahan-Vaughan showed that when measured during the rodent light phase (when rodents sleep), evoked cortical excitatory postsynaptic potentials (EPSPs) do not decline across the sleep period, and peaks in SWA precede increases in EPSPs. These results suggest that in the adult visual cortex, sleep and possibly SWA, might promote synaptic strengthening. This is consistent with Tsanov and Manahan-Vaughan’s own conclusions, i.e., that the light (sleep) phase “…leads to synaptic potentiation” (Tsanov and Manahan-Vaughan 2007).
More direct evidence that SWA promotes synaptic potentiation comes from Chauvette et al. (2012) who showed that cortical postsynaptic potentials in vivo are potentiated after a period of NREM SWA, but not wakefulness. They also showed that periods of wakefulness did not result in synaptic potentiation. Intriguingly, experiments in vitro which simulated SWA specifically led to synaptic potentiation, while simulations of waking activity did not. It has been suggested that this is due to sleep inertia in the waking periods following sleep (Tononi and Cirelli 2014), but this is unlikely for several reasons. First, there were no significant differences in membrane potential in wake before or after NREM sleep (Timofeev personal communication). Second, the initial enhancement of the electrophysiological response persisted over several sleep-wake cycles, which would be unlikely if this was due to a transient inertial effect. Third, sleep inertia cannot explain the results of the experiments in vitro where membrane potential was controlled (Chauvette et al. 2012).
It also appears that synaptic scaling , as presently understood, does not operate in a manner consistent with SHY. As discussed above, the concepts of synaptic scaling are incorporated to a large degree into SHY. It is therefore reasonable to ask whether mechanisms known to govern synaptic downscaling might also govern “synaptic renormalization” in sleep. As recently reviewed (Frank 2012), many molecular and electrophysiological changes reported across the sleep-wake cycle are inconsistent with only global synaptic downscaling during sleep. For example, it has been suggested that global decreases in cortical activity (down-states) that occur during NREM sleep might downscale synapses. However, the basic principle of synaptic scaling is that global decreases in neuronal activity upscale synapses, while increases in neuronal activity downscale synapses. Consequently, down-states in NREM sleep should upscale, not downscale synapses (Frank 2012).
An additional consideration is that non-Hebbian synaptic scaling might be state-independent and thus occurs without sleep (Frank 2012). This possibility is supported by findings in the visual cortex in vivo. In one study, changes in cortical firing rates were chronically recorded after manipulations known to induce non-Hebbian synaptic scaling in the monocular segment of the visual cortex. There was no evidence of state-dependent alterations in scaling; rather decreases and increases in cortical activity were similar in wake and sleep (Hengen et al. 2013). Whether downscaling and upscaling both occur equally independently of vigilance state is presently unknown. Collectively, however, the above findings do not support a unique role for sleep in synaptic homeostasis.
Given the above considerations, it is not clear if the synaptic changes reported after sleep in support of SHY reflect an active sleep-dependent mechanism. They may instead result from other physiological processes that coincide with sleep, but are themselves not sleep-dependent (Frank 2012). These include circadian rhythms in brain temperature and in mammal’s glucocorticoid secretion.
3.6 Independent Factors that Alter Synapses: Brain Temperature
In mammals, the biological clock produces rhythms in brain temperature with a peak in the active phase and a trough in the sleep phase (Glotzbach and Heller 2000). Therefore, the effects of elevated brain temperature on synapses will accumulate during the normal waking period and decline during sleep. What might these effects be? A number of studies in vitro show that cooler temperatures cause a number of synaptic changes similar to those reported after sleep. These include a reduction in dendritic spines (Roelandse and Matus 2004) and concentrations of proteins that make up the postsynaptic density (Roelandse and Matus 2004). Cooling also reversibly reduces excitatory postsynaptic field potentials (EPSPs), and reverses (de-potentiates) LTP (Bittar and Muller 1993) and reduces cortical synaptic strength as measured by mini-EPSPs (Simkus and Stricker 2002).
Strong effects of naturally occurring brain temperature gradients on EPSPs are also reported in freely behaving rodents (Moser et al. 1993). Prior to Moser’s now classic studies, field recordings from the hippocampus (EPSPs) in vivo were thought to exclusively reflect plastic changes associated with learning or novel experience. They were higher after rodents engaged their environments during exploration, but not when they were less mobile. However, motor activity alone increases hippocampal temperature and EPSPs in a manner unrelated to learning-related plasticity. It is instead caused by the normal rise in brain temperature associated with waking movement and dissipates as the brain naturally cools. Similar temperature gradients across the subjective day and night have been reported in rodent cortex (Franken et al. 1991, 1993). It is also interesting that reports of heightened synaptic potentiation in wake versus sleep based on electrophysiological recordings rely on various forms of novel experience to maintain wakefulness (Hanlon et al. 2011; Vyazovskiy et al. 2008). Therefore reports of heightened cortical excitability or responsiveness in mammals after prolonged wakefulness or sleep deprivation (relative to sleep) may reflect circadian rhythms in brain temperature and secondary increases associated with motor activity—rather than vigilance state per se.
The effects of temperature may be even more extreme in insects commonly used in sleep studies. Insects are ectotherms and do not internally generate heat as do mammals. Temperature is instead behaviorally regulated either by selecting warmer environments or through activity (Stevenson 1985). Temperature gradients as small as ≈8° C are sufficient to alter synaptic structures in Drosophila (Peng et al. 2007; Zhong and Wu 2004). These include increased axonal arborization in mushroom body neurons (Peng et al. 2007) and motor nerve terminals in vivo (Zhong and Wu 2004) and neurite extension in vitro (Peng et al. 2007). Intriguingly, these temperature effects are mediated by signaling pathways shared by activity-dependent synaptic plasticity [e.g., cAMP] (Peng et al. 2007). Whether similar temperature gradients exist across Drosophila wake and sleep is unknown, but similar gradients in ambient temperature are encountered under natural conditions (Vanin et al. 2012). They may even occur in insects housed under constant ambient temperatures. This is because core temperature tracks motor/muscle activity in small terrestrial insects (Stevenson 1985); processes which are strongly influenced by the biological clock. Interestingly, this may be especially true for flying insects which expend considerable energy to bring thoracic flight muscles to high temperatures prior to flight [a phenomenon called “pre-flight warm-up”] (Heinrich 1974). Although, as discussed by Heinrich, small flying insects in flight will dissipate increases in core temperature due to convection, this may not be true under housing conditions typically used in sleep studies of Drosophila. Typically, Drosophila are housed in glass tubes that prevent flight and the convection that would occur as the animal flies through a large space. These tubes, however, do not prevent nonflight motor activity, including presumably, movements of the wings which are part of the pre-flight warm-up.
3.7 Independent Factors that Alter Synapses: Glucocorticoids
In rodents and humans, glucocorticoid (corticosterone and cortisol) concentrations rise and fall in parallel with the circadian wake and sleep phases of the 24 h day (Van Cauter 2005). They also are higher during wakefulness (relative to sleep), when waking is enforced during the normal sleep phase. There is considerable evidence that outputs of the Hypothalamic-Pituitary-Axis (HPA) profoundly influence synaptic efficacy and morphology. Glucocorticoid effects are diverse and dependent upon different classes of receptors (Joels et al. 2008). They have also been chiefly explored in the hippocampus rather than the neocortex. Nevertheless, increases in corticosterone during the normal waking period or after sleep deprivation may generally promote glutamatergic neurotransmission and neuronal excitability (Joels et al. 2008). Acute increases in corticosterone (or stress) increase the frequency (Olijslagers et al. 2008) and amplitude of mEPSCs in the hippocampus (Karst and Joels 2005), strengthen glutamatergic synapses onto dopamine neurons (Daftary et al. 2009), and increase glutamatergic release/calcium mobilization in cortical synaptoneurosomes (Satoh and Shimeki 2010). Acute increases in corticosterone also promote AMPAR synaptic transmission, AMPAR trafficking and insertion into cortical and hippocampal synapses, and cortical dendritic spine turnover (Conboy and Sandi 2009; Groc et al. 2008; Liu et al. 2010; Yuen et al. 2011). More recently it has been shown that even relatively small, transient increases in exogenous corticosterone can lead to rapid spinogenesis in vivo, which slowly decline over 5 h (Komatsuzaki et al. 2012).
Perhaps the most compelling evidence to date that normal circadian cycles of HPA activity influence cortical synaptic plasticity comes from Liston et al. (2013). In this study, it was shown that the normal peaks in glucocorticoid concentrations (during the rodent active phase) directly promote cortical dendritic spine formation that accompanies motor learning. Interestingly, they also found that the normal troughs (which correspond to the sleep phase) had dual effects; they promote the stabilization of newly formed spines associated with learning, and the pruning of preexisting spines, not associated with learning. These findings are consistent with previously reported biphasic effects of glucocorticoids which are comprised of rapid increases in synaptic efficacy (and spine formation) followed by a slower, time-dependent normalization of synapses to baseline levels [for discussion, see (Joels et al. 2008; Tse et al. 2012)]. These biphasic and prolonged synaptic changes are strikingly similar to those ascribed to wakefulness and sleep in SHY. They are, however, ultimately driven by the biological clock and are thus not state-dependent.
4 Discussion
There has been impressive progress in our understanding of how sleep and sleep loss impact brain plasticity. There also remain a number of unresolved issues. For example, while abundant evidence exists to support a general synaptic weakening after sleep, it is not at all clear that these changes are caused by sleep. Many findings cited in support of one theory of sleep-dependent plasticity (SHY) can be explained by circadian rhythms in brain temperature and HPA activity. There is also strong evidence that under certain conditions, sleep may instead strengthen synapses. These include changes in sensory input during early life that lead to cortical re-mapping. I discuss these topics in more detail in the following sections.
4.1 Brain Plasticity in Adult and Developing Brains: Difference in Degree or Kind?
An important unanswered question is whether sleep-dependent plasticity in the developing and adult brain is different. It has been suggested, for example, that synaptic downscaling as described in SHY is even more important during early life. For example, during times of overall synaptogenesis (Tononi and Cirelli 2012). However, if these developmental ages are characterized by an overall gain of synapses (Aghajanian and Bloom 1967; Sur and Leamey 2001), and animals at these ages spend most of their time in sleep [e.g., rats ≈75 % (Frank and Heller 1997), ferrets (Thurber et al. 2008) ≈85 % total recording time], then it follows that sleep cannot be a time for net synaptic weakening. This could only be true if all the synaptogenesis is compressed into the tiny fraction of time spent awake. This seems highly unlikely. Indeed, recent work in infant rodents indicates that bursts of activity during sleep are well-suited for forming sensory/motor circuits, a process known to involve synapse formation (Khazipov et al. 2004; Tiriac et al. 2012).
It also appears that a global downscaling function for sleep cannot fully explain experience-dependent plasticity occurring at later developmental ages (Fig. 3). On the contrary, investigations into a classic, physiological form of developmental plasticity (ODP) show unequivocally that sleep potentiates responses in some circuits, while maintaining depression in others. In addition, sleep appears to play no special role in at least one type of synaptic scaling in vivo in rodents at similar ages (Hengen et al. 2013). Reconciling these findings with SHY is complicated by the fact that while progress has been made identifying the cellular mechanisms governing sleep-dependent ODP (from receptors to circuits (Frank 2011), very little is known about the mechanisms governing SHY. It is also unclear if synaptic changes ascribed to sleep in SHY are in fact sleep-dependent (Frank 2012). Nevertheless, it remains possible that both Hebbian and non-Hebbian forms of plasticity operate during sleep in early life. For example, the effects of sleep on ODP suggest that synaptic weakening and strengthening both occur during sleep, but at different time points. The first few hours of post-MD sleep are accompanied by activation of several kinases and the synthesis of proteins implicated in LTP (Aton et al. 2009; Seibt et al. 2012). However, these events are transient, and by 6 h they return to baseline or even drop below baseline values. This is consistent with a “Boom and Bust” model shown in Fig. 3, according to which sleep first leads to synaptic potentiation, and then a general synaptic downscaling. Similar models that integrate synaptic strengthening and weakening across sleep have been proposed for the adult brain (Genzel et al. 2014; Ribeiro 2011).
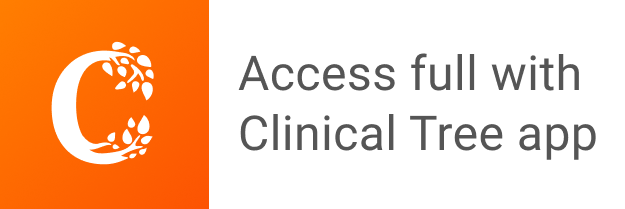