Fig. 2.1
Components of a basic test accuracy question with examples. The top row gives the terminology used. Other rows give examples of varying complexity; these include both the traditional “cross-sectional” assessment and a delayed verification based study (bottom row)
2.3.1 Index Test
The index test is the assessment or tool of interest. Index tests in dementia take many forms—examples include cognitive screening tests (e.g., MMSE [8]); tissue/imaging based biomarkers (e.g., cerebrospinal fluid proteins) or clinical examination features (e.g., presence of anosmia for diagnosis of certain dementias).
The classical test accuracy paradigm requires binary classification of the index test. However, many tests used in clinical practice, particularly those used in dementia, are not binary in nature. Taking MMSE as an example, the test can give a range of scores suggestive of cognitive decline. In this situation, criteria for determining test positivity are required to create a dichotomy (test positive and test negative). The score at which the test is considered positive or negative is often referred to as a cut-point or threshold. Thresholds may vary depending on the purpose and setting of the assessment. For example in many acute stroke units, the suggested threshold MMSE score is lower than that often used in memory clinic settings [9]. Sometimes, within a particular setting, a range of thresholds may be used in practice and test accuracy can be described for each threshold [6, 9].
In many fields there is more than one potential index test and the clinician will want to know which test has the best properties for a certain population. Ideally, the diagnostic accuracy of competing alternative index tests should be compared in the same study population. Such head-to-head evaluations may compare tests to identify the best performing test(s) or assess the incremental gain in accuracy of a combination of tests relative to the performance of one of the component tests [10]. Well-designed comparative studies are invaluable for clinical decision making because they can facilitate evaluation of new tests against existing testing pathways and guide test selection [11]. However, many test evaluations have focused on the accuracy of a single test without addressing clinically important comparative questions [12, 13].
A DTA study can compare tests by either giving all patients all the tests (within-subject or paired design) or by randomly assigning a test to each subject (randomized design). In both designs, all patients are verified using the same gold or reference standard. As an example, Martinelli et al. [14] used the within-subject design to compare the accuracy of neuropsychological tests for differentiating Alzheimer’s disease from the syndrome of mild cognitive impairment (MCI). Although comparative accuracy studies are generally scarce, the within-subject design is more common than the randomized design [12]. Nevertheless, both designs are valid and relevant comparative studies should be more routinely conducted.
2.3.2 Target Condition
The target condition is the disease or syndrome or state that you wish to diagnose or differentiate. When considering a test accuracy study of cognitive assessment, the target condition would seem intuitive—diagnosis of dementia. However, dementia is a syndrome and within the dementia rubric there are degrees of severity, pathological diagnoses and clinical presentations [4]. The complexity is even greater if we consider the broader syndrome of cognitive impairment.
As a central characteristic of dementia is the progressive nature of the disorder, some have chosen to define an alternative target condition as development of dementia in a population free of dementia at point of assessment [15]. This paradigm is based on the argument that evidence of cognitive and functional decline over time is a more clinically valid marker than a cross-sectional “snap shot”. For example, we may wish to evaluate the ability of detailed structural brain imaging to distinguish which patients from a population with MCI will develop frank dementia. This study design is often used when assessing biomarkers that purport to define a pre-clinical stage of dementia progression [16]. The approach can be described as longitudinal, predictive or ‘delayed verification’ because it includes a necessary period of follow up.
In formulating a question or in reading a DTA paper it is important to be clear about the nature of the target condition. We should be cautious of extrapolating DTA results from a narrow to a broader target condition; interpretation of results is particularly difficult if the disease definition is ambiguous or simply not described. For example, the original derivation and validation work around the MoCA focused on community dwelling older adults with MCI [17]. Some have taken the favorable test accuracy reported in these studies and used this to endorse the use of MoCA for assessment of all cause dementia [18]. The ideal would be that MoCA is subject to further assessments of test accuracy for this new target condition.
2.3.3 Reference Standard
The gold or reference standard is the means of verifying the presence or absence of the target condition. There is no gold standard for many conditions, hence the use of the term reference standard. The reference standard is the best available test for determining the correct final diagnosis and may be a single test or a combination of multiple pieces of information (composite reference standard) [19]. The term gold standard is particularly misleading in studies with a dementia focus. There is no in-vivo, consensus standard for diagnosis of the dementias [20]. Historically, neuropathological examination was considered the gold standard, however availability of subjects is limited and the validity of neuropathological labels for older adults with dementia has been questioned [21]. Thus we have no single or combination assessment strategy that will perfectly classify “positive” and “negative” dementia status. This lack of a gold standard is not unique to cognitive test accuracy studies, but it is particularly relevant to dementia where there is ongoing debate regarding the optimal diagnostic approach [22].
Rather than use a gold standard, many studies employ a reference standard that approximates to the (theoretical) gold standard as closely as possible. A common reference standard is clinical diagnosis of dementia using a recognized classification system such as International Classification of Disease (ICD) or Diagnostic and Statistical Manual of Mental Disorders (DSM). Validated and consensus diagnostic classifications are also available for dementia subtypes such as Alzheimer’s disease dementia and vascular dementia and these may be preferable where the focus is on a particular pathological type.
2.3.4 Target Population
The final, often forgotten, but crucial part of the test accuracy question is the population that will be tested with the index test. It is known that test accuracy varies with the characteristics of the population (i.e., spectrum) being tested [23, 24]. Therefore, it is important to describe the clinical context in which testing takes place, presenting features and any tests received by participants prior to being referred for the index test (i.e., the referral filter). Cognitive assessment may be performed for different purposes in different settings. The prevalence, severity and case-mix of cognitive syndromes will differ accordingly and this will impact on test properties and interpretation of results. For example a multi-domain cognitive screening tool will perform differently when used by a General Practitioner assessing someone with subjective memory problems compared to a tertiary specialist memory clinic assessing an inpatient referred from secondary care [25, 26]. In describing the context of testing it is useful to give some detail on the clinical pathway in routine care; whether there will have been any prior cognitive testing; the background and experience of the assessor and the supplementary tools available.
2.4 Test Accuracy Metrics
The perfect index test will correctly classify all subjects assessed, i.e., no false negatives and no false positives. However, in clinical practice such a test is unlikely to exist and so the ability of an index test to discriminate between those with and without the target condition needs to be quantified. Different metrics are available for expressing test accuracy, and these may be paired or single descriptors of test performance. Where a test is measured on a continuum, such as the MMSE, paired measures relate to test performance at a particular threshold. Some single measures are also threshold specific while others are global, assessing performance across all possible thresholds.
The foundation for all test accuracy measures is the two by two table, describing the results of the index test cross classified against those of the reference standard [27]. The four cells of the table give the number of true positives, false positives, true negatives and false negatives (Table 2.1). We have summarized some of the measures that can be derived from the table (Table 2.2). Paired measures such as sensitivity and specificity, positive and negative predictive values, and positive and negative likelihood ratios (LR+ and LR–), are typically used to quantify test performance because of the need to distinguish between the presence and absence of the target condition. We will focus our discussion below on two of these commonly used paired measures and one global measure derived from receiver operating characteristic (ROC) curves.
Table 2.1
Cross classification of index test and reference standard results in a two by two table
Dementia present (or other target condition) | Dementia absent (or other target condition) | ||
---|---|---|---|
Index test positive | True positives (a) | False positives (b) | Positive predictive value =number of true positives ÷ number of test positives |
Index test negative | False negatives (c) | True negatives (d) | Negative predictive value =number of true negatives ÷ number of test negatives |
Sensitivity =number of true positives ÷ number with dementia | Specificity = number of true negatives ÷ number without dementia |
Table 2.2
Some of the potential measures of test accuracy that can be derived from a two by two table
Test accuracy metric | Formula |
---|---|
Paired measures of test performance | |
Sensitivity | a/(a + c) |
Specificity | d/(b + d) |
Positive predictive value (PPV) | a/(a + b) |
Negative predictive value (NPV) | d/(c + d) |
False positive rate | 1 – specificity |
False negative rate | 1 – sensitivity |
False alarm rate | 1 – PPV |
False reassurance rate | 1 – NPV |
Positive likelihood ratio (LR+) | Sensitivity/(1 – specificity) |
Negative likelihood ratio (LR−) | (1 – sensitivity)/specificity |
Clinical utility index (positive) | Sensitivity × PPV (rule in) |
Clinical utility index (negative) | Specificity × NPV (rule out) |
Single measures of test performance | |
Diagnostic odds ratio (DOR) | ad/bc |
Overall test accuracy | (a + d)/(a + b + c + d) |
Youden index | Sensitivity + specificity – 1 |
2.4.1 Sensitivity and Specificity
Sensitivity and specificity are the most commonly reported measures [28]. Sensitivity is the probability that those with the target condition are correctly identified as having the condition while specificity is the probability that those without the target condition are correctly identified as not having the condition. Sensitivity and specificity are reported as percentages or proportions. Sensitivity and specificity are not conditional upon the prevalence of the condition of interest within the population being tested. Sensitivity is also known as the true positive rate (TPR), true positive fraction (TPF) or detection rate, and specificity as the true negative rate (TNR) or true negative fraction (TNF). The false positive rate (FPR) or false positive fraction (FPF), 1–specificity, is sometimes used instead of specificity. There is a trade-off between sensitivity and specificity (a negative correlation) induced by varying threshold. For example by increasing the threshold for defining test positivity on MMSE we decrease sensitivity (more false negatives) and increase specificity (fewer false positives) (Fig. 2.2). This is explained further in the section on ROC plots.


Fig. 2.2
Graphical illustration of test accuracy at a threshold (Used with permission of Professor Nicola Cooper and Professor Alex Sutton, University of Leicester)
2.4.2 Predictive Values
The positive predictive value (PPV) is the probability that subjects with a positive test result truly have the disease while the negative predictive value (NPV) is the probability that subjects with a negative test result truly do not have the disease. Thus, predictive values are conditional on test result unlike sensitivity and specificity which are conditional on disease status. As discussed earlier, the spectrum of disease in a population is dependent on prevalence, disease severity, clinical setting and prior testing. While all measures are susceptible to disease spectrum, predictive values are directly related and mathematically dependent on prevalence as illustrated in Fig. 2.3. As predictive values tell us something about the probability of the presence or absence of the target condition for the individual patient given a particular test result, predictive values potentially have greater clinical utility than sensitivity and specificity [29]. However, because predictive values are directly dependent on prevalence, they are difficult to generalize even within the same setting and should not be derived from studies that artificially create prevalence such as in diagnostic case-control studies.


Fig. 2.3
Impact of prevalence on predictive values. For a hypothetical cognitive screening test with a sensitivity of 85 % and a specificity of 80 %, the plot in (a) shows a positive relationship between positive predictive values and prevalence while the plot in (b) shows a negative relationship between negative predictive values and prevalence
2.4.3 Receiver Operating Characteristic (ROC) Plots
A receiver operating characteristic (ROC) plot is a graphical illustration of the trade-off between sensitivity and specificity across a range of thresholds [30]. Thus, the ROC plot demonstrates the impact of changing threshold on the sensitivity and specificity of the index test. Traditionally, the ROC plot is a plot of sensitivity against 1-specificity. The position of the ROC curve depends on the discriminatory ability of the test, the more accurate the test, the closer the curve to the upper left hand corner of the plot. A test that performs no better than chance would have a ROC curve along the 45° axis (Fig. 2.4).


Fig. 2.4
ROC plot. AUC area under the curve. The ROC plot shows the ROC curve (solid line) for a hypothetical cognitive screening test with a high AUC of 0.99 and another ROC curve (dashed line) for an uninformative test with an AUC of 0.5
The area under the curve (AUC) is a global measure of test accuracy commonly used to quantify the ROC curve. The AUC represents the probability that a randomly chosen diseased subject is (correctly) rated or ranked with greater suspicion than a randomly chosen non-diseased subject [31]. An AUC of 0.5, equivalent to a ROC curve along the 45° axis, indicates that the test provides no additional information beyond chance; an AUC of 1 indicates perfect discrimination of the index test. A classical ROC curve includes a range of thresholds which may be clinically irrelevant; calculation of a partial AUC that is restricted to clinically meaningful thresholds is a potential solution [32].
ROC curves and AUCs are often described in medical papers [28]. However, in isolation, the clinical utility of the AUC is limited. AUCs are not unique; two tests—one with high sensitivity and low specificity, and the other with high specificity and low sensitivity—may have the same AUC. Furthermore, the AUC does not provide any information about how patients are misclassified (i.e., false positive or false negative) and should therefore be reported alongside paired test accuracy measures that provide information about error rates. These error rates are important for judging the extent and likely impact of downstream consequences [33].
2.5 Interpreting Test Accuracy Results
It is often asked, what is an acceptable sensitivity and specificity for a test? There are broad rules of thumb, for example, if a test is used to rule out disease it must have high sensitivity, and if a test is used to rule in disease it must have high specificity. However, the truth is that there is no “optimal”, the best trade-off of sensitivity and specificity depends on the clinical context of testing and consequences of test errors [34]. In clinical practice there may be different implications for false positive and false negative test results and so in some situations sensitivity may be preferred with a trade-off of lower specificity or vice-versa. We can illustrate this using a real world example of a dementia biomarker. Cerebrospinal fluid based protein (amyloid, tau) levels are said to change in preclinical stages of Alzheimer’s disease and have been proposed as an early diagnostic test for this dementia type [35]. If the test gives a false negative result in a middle aged person with early stage Alzheimer’s disease, then the person will be misdiagnosed as normal. The effects of this misdiagnosis are debatable, but as the natural history of preclinical disease states is unknown and as we have no proven preventative treatment, the misdiagnosis is unlikely to cause substantial problems. If another person without early stage Alzheimer’s disease receives a false positive result, they will be misdiagnosed as having a progressive neurodegenerative condition with likely substantial negative effects on psychological health [36]. In this situation we would want the test to be highly specific and would accept a poorer sensitivity.
Test accuracy is a fundamental part of the evaluation of medical tests; but it is only part of the evaluation process. Test accuracy is not a measure of clinical effectiveness and improved accuracy does not necessarily result in improved patient outcomes. Although test accuracy can potentially be linked to the accuracy of clinical decision making through the downstream consequences of true positive, false positive, false negative and true negative test results, benefits and harms to patients may be driven by other factors too [37]. Testing represents the first step of a test-plus-treatment pathway and changes to components of this pathway following the introduction of a new test could trigger changes in health outcomes [38]. Potential mechanisms have been described as resulting from direct effects of testing, changes to diagnostic and treatment decisions or timeframes, and alteration of patient and clinician perceptions [38]. Therefore, diagnostic testing can impact on the patient journey in ways that may not be predicted based on sensitivity and specificity alone.
In addition to the classical test accuracy metrics, measures that go beyond test accuracy to look at the clinical implications of a test strategy are available [37]. Important aspects will include feasibility of testing, interpretability of test data, acceptability of the test and clinician confidence in the test result. At present there are few studies looking at these measures for dementia tests [39]. Where a test impacts on clinical care, we can describe the proportion of people receiving an appropriate diagnosis (diagnostic yield) and the proportion that will go on to receive appropriate treatment (treatment yield) [40]. Where a test is added to an existing screening regime, we can describe the incremental value of this additional test [41]. In a recent study looking at imaging and CSF biomarkers, the authors found reasonable test accuracy of the biomarkers, but when considered in the context of standard memory testing there was little additional value of these sophisticated tests (calculated using a net re-classification index) [42].
2.6 Issues in Cognitive Test Accuracy
While we have kept our discussion of DTA relevant to dementia assessment, many of the issues covered so far are generic and common to many test accuracy studies. Nevertheless, there are certain issues that are pertinent in the field of cognitive assessment [7, 43].
2.6.1 Reference Standards for Dementia
We have previously alluded to the difficulty in defining an acceptable reference standard for dementia [20, 22]. Many of the reference standards used in published dementia DTA studies (postmortem verification, scores on standardized neuropsychological assessment and progression from MCI to dementia due to Alzheimer’s disease) have limitations with attendant risk of disease misclassification [7, 21]. Clinical diagnosis made with reference to a validated classification system is probably the preferable option, but even this is operator dependant and has a degree of inter-observer variation [44, 45]. The issue is further complicated by the different classification criteria that are available, for example, agreement on what constitutes dementia varies between ICD and DSM [46]. For creating our two by two table, we require a clear distinction between target condition positive and negative. In clinical practice, dementia diagnosis is often more nuanced, particularly on initial assessments and we often qualify the diagnosis with descriptors like “possible” or “probable”. Incorporating this diagnostic uncertainty into classical test accuracy is challenging.
The use of detailed neuropsychological assessment is often employed as a reference standard and warrants some consideration. Testing across individual cognitive domains by a trained specialist provides a comprehensive overview of cognition. However, conducting the battery of tests is time consuming (much greater than the 15 min suggested by the Research Committee of the American Neuropsychiatric Association) [47] and not always practical, economical or acceptable to patients. This can lead to biases in data from differential non-completion of the reference standard (see Sect. 2.6.2). Also, classical neuropsychological testing does not offer assessment of the functional impact of cognitive problems, a key criterion for making the diagnosis of dementia [48]. In some DTA primary studies and systematic reviews, clinical diagnosis and neuropsychological testing are used interchangeably as reference standards but the two approaches are not synonymous. In general, to avoid bias when analyzing test accuracy, the same reference standard should be applied to the whole study population.
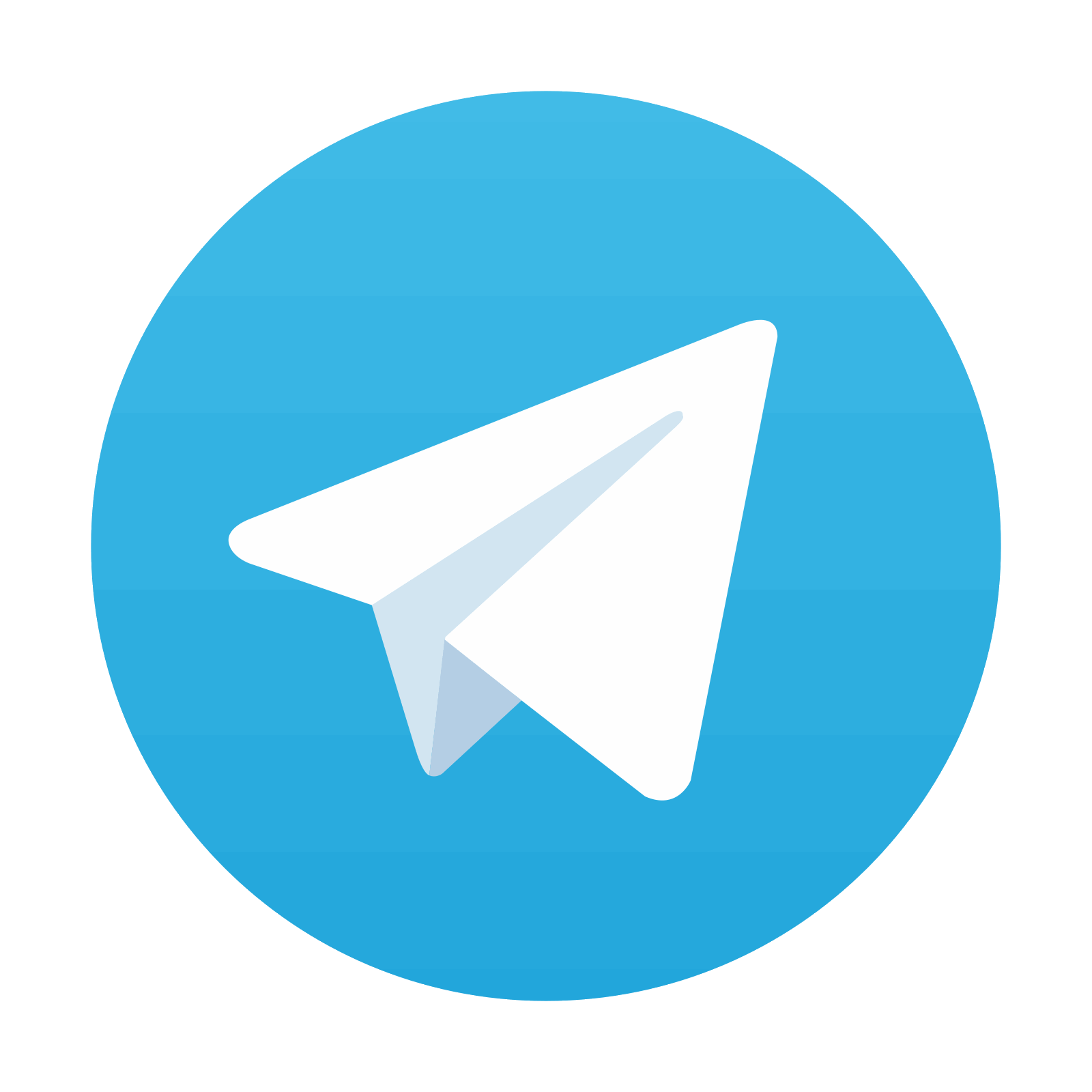
Stay updated, free articles. Join our Telegram channel

Full access? Get Clinical Tree
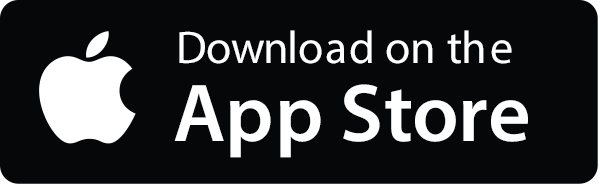
