, Daniel Campbell1 and Fred R. Volkmar1
(1)
Yale Child Study Center, New Haven, USA
Keywords
DiagnosisSpectrumContinuumIn 1943, Leo Kanner described 11 children as having come into the world without the usual disposition to make social contact, a condition he called early infantile autism (Kanner 1943). In his description of these 11 children, Kanner noted that despite limited interest in the social world, they were highly engaged with nonsocial aspects of the environment and had difficulties with change. In 1944, Hans Asperger, an Austrian pediatrician, described four children who had difficulty integrating socially into groups despite seemingly adequate cognitive and verbal skills, a condition he called autistischen psychopathen im kindesalter, which translates in English to “autistic personality disorders in childhood” (Asperger 1944). Asperger was apparently unaware of Kanner’s classic description of autism, thus the focus both authors made on the marked social dysfunction is remarkable and speaks to the centrality of social deficits as the defining feature of these disorders. Beginning with Wing’s seminal work and description of the condition (Wing 1981), interest in Asperger’s syndrome has increased greatly, leading to inclusion of Asperger’s syndrome in American Psychiatric Association’s (APA) Diagnostic and Statistical Manual of Mental Disease, fourth edition (DSM-IV; American Psychiatric Association 1994) and the World Health Organization’s (WHO) International Classification of Disease, tenth edition (ICD-10; World Health Organization 1992) culminating in the recognition of a broad spectrum of individuals with social disability that form what we now term autism spectrum disorders (ASDs) .
The current diagnostic taxonomy of the DSM-IV (APA 1994) and the ICD-10 (WHO 1992) place autism, Asperger’s disorder, and three related social disabilities in the category of pervasive developmental disorders (PDDs). The three most common PDDs (autistic disorder, Asperger’s disorder, and pervasive developmental disorder, not otherwise specified (PDD-NOS)), are often referred to more generally as “autism spectrum disorders”. Autistic disorder is characterized by severe social deficits, impaired communication skills, the presence of restricted and repetitive behaviors, and an onset in early childhood. Asperger’s disorder differs from autistic disorder in (a) omission of the diagnostic criteria in the communication domain; (b) absence of a requirement for onset prior to age three; and (c) addition of criteria specifying impairing dysfunction, absence of a language delay, and absence of deficits in cognitive development or nonsocial adaptive function. Furthermore, a precedence rule indicates that, to meet criteria for Asperger’s disorder, one cannot meet criteria for another specific PDD. PDD-NOS denotes a subthreshold form of autism, or a manifestation of PDD that is atypical in terms of onset patterns or symptomatology such that defining features of other PDDs are not met. Diagnosis requires that the individual exhibits autistic-like social difficulty along with impairment in either communication or restricted and repetitive interests or behaviors.
Most people now consider autism to be a spectrum of disorder(s). However, there has been much controversy regarding the distinctiveness of the different subtypes and the ability of clinicians to accurately and reliably distinguish between them (Charman et al. 2009; Baird et al. 2003; Volkmar and Klin 2005; Lord and Bishop 2009). In this chapter, we set out to address, at least in part, some of this confusion and provide a systematic way of thinking about the classification of disorders that reside along a spectrum. We begin the chapter by providing an overview of two classification approaches (categorical and dimensional), which is followed by a discussion of statistical techniques that can be used to subtype and classify disorders. Next, a description of the current categories of PDDs is provided along with a discussion of several pertinent areas of debate. The chapter then describes the changes in diagnostic criteria in DSM-5 and a discussion of the implications that the changes might bring. We close the chapter by providing our concluding thoughts and areas for future research.
Categorical vs. Dimensional Classification Approaches
A host of considerations arise in the development of any approach to classification. Major considerations include overall goals and purpose (e.g., is the primary purpose rigorous definition for research purposes or broader definitions for clinical use? Will subthreshold conditions be included and if so how? Can information on other conditions (medical conditions or comorbid psychiatric/developmental ones) also be coded ? Approaches to diagnosis (both categorical and dimensional) lose their value if they are overly broad or overly narrow. Similarly, if the intended use is for research, definitions must be more specific and detailed while for general clinical, use a different approach might be used. The US (DSM) system has consistently been “dual use” while the international (ICD) approach has different volumes of detailed research criteria as well as a more general and descriptive cynical guideline (Rutter 2011).
Categorical Approaches
Medical classification systems arose in the context of important public health issues (e.g., controlling infection) and an awareness of the need to monitor public health at a macro level (e.g., causes of mortality). These systems tend to be categorical, although dimensional approaches can also be used quite readily (see Rutter 1992; Volkmar et al. 2005; Rutter 2011). Both DSM-IV and ICD-10 adopt an explicit dichotomous categories approach (i.e., an individual does or does not have a specific disorder) although they differ in some respects (as noted above in the dual approach vs. unitary approach and also in some other ways as in the overall approach to comorbid conditions).
The DSM-IV and ICD-10 categorical approaches are very closely related and both definitions were based on the results of a large international field trial (Volkmar et al. 1994). As part of this, 21 sites with more than 100 raters provided information on nearly 1,000 cases who were included if autism was reasonably thought to be a part of differential diagnosis. The sample exhibited a range of ages, levels of functioning, and severity, and in most cases, raters felt the quality of information available to them for their ratings was good to excellent. Based on a series of preliminary data reanalyses, it was agreed that the system developed for autism should aim to have a reasonable balance of sensitivity and specificity across the IQ range as well as age. Interrater reliability of individual criteria was generally good to excellent (see Volkmar et al. 1994). The final definition included 12 criteria grouped in three categories (social, communication-play, and restricted interests and behaviors) with a minimum requirement of a total of six criteria, two of which had to be social ones (the latter in view of the strength of social features in predicting diagnosis). In addition, data were felt to be sufficient to include several disorders “new” to DSM-IV and/or ICD-10. These included Asperger’s disorder, Rett’s disorder, and childhood disintegrative disorder (see Volkmar et al. 1994, 2005 for a review). This approach has proven relatively robust probably because it is readily applicable and because the system is the same for both DSM-IV and ICD-10. The large increase in research papers, from about 300 published in 1992 to over 2,400 during 2012, is a testament to the utility of the system. At the same time, issues have been raised, particularly about the definition of Asperger’s disorder (Volkmar and Klin 2005), and major changes are planned for the upcoming revisions to the DSM, which are discussed later in this chapter.
Dimensional Approaches
Even with a categorical approach there is an awareness that symptoms may exist on a dimension, e.g., of function or of dysfunction. For example, blood pressure, IQ, height, and weight are all dimensional measures, but by convention (ideally based on good data), some threshold may be selected for a categorical diagnosis like hypertension and intellectual disability. In this regard, dimensional approaches offer many advantages.
Dimensional approaches in autism can take various forms. These include the use of standardized normative assessments (e.g., of intelligence, communication, motor development, adaptive behavior; Klin et al. 2005). Some work has even used a normative measure of social competence, for example, on the Vineland Adaptive Behavior Scales (Volkmar et al. 1987). Other instruments focus more on abnormal behaviors or development, e.g., the Childhood Autism Rating Scale (Schopler et al. 1980) which assess 15 kinds of behaviors on a continuum of severity ranging from 0 (normal) to 4 (severely autistic). More recently, the approach as in the Autism Diagnostic Schedule (ADOS; Lord et al. 2000) and the Autism Diagnostic Interview -Revised (ADI; Lord et al. 1997) has been to focus on assessments that can be related to (and thus operationalized in greater detail) formal categorical criteria. The ability to “cross walk” back to categorical criteria has many advantages for research purposes. On the other hand, instruments that take considerable training may be impractical for general clinical use, i.e., a general practitioner who wants a simple description of the condition and clinical guidelines would not be able to obtain advanced training easily, and thus might opt not to use standardized instruments for diagnosing autism in their practice. For that individual another set of issues arise in terms of guidelines for screening (and practice; Hyman and Johnson 2012).
Screening instruments
Issues in the development of screening approaches to autism raises other issues (see Barton et al. 2012). Level I screening is intended for general developmental screening while Level II screeners focus more specifically on autism. A recent trend has been the encouragement of simple screeners useful in general practice for assessment of relatively young children (see Coonrod and Stone 2005 and Hyman and Johnson 2012); however, practical issues arise given the relatively small number of clearly relevant developmental and behavioral milestones observed in the youngest children (see Chawarska and Volkmar 2005; Volkmar et al. 2005, 2007). A number of excellent instruments are available (see Barton et al. 2012; Conrod and Stone 2005; Johnson and Myers 2007), although as Hyman and Johnson (2012) have emphasized, there are many areas of potential difficulty with only a small proportion of cases that screen positive eventually receiving services. Another problem is that issues of diagnosis can be particularly challenging for infants under three and particularly under 18 months when some features (often social and communication ones) may be present but restricted interests have yet to develop to the threshold level (e.g., the child with unusual sensory interests who does not yet manifest the range of restricted interests typically required). Another set of challenges arise for screening relative to older individuals (those above age three in this context) and to those at the two “ends” of the spectrum in terms of cognitive functioning (see Campbell 2005, Coonrood and Stone 2005; Reilly et al. 2009). A few behaviors, e.g., attachments to unusual objects, are predictive at one age but not another, and for this reason, this symptom was not used in the final DSM-IV/ICD-10 definitions. For all, screening instruments, many different issues arise pertaining to the intended user and context (parent report, observation, or both). For parent report, issues of parental perception, age of child, and problems like “telescoping” effects are a complication in terms of reliability and the potential significance of low-frequency behaviors, particularly in older children (Lord and Corsello 2005). Observational approaches have their own limitations, including the potential of missing important behaviors of low frequency but diagnostic importance, thus giving rise to issues with reliability and validity.
Statistical Approaches to Diagnosis
The assignment of individuals to known diagnostic categories and the determination of whether such categories exist within a class of disorders are two distinct but complementary questions that can both be addressed using statistical methods . The main difference between the two approaches to diagnostic categorization is in whether a set of categories already exists, and one wishes to determine how well the categories describe individuals in different groups and how well they are separated, or if no such categories exist, and one attempts to infer them from the data if possible. The former is termed classification and the latter clustering or subtyping, and the distinction between them parallels a fundamental difference in statistics and machine learning between supervised and unsupervised learning methods (Hastie et al. 2009). Supervised methods rely on group labels to “supervise” or oversee the partitioning of data into known subgroups, utilizing the differences in the data between the known subgroups to best distinguish between them. Unsupervised methods, on the other hand, use only the structure of the data itself to determine the presence and the number of subgroups .
Included in the class of supervised methods are the classification methods familiar from most introductory statistics courses, such as logistic regression (Agresti 1990; Dobson 2001) and Fisher’s linear discriminant analysis (Fisher 1936; Rao 1973), as well as other, more advanced statistical methods like Classification and Regression Trees (CART; Breiman et al. 1984) and Support Vector Machines (SVM; Vapnik 1996, Wahba et al. 2000). Common to all these methods (when used for classification, i.e., CART and SVM are much more general) is the fact that the diagnostic category is the dependent variable in the statistical model, which is being predicted or described by the set of independent/predictor variables. As such, these methods are ill suited to address the question of whether a set of diagnostic categories makes sense; they take the categories as given, and two models that use two different sets of dependent categories are not directly comparable. However, they are very useful in understanding the patterns in symptom presentation between different diagnostic categories or subgroups, and in identifying which features distinguish between known subgroups and which do not .
On the other hand, unsupervised methods are directly applicable to the problem of subgroup identification and assessment. The construction of subtypes from a set of features is the purpose for which these methods were designed, and they provide a set of subgroups (or multiple sets, for some methods) that can be assessed for internal cohesion and external dissimilarity, or compared to a set of known subtypes. We describe here three commonly used clustering methods: k-means, mixture modeling, and hierarchical clustering.
k-Means
k-means (Lloyd 1957, MacQueen 1967, Hartigan and Wong 1979) is a popular clustering algorithm that assigns observations to clusters based on how close they are to cluster centers. The algorithm computes distances from each observation to each of k cluster centers using Euclidean distance. k-means then assigns each observation to its closest center, recalculates the k cluster means (hence the name), and repeats this back-and-forth process until the cluster assignments do not change anymore and the algorithm has “converged.” An example of a set of clusters obtained via k-means with two variables, 100 data points, and k = 3 clusters is shown in Fig. 2.1 .
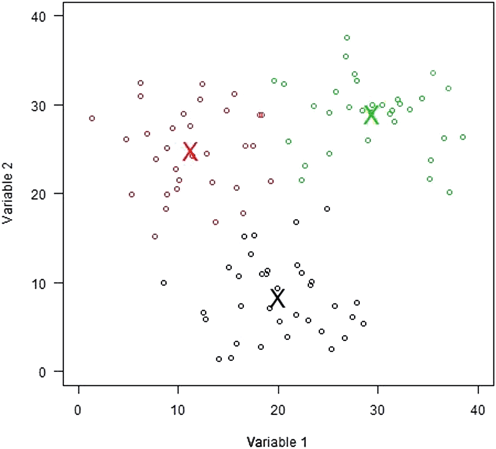
Fig. 2.1
k-means results using k = 3 on a sample data set with 100 observations, with the clusters differentiated by color. The X’s indicate the centers of each cluster. (Reprinted from D. Campbell, Statistical Approaches to Subtyping appearing in Encyclopedia of Autism Spectrum Disorders, (F. Volkmar, editor), Fig. 2.1. With Kind permission of Springer Science + Business Media)
The k-means algorithm is computationally very fast, and the clusters it provides tend to be roughly comparable in size and shape. Because it uses Euclidean distance, it works best when all measurements are on the same scale, or else the variable with the largest range will dominate the distances between points; the data can, and usually should, be scaled to adjust for this. A significant limitation is that k-means is not designed to handle categorical measurements, because the distance between categorical labels, such as “Yes” and “No,” is undefined. Like most clustering methods, k-means requires specification of the number of clusters, and making the wrong choice can yield poor clustering results.
Mixture Modeling
A broad set of clustering methods are collectively known as mixture models (Everitt and Hand 1981; McLachlan and Basford 1988). In these methods, a number of “latent” or unknown subgroups are assumed, and a probability distribution for each subgroup is specified in a statistical model. For example, the observations from each cluster may come from a multivariate Gaussian distribution, with unknown mean and standard deviation. Then, for each observation, the model calculates the probabilities of each observation belonging to each cluster. The discretized cluster assignment for each observation is determined by the cluster with the largest probability.
Mixture models have several advantages. First, unlike in k-means where clusters tend to be compact and circular in shape, the latent clusters found in a mixture model can take any arbitrary shape by defining the model appropriately. Second, there is a measure of uncertainty in cluster assignments, so an observation on the border between two cluster regions can have nonzero probability of belonging to either cluster. k-means, on the other hand, creates absolute cluster assignments—an observation either belongs to a given cluster or it does not, and each observation can only belong to one cluster at a time. The controlled uncertainty allowed in mixture modeling can be very helpful in understanding how well the clusters describe the data, and in identifying outliers that do not easily fit into any cluster .
Like the k-means algorithm, mixture models require you to specify the number of clusters. In addition, they also require that the distributions of each cluster be specified as well, which can yield a poor fit to the data if they are chosen incorrectly. While this can make the use of mixture models somewhat more complicated, the added complexity also allows clustering of more interesting and complex types of data, such as longitudinal data. By placing certain covariance, mixture modeling can find clusters in sets of curves; popular variants of mixture modeling that do this are latent growth curve analysis (Meredith and Tisak 1990; Muthen 1989; Willett and Sayer 1994) and latent trajectory analysis (Jones et al. 2001; Nagin and Tremblay 2001), and these methods have immediate applications for identifying subtypes in patterns of development of childhood with ASD.
Hierarchical Clustering
Methods like k-means build their clusters by calculating the distance of each cluster to some cluster center. The cluster centers are not observations themselves, but rather arbitrary points. Alternatively, one could build clusters by grouping together observations that are close to each other but distant from others. This is the strategy employed by hierarchical clustering (Everitt 1974; Hartigan 1975) .
The hierarchical clustering algorithm takes the set of all pairwise distances—distances from every observation to every other observation—and merges observations together into sets based on their proximity. The two closest observations get merged first, followed by the next two, and so on. The algorithm also needs one other piece of information, called the linkage criterion, which defines the distance from a set to other observations (or to other sets) given the pairwise distances. Using single linkage (McQuitty 1957; Sibson 1973), the distance from an observation to a set is the minimum distance to any of the members of the set, so two sets are close if they have a “single link” making them close. Complete linkage (Sorensen 1948; Everitt et al. 2001) instead uses the maximum distance to any member of the set, and two sets will be close under this criterion only if every pair of observations is close to each other. Average linkage (Sokal and Michener 1958; Murtagh 1984) attempts a compromise between single and complete linkage, and averages the minimum and the maximum distances. Ward’s method (Ward 1963, Székely and Rizzo 2005) takes a different approach, and combines the observations/sets together that give the smallest increase in variability by merging, so that each new cluster has the smallest possible variance.
Hierarchical clustering combines sets until all observations have been merged into a single, all-encompassing cluster. The end result is a cluster tree or cluster dendrogram made up of nested sets of clusters, where a pair of clusters is merged to give the partition with one fewer cluster. As such, it provides the cluster solution for all values of k simultaneously, although it is still up to the researcher to determine which value of k to select. Examples of cluster trees using four different linkage criteria are shown in Fig. 2.2.
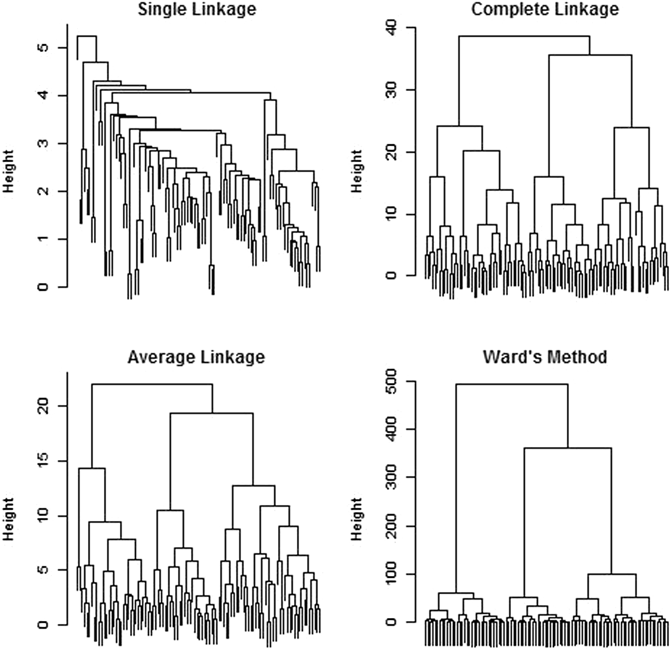
Fig. 2.2
The results of hierarchical clustering using four different linkage criteria on the same dataset used in Fig. 2.1. Notice that single linkage tends to produce many tiny clusters, while average linkage and Ward’s method favor larger groups. While the overall tree structures are markedly different, on this particular dataset, complete linkage, average linkage, and Ward’s method give nearly the same subgroupings if the tree is cut into three clusters. This is because the data strongly displays a three-cluster structure, as seen in Fig. 2.1. (Reprinted from D. Campbell, Statistical Approaches to Subtyping appearing in Encyclopedia of Autism Spectrum Disorders, (F. Volkmar, editor), Fig. 2.2.
With Kind permission of Springer Science + Business Media)
Determining the “correct” number of clusters—and by extension, determining if multiple clusters are preferable to a single continuum—is one of the most difficult aspects of clustering. Because there is no known “true” grouping in the data (if there were, clustering analysis would not be necessary), there is no way to know the number of clusters with certainty, and instead alternative means of estimating the number of clusters and assessing the validity of a set of clusters must be used. Such methods can be internal, by looking at the statistical differences between clusters on the same variables used to make the clusters, or external, by relying on other variables not included in the clustering analysis to validate the clusters .
In practice, choosing the number of subgroups using internal aspects of the cluster solution is commonly done by means of a variance plot, sometimes called a scree plot (Fig. 2.3). This plot graphs the number of clusters, k, on the x-axis, and a measure of variability or dispersion of the data on the y-axis, typically the within sum of squares summed over the k clusters. When k = 1, the sum of squares gives the total amount of variability in the data; for larger values of k, the sum of squares will be smaller because the clusters explain some of this variability. The plot shows how much explanatory power (measured by a drop in variability) is gained by each additional cluster, with big drops in variability for the first few clusters, but much smaller drops as more and more clusters are added. Such a plot can suggest the best choice of k if it displays a “kink” or “elbow,” where the marginal benefit of adding more clusters is relatively small and a more parsimonious clustering is preferable. Variants of this technique use different statistics for cluster dissimilarity than the sum of squares, like the Gap statistic (Tibshirani et al. 2001). A wide selection of methods for choosing the number of clusters in a dataset is compared in Milligan and Cooper (1985) .
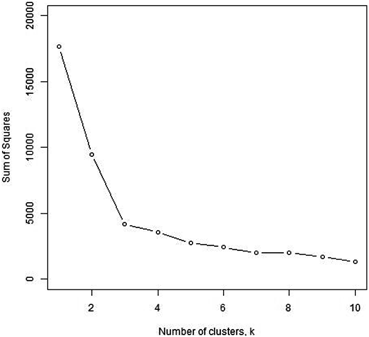
Fig. 2.3
A scree plot showing the decreasing in sum of squares with increasing k, using k-means on the same data as in Fig. 2.1. Notice the kink at k = 3, suggesting three subgroups in the data. (Reprinted from D. Campbell, Statistical Approaches to Subtyping appearing in Encyclopedia of Autism Spectrum Disorders, (F. Volkmar, editor), Fig. 2.3.
With Kind permission of Springer Science + Business Media)
External information can also be useful in choosing the preferred number of clusters, as well as in assessing the meaning and interpretation of a given set of clusters once the number has been decided. A set of clusters can be validated though hypothesis testing, e.g., comparing differences in means between clusters using Student’s t-test (for two clusters) or an F-test (for three or more clusters). Statistically significant differences in mean between some or all of the clusters on variables not used in the clustering algorithm can indicate clinically relevant differences between the subgroups. If some clusters do not exhibit statistically significant differences from each other, then they should perhaps be merged together to yield a more parsimonious subgroup structure. Care should be taken not to read too much into differences among the variables used to create the clusters; however, because the clustering procedure is designed to maximize differences on these variables, they cannot serve as outside sources of validation.
Another consideration is that a statistically significant difference in mean between groups does not necessarily imply that the data structure is better described by two clusters than by a continuous spectrum. In the extreme case of scores obtained by a single Gaussian distribution, with no evident bimodality in scores, a t-test comparing the means of scores above the average to those below will almost certainly reject the null hypothesis of equal mean. The fact that the means of the upper and lower halves of a distribution are unequal is not proof of bimodality in the distribution of scores, as this will likely be true regardless of the existence or nonexistence of cluster structure in the distribution. Thus, an accurate representation of the number of subgroups in a diagnostic category depends as much on the structural coherence and separation of subgroups as on the clinical differences between them .
Current Classification Systems and Issues of Autism Spectrum Disorders
The Pervasive Developmental Disorders of DSM-IV and ICD-10
Five distinct disorders were included under the category of pervasive developmental disorders in the DSM-IV (APA 1994), all of which have corresponding disorders defined in ICD-10 (WHO 1992). Except for a few minor differences, mainly in PDD-NOS/atypical autism, DSM-IV and ICD-10 have identical diagnostic criteria—this represented the first time that the American and international criteria were matched. The diagnostic criteria for each disorder were based on the best available clinical evidence, which, as stated previously, was based on the results of an extensive field trial (see Volkmar et al. 1994). The current diagnostic criteria for the five pervasive developmental disorders from ICD-10 are provided in Appendixes A–E. This section of the chapter provides an overview of these five disorders.
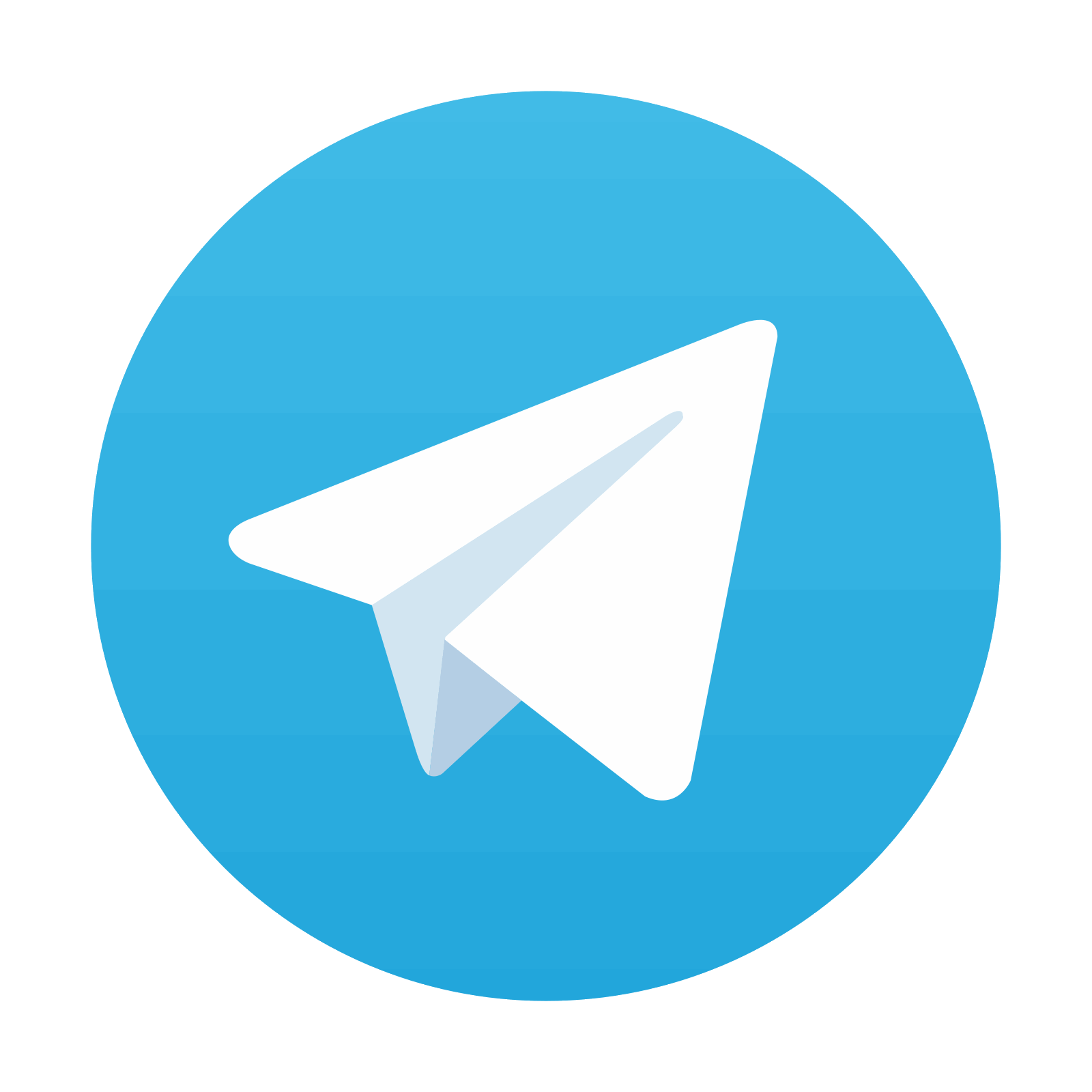
Stay updated, free articles. Join our Telegram channel

Full access? Get Clinical Tree
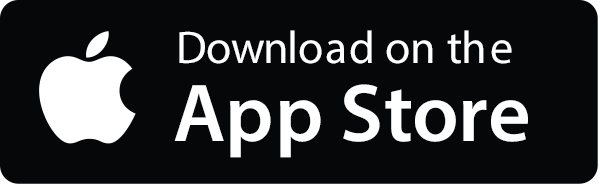
