A basic familiarity with statistical terms and research methodology is helpful when critically assessing literature for practical evidence-based clinical application. Statistics is the study of the collection, analysis, interpretation, presentation, and organization of data. Statistical analysis helps determine the likelihood that an observed result occurred by chance alone or with a relationship to a study variable.
Key terms and concepts
Hypothesis
- •
An assumption or proposed explanation, a belief
- •
States the expected relationship between variables
- •
Is testable: can be rejected or not rejected
Null hypothesis (H 0 )
- •
Assumed to be true unless there is evidence to the contrary
- •
States there is no difference between groups being tested
- •
If the researcher is interested in whether there exists a relationship exists between two groups or a difference between two treatments, then the null hypothesis is: “There is no relationship between the two groups” or “No difference exists between the two treatments or variables under study.”
- •
Researchers’ bias is to try to disprove the null hypothesis
Alternative (research) hypothesis (H A )
- •
There is a relationship or difference between the variables under study.
- •
Often represents what the researcher is trying to prove
Hypothesis testing
- •
State the hypothesis.
- •
Choose a statistical significance level denoted as alpha (α).
- •
Probability of type I error ( Table 61.1 )
TABLE 61.1
Hypothesis Testing Decisions: H 0 = Null Hypothesis
Decision
H 0 is true
H 0 is false
Reject H 0
Type I error = α
Correct decision
Fail to Reject H 0
Correct decision
Type II error = β (1-power)
- •
The threshold value that p-values are measured against
- •
By convention, α is set between 0.01 and 0.10, usually set at 0.05.
- •
- •
Determine the sample size, the total number of subjects involved in the study, needed to determine statistical significance.
- •
Test the hypothesis by applying a statistical test to the collected data to determine how likely the observed results are because of chance.
- •
A statistical test will generate a calculated p-value
- •
P = probability, the probability the observed data occurred by chance
- •
P = 0.5 is a 50% probability the observed data occurred by chance
- •
P = 0.05 is a 5% probability the observed data occurred by chance
- •
Small p-values (0.05, 0.01, 0.001) suggest that the null hypothesis is unlikely or very unlikely to be true
- •
- •
Statistical significance: compare obtained p-value from statistical test to the significance level (alpha)
- •
If p-value is less than alpha → reject null hypothesis, the result is statistically significant; observed data is unlikely to occur by chance
- •
If p-value is greater than alpha → accept the null hypothesis; the result is not statistically significant; observed data are likely to occur by chance
Hypothesis testing errors ( table 61.1 )
Type I error = alpha (α)
- •
A true null hypothesis is rejected.
- •
Considered more serious than type II errors
- •
If α = 0.05, then the null hypothesis is rejected if the sample would only happen 5% of the time if the null hypothesis is true. Therefore, there is a probability of 0.05 that the null hypothesis will be rejected when in fact it is true.
Type II error = beta (β) = (1-power)
- •
A false null hypothesis is not rejected
- •
If the null hypothesis is not rejected, it may still be false, because the sample may not be big enough to identify the false null hypothesis.
Statistical power
- •
The ability to reject a false null hypothesis
- •
1- β, where β = risk of type II error defined earlier
- •
The higher the power, the greater probability of producing statistically significant results.
- •
Statistical power is increased by:
- •
Larger sample size
- •
Larger effect size
- •
Larger alpha level
- •
Test direction (e.g., one-tailed t-test)
- •
Using parametric statistics
- •
Decreasing error
- •
- •
For instance, if alpha is changed from 0.05 to 0.01, the power of the study will decrease, because alpha is changed to a smaller number.
Types of data or scales of measurement
Categorical
- •
Nominal data: nonordered category for classification (e.g., sex, ethnicity, city, political party)
- •
Ordinal data: ordered category, allows one to rank in terms of less or more (e.g., Likert scales, percentile ranks)
Continuous
- •
Interval data: score or numerical data with equal intervals with no absolute zero score (e.g., temperature in degrees Fahrenheit and degrees Celsius)
- •
Ratio data: interval data with absolute zero value (e.g., weight, height, money in bank account, temperature in Kelvins)
Variables
- •
Things that are measured, controlled, or manipulated in research
- •
Dependent variable (DV)
- •
The variable that depends on another variable (independent variable)
- •
Variable that is measured or registered (e.g., pain, body mass index [BMI], Glasgow Coma Scale [GCS])
- •
Usually the outcome variable
- •
- •
Independent variable (IV)
- •
The variable that is believed to affect the status of another variable (DV)
- •
Variable that is manipulated (e.g., therapy type, drug dose)
- •
Usually considered the intervention or treatment
- •
- •
To determine which variable is the DV and IV, one can use this phrase: What is the effect of the IV on the DV?
Descriptive statistics
- •
Used to describe a sample or population characteristics (e.g., diagnoses, height, weight, body mass index, temperature, pain scores, treatment outcomes)
Data distribution ( fig. 61.1 )
When a large enough number of samples are collected or observations made, the data for many variables will follow a normal distribution (a symmetrical, bell-shaped curve with values and data points equally distributed above and below the mean). Data that are not normally distributed are called skewed or kurtotic . In a positive skew, there is a larger proportion of data points with lower values. In a negative skew, there is a larger proportion of data points of higher values.
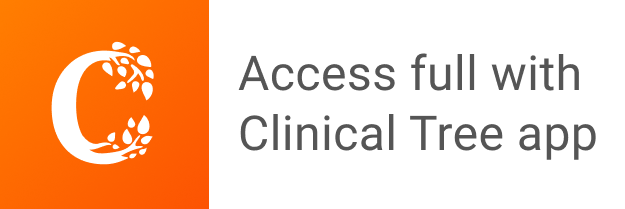