Summary of Key Points
- •
Big data analytics refers to a collection of tools that will analyze large volumes of data and allow for predictions based on those analytics to convert data into actionable change.
- •
Health care is at a tipping point with regard to big data implementation. Key factors fueling the increasing adoption of this technology include increasing cost consciousness and emphasis on outcomes in health care, changing payer reimbursement patterns from the traditional fee-for-service system to risk-sharing models that place additional financial incentive on cost consciousness and demonstration of outcomes, and the increasing liquidity of data.
- •
Big data analytics has the potential to save the health care system an average of $300 to $450 billion through its application in areas such as research, cost optimization, and development of evidence-based paradigms.
- •
The medical industry has been slower than other industries to adopt big data. This is largely due to a concern for patient privacy and the high costs associated with adding analytics capabilities to existing Electronic Health Records (EHR).
- •
The use of big data in health care is currently in its infancy. Successful adoption and growth of this technology relies on a paradigm shift in mindset regarding health information from “protect” to “share with protection.”
Data of all types are accumulating at an astounding rate. It is estimated that 90% of the world’s data have been created since 2013. The increasing expansion of data has affected all industries including health care.
Health care has undergone significant changes that have exponentially increased the amount of data being stored. Electronic medical records (EMRs) have seen broad adoption since the early 2000s. Estimates in 2011 suggested that 50% of physicians and 75% of hospitals in the United States have adopted an EMR, and the number continues to increase. This transition from paper charts has led to an increasing supply of digital information. However, this seemingly endless supply of data has limited utility on its own. “Big data” analytics has become a buzzword for a set of tools that enable users to work with large volumes of data and convert it into actionable change.
What is Big Data Analytics?
Big data analytics is everywhere and has affected your day-to-day life whether you are aware of it or not. Over 90% of Fortune 2000 companies use big data analytics in one form or another. Netflix uses it to recommend your favorite movies, iTunes and Spotify to recommend music, credit bureaus to estimate your credit score, large stores to track customer spending, and supermarkets to correlate item location with spending. UPS uses big data analytics to predict truck maintenance schedules. Each of these is an example of how businesses have gathered large volumes of data and extracted the value hidden within that data.
The exact definition of big data analytics is difficult to pin down, but in general the term refers to analyzing large volumes of data in order to reveal patterns, trends, and associations within the data set. People, sensors, and machines are producing unprecedented quantities of data. The important aspect of big data is not the sheer volume of information, but the valuable signal hidden within the noise of that data that can be used to build predictive models. The goal of big data is often simple: to help you understand your target population better. Whether looking at the behaviors of shoppers, movie watchers, or patients, big data analytics offers the potential to better understand those populations.
Role of Big Data in Health Care
Although big data analytics has a proven track record of success in multiple industries, a common question is “What does big data offer analytics to health care?” Five areas of potential benefit have been described in terms of evidence-based medicine, research, communication with patients, defining value, and cost optimization.
Evidence-Based Medicine
The term evidence-based medicine (EBM) was coined by David Sackett in the 1980s. Sackett was a strong advocate for careful evaluation of patient-centered outcomes data in the assessment of diagnostic and therapeutic modalities. Careful scrutiny of effectiveness was mandated prior to recommending the use of an intervention. Sackett defined EBM as “conscientious, explicit, and judicious use of current best evidence in making decisions about the care of individual patients.” In the practice of EBM, Sackett emphasized the importance of integrating individual clinical expertise and patients’ choice with the best external evidence.
The best available evidence is often presented as evidence-based guidelines compiled by specialty associations such as the American Academy of Orthopaedic Surgeons (AAOS) or the North American Spine Society (NASS). Guidelines are useful for providing a general overview of the current state of literature and incorporating expert opinion on specific clinical questions. Guidelines can often provide treatment algorithms for common conditions. The application of standardized treatment paradigms results in more homogenous treatment practices that lend themselves to comparison with alternative treatments. Without an organized, homogenous cohort, analysis of outcomes of a given treatment or diagnostic modality become more difficult. The guidelines process must also follow several tenets as presented by the Institute of Medicine including transparency, appropriate panel composition, conflict of interest management, and external review. In the pursuit of optimized evidence-based treatment protocols, it is important to remember David Sackett’s original message: EBM is not “cookbook” medicine. Best available evidence must be combined with individual clinical expertise and patients’ wishes.
Evidence-based medicine has become a mantra of modern day practice. An important aspect to consider in the implementation of EBM is defining best evidence . The strength of evidence varies depending on the type of data used. The Centre for Evidence-Based Medicine developed a grading system for levels of evidence, ranging from level I evidence, the highest quality, involving randomized clinical trials (RCTs), to level V evidence, the lowest quality, involving expert opinion. In the middle are lesser quality RCTs and prospective cohort studies (level II), observational data obtained from case-control or retrospective comparative studies (level III), and case series (level IV).
The highest quality evidence comes from level I RCTs. This type of data is inherently problematic to procure in surgical specialties. Strict randomization of patients to surgical versus nonoperative treatment can pose a significant ethical dilemma. If a patient has been randomized to the nonoperative treatment arm, but has attempted nonoperative treatment in the past and now demands surgery, is it ethical to withhold that treatment? The Spine Patient Outcomes Research Trial (SPORT) was a $15 million RCT of surgical versus nonoperative interventions; although commonly considered the best-designed RCT in spine literature, it was still plagued by the issue of patient crossover. In addition to problems with design and randomization, RCTs are logistically difficult and expensive. The logistical difficulties of RCTs are compounded if the topic of interest is an event of low prevalence, such as rare surgical complications, and requires a large sample.
As a result of the previously described problems with RCTs in orthopaedic surgery, the majority of evidence for current practice comes in the form of nonrandomized observational studies. Although these data are considered inferior to RCTs in quality, observational studies have significant potential to generate hypotheses, identify areas of uncertainty within a treatment algorithm, and provide evidence to answer certain clinical questions. It is by providing an unprecedented amount of observational data and sophisticated analytics necessary to transform that data into actionable change that big data analytics has the potential to revolutionize surgical care.
Big Data and Research
The traditional approach to clinical research is “frequentist” in nature, involving the study of a relatively small sample of the population and making inferences based on that sample. Great care is taken to ensure the high quality of data in this sample and the elimination of as many confounding variables as possible. Typically this results in a low volume of very high quality data. In general, high levels of accuracy can be obtained using this method. This approach becomes problematic as the necessary sample size increases in order to yield the statistical power necessary to study rare outcomes such as certain postoperative complications. As the study size increases, logistical difficulty and cost increase substantially and quickly become prohibitive.
Big data analytics offers a potential solution to this dilemma. The big data approach uses large volumes of data that are lower in quality than that collected as part of an RCT. As the data sample increases in volume and approaches 100% of the population, the predictive power of that sample can be greater than that of very high quality data composing a 1% sample. The volume of the data allows for the use of sophisticated analytic techniques that use complex algorithms to make predictions. In this regard, big data analytics poses a contrast to traditional single hypothesis-driven research. Instead of rigorously testing a single hypothesis, big data analytics uses complex algorithms to generate a series of hypotheses or associations.
The role of both the traditional and big data approaches in the future of clinical research must be clearly defined. One approach does not replace the other. In fact, the two are complementary. Big data analytics uses large volumes of data to identify associations and generate novel hypotheses. As the analytic techniques associated with big data become more sophisticated, the associations hidden within the noise of data become more evident. Big data analytics is not intended to replace traditional prospective research. However, it can provide areas of particular interest that lend themselves to further research with the traditional approach.
Value
The United States spends approximately $9000 per capita on health care. This is roughly twice that of other industrialized nations. The financial climate has brought a new national focus on value in medicine. Value is defined as outcomes per unit cost. Quantifying value in health care has been persistently difficult. Two central challenges to demonstrating value are tracking outcomes measures and measuring cost. Big data can play a role in surmounting both obstacles.
Defining the costs associated with delivery of care has been extraordinarily difficult due to the complex nature of medical billing. A study by Porter and Lee stated that there is “near complete absence of data on the true costs of care for a patient with a particular condition over the full care cycle, crippling efforts to improve value.” A novel approach to quantifying the cost of delivering care has been developed by the University of Utah, termed the Value Driven Outcomes (VDO) initiative.
This system involves combining patient clinical data with hospital financial information to estimate the total cost of each individual encounter. The VDO approach takes all general ledger costs of the health care system and assigns a subsection of those costs based on the amount of use of each resource that each patient encounter utilized. For example, the total costs of running a surgical intensive care unit (based on personnel, facility costs, etc.) would be allocated to encounters for patients that stayed in the unit based on the amount of time spent in that unit. In addition to these estimated systemic costs, the discrete costs of resources used by the patient such as medications and durable medical equipment are assigned to the specific patient encounter. Alternatively, Kaplan of Harvard Business School has championed time-derived activity-based costing to assign costs for each encounter, based on the time and cost of each person that a patient encounters during the care process.
These sophisticated methods of quantifying costs of health care delivery are only possible through significant investment in health care information technology (HIT) platforms and electronic medical records (EMRs). Although these systems pose a significant initial investment, they are imperative for defining costs and demonstrating value in the practice of orthopedics.
Communicating with Patients
One of the most exciting applications of big data is in helping physicians communicate effectively with patients. Big data in the form of observational outcomes data on large numbers of patients can make it possible to quantify the risks and benefits of interventions, as well as to inform patients about their symptoms compared to thousands of patients just like them. Eventually it may be possible to unify multiple data sources across formats and platforms in order to create a longitudinal patient record that spans across inpatient, outpatient, and ambulatory settings. That data could be combined with analytics to communicate insightful patterns within the data and thus improve quality, decrease cost, and advance research.
The important aspect of this application is in how patients and physicians interact with the data. Although an ocean of outcomes data can help a patient make an informed decision, it can also confuse both of the parties involved. For data to be useful, the patient and physician must first be able to make sense of the numbers. Studies have shown that people on average have poor comprehension of simple probabilistic information.
Poor comprehension has been identified when patients or physicians were presented with more than three pieces of information. The manner in which the physician and patient interact with the data will determine whether the data will aid or hinder communication. A sophisticated user interface will be a key component of making big data usable in patient-physician interactions. If such an interface is able to display data relevant to the specific patient in the form of graphs and figures, this will be paramount to helping patients make informed decisions regarding their care.
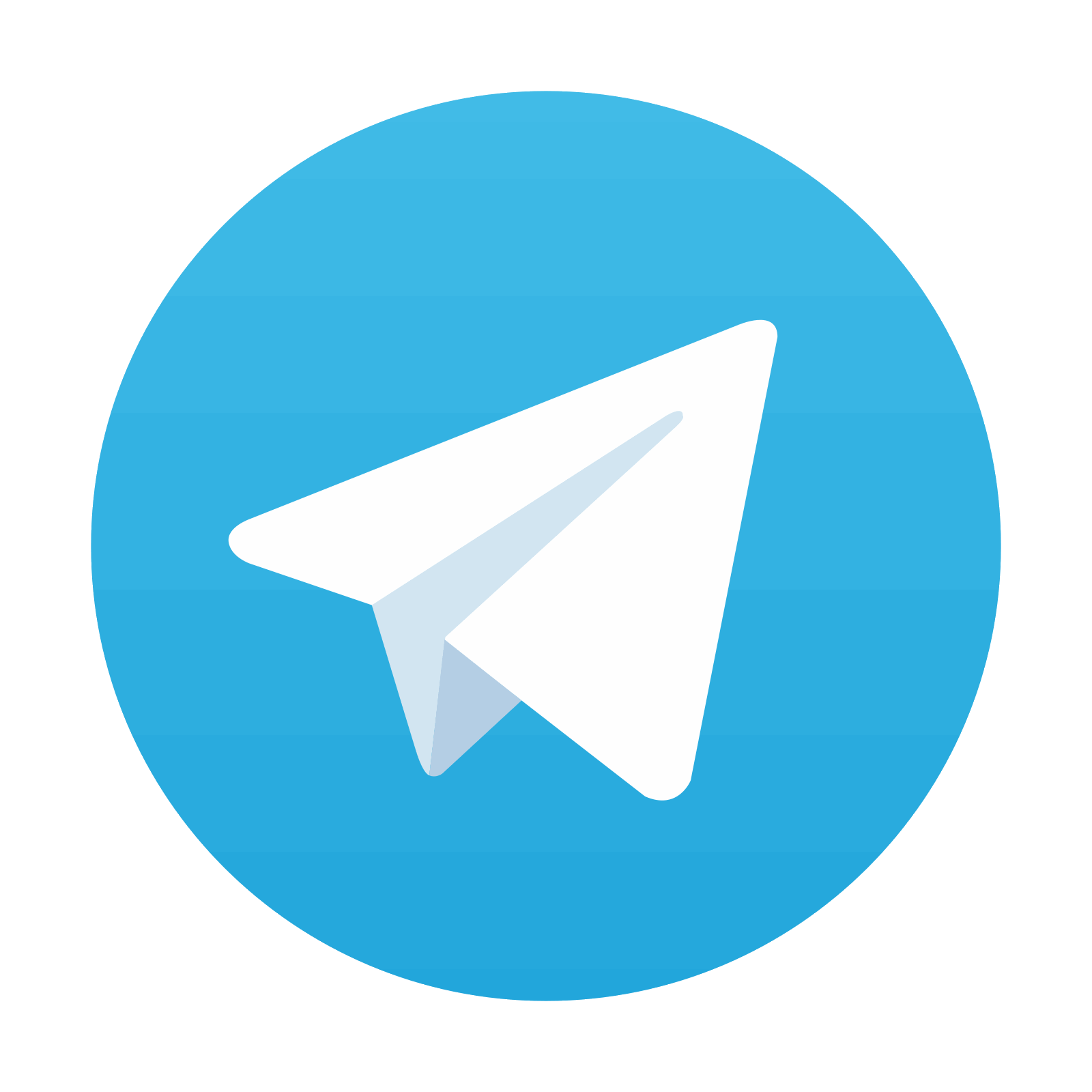
Stay updated, free articles. Join our Telegram channel

Full access? Get Clinical Tree
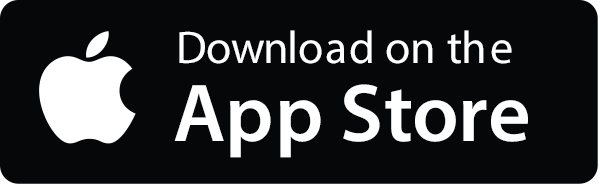
