Fig. 11.1
A typical brain-computer interface schema. Modifications of brain activity due to a task/stimulus are recorded with fMRI, fNIRS, EEG, or ECoG. These neural data are pre-processed before discriminative features are extracted. Machine learning techniques are then used to train classifiers to detect statistical patterns in the features that are reliably associated with prespecified (supervised) volitional states of the user. The trained classifier is then used to classify new features corresponding to states now selected by the user to communicate choices. Finally, the result of the classification is fed back to the user to help him/her train themselves in the use of the BCI and to control external devices (e.g., word spelling, control of a wheelchair, a robotic prosthesis) or to help clinicians detect a response to command or functional communication
In the context of DOC, the first goal of a BCI is to detect command-specific changes in brain signals as evidence of conscious thoughts. Then, if the patient is able to reproducibly follow a command using the system, the software and hardware can be extended to test communication. The acquisition of voluntary responses such as command-following and functional communication is keystones in diagnosis as defined by behavioral criteria (Giacino et al. 2002; Plum and Posner 1966; Laureys et al. 2010). The presence of command-following indicates emergence from the vegetative/unresponsive wakefulness state (VS/UWS; (Laureys et al. 2010)) or recovery of a minimally conscious state (MCS; (Giacino et al. 2002; Bruno et al. 2012)). The recovery of functional communication indicates the emergence from MCS (EMCS; (Giacino et al. 2002)). Command-following and functional communication also distinguish locked-in syndrome (LIS) (Plum and Posner 1966) from VS/UWS patients. Because a better outcome for MCS versus VS/UWS patients has been reported (Luauté et al. 2010), patient access to rehabilitation is likely to be influenced by the clinical diagnosis. In addition, a recent study of 108 patients with traumatic brain injury reported that 56–85 % of patients showing command-following before discharge from the acute inpatient rehabilitation were functioning independently by 5 years post-injury, as compared to 19–36 % of patients who did not show command-following at discharge (Whyte et al. 2013). It remains unclear whether it was the presence of command-following, the quality of rehabilitation treatment (e.g., duration, hours of therapy, etc.), or both that contributed to better outcomes for command-followers. For LIS patients, the difficulty to recognize unambiguous signs of consciousness in the acute stage often results in the diagnosis being delayed or even missed (Laureys et al. 2005), with potentially catastrophical implications. Conversely, an early diagnosis allows clinicians to start developing a communication tool tailored to the patients’ residual abilities.
To benefit from a BCI, the patient would need to first understand the task and repeat it several times, then to be able to attend to stimuli/questions while retaining task information in working memory. However, current BCIs require that the patient have much more capacity than required by behavioral testing, leading to undetectable command-following in many patients. When looking at the results obtained in studies of patients with DOC, we therefore need to take into account the number of patients showing command-following with the system, and how many of them were able to follow a command at bedside that could not be detected by the system (i.e., false negatives; see Table 11.1). False-positive rate should also be considered, but will not be discussed here as it is difficult to determine the level of consciousness of patients diagnosed unconscious but showing response to command with a BCI.
Table 11.1
Studies using brain-computer interfaces (BCIs) and alternative systems in patients with disorders of consciousness for assessing response to command and communication with false-negative ratios (patients showing command-following at the bedside, but not detected by the BCI)
References | Technique used – brain response | Task | Total number ofpatients included | False-negativeratio (%) |
---|---|---|---|---|
BCI applications | ||||
fMRI – motor imagery | Playing tennis vs. walking through your house (command-following and communication) | 55 (24 VS/UWS; 31 MCS) | 17/18 (94 %) | |
Bardin et al. (2011) | fMRI – motor imagery | Swimming (command-following and communication) | 6 (3 MCS; 2 exit MCS; 1 LIS) | 2/5 (40 %) |
Monti et al. (2009) | fMRI – P3 | Count a target word – neutral (command-following) | 1 (MCS) | 0/1 (0 %)a |
Naci and Owen (2013) | fMRI – P3 | Count a target word – neutral (command-following and communication) | 3 (1 VS/UWS; 2 MCS) | 0/1 (0 %)a |
EEG – P3 | Count a target word – subject’s own name (command-following) | 23 (8 VS/UWS; 14 MCS; 1 LIS) | 2/8 (25 %) | |
Lulé et al. (2013) | EEG – P3 | Count a target word (communication) | 18 (3 VS/UWS; 13 MCS; 2 LIS) | 5/6 (83 %) |
Goldfine et al. (2011b) | EEG – motor imagery | Swimming vs. walking through your house (command-following) | 3 (1 MCS, 1MCS/exit MCS, 1 LIS) | 1/3 (33 %) |
EEG – motor imagery | Squeeze your right hand vs. move your toes (command-following) | 39 (16 VS/UWS; 23 MCS) | 13/15 (87 %) | |
Cruse et al. (2012b) | EEG – motor imagery | Squeeze your right vs. left hand (command-following) | 1 (VS/UWS) | Not applicable |
Pokorny et al. (2013) | EEG – P3 | Count the number of deviant tones (command-following and communication) | 12 (1 VS/UWS, 10 MCS, 1 exit MCSb) | 1/3 (33 %)b |
Chennu et al. (2013) | Count the number of target word (command-following) | 21 (9 VS/UWS; 12 MCS) | EEG: 7/7 P3b (100 %), 5/7 P3a (71 %); fMRI: 3/7 (43 %) | |
Alternative systems | ||||
Bekinschtein et al. (2008) | EMG – muscle activity | Move your right hand (command-following) | 10 (8 VS/UWS, 2 MCS) | 0/1 (0 %)a |
Stoll et al. (2013) | Infrared camera – pupil dilation | Perform arithmetic problem (communication but command-following with the MCS patient) | 13 (12 LIS; 1 MCS) | 0/1 (0 %)a when used for command-following, but 9/12 (69 %) when used for communication |
11.3 Absence of Motor Responses and Brain-Computer Interfaces
The first study showing the possibility of detecting response to command with BCI was conducted by Owen et al. in 2006 and reported that one patient diagnosed as being in VS/UWS was able to follow the instruction to “imagine playing tennis” and “walking through her house” during an fMRI session (Owen et al. 2006). The paradigm consisted of several sessions of mental imagery followed by a resting period, both lasting 30 s. This patient displayed similar brain activation as compared to healthy volunteers for both tasks. In addition, the patient behaviorally evolved into MCS a few months after the study. In a follow-up study (Monti et al. 2010) including 54 patients (23 VS/UWS and 31 MCS), five (four VS/UWS) showed ability to willfully modulate their brain activity according to the task. One of them was also able to answer simple questions, e.g., “Is your father’s name Alexander?” using one task for “yes” and the other for “no.” However, out of 18 patients showing command-following at the bedside, only one could be identified with the system (false-negative rate: 94 %). Bardin et al. (Bardin et al. 2011) investigated the use of a different imagery task instructing patients to imagine themselves swimming or playing tennis with their right hand, using a similar protocol to the one used by Owen et al. (2006) and Monti et al. (2010). Out of six patients (three MCS, two MCS/emerging MCS, one LIS), three were able to follow commands with the system (one MCS, one MCS/emerging MCS, one LIS). However, of the five patients who were able to follow commands at the bedside, two of them could not be identified with the system (false-negative rate: 40 %). Similarly, using an active task in fMRI task (counting a target-neutral monosyllabic word in an auditory sequence of nontargets words), Monti et al. reported preserved working memory abilities in a MCS patient exceeding that which could be observed with standard behavioral assessment (Monti et al. 2009). This patient was able to follow a command and communicate non-functionally at the bedside. Finally, three patients (one VS/UWS, two MCS) were instructed to either count the occurrences of a target word (“yes” or “no”) or to simply relax and passively listening to a sequence of “yes” and “no” presented in a random series of numbers (Naci and Owen 2013). Command-following could be detected in all of the patients, and two patients (one VS/UWS and one MCS) were able to focus their attention to communicate correct answers to two different binary (“yes” or “no”) questions such as “are you in a supermarket?” or “is your name Steven?” Because the latter two studies included few patients, and the results have not been replicated yet, interpretation of false-negative rates has not been conducted.
These first BCI studies showing response to command in DOC patients were conducted using fMRI, a technique that has many limitations preventing it from being applied universally to the DOC population. First, ferrous metallic implants are a contraindication to MRI, preventing many patients from undergoing this procedure. Even if implants are nonferrous, metal in the head can cause significant image artifact, making the analysis of the results difficult or impossible. Second, fMRI is sensitive to motion, which can result from reflexive movement in the scanner, general restlessness, or decreased patient cooperation. Images that are affected by significant motion artifact cannot be interpreted. Specifically, over three years of the European FP7 project DECODER during which the fMRI active sport/navigation paradigm described above was used in clinical settings at the Centre Hospitalier Universitaire de Liège, 169 patients were elected for the fMRI procedure. From this cohort, only 60 studies yielded active paradigm data that were interpretable, outlining the difficulties in generalizing this approach. The main reason for data rejection was artifact caused by head motion in the scanner. Finally, many clinical settings do not have access to MRI because it is an expensive technique to implement. Furthermore, fMRI requires executing complicated data processing methods, which necessitates involvement of personnel with expertise in this area. Given these limitations, EEG may be better suited for assessing DOC patients as it is not contraindicated by metallic implants and is less sensitive to motion. EEG is relatively inexpensive, and compact systems can be readily deployed at the bedside. In recent years researchers have been developing EEG-based BCI to assess response to command in DOC.
As suggested by fMRI studies, BCI using imagination of movement may be a reasonable supplement to observation of actual movement during standard behavioral assessment. EEG studies have shown that motor imagery is associated with a power decrease (event-related desynchronization) in the sensorimotor or mu rhythm (8–15 Hz; (Pfurtscheller et al. 1997; Neuper et al. 2005)), focused in the motor region that is implicated in the movement being imagined (Pfurtscheller and Lopes da Silva 1999). Goldfine and colleagues (2011) recorded EEG from three patients showing command-following at the bedside (MCS, MCS/emerging MCS, and LIS), while they were involved in motor imagery and spatial navigation tasks. The session alternated eight 15-second periods of mental imagery with 15-second periods of rest. All of the patients demonstrated the capacity to generate mental imagery on the same tasks on independent fMRI studies. With univariate comparisons (individual frequencies), these investigators showed evidence of significant differences between the frequency spectra accompanying the two imagery tasks in one MCS patient (however, results were not stable between the two runs) and one LIS patient (false-negative rate: 33 %).
In another study from Cruse and colleagues, motor imagery tasks were investigated in 16 VS/UWS (Cruse et al. 2011) and in 23 MCS patients (Cruse et al. 2012a). Eight (three VS/UWS, five MCS) were able to voluntarily control their brain activity in response to a command (“imagine squeezing your right hand” versus “imagine moving all your toes”). Out of 15 patients showing command-following, 13 could not be identified by the system (false-negative rate: 87 %). In order to decrease the cognitive load required to complete the task (e.g., minimize task switching and the duration of the session), the latter study used a block design with instructions to perform motor imagery following each of 15 subsequently presented tones. However, in this population block design may be problematic because changes in the EEG signal across and within blocks may be influenced by vigilance and motor artifacts leading to lack of independence between trials. For this reason, these results should be interpreted cautiously because dependence between trials was not accounted for in the statistical analyses. This issue is specifically relevant for the severely brain-injured population, and BCI studies in the future will need to take it into account (Cruse et al. 2013; Goldfine et al. 2013).
In an attempt to circumvent the statistical pitfalls of block design, an alternate paradigm has been investigated. In this paradigm, each trial is started with one of three instructions (i.e., “try to move your right hand,” “try to move your left hand,” and “and now, relax”) that are presented through sounds in a randomized order. Because the instructions are presented before each trial as oppose to at the beginning of a block, significantly less working memory capacity is required to carry out the task. In addition, this method is technically less challenging and more efficient as it requires the use of only four electrodes. The utility of this paradigm as a diagnostic tool has been reported in a single patient diagnosed as being in a VS/UWS at bedside (Cruse et al. 2012b). However, this type of protocol still requires higher-level cognitive abilities, such as sustained attention and task switching, as compared to behavioral assessment.
Several studies have suggested that motor imagery cannot be reliably used in motor-disabled patients (Kasahara et al., 2012; Fiori et al., 2013). Instead of motor imagery, Nijboer et al. has recommended the preferential use of the P3-based BCI in patients with severe motor impairment (Nijboer et al. 2010) (see also Chap. 8). The P3 response (also called P300) is a positive deflection in the EEG appearing around 200–500 ms following a target stimulus (see also Chaps. 7 and 9). The advantage of the P3 is that it can be elicited by meaningful stimuli and requires a limited working memory load from the patient. Some of the earliest EEG-based BCI systems were based on the P3 component (Farwell and Donchin 1988; Donchin et al. 2000). The successful use of P3-based BCIs by a larger population of healthy users versus the sensorimotor rhythm has also been reported by Guger et al. (Guger et al. 2009; Guger et al. 2003). Moreover, many studies have shown that this system is feasible and practical for patient groups (see, e.g., Sellers et al. 2006; for a review, Hoffmann et al. 2008; Manyakov et al. 2011) and offers a stability of the performance over time in this population (Sellers et al. 2010; Nijboer et al. 2008; Silvoni et al. 2009). Consequently, auditory P3 responses are more likely to be usable by a greater number of patients (Chatelle et al. 2012). However, some of the most successful P3-based BCI systems are based on visual P3 responses which may be difficult to elicit in brain-injured patients as they frequently present with gaze fixation impairments (Lew et al. 2009; Alvarez et al. 2012) preventing them from attending to visual stimuli. Schnakers et al. proposed using an auditory P3 for detecting command-following using EEG (Schnakers et al. 2008a). They used a paradigm instructing patients to count the number of times a name (subject’s own name or unfamiliar name) was presented within an auditory sequence of random names in 22 patients (eight VS/UWS, 14 MCS) (Schnakers et al. 2008a). Results showed that five out of 14 MCS patients showed significantly larger P3 responses when actively counting the occurrence of their own name as compared to when only passively listening to it. In addition, four other MCS patients showed a response only when they were asked to count an unfamiliar name as compared to passive listening. These results suggest that fluctuation of vigilance may play a role in task performance in this population. The eight VS/UWS patients did not show any response to the active task. The same paradigm has been used in a patient behaviorally diagnosed as being comatose, who showed a significant difference between the passive and the active task (Schnakers et al. 2009). Following this finding, this patient was reassessed and diagnosed with complete LIS. This extreme case illustrates the clinical utility of BCI as a supplement to behavioral assessment. Using this paradigm, two out of eight patients showing command-following at bedside could not be detected with the system (false-negative rate: 25 %). Similar results have been replicated in a recent study including patients with DOC (Risetti et al. 2013).
When the data were analyzed offline, one LIS patient reached 79 % accuracy. Out of six patients showing command-following at bedside (four MCS, two LIS), five could not be detected with the system (false-negative rate: 83 %). However, these results should be interpreted cautiously because the offline analysis used data from both the command-following and communication sessions to determine the presence of command-following.
A study by Lulé et al. used a four-choice auditory-based paradigm for communication with three VS/UWS, 13 MCS, and two LIS patients (Lulé et al. 2013). After a command-following training phase (four runs of counting “yes” or “no’s”), each patient was asked to communicate by answering 10 questions (counting “yes” or “no’s” depending on the answer). When using the system online, no patient could achieve performances allowing communication (>70 % accuracy (Kubler and Birbaumer 2008)). When the data were analyzed offline, one LIS patient reached 79 % accuracy. Out of six patients showing command-following at bedside (four MCS, two LIS), five could not be detected with the system (false-negative rate: 83 %). However, these results should be interpreted cautiously because the offline analysis used data from both the command-following and communication sessions to determine the presence of command-following.
Pokorny et al. tested a different auditory P3-based paradigm based on tone stream segregation allowing for binary decisions in 12 patients (10 MCS, one VS/UWS, one emerging MCS1). Two tone streams with infrequently and randomly appearing deviant tones were presented to the patient. This paradigm is suggested to be simpler than the previous ones as only two classes of stimulation are used. The patients were asked to count the number of deviants in one stream and thus modulate the P3 response in the attended stream. Only five patients could achieve results above chance level, and none of them achieved performances allowing communication with the system. In addition, response to command could be detected in nine patients after averaging all the responses obtained, although in two of them the response duration was very short (between 30 and 60 ms). Finally, out of three patients showing response to command at bedside (two MCS, one emerging MCS1), two could be detected with this paradigm. Note that command-following in the emerging MCS patient could not be detected with BCI. It is important to highlight that this paradigm was first used in healthy controls and had to be adapted to be usable with patients with DOC, reflecting the difficulty of applying a BCI paradigm efficient in non-neurologically impaired samples to brain-injured patients. Modifications to the paradigm included using fewer electrodes, adding a simple paradigm to habituate the patient to the task and to test the presence of a P3 response, using blocks of five consecutive trials with the same target stream instead of a randomized order to decrease the cognitive load, and adding additional auditorily presented instructions at the beginning of each run (Pokorny et al. 2013).
Finally, extensive research on attention involving healthy subjects has suggested that the P3 response should be deconstructed into separable subcomponents represented by the P3a and P3b. The relatively early frontally centered novelty P3a is thought to reflect exogenous attention, triggered by “bottom-up” stimulus novelty that may be task irrelevant. The later, parietally focused target P3b, on the other hand, is seen as a marker of “top-down” or volitional engagement of endogenous attention to task-relevant targets to be consolidated into working memory and made available for conscious access (Polich 2007). Based on this idea, Chennu et al. (2013) used a task designed to engender exogenous or endogenous attention, indexed by the P3a and P3b components, respectively, in response to a pair of word stimuli presented auditorily among distracters. They included 21 patients (nine VS/UWS; 12 MCS). Among these patients, three of them (MCS) generated only early non-discriminative responses to targets, suggesting that involuntary bottom-up attentional orienting might be preserved in a greater proportion of patients. In addition, one patient in VS/UWS generated a P3a as well as a P3b response, suggesting a preserved “top-down” or volitional engagement of endogenous attention. Out of the seven patients showing command-following at bedside, none of them generated a P3b (false-negative rate: 100 %), and only two of them showed a P3a (false-negative rate: 71 %). Interestingly, 20 of these patients were also administered the fMRI paradigm developed by Owen et al. (Owen et al. 2006; Monti et al. 2010). In six patients in whom a discernible P3a/P3b response could be elicited, a response to command using fMRI tennis imagery task could be detected. This discrepancy may be explained by vigilance fluctuation, as the paradigms were completed at different times. These results also suggest that the level of difficulty required by this attention task is too high to enable a good rate of detection of conscious patients. However, the VS/UWS patient who showed P3a/P3b responses did also show a response to command with the fMRI, supporting that the presence of a P3a and P3b may highlight a preserved volitional attention process.
11.4 Systems to Detect Response to Command at Bedside
BCI research has classically focused on systems using sophisticated EEG or fMRI techniques, which may be practical for clinical diagnosis, but become challenging in daily use. For this reason, other tools have also been developed and tested in patients with DOC to detect motor-independent response to command at bedside.
Bekinschtein et al. studied 10 patients with DOC (eight VS/UWS, two MCS) using electromyography (EMG; recording of muscle activity) (Bekinschtein et al. 2008). They auditorily presented four different 30 s blocks of commands to the patient: “Please try to move your right hand” and “Please try to move your left hand.” At the end of the block, the instruction was “Please do not move, stay still.” Two control auditory phrases were used: “Today is a sunny day” and “It is raining outside today.” They reported that one VS/UWS patient and both MCS patients demonstrated an increased EMG signal specifically linked to the command, suggesting that electromyography could be used to objectively detect residual motor responses in this population. One MCS patient could follow command at bedside and showed increased EMG activity with the system.
Stoll et al. (2013) investigated the applicability of an alternative physiological signal, the pupil dilation, that can be readily and noninvasively measured with robust, inexpensive, easy-to-use equipment, to communicate with motor-disabled patients and patients with DOC. Pupil dilation has been related to a variety of cognitive functions and is a response that could be used to circumvent the challenges associated with the practical use of traditional BCI approaches. Twelve LIS patients (seven typical LIS and four severely brain-injured LIS with supratentorial lesions) and one MCS patient were included in the study. They reported that three out of seven LIS patients showed significantly higher performances than chance when answering yes-no questions using pupil dilation. However, none of the severely brain-injured LIS patients reached significance. Interestingly, they also used pupil response to detect command-following in one MCS patient following command at bedside. In this study, nine out of 12 patients could communicate at bedside, but could not use the system (false-negative rate: 69 %).
Although preliminary results suggest that these tools may provide simpler bedside methods of detecting command-following and communication with the potential to assist the clinician and improve the accuracy of diagnosis, some limitations prevent their use in patients with DOC. First, EMG still necessitates the preservation of some residual voluntary muscle activity which would prevent its use in patients with severe paralysis or chronic spasticity. Second, pupil response can be altered by the use of centrally acting drugs. Finally, as in fMRI and EEG, restlessness can lead to non-interpretable results. However, future studies should start using these alternative systems in conjunction with EEG and/or fMRI to investigate the integrity of cognitive function in this population.
11.5 Guidelines for Future Research
The high false-negative rate achieved with current BCIs highlights the need to develop more accurate paraclinical diagnostic tools for the DOC population (see Table 11.1). Indeed, a system that is not sensitive to detecting patients diagnosed as conscious at the bedside could not be reliably used in patients with unclear diagnoses. Similarly, a system which is very sensitive and detects signs of consciousness in all patients behaviorally diagnosed as conscious but also a majority of unconscious patients would not be specific enough to be reliable for clinicians. Currently, research on BCI in patients with DOC will have to overcome a number of challenges:
1.
Brain-injured patients are likely to present arousal fluctuation, fatigue, and limited attention span, especially in MCS (Giacino et al. 2002). For this reason, paradigm complexity (stimulus, instructions) and duration are important factors to consider when evaluating BCI applications. Moreover, multiple repetitions of the BCI session must be considered to ensure a reliable diagnosis and account for fluctuation. In terms of communication, evaluation should be assessed with simple questions as severely brain-damaged patients may have difficulty giving accurate answers to trivial yes/no questions (Nakase-Richardson et al. 2009).
2.
Brain injury can be associated with sensory deficits (such as cortical deafness, blindness, or oculomotor impairments (Lew et al. 2009; Alvarez et al. 2012; Pogoda et al. 2012; Rowe et al. 2013)). While BCI research in healthy participants seems to highlight better performance with a visual as compared to auditory or tactile BCIs (Kubler et al. 2009; Halder et al. 2010; Pham et al. 2005), the key challenge here will be to develop reliable systems offering stimuli, instruction, and/or question presentation through multiple domains. A recent study reporting the applicability of a vibrotactile P3-based BCI in LIS patients might enable us to provide systems using a wider range of modalities taking into account various sensory deficits (Lugo et al. 2014).
3.
A certain amount of cerebral reorganization and neuroplasticity might occur in several cases resulting in the recruitment of other brain areas during the performance of a given cognitive task, limiting a direct comparison with results observed in healthy controls (Chennu et al. 2013; Nam et al. 2012). In addition, future studies should take into account the topographic and latency variability observed in healthy subjects to interpret patients’ data (Kaufmann et al. 2011; Bianchi et al. 2010).
4.
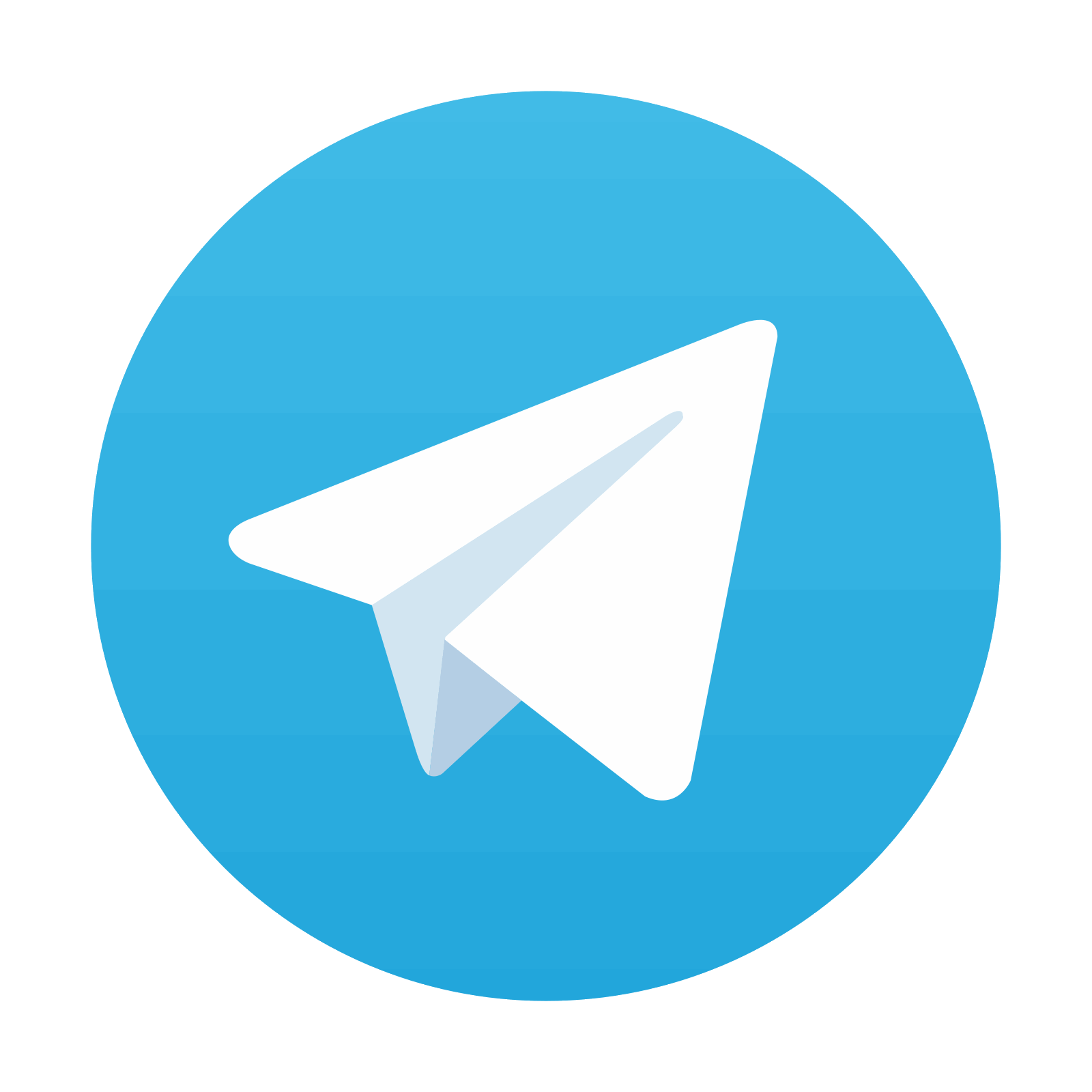
Suboptimal data quality due to movement, ocular, and respiration artifacts in these challenging populations may also be confounding factors that need to be overcome with the assistance of appropriate statistical analyses. It also needs to be pointed out is that, in EEG,the classification accuracy achieved with a BCI naturally depends on the quality and inter-trial consistency of the data used to train the classifier (Goldfine et al. 2011, 2013; Cruse et al. 2013). This is problematic for most patients with DOC, particularly those in MCS, who are prone to frequent and prolonged bouts of fatigue and fluctuation of vigilance preventing them from paying attention for sufficiently long periods. For many patients, this limitation will adversely affect the classification results (e.g., dependency). It is therefore important to design protocols accordingly (i.e., avoid using blocks of the same stimulation and long-lasting sessions; assess the patient at different time periods (Cruse et al. 2013; Goldfine et al. 2013)), in order to decrease the number of false negatives. In addition, this will help us to take care of the false positives (patients detected as “responders” with the system who are actually unconscious (Goldfine et al. 2013)).
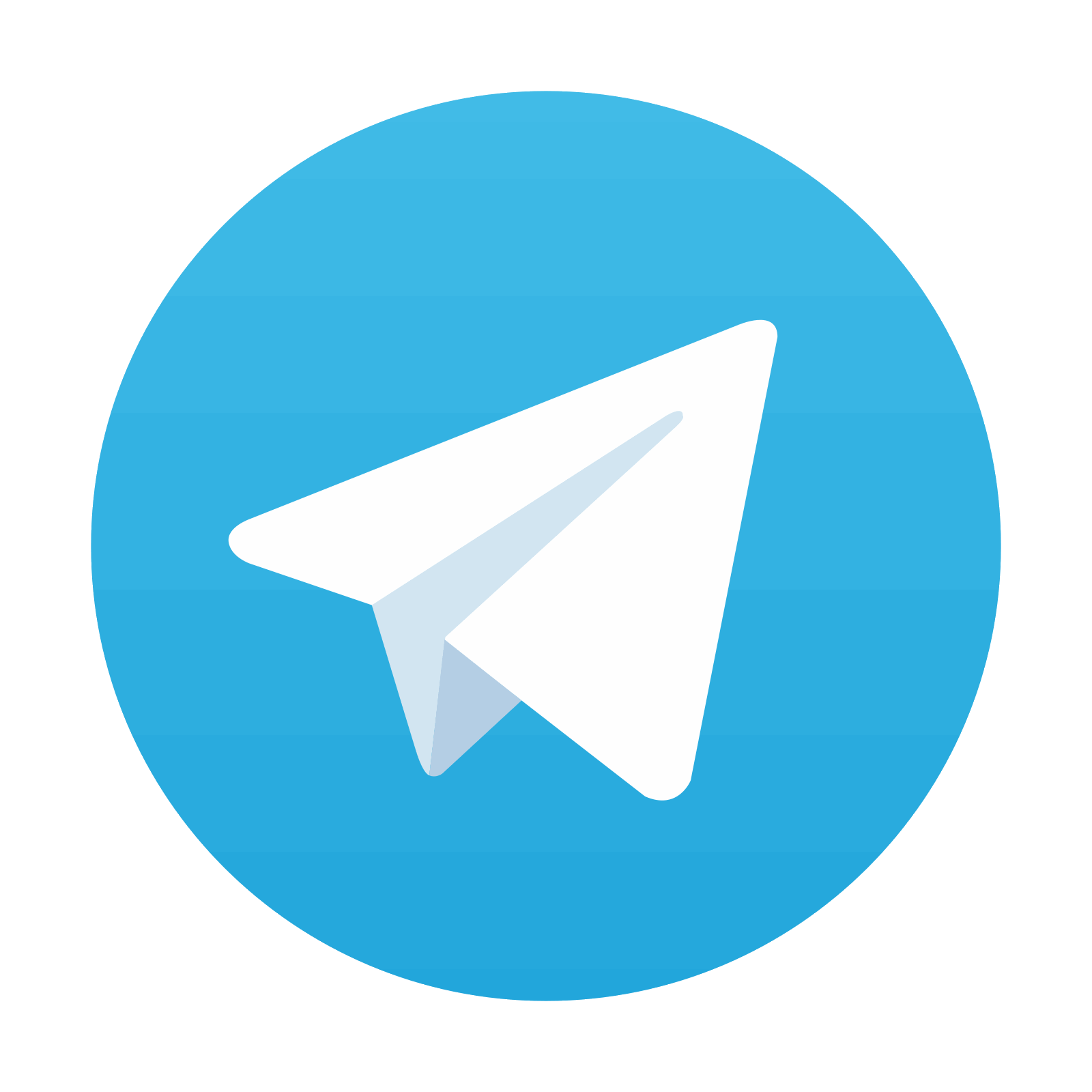
Stay updated, free articles. Join our Telegram channel

Full access? Get Clinical Tree
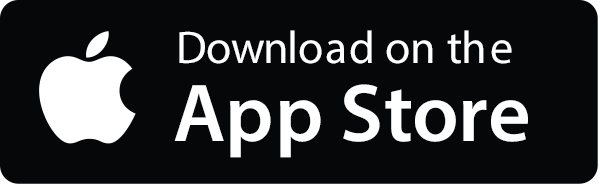

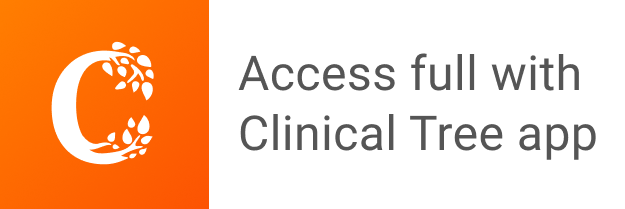