Fig. 21.1
Bidirectional brain-machine interface. (a) A monkey is engaged in a motor task that requires reaching toward screen targets with a cursor. The animal performs the task either with a hand held joystick or using a BMI that converts cortical activity into the cursor position. Two targets are shown, and the correct one is signaled by joystick vibration or intracortical microstimulation applied to somatosensory cortex. (b) Locations of cortical implants. (c) Cortical locations to which microstimulation was applied. Neurons in this area had receptive fields on digits D2–D5
Furthermore, BMIs could be used to augment brain function in healthy people. Examples include BMIs that aid computer gaming (Tangermann et al. 2009) and BMIs that detect drowsiness in drivers (Lin et al. 2010).
We will consider three broadly defined classes of BMIs: (1) motor, (2) sensory, and (3) cognitive. Brain circuits are often classified the same way. While this classification is useful, one should bear in mind that there are no separate motor, sensory and cognitive systems in the brain. Rather, it has been long known that these functions are represented in distributed way by many brain areas, and each individual area multiplexes numerous functions (Lilly 1956; Evarts 1973). Notably, a recent trend in BMIs was to combine sensory and motor functions in one design (O’Doherty et al. 2011).
Facilitated by the improvement of neurophysiological recording methods and the advances in computer systems, BMIs have experienced an explosive development during the last two decades. The number of BMI publications has been growing exponentially. Because of this impressive progress, many ideas previously entertained only in science fiction (e.g., cyborgs, reading-out thoughts and memories) have become feasible today.
Cochlear implants have been by far the most successful neuroprosthetic application, with hundreds of thousands of patients with auditory deficits receiving these devices (Shannon 2012; Wilson and Dorman 2008). Many other BMI types are still at the stage of laboratory research, and much work will be needed to translate them to clinical practice and consumer applications.
As BMIs develop, they bring out a number of ethical issues related to the possibility of BMIs interfering with our thoughts, intruding the mind and taking away our free will (Farah 2002; Vlek et al. 2012). These questions are especially relevant to BMIs that work in the cognitive domain, for example BMIs that mediate decision making (Andersen et al. 2010) and memory processing (Berger et al. 2005).
21.2 Brief History
Multichannel neural recordings is the key technology of modern BMIs. Recording devices that include many electrodes date back to the 1950s, when Lilly implanted several hundreds of electrodes in the monkey cortex and evoked body part movements by the application of electrical stimulation through those electrodes (Lilly 1956).
In the 1960s, research started on neurofeedback, another essential component of modern BMIs. Nowlis, Kamiya, Black and Sterman experimented with electroencephalograms (EEGs) as a source of such feedback (Lebedev and Nicolelis 2006). They converted EEG parameters into auditory or visual signals. Aided with this neurofeedback, subjects (both humans and animals) learned to modulate their brain potentials voluntarily.
In 1963, Walter conducted the first demonstration of an interface between the brain and a mechanical device. He recorded from the motor cortex of patients undergoing neural surgery (Dennett 1992). Walter instructed his patients to advance a slide projector by pushing a button with their hands. The motor cortex electrodes registered readiness potentials, which occurred shortly before the movements were initiated. The potentials were prominent enough to serve as a reliable trigger for the projector instead of the button. Accordingly, Walter disconnected the button and made human brain potentials control the projector directly. The patients continued to perform button presses, and were surprised that the brain interface often completed the job before they moved.
Several years later, Fetz and his colleagues utilized single-unit recordings from monkey cortex as the source of neurofeedback (Fetz 1969). They operantly conditioned monkeys to control the firing of single cortical neurons. Neuronal rates were fed back to the monkeys as visual or auditory signals. The monkeys successfully learned this volitional control, and were even able to modulate single neurons without producing any movements of their own limbs.
Similar research started at National Institutes of Health (NIH) under the leadership of Frank (Frank 1968). Frank proclaimed: “We will be engaged in the development of principles and techniques by which information from the nervous system can be used to control external devices such as prosthetic devices, communications equipment, teleoperators … and ultimately perhaps even computers”. The NIH group recorded from several neurons in monkey motor cortex while their monkeys performed wrist movements (Humphrey et al. 1970). In an offline analysis, wrist kinematics were decoded from the neuronal activity using multiple linear regression (Humphrey et al. 1970). Eventually the decoding was performed online (Schmidt 1980). In these experiments monkeys learned to control a cursor on a LED screen with their cortical modulations.
Research on sensory BMIs (i.e. BMIs that deliver sensory information to the nervous system) started in the late 1950s. In 1957, Djourno and Eyriès implanted deaf patients with single-channel cochlear implants that electrically stimulated the auditory nerve to produce auditory sensations (Wilson and Dorman 2008). Simmons enhanced the cochlear implant with multiple stimulation channels in 1964, and House and Urban obtained an FDA approval for their design of the implant in the 1970s.
Research on prosthetic vision was pioneered by the laboratories led by Brindley and Lewin (1968) and Dobelle et al. (1974). Electrical stimulated of the primary visual cortex was employed to produce artificial visual sensations in blind humans. The subjects experienced sensations of light spots in their visual field, called phosphens. Multichannel stimulation of the visual cortex successfully conveyed simple visual patterns composed of several phosphens.
The late 1990s were marked by a renewed interest to BMI research and its considerable acceleration. Nicolelis and Chapin pioneered invasive BMIs that controlled robotic devices (Chapin et al. 1999). In their experiments, motor commands were decoded from neuronal population activity recorded in the cortex and thalamus of awake, behaving rats. The animals learned to operate a one-dimensional robot with their brain activity alone.
Following this successful study in rodents, Nicolelis moved on to implement a number of motor and sensorimotor BMIs in nonhuman primates, namely cortical BMIs that enacted reaching movements of robotic arms (Wessberg et al. 2000; Carmena et al. 2003; Lebedev et al. 2005), delivered artificial tactile sensations to the brain (O’Doherty et al. 2011), subserved bimanual movements (Ifft et al. 2013), and decoded locomotion kinematics (Fitzsimmons et al. 2009).
Kennedy and his colleagues invented a neurotrophic electrode where myelinated fibers grew into the recording tip (Kennedy and Bakay 1998). An ALS patient implanted with this electrode was able to perform neural on/off control.
A series of BMI studies were conducted by Donoghue and his colleagues. In particular, they implanted paralyzed patients with motor cortical implants. The patients were able to control a computer cursor (Hochberg et al. 2006) and a robotic arm (Hochberg et al. 2012).
Schwartz and his colleagues developed a BMI that enabled three dimensional (3D) movements of a cursor (Taylor et al. 2002) and a robotic arm (Velliste et al. 2008). They also implanted human patients and achieved cortical control of complex movements performed by an antropomorphic robotic arm (Collinger et al. 2013).
Significant contributions to the development of invasive BMIs were made by the laboratories of Andersen, Shenoy and Vaadia. A number of laboratories have been developing noninvasive BMIs, which were extensively tested in humans. Here, valuable contributions came from the groups of Birbaumer, Millan, Müller, Neuper, Kübler, Pfurtscheller, Schalk, Walpaw, and others.
21.3 Neuronal Tuning and Decoding
BMI researchers are to a certain extent lucky, as they can decode behavioral variables from the brain activity even though they very often do not have a clear understanding of what this activity means. Such decoding is possible because neuronal rates are correlated with behaviors. This correlation is fairly consistent, but noisy.
The relationship between neuronal rates and behavioral parameters is often called neuronal tuning. Pioneering studies of neuronal tuning were conducted in the late 1950s–1960s by Mouncastle in the somatosensory system (Mountcastle 2005), Hubel and Wiesel in the visual system (Hubel and Wiesel 2005), and Evarts in the motor system (Evarts 1973). In the 1980s. Wise (1985), Kalaska et al. (1997) and others studied neuronal tuning that represented motor preparation, spatial attention and visuomotor transitions.
An important milestone was achieved by Georgopoulos and his colleagues who studied directional tuning of motor cortical neurons and explored the possibility of decoding motor parameters from neuronal populations. They described the relationship between neuronal rates and movement direction as cosine tuning curves (Georgopoulos et al. 1982). Furthermore, the concept of population vector was introduced to describe encoding at the population level. Population vector proved to be a powerful method not only for decoding ongoing movements, but also for decoding mental transformations, such as mental rotation required to transform stimulus spatial location into movement direction (Georgopoulos et al. 1989). Georgopoulus suggested decoding accuracy can be improved by the recordings from large neuronal populations.
21.4 Decoding from Neuronal Ensembles
Georgopoulos’s population approach was extended by other laboratories and eventually became the mainstream in BMI research (Nicolelis and Lebedev 2009). Decoding improves with the size of neuronal population because noisy fluctuations in the firing of individual neurons cancel each other when the signals of many neurons are combined by the decoding algorithm (Lebedev and Nicolelis 2006; Nicolelis and Lebedev 2009).
Although large neuronal ensembles are generally preferred for BMI decoding, small neuronal samples can be useful in certain cases (Moritz et al. 2008; Taylor et al. 2002). The best known of highly informative neurons, which can be useful for such decoding, are neurons specialized to represent a particular person – the so-called grandmother neurons or Jennifer Aniston neurons (Quiroga et al. 2005).
21.5 Decoding Algorithms
Many BMI decoding algorithms have been developed over the years. The choice of algorithm in each particular case depends on the parameters that have to be decoded from neuronal activity, characteristics of neuronal signals, number of recording channels, and other factors.
Historically, population vector was for a long time the mainstream method for decoding of movement kinematics from cortical activity (Georgopoulos et al. 1989). In this method, each neuron in the population is characterized by an individual vector that points in the preferred direction, i.e. movement direction for which the neuron exhibits the highest firing. The population vector is calculated as a weighted sum of the individual-neuron vectors. The weights are equal to the firing rates of the corresponding neurons. Notwithstanding the historical significance of this method, it is suboptimal for decoding because it does not minimize decoding error.
The Wiener filter is an optimal linear method which, similar to the population vector, represents decoded parameters as a weighted sum of neuronal rates (Haykin 2001). The weights are assigned to the measurements of neuronal rates at several time points in the past, called lags or taps. The weights are optimized to minimize mean-square error.
The Kalman offers additional advantages compared to the Wiener filter, particularly for the paradigms that involve stereotyped behaviors. The Kalman filter handles two groups of variables: state variables, such as limb position and velocity, and observable variables, such as neuronal firing rates. During the decoding, the states are updated in discrete steps, typically every 10 ms–100 ms. Each update incorporates two computations. First, an estimation of a new state is derived from the current state. Second, this estimation is adjusted based on the values of neuronal signals. This latter adjustment utilizes a model of the relationship between neuronal activity and the state variables, i.e. neuronal tuning model.
The unscented Kalman filter (UKF) adds several improvements (Li et al. 2009). It introduces nonlinearity to the neuronal tuning model and also adds a more detailed time history of neuronal rates.
Artificial neural networks (ANNs) are often utilized for BMI decoding (Chapin et al. 1999; Wessberg et al. 2000). Popular ANN methods include Gaussian classifier, multilayer perceptron, Bayesian logistic regression network, adaptive logic network and learning vector quantization network. A recently developed dynamical ANN, called recurrent neural network (RNN), improves decoding by treating neuronal activity as a function of its history (Sussillo et al. 2012).
In addition to continuous decoding BMIs can employ discrete classifiers. For example, BMI spellers extract discrete selections of font characters from EEG activity (Birbaumer et al. 1999, 2008). The list of discrete classifiers used in BMIs includes linear discriminant analysis, support vector machine, hidden Markov models, k nearest neighbors algorithm, and fuzzy logic decoder.
21.6 Theoretical Basis for Motor Decoding
A clear relationship can be seen between BMI approaches to motor decoding and the theories of motor control, which prevailed in the past or currently prevail.
Motor circuitry of the nervous system is commonly described as a hierarchical structure. Cortical areas are typically attributed advanced motor functions, and placed at the top of motor hierarchy. Lower-order areas, such as the brainstem and spinal cord, are attributed simpler, automated motor functions, for example spinal reflexes (Sherrington 1906) and locomotion patterns produced by central pattern generators (CPGs) (Guertin 2009).
The concept of reflex arc (Sherrington 1906) has prevailed for a long time in the motor control field. Reflexes are highly automated motor responses generated in response to sensory inputs. Many simple reflex arcs reside in the spinal cord. Voluntary movements are more complexly organized. They are controlled by higher-order motor areas, including a network of cortical areas. Motor activities usually incorporate both voluntary and reflex components (Cordo and Gurfinkel 2004).
A distinction between voluntary and reflex components can be found in BMI designs. For example, in a shared control BMI the user has control over higher-order parameters, while a robotic controller handles reflex-like tasks.
The concept of internal model is essential for several modern theories of motor control. This concept was first introduced a century ago by Head and Holmes (1911). They proposed that the brain maintains an internal representation of the body, called the “body schema”. A more recent theory, the internal model theory (Kawato 1999), defines two parts of the motor system: the plant and the controller. The controller programs future motor states based on a model of the plant. If there is discrepancy between the programmed state and the sensory feedback from the plant, a correction command is issued by the controller. One possible implementation of such control mechanism is proposed by the equilibrium point hypothesis (Feldman et al. 1998). Here, higher-order motor centers generate a goal for the plant, called an equilibrium point. The plant is then placed to the equilibrium point by a servo mechanism that resides in the spinal cord and utilizes spinal reflexes.
21.7 Arm Movements Enabled by BMIs
A considerable number of BMI studies focused on the control of reaching and grasping movements. In one implementation of such a BMI, monkey cortical activity was converted into the reaching and grasping performed by a robotic arm (Carmena et al. 2003; Lebedev et al. 2005). Monkeys did not see the actual robot, but rather viewed a computer screen. The robot position was depicted by the position of a screen cursor, and the gripping force was indicated by the cursor diameter. Cortical activity was recorded with multielectrode implants placed in multiple cortical areas. Discharges of several hundreds of neurons were sampled simultaneously. The behavioral task consisted of placing the cursor on a screen target and then adjusting the cursor diameter to match the target size. The animals first learned to perform this task with a joystick. The joystick movements positioned the robot, and the animals squeezing the joystick handle to exert a gripping force by. Multiple Wiener filters were trained to extract the robot kinematics and gripping force from cortical activity. During BMI control, the joystick was electrically disconnected from the robot and in some experiments physically removed from the apparatus, and the BMI output controlled the robot instead.
In a similar experiment, Schwartz and his colleagues decoded monkey cortical activity with a population vector decoder (Taylor et al. 2002). This BMI enacted cursor reaching movements in three dimensions (3D). A co-adaptive algorithm was introduced to improve the performance on this task. The algorithm adjusted the population vector weights to minimize the discrepancy between the BMI-generated trajectories and the ideal trajectories connecting the starting point and the target. Following this study, Schwartz and his colleagues developed a BMI driven robotic arm that grasped pieces of food and brought them to the monkey’s mouth (Velliste et al. 2008).
In the next development, the groups of Donoghue (Hochberg et al. 2012) and Schwartz (Collinger et al. 2013) constructed BMI controlled robotic arms for paralyzed patients. The patients were implanted with invasive cortical arrays. The BMIs executed real-time control of reaching, grasping, and object manipulations with the robotic arms.
The researchers at the Nicolelis laboratory advanced this research further by developing a bimanual BMI that operated a pair of virtual arms (Ifft et al. 2013).
21.8 Functional Electrical Stimulation
Several groups explored the possibility of connecting cortical output to the subjects’ own muscles. In these studies, muscle contractions were induced by functional electrical stimulation (FES).
Pfurtscheller and his colleagues powered the paralyzed hand of a tetraplegic patient with an FES device attached to the forearm (Pfurtscheller et al. 2003). The FES was driven by cortical commands extracted from EEG beta rhythms, which the subject modulated by imagining foot movements.
Fetz and his colleagues developed a BMI that decoded activity of motor cortical neurons and drove temporarily paralyzed monkey hands with FES (Moritz et al. 2008).
21.9 BMIs for Locomotion
Recently there has been an increased interest to BMIs that enable locomotion. The Nicolelis laboratory pioneered this research by showing that kinematics of bipedal locomotion can be decoded from neuronal ensembles recorded in monkey cortex (Fitzsimmons et al. 2009). Following this proof of concept demonstration, Nicolelis and his colleagues founded the Walk Again Project, an international consortium for the advancement of BMI controlled exoskeletons (Nicolelis and Lebedev 2009). An European project, called Mindwalker, declared similar goals (Cheron et al. 2012). Yet another group, led by Contreras Vidal, focused on EEG controlled BMIs that enable human walking (Presacco et al. 2012).
In addition to restoration of locomotion by cortical signals, there is an alternative strategy, which is based on the idea of reactivating spinal CPGs. This approach was tested in rats with spinal cord transactions (Courtine et al. 2009). Spinal cord circuitry was pharmacologically conditioned with serotonergic agonists, after which epidural electrical stimulation reactivated the CPG and induced locomotion.
21.10 BMIs and Brain Plasticity
BMI control of an external actuator has much in common with normal usage of tool, the behavior known to provoke brain plasticity. Plasticity associated by tool usage was first shown by Iriki and his colleagues (Iriki et al. 1996). Their monkeys learned to reach toward distant objects with a rake. This learning was accompanied by cortical plasticity: neurons in posterior parietal cortex acquired visual receptive fields that extended along the length of the rake.
Several studies sought for brain plasticity that would result from learning to control a BMI. Overall, the results of these studies support the suggestion that the brain can plastically adapt to represent a BMI-controlled prosthesis, and effectively incorporate the prosthesis into the body schema (Lebedev and Nicolelis 2006; Nicolelis 2011). Several manifestations of such plasticity have been reported: changes in directional tuning of the neurons involved in BMI control (Lebedev et al. 2005), novel modulation patterns associated with learning to operate a BMI (Zacksenhouse et al. 2007), changes in pairwise correlation between neurons (Carmena et al. 2003; Ifft et al. 2013), and adaptions to rotational transformation applied to a subset of neurons used for BMI decoding (Chase et al. 2012).
21.11 Noninvasive BMIs
The safest, nonivasive BMIs, sample neural signals without penetrating into the body. Because of the simplicity of their implementation, noninvasive BMIs have been extensively tested in humans. These BMIs have been utilized for communication tasks, control of limb prostheses, and wheelchair navigation (Galán et al. 2008; Muller-Putz and Pfurtscheller 2008; Nicolas-Alonso and Gomez-Gil 2012; Sellers et al. 2010). Aided with noninvasive BMIs, severely impaired “locked in” patients were able to communicate with the outside world (Birbaumer et al. 1999, 2008).
EEGs are used most commonly in noninvasive systems. There are two major classes of EEG-based BMIs: independent (endogenous) and dependent (exogenous). In an independent BMI, subjects volitionally modulate their brain activity, for example by imagining movements of their body parts. All known EEG rhythms have been utilized in BMIs (McFarland et al. 2006). EEG-based BMIs often employ adaptive decoders (Wolpaw and McFarland 2004).
A dependent BMI relies on an external stimulus that paces EEG responses. Such stimuli are usually presented on a computer screen. A dependent BMI then reads out subjects’ intentions by detecting the differences in EEG patterns associated with attended versus unattended stimuli. For example, visual evoked potential (VEP) based BMIs detect enhanced VEPs over the visual cortex when the subject attends to a particular stimulus on the screen. Steady state visual evoked potentials (SSVEPs) can be also used for the same purpose (Vialatte et al. 2010). SSVEP based BMIs employ/register visual responses to several screen objects, each flickering at its own frequency. P300 evoked potentials can be utilized in a very similar way (Farwell and Donchin 1988).
Several clinically relevant implementations of EEG-based BMIs been developed, such as BMIs that control robots (Millan et al. 2004), wheelchair navigation (Galán et al. 2008), and limb orthoses (Tavella et al. 2010; Pfurtscheller et al. 2003).
EEG artifacts present a considerable problem for EEG-based BMIs. Such artifacts are often not handled adequately (Fatourechi et al. 2007). Consequently, artifacts could be confused with neural signals and could be even used as a control signal for a BMI.
Electrocorticographic (ECoG) BMIs work similarly to the ones based on EEGs while offering a neural signal of better quality. However, implantation of ECoG electrodes is an invasive procedure that requires piercing the skull and dura mater.
Magnetoencephalography (MEG) has found use in BMIs (Mellinger et al. 2007). Brain magnetic fields are detected by magnetometers, superconducting quantum interference devices (SQUIDs). MEG recordings have better spatial and temporal resolution compared to EEGs, but require a specialized, magnetically shielded facility.
Another recording method, Near infrared spectroscopy (NIRS), utilizes an infrared light that penetrates through the skull and monitors oxyhemoglobin and deoxyhemoglobin concentration in the brain blood supply (Sitaram et al. 2009). The spatial resolution of this method is approximately 1 cm, and temporal resolution is on the order of 100 ms. A drawback of this approach is a considerable delay of the hemodynamic response (several seconds).
Functional magnetic resonance imaging (fMRI) has been used in BMIs, as well (Sitaram et al. 2009). The spatial resolution is superb throughout the brain. The temporal resolution is 1 s–2 s, and response lag is several seconds.
21.12 Sensory BMIs
Sensory BMIs strive to repair neural circuitry that processes sensory information. Here, there are many sensory modalities that could be recreated with a BMI: hearing, sight, touch, smell, taste, vestibular sensation, and proprioception.
Normal sensation involves processing of the signals originating from peripheral receptors by a hierarchically organized neural network. Sensory receptors send their information to the spinal cord and brainstem. From there, information ascends to the higher-order areas: thalamus, cerebellum, cortex, and basal ganglia. Neural areas that encode sensory information are often called somatotopic maps. These somatotopic representations resemble a homunculus – a disproportional body with large hands and face, a small trunk and small legs (Schott 1993).
Sensory impairments can be related to problems with sensory periphery, or they can result from damage to higher-order areas. For example, patients with lesions to visual cortex do not perceive visual stimuli, but they may have blindsight, an ability to utilize visual information subconsciously (Barton 2011).
Current sensory BMIs mostly focus on neurological damage to peripheral sensors. BMI systems strive to replace damaged sensory components by artificial sensors. An artificial sensor is interfaced to an intact sensory area, such as cortex or thalamus, and electrical stimulation is usually employed to evoke neural responses (Romo et al. 2000; Fitzsimmons et al. 2007; O’Doherty et al. 2011). Recently invented optogenetic stimulation methods can be used for this purpose, as well (Zhang et al. 2007).
The approach adopted by sensory BMIs bears similarity to the method called sensory substitution. Sensory substitution implies that a compromised sensory channel is substituted by an intact physiological sensation, such as tactile sensation from an unaffected body part. The modality of the substitution sensation can be different from the sensory modality being repaired. For example, vision can be substituted by a skin tactile display connected to a video camera (Jones 2011). As long as intact physiological receptors are used for information delivery, the approach is called sensory substitution. If the method to produce sensation is artificial (e.g., electrical stimulation of the cortex), the approach is called sensory BMI. In some cases, it is difficult to decide if the stimulation method is natural or artificial. For instance, vision can be recreated by electrical stimulation of the tongue (Bach-y-Rita and Kercel 2003; Sampaio et al. 2001).
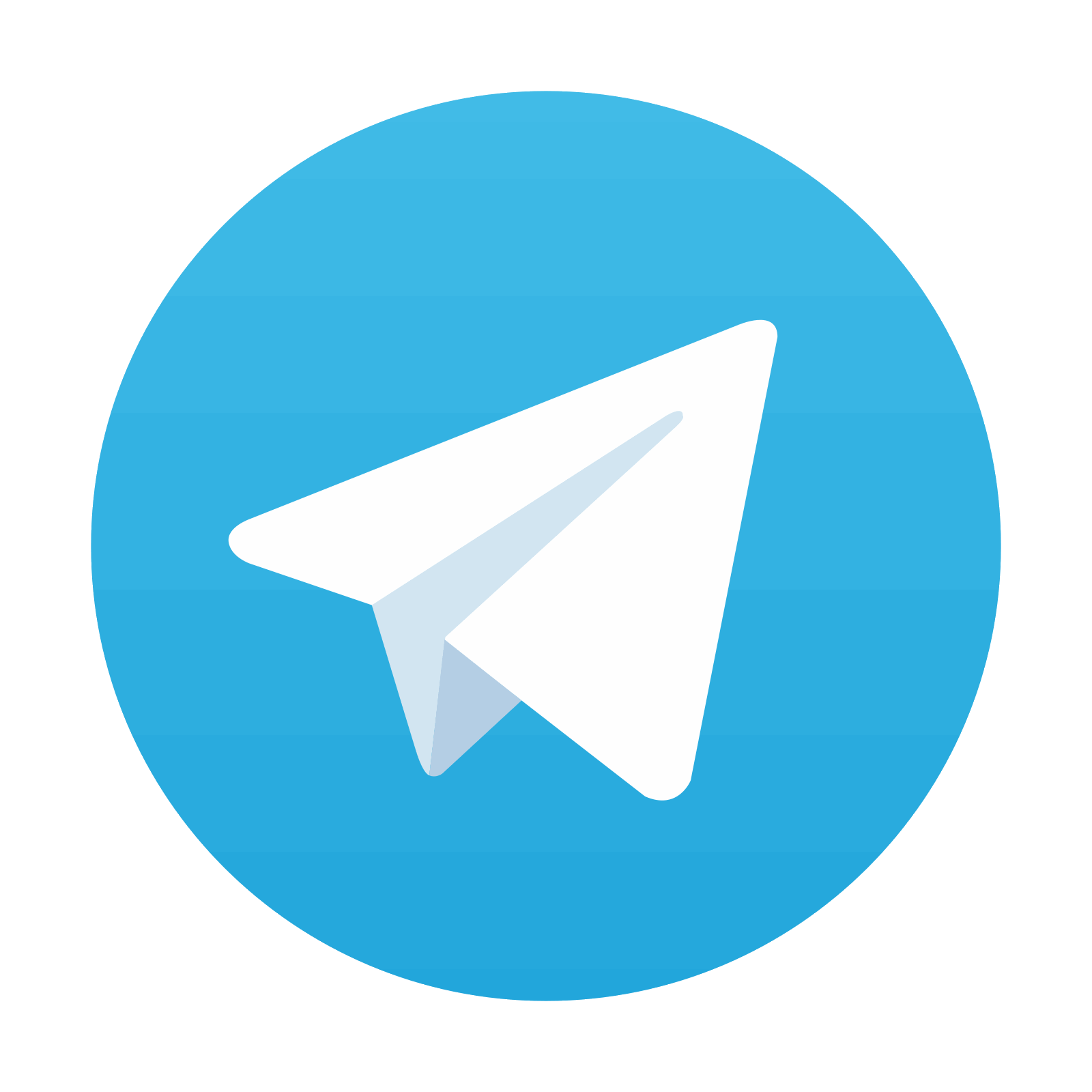
Stay updated, free articles. Join our Telegram channel

Full access? Get Clinical Tree
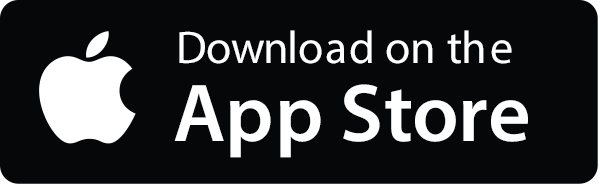
