Fig. 1.1
Illustration of a brain–machine interface (BMI) system for stroke neurorehabilitation training (adapted from Soekadar et al. 2015 [79]). Biosignals, here assessed by whole-head magnetoencephalography (MEG) associated with attempted movements of the paralyzed hand and fingers, are translated into online feedback delivered, e.g., by an orthotic device attached to the paralyzed hand and fingers and combined with simultaneous (brain-state-dependent) transcranial electric stimulation
1.4 Current Challenges of Clinical BMI in Stroke Neurorehabilitation
Almost all BMIs used for stroke neurorehabilitation have been noninvasive, so far. Yet having provided remarkable results, there are some challenges ahead that have to be mastered before broad and out-of-the-lab application of assistive and rehabilitative BMI will belong to the standard treatment options of severe motor paralysis. While low-frequency rhythms, such as SMR, showed limited correlation and contingency with intended movements [80–83], decoding of other brain signals, e.g., high gamma band and action potentials (single- or multiunit) [81–83], allowed for control of high degree-of-freedom prosthetic limbs [9, 36] or FES [12]. As it is hypothesized that Hebbian plasticity involving simultaneous activation of pre- and postsynaptic neurons is critical to driving functional connectivity in an optimal way, invasive BMIs or BMIs providing repetitive and precise contingency between efferent and afferent neural fibers’ activity may prove superior compared to previously used approaches [84]. Recently, it was demonstrated that high gamma band signals allow reconstruction of highly fractionated movements [28] most effectively obtained by invasive recordings using intracortical, subdural, or epidural electrodes [81, 82]. While intracranial electrodes require implantation, epidural or subdural electrodes could ultimately be implanted through a small burr hole reducing risk and cost of surgery.
A substantial barrier here is the lack of fully implantable, wireless intracranial devices. Once fully internalized systems are available, the risk–benefit ratio for paralyzed individuals may change significantly. Similarly, as noninvasive BMIs advance, these may, if providing comparable motor function similarly, change the risk–benefit ratio. Ultimately, the decision of a BMI implantation will be strongly influenced by individual patient circumstances.
In a recent study, the primary motor cortex of a rat was injured leading to a disruption of fibers between the motor and somatosensory areas [85]. An implanted neural prosthesis that translated action potentials in premotor cortex into contingent electrical stimulation in somatosensory cortex led to motor restoration of grasping movements that was indistinguishable from movements performed before the lesion.
Another study used ECoG signals and linked them to direct electric stimulation of a monkey’s spinal cord’s anterior horns. Besides reestablishing motor control of lower limb movements, this approach may prove useful for the rehabilitation of spinal cord injuries or subcortical stroke [86].
Although there is increasing clinical evidence for the efficacy of BMI-related tools in stroke neurorehabilitation, more and larger clinical studies are needed. Also, it is critical to further investigate the underlying mechanisms of BMI-induced functional recovery. A better understanding of the mechanisms underlying motor recovery could also lead to identification of specific and reliable biomarkers predicting treatment response (e.g., [57, 65]. The optimal dosage (frequency and intensity) of BMI training and/or brain stimulation applied in its context still needs to be investigated. Expansion of BMIs from hospital-based to home-based applications may further facilitate applicability of BMI in stroke neurorehabilitation and lower the threshold of including these devices in daily rehabilitation programs. In cases in which ipsilesional BMI training is not feasible, exploration of other strategies, e.g., training of contralesional, ipsilateral brain activity, may be effective [87, 88].
Another challenge is to identify and provide optimal frameworks for generalization of skills learned in the lab or hospital to the patient’s daily life activities. BMI systems following a hybrid assistive and rehabilitative approach may fill this gap and facilitate generalization of motor function reducing the necessity of behavioral physiotherapy. An important aspect when evaluating the efficacy of novel treatment strategies in severe stroke with no residual movements is the availability of reliable and valid instruments for assessment of motor function, as the established assessment scales (e.g., the Fugl-Meyer scale, Wolf test, NIH stroke scale, or ARAT) are rather insensitive to small but significant changes in upper limb movements. Kinematic measures such as sub-movement changes, joint flexibility, and proximal and distal limb movement accuracies [84, 89] should be taken into account as complementary measures to assess BMI-related functional motor recovery in severely affected stroke survivors.
Conclusions
BMIs are novel and powerful tools allowing stroke survivors with severe motor impairment to regain motor function. While larger clinical studies are needed to further investigate mechanisms underlying BMI-related stroke recovery and predictors of treatment response, BMI technology is evolving towards a substantial component of stroke neurorehabilitation. Combination of BMIs with invasive and noninvasive brain stimulation will further improve BMI applicability and promises to close an important knowledge gap linking brain physiology and recovery of brain function after stroke.
Acknowledgments
This work was supported by the Intramural Research Program (IRP) of the National Institute of Neurological Disorders and Stroke (NINDS), USA; the German Federal Ministry of Education and Research (BMBF, grant number 01GQ0831, 16SV5838K to SRS and NB); the BMBF to the German Center for Diabetes Research (DZD e.V., grand number 01GI0925), the Deutsche Forschungsgemeinschaft (DFG, grant number SO932-2 to SRS and Reinhart Koselleck support to NB); the European Commission under the project WAY (grant number 288551 to SRS and NB); and the Volkswagenstiftung (VW) and the Baden-Württemberg Stiftung, Germany. This chapter is an updated and shortened version of “Brain–Computer Interfaces in the Rehabilitation of Stroke and Neurotrauma” published in the first edition of Systems Neuroscience and Rehabilitation and the most recent review article on this topic [79] by the same authors.
References
1.
Feigin VL, Forouzanfar MH, Krishnamurthi R, Mensah GA, Connor M, Bennett DA, Moran AE, Sacco RL, Anderson L, Truelsen T, O’Donnell M, Venketasubramanian N, Barker-Collo S, Lawes CM, Wang W, Shinohara Y, Witt E, Ezzati M, Naghavi M, Murray C (2014) Global and regional burden of stroke during 1990–2010: findings from the Global Burden of Disease Study 2010. Global Burden of Diseases, Injuries, and Risk Factors Study 2010 (GBD 2010) and the GBD Stroke Experts Group. Lancet 383:245–254PubMedCentralPubMedCrossRef
2.
Gustavsson A, Svensson M, Jacobi F, Allgulander C, Alonso J, Beghi E, Dodel R, Ekman M, Faravelli C, Fratiglioni L, Gannon B, Jones DH, Jennum P, Jordanova A, Jönsson L, Karampampam K, Knappm M, Kobelt G, Kurthm T, Lieb R, Linde M, Ljungcrantz C, Maercker A, Melin B, Moscarelli M, Musayev A, Norwood F, Preisig M, Pugliatti M, Rehm J, Salvador-Carulla L, Schlehofer B, Simon R, Steinhausen HC, Stovner LJ, Vallat JM, Van den Bergh P, van Os J, Vos P, Xu W, Wittchen HU, Jönsson B, Olesen J, CDBE2010Study Group (2011) Cost of disorders of the brain in Europe 2010. Eur Neuropsychopharmacol 21:718–779PubMedCrossRef
3.
4.
5.
6.
7.
9.
Collinger JL, Wodlinger B, Downey JE, Wang W, Tyler-Kabara EC, Weber DJ, McMorland AJ, Velliste M, Boninger ML, Schwartz AB (2013) High-performance neuroprosthetic control by an individual with tetraplegia. Lancet 381:557–564PubMedCentralPubMedCrossRef
10.
Moritz CT, Perlmutter SI, Fetz EE (2008) Direct control of paralysed muscles by cortical neurons. Nature 456:639–642PubMedCentralPubMedCrossRef
11.
Pohlmeyer EA, Oby ER, Perreault EJ, Solla SA, Kilgore KL, Kirsch RF, Miller LE (2009) Toward the restoration of hand use to a paralyzed monkey: brain-controlled functional electrical stimulation of forearm muscles. PLoS One 4:e5924PubMedCentralPubMedCrossRef
12.
Ethier C, Oby ER, Bauman MJ, Miller LE (2012) Restoration of grasp following paralysis through brain-controlled stimulation of muscles. Nature 485:368–371PubMedCentralPubMedCrossRef
13.
McGie SC, Zariffa J, Popovic MR, Nagai MK (2014) Short-term neuroplastic effects of brain-controlled and muscle-controlled electrical stimulation. Neuromodulation (in press). doi:10.1111/ner.12185
14.
15.
16.
17.
Dobkin BH (2007) Brain–computer interface technology as a tool to augment plasticity and outcomes for neurological rehabilitation. J Physiol 579:637–642PubMedCentralPubMedCrossRef
18.
19.
20.
Soekadar SR, Witkowski M, Birbaumer N, Cohen LG (2014) Enhancing Hebbian learning to control brain oscillatory activity. Cereb Cortex Neuromodulation (in press). doi:10.1093/cercor/bhu043
21.
22.
Farwell LA, Donchin E (1988) Talking off the top of your head: toward a mental prosthesis utilizing event-related brain potentials. Electroencephalogr Clin Neurophysiol 70:510–523PubMedCrossRef
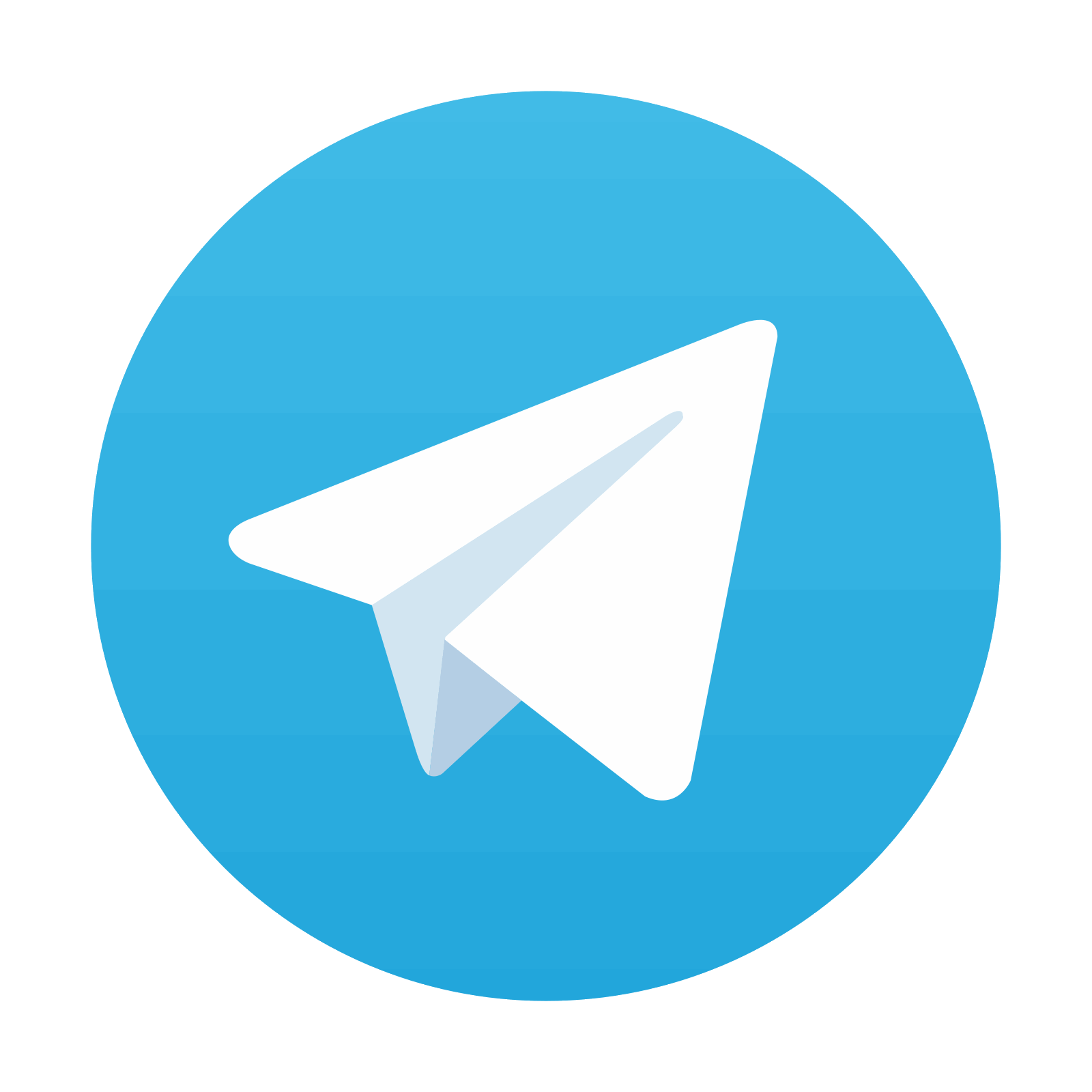
Stay updated, free articles. Join our Telegram channel

Full access? Get Clinical Tree
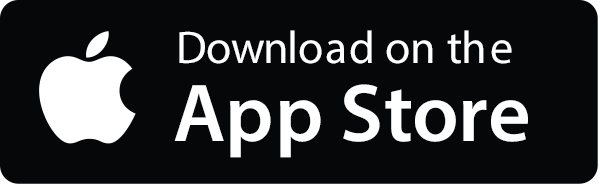
