Fig. 9.1
Meta-analysis of the effects of breastfeeding on cognitive development. Numbers indicate co-variate-adjusted mean differences (and 95 % confidence intervals) for matched composite observations across 11 different studies. Reprinted from Anderson et al. (1999)
In genetics, we can use meta-analyses in order to narrow down the number of genes and/or genetic variants that can be tested for their associations with a given brain phenotype. Given the increasing availability of genome-wide coverage with SNPs, one way to achieve this is through meta-analyses of genome-wide association studies (see Sect. 4.5). Using the catalogue of published GWAS (Hindorff et al. 2012; www.genome.gov/gwastudies/), one can select, for example, a set of SNPs associated—across multiple studies—with a particular group of psychiatric disorders. A somewhat different approach would target all mutations, such as single-point non-synonymous SNPs or small deletions/insertions, linked to a particular disorder through linkage-based analyses (see Sect. 4.5). The human gene mutation database (HGMD, www.hgmd.cf.ac.uk/ac/index.php; see Sect. 4.4) has catalogued over 100,000 disease-causing and disease-associated germ-line DNA mutations, affecting ~3,700 human genes (Cooper et al. 2010). We have used this resource to identify 300+ non-synonymous SNPs contained in our genotyping platform (Illumina HumanOmniExpress BeadChip) and—based on HGMD—linked with various psychiatric disorders, such as autism, schizophrenia, bipolar disorder and Alzheimer’s disease. We then asked whether there is a cumulative effect for being a risk-allele homozygote across these 300+ mutations on the overall cortical surface. As shown in Fig. 9.2, this was the case, but only for female adolescents exposed prenatally to maternal cigarette smoking (Bezgina, French, Paus, unpublished observation). Thus, in addition to the candidate gene and candidate panel approaches for selecting relevant genetic variants, meta-analyses of the catalogued gene–phenotype relationships provide a nice entry point for brain-based analyses.
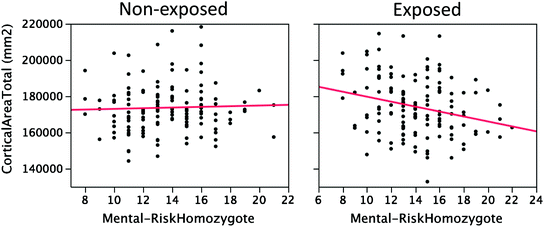
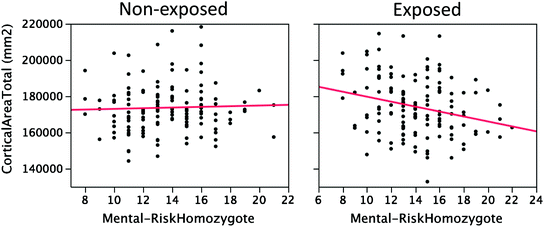
Fig. 9.2
Cortical area as a function of mutation load (number of homozygous genotypes) in exposed (R 2 = .07, p = .002) and non-exposed (R 2 = .00) adolescent females. From Bezgina, French, Paus (unpublished observation)
Finally, can we use meta-analyses for targeting the most relevant brain phenotype? One approach available to us is that of the “activation likelihood estimation” (ALE; Eickhoff et al. 2009), a meta-analytical approach allowing one to identify brain regions that show consistent response to a given stimulus (e.g. a face) across multiple imaging studies.1 The ALE approach can be applied to any imaging modality, functional (e.g. fMRI, PET) or structural (e.g. DTI, VBM), as long as the results have been based on a voxel-wise analysis carried throughout the entire brain and reported using a standardized coordinate system. For example, we have constructed an ALE map of group differences in glucose metabolism—between 915 patients with Alzheimer’s disease and 715 age-matched controls, scanned in 33 studies (Fig. 9.3; Schwartz and Paus, unpublished observation). In this way, we have identified a “vulnerable” and “resistant” brain space in which to test effects of various exposures and genes.
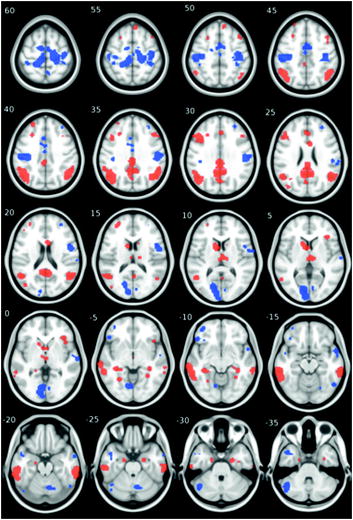
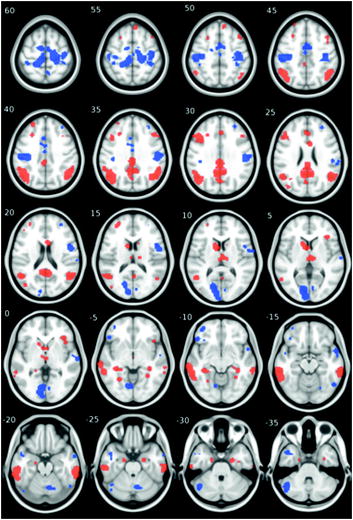
Fig. 9.3
“Vulnerable” (red) and “resistant” (blue) cortical regions. The “vulnerable” regions were identified as showing consistent hypometabolism in resting fluorodeoxyglucose scans in patients with Alzheimer’s disease, as compared to age-matched controls. The “resistant” (control) regions were chosen by positioning an equivalent set of regions in parts of the brain not containing the “vulnerable” regions. Numbers indicate Z coordinates of the axial slices in the ICBM152 stereotaxic space. From Schwartz and Paus (unpublished observation)
9.2 Association Versus Causality
With observational studies, we tend to identify correlations (“breastfeeding is associated with intelligence”) rather than cause-and-effect relationships (“breastfeeding affects intelligence”). Of course, the most desirable findings are those that indicate a causal relationship between exposure and outcome. Is there some way of injecting causality into observational studies?
The use of genetics through the so-called Mendelian randomization is one way of doing so. Mendelian randomization is a special case of the instrumental-variable approach used in econometrics and epidemiology to infer causality (Bowden and Turkington 1984; Greenland 2000; Hernan and Robins 2006). Here, a functional genetic variant (an instrumental variable), which is “randomized” with regards to the inheritance of other traits, acts as a proxy for an environmental exposure without being confounded by other variables (Ebrahim and Davey Smith 2008; Sheehan et al. 2008). For example, the known association between breastfeeding and IQ may be due to a number of factors—from mother–infant attachment to various macro- and micronutrients contained in breast milk. A study of a gene variant (FADS2) known to influence plasma levels of polyunsaturated fatty acids suggests that, at least in part, this particular exposure “leads” to the higher IQ (Caspi et al. 2007). In our work, we used this approach to show that the hormonal environment, namely testosterone, “affects” the volume of white matter during male adolescence. Male adolescents with an “efficient” (short) version of the androgen receptor (AR) gene showed much stronger relationship between testosterone and the volume of white matter than males with less “efficient” (long) AR (Fig. 9.4; Perrin et al. 2008). This suggests that the androgenic effect of testosterone indeed “causes” the increase in the volume (see below, for additional follow-ups of this observation).
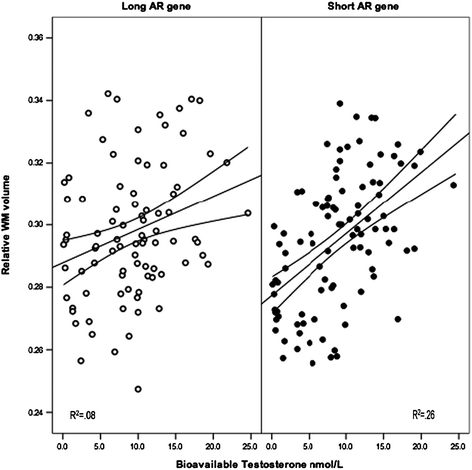
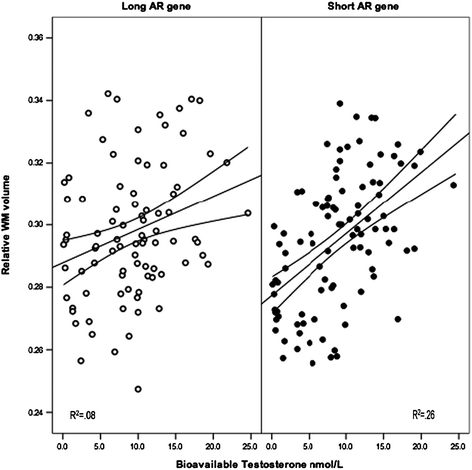
Fig. 9.4
Relative volume of white matter (WM) plotted as a function of plasma levels of bioavailable testosterone in male adolescents with high (long AR gene) and low (short AR gene) number of CAG repeats in the AR gene. From Perrin et al. (2008)
Causality and its direction can also be determined by temporal criteria (i.e. the cause must precede the effect). Provided enough data have been acquired with an appropriate sampling frequency, the directionality of “transactions” occurring across time between hypothetical exposures and outcomes can be examined with appropriate statistical models (e.g. time-lagged analysis; see Sect. 1.5 on developmental cascades). This is very important given the bidirectional nature of brain–behaviour relationships (see Sect. 7.1). Let us assume, for example, that we assessed repeatedly a large sample of typically developing adolescents for the use of marijuana, the presence of psychosis-like symptoms and their brain structure, at 12, 16 and 20 years of age. As illustrated in Fig. 9.5, the difference in the size of the nucleus accumbens at 12 years of age would predict the use of marijuana at 16 years of age, and the use of marijuana at 16 years of age would predict, in turn, white matter properties and the presence of psychosis-like symptoms at 20 years of age.


Fig. 9.5
Cascade of hypothetical events leading to the occurrence of changes in white matter and psychosis-like symptoms at 20 years of age
This sequence of events suggests that unknown prepubertal factors (genetic and/or environmental) underlie the inter-individual variation in the size of the nucleus accumbens and that, in turn, increases the probability of smoking marijuana at 16. Marijuana use then leads to the simultaneous emergence of changes in the white matter and psychosis-like symptoms by the time the individual reaches 20 years of age. Needless to say, this timeline alone is insufficient for establishing an unequivocal cause-and-effect relationship between the above events. If combined with the Mendelian randomization (e.g. functional polymorphisms in the cannabinoid receptor CB1 gene), it would represent an excellent basis for an intervention designed as a randomized controlled trial.
Randomized controlled trials represent the ultimate test of causality available in human populations. Exposures are assigned at random in a sample drawn from the general population. Relevant phenotypes are measured before and after the exposure. But for a number of reasons—including ethics, feasibility and cost—few exposures can be tested in this manner. Yet it is possible to design relevant interventions using ethically acceptable alternatives. For example, one cannot assign randomly selected newborns to be either breastfed or formula fed. As implemented in the promotion of breastfeeding intervention trial (PROBIT), however, it was possible to randomize individual maternity hospitals (in a country) into ones that comply with breastfeeding practices based on the WHO/UNICEF Baby-Friendly Hospital Initiative vs. those that continue with the practices in place at the time of randomization (Kramer et al. 2002). Using this approach, breastfeeding was found to be associated with higher verbal IQ but not with variations in BMI or blood pressure in childhood (Martens 2012 ). Overall, as suggested by Wareham et al. (2008), “Mixed approaches combining data from large-scale observational studies with smaller intervention trials may be ideal”.
Of course, experimental studies carried out in vivo (animal models) or in vitro (e.g. brain slices, tissue cultures) provide an excellent vehicle for testing causality of the relationships observed in human studies. In the Saguenay Youth Study, for example, we have shown that prenatal exposure to maternal cigarette smoking is associated with drug experimentation (i.e. number of substances tried in the lifetime) during adolescence (Lotfipour et al. 2009). Even though we controlled for a number of potential confounders, we could not be certain about the causality of this relationship. Also, given that cigarette smoke contains thousands of chemicals, we could not simply attribute this “effect” to nicotine. This is why a study carried out by Franke et al. (2008) in rats was essential for guiding the interpretation of our findings. In this study, adolescent rats prenatally exposed to nicotine showed no inclination to self-administer cocaine at a low dose (Fig. 9.6a); they did so, however, when offered a high dose (Fig. 9.6b). The opposite was true about the non-exposed rats. By using an experimental manipulation, this finding reveals a causal relationship between prenatal exposure to nicotine and adolescent behaviour. It also offers a possible interpretation of our human studies that nicotine lowered the reward threshold, possibly leading to further search for a substance associated with (a stronger) reward. This is but one illustration of the importance of combining both experimental and population neurosciences.
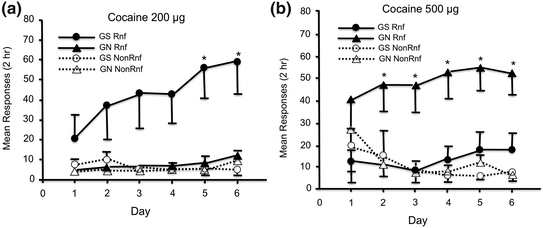
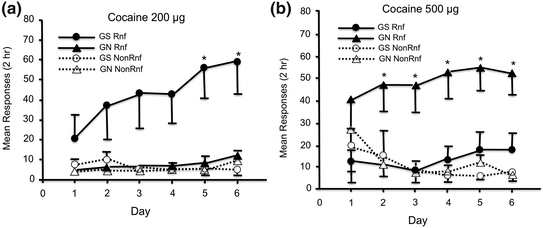
Fig. 9.6
Self-administration of cocaine by rats exposed (triangles) and not-exposed (circles) prenatally to nicotine. GS, gestational saline; GN, gestational nicotine; Rnf, reinforced; Non-Rnf, not reinforced. From Franke et al. (2008)
9.3 Beyond the MR Brain
Without the doubt, MRI is a powerful technique for deriving a large number of structural and functional brain phenotypes. But when interpreting these MR-based measures, we must keep in mind that MR provides but a representation of the human brain—a representation built on specific acquisition sequences and image analysis methods (as reviewed in Chap. 7). It is therefore critical that we keep checking the veracity of such representations, the “MR brain”, against the neurobiological reality. Let us illustrate this challenge with two examples drawn from the work on two structural features relevant to the study of white matter, namely a fibre path and a fibre diameter.
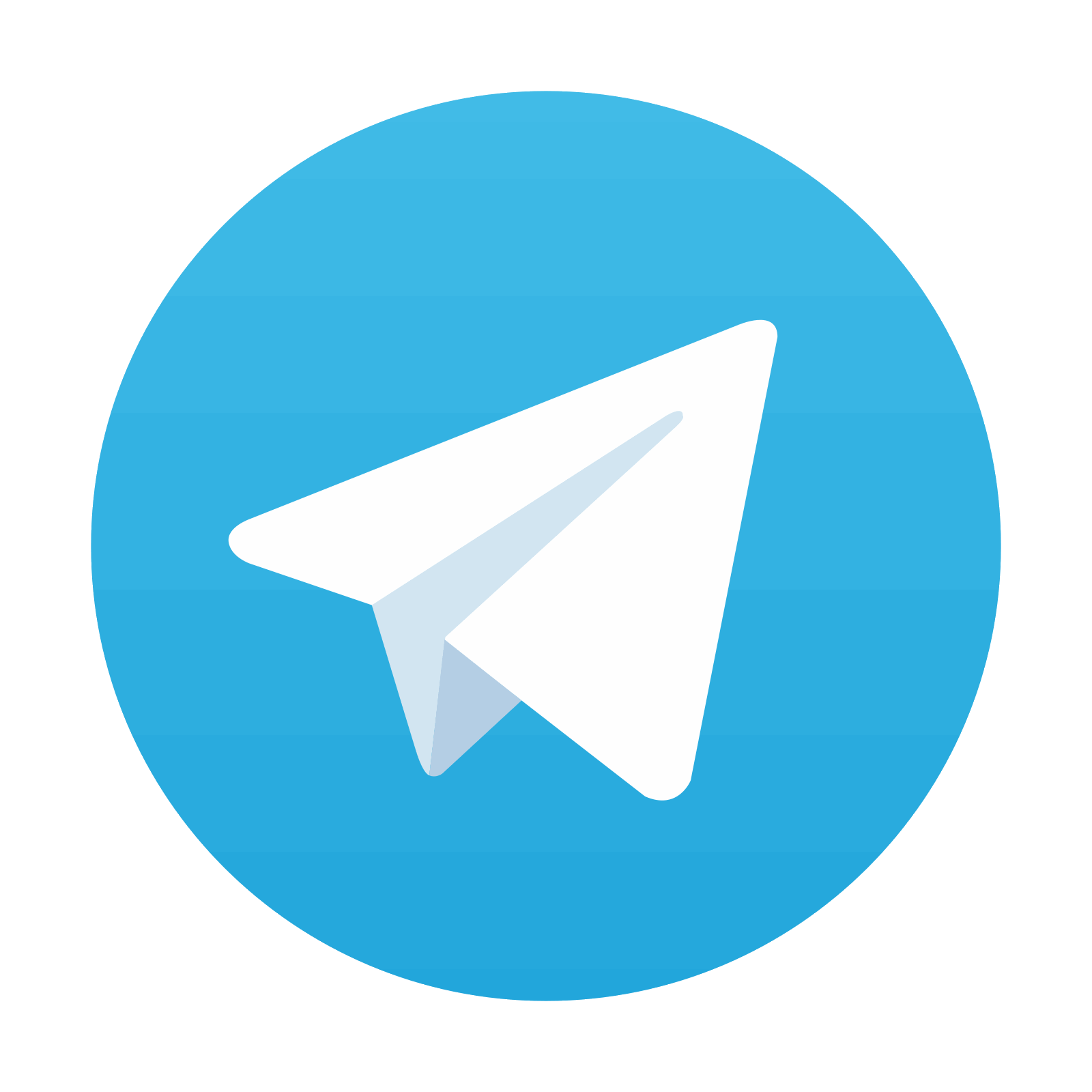
Stay updated, free articles. Join our Telegram channel

Full access? Get Clinical Tree
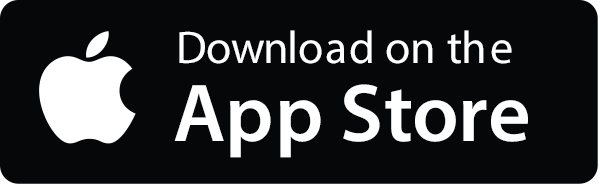
