© Springer Japan 2015
Kenji Kansaku, Leonardo G. Cohen and Niels Birbaumer (eds.)Clinical Systems Neuroscience10.1007/978-4-431-55037-2_1818. Changes in Human Brain Networks and Spontaneous Activity Caused by Motor and Cognitive Learning
(1)
ATR Cognitive Mechanisms Laboratories, Advanced Telecommunications Research Institute International, 2-2-2 Hikaridai, Keihanna Science City, Soraku 619-0288, Japan
Abstract
Recent studies have shown that individual experiences during motor and cognitive learning immediately affect and modify intrinsic functional connectivity and networks, which manifest as correlations in spontaneous brain activities. Resting-state functional magnetic resonance imaging (rs-fMRI) is a powerful tool for investigating such changes in humans. It has been suggested that changes in rs-fMRI after learning reflect (1) a consolidation or encoding process of acquired memory and/or (2) plastic changes in intrinsic connectivity and networks induced by learning. Because these changes are thought to construct brain networks in individuals while interacting with genetic factors, it has been demonstrated that individual differences in behavioral or cognitive performance can be predicted from the states of connectivity and networks observed in rs-fMRI. This chapter reviews studies on changes in resting-state connectivity caused by learning in humans. Possible applications of their results to rehabilitation are suggested, including evaluation of training effects and development of tailor-made programs for rehabilitation.
Keywords
Cognitive learningIntrinsic networksMotor learningResting-state connectivity18.1 Introduction
The traditional view of spontaneous neural activity as “noise” has been challenged by recent findings that spontaneous activity is highly structured in space and time. Such structures of activities are linked to the underlying connectivity of the brain networks, and they provide important clues to understanding the networks [1]. In functional neuroimaging studies on humans, a growing number of studies have used resting-state functional connectivity MRI (rs-fcMRI) , which detects correlations in low-frequency oscillations of spontaneous blood oxygen level-dependent (BOLD) signals during rest [2–4]. These studies have provided insights into the intrinsic architecture of large-scale networks associated with perception, cognition, and action [5, 6], as well as the default or resting state [3].
Animal neurophysiological studies suggest that both genetic and learning factors determine the local structure of spontaneous activity. For example, the firing order of hippocampal cells of mice during resting periods indicates that prewired firing sequences genetically exist for encoding future events [7]. Spontaneous activity in the primary visual cortex (V1) of adult ferrets is more optimally adapted to statistical features in natural images than is that of young ferrets, suggesting organization of the underlying intrinsic networks by visual experience [8]. However, these studies investigated local intrinsic networks in particular brain regions as revealed by neuronal firing patterns.
Regarding large-scale intrinsic networks distributed over the brain as revealed by rs-fcMRI, many findings have focused on genetic and inherent factors. Such evidence is mainly provided by studies related to the task-negative network or default-mode network (TNN or DMN) of regions exhibiting task-related deactivations, as well as such evidence’s anticorrelation with the task-positive network (TPN) that consists of regions exhibiting task-related activations. Namely, the genotype of the dopamine transporter affects the degree of the anticorrelation [9]. The existence of consistent TNN across mammalian species suggests evolutional and genetic organization of the network [5, 6, 10–13]. In a simulation study, anticorrelated clusters arose purely from self-organizing oscillations without external inputs (i.e., experiences) [14].
Recently, however, it has been suggested that large-scale intrinsic networks change according to experience. A study indicated that support vector machine-based multivariate pattern analysis can extract sufficient information from rs-fcMRI data to make accurate predictions on an individual’s brain maturity across development, suggesting that individual experiences in daily life lead to changes in intrinsic connectivity [15]. However, cross-sectional developmental studies have difficulty in identifying particular experiences that cause changes in rs-fcMRI. By contrast, studies investigating changes in rs-fcMRI after a specific learning task are helpful for identifying experiences that cause actual changes in intrinsic networks. This chapter reviews studies on changes in intrinsic networks after learning, and it discusses possible application of rs-fcMRI to rehabilitation.
18.2 Changes in rs-fcMRI Caused by Short-Term Motor Learning
A seminal study investigated rs-fcMRI before and after sensorimotor learning for 11 min [16]. Human subjects manipulated a joystick to move a cursor to a given target on a screen while the direction of the cursor movement gradually became dissociated from that of the joystick movement in every trial, from 0° to 90°. During the 11-minute training session, subjects gradually adapted to the dissociation between the cursor and joystick movements (visuomotor rotation ). The BOLD signal was recorded during rest periods before and after the training session, and intrinsic networks were extracted from low-frequency fluctuation of the obtained BOLD signals by using an independent component analysis. Frontal-parietal and cerebellar components were reliably identified in both rest periods. Moreover, the strength of both components significantly increased in the rest period after the training in comparison to that before training. By contrast, such increase could not be identified in subjects who did not learn the visuomotor rotation and conducted a control task in which the cursor movement was not dissociated from that of the joystick. Therefore, the increase in component strength is specific to the learning but not to the performance (just moving a joystick). This was the first finding indicating a connection between the neuroplastic mechanism of motor learning (probably consolidation of sensorimotor memory) and resting-state activity.
Vahdat and his colleagues investigated specific changes in rs-fcMRI not only for motor areas but also for sensory areas, supporting changes in perception after motor learning [17]. It is known that motor learning results in systematic perceptual changes to the sensed position of the limb [18]. In this study [17], the authors used a “force field ” learning paradigm. While subjects make straight-ahead reaching movements, their hands are pushed leftward or rightward by a robot in proportion to the hand velocity. After repeated reaching in the force field (training), the subjects can move their hand straight ahead by canceling in a predictive manner the force generated by the robot. Moreover, as indicated by the earlier study [18], the sensed limb position shifted in the direction of the applied force after 10 min of training. The BOLD signal was recorded during rests before and after the training, and changes were investigated in functional connectivity. Vahdat and his colleagues developed an analysis technique that allows them to distinguish changes in connectivity that can be attributed to motor learning from those that are related to perceptual changes. Using this technique, they identified a new network in motor learning involving the second somatosensory cortex, ventral premotor cortex (PMv), and supplementary motor area (SMA), and activation of these areas is specifically related to perceptual changes that occur in conjunction with motor learning. They observed the relationships between connectivity changes and behavioral measures of either motor learning or somatosensory perception, suggesting that motor learning results in functionally specific changes to distinct resting-state networks in the brain.
18.3 Changes in rs-fcMRI During Long-Term Motor Learning
Taubert and his colleagues investigated the effects of long-term motor training on resting-state networks and related brain structure [19]. They trained human subjects in a dynamic balancing task , in which subjects tried to keep an unstable platform in a horizontal position as long as possible. The training was conducted for 45 min once a week over six consecutive weeks. The BOLD signal during rest was obtained prior to the training sessions in the first, third, and fifth week as well as in the seventh week. They also scanned structural (diffusion-weighted and anatomical) MR images and investigated the relationship between changes in structural and functional (rs-fcMRI) data. They analyzed rs-fcMRI data using graph-based network analysis and found that the SMA and PMv regions showed increased functional connectivity within their local networks as learning proceeded. Network analysis of the diffusion-weighted images indicated similar changes in connectivity. Voxel-based morphometric analysis of the anatomical images indicated that gray matter volume increased in the SMA after learning. Their results indicate a tight correlation between training-induced functional and structural brain plasticity on the systems level and, moreover, suggest the functional relevance of rs-fcMRI for morphological adaptation in the brain.
18.4 Change in rs-fcMRI Caused by Perceptual Learning or Associative Encoding
The above studies mainly investigated changes in rs-fcMRI after motor learning. It is known that other types of learning such as perceptual and associative learning also cause changes in rs-fcMRI. Lewis and his colleagues investigated how visual perceptual learning modifies rs-fcMRI between networks engaged by the task [20]. They intensively (thousand of trials) trained human subjects on a shape-identification task constrained to one visual quadrant; consequently, they found that the functional connectivity between the trained visual cortex and the frontal-parietal areas involved in the control of spatial attention significantly decreased after the learning. Moreover, this change correlated with the degree of perceptual learning. Reduced connectivity between the visual cortex and frontal-parietal attention networks is consistent with reports of subjects seeing the target shape effortlessly, as if it “pops out” from the background. These results suggest that a perceptual template for the target shape was developed in the visual cortex and that unattended information from distractors was filtered. That is, the importance of processing in the visual cortex is supported, while such an influence is less pronounced from the higher-order control (frontal-parietal) areas to the visual cortex after the learning.
Tambini and her colleagues investigated the temporal correlation in BOLD signals between the hippocampus and cortical regions during rest following an associative encoding task [21]. They found that the magnitude of correlation between the hippocampus and a portion of the lateral occipital complex (LO) is related to later associative memory performance. Furthermore, the magnitude of hippocampal-LO correlations during rest predicts individual differences in later associative memory. Their results are consistent with the hypothesis that long-term storage of episodic memories is formed by the off-line transfer of information from the hippocampus to the cerebral cortex (LO), allowing a hippocampal-independent cortical representation to emerge. By using rs-fcMRI, they demonstrated that off-line hippocampal-cortical interactions are linked with long-term memory in humans.
18.5 Changes in rs-fcMRI Caused by Neurofeedback Training
Recent studies suggest that humans can learn to control brain activation in a localized region by observing online feedback information from their brain while inside an MRI scanner (fMRI neurofeedback training; for reviews: [22–24]). For example, subjects can learn to control activation in brain regions related to pain processing with corresponding changes in experienced pain [25]. It has been shown that neurofeedback using decoded fMRI signals can induce activity patterns in the early visual cortex corresponding to an orientation, and that the training causes visual perceptual learning specific to the orientation without stimulus presentation or the participants’ awareness of what is to be learned [26]. These training methods may provide a noninvasive means of controlling brain function and cognitive processes, potentially altering neurological or psychiatric disease.
Several studies have used rs-fcMRI to investigate the effects of neurofeedback training on intrinsic connectivity and networks. Schizophrenia patients were trained to achieve volitional control of the bilateral anterior insula cortex [27]. The effects of learned self-regulation were investigated on face emotion recognition and on brain network connectivity. After training, patients recognized disgust faces more accurately and happy faces less accurately. Improvements in disgust recognition were correlated to levels of self-activation of the right insula. According to Granger causality modeling analysis of rs-fMRI time courses, the training increased in the incoming and outgoing effective connections of the anterior insula with other brain regions, including the amygdala, the anterior cingulate cortex, and the prefrontal and parietal regions.
In another study, subjects with significant but subclinical contamination anxiety were trained to regulate activity of a region of the orbitofrontal cortex associated with anxiety by using online feedback information [28]. Subjects’ control over contamination anxiety increased several days after the completion of training. Changes in resting-state connectivity in the target orbitofrontal region with other brain regions correlated with these improvements in anxiety.
A recent study used fMRI neurofeedback to activate the dorsal anterior cingulate cortex for 30 min [29]. Resting-state correlations between all of the cortical voxels’ fMRI time courses were mapped before, immediately after, and 1 day after the training session. The 30-minute epoch of cortical activation induced a lasting restructuring of the functional connections, and the change in connectivity strength of cortical voxels during rest reflected the level of their prior co-activation during the training epoch.
Fukuda and her colleagues directly manipulated functional connectivity between two cortical regions (the primary motor cortex and the lateral parietal cortex) by providing subjects with online information on temporal correlation in fMRI time courses between these regions [30]. Subjects were asked to increase a score reflecting this correlation by conducting a mental motor imagery task. Subjects were not informed that the score reflected the correlation but rather that it reflected the goodness of the imagery task performance; nevertheless, functional connectivity between the target regions during rest increased after 4 days of training. Moreover, the global correlation between the sensorimotor network (including the primary motor cortex) and the default-mode network (including the lateral parietal cortex) increased after training, suggesting propagation of the increase in correlation from regional to global network levels. It was confirmed that the effect lasted more than 2 months.
18.6 Meaning of Resting-State Activity After Learning
To summarize the above studies, resting-state activity or connectivity after learning reflects neural correlates for (1) a consolidation or encoding process of acquired memory [16, 21] or (2) intrinsic connectivity and networks formed by learning [18–20]. These studies indicate that specific experiences during learning change spontaneous activity and intrinsic networks. They also suggest transfer of the training effect into intrinsic spontaneous activity at a resting state. Conversely, intrinsic activity predicts performance of goal-directed tasks such as working memory [31, 32], perceptual learning [33], and intertrial variability in motor response [34]. These studies indicate mutual dependence of task-related activity and intrinsic activity: Activity during tasks modifies intrinsic activity, while intrinsic activity forms the scaffold supporting the activity during goal-directed tasks.
There are ongoing discussions related to contamination of rs-fcMRI by physiologic noise, such as respiratory [35] and cardiac [36] artifacts, and thus considerable skepticism remains regarding the possible existence of intrinsic networks, including the DMN (i.e., TNN), delineated by rs-fcMRI. However, many studies have confirmed the consistency of the networks, across sessions, subjects [5], and species [12]. The majority of the studies reviewed in the previous sections indicate that changes in intrinsic networks after learning are consistent with previous findings in neuroscience, suggesting that rs-fcMRI provides results with reasonable meaning.
18.7 Conclusions: Possible Applications of rs-fcMRI to Rehabilitation
As reviewed above, several studies have utilized rs-fcMRI to investigate brain mechanisms for various types of learning, including motor adaptation and learning, cognitive learning, and neurofeedback training. However, little is known about how rs-fcMRI contributes to studies on rehabilitation, which depends on human learning mechanisms. Possible applications of rs-fcMRI are discussed below based on the results of previous studies.
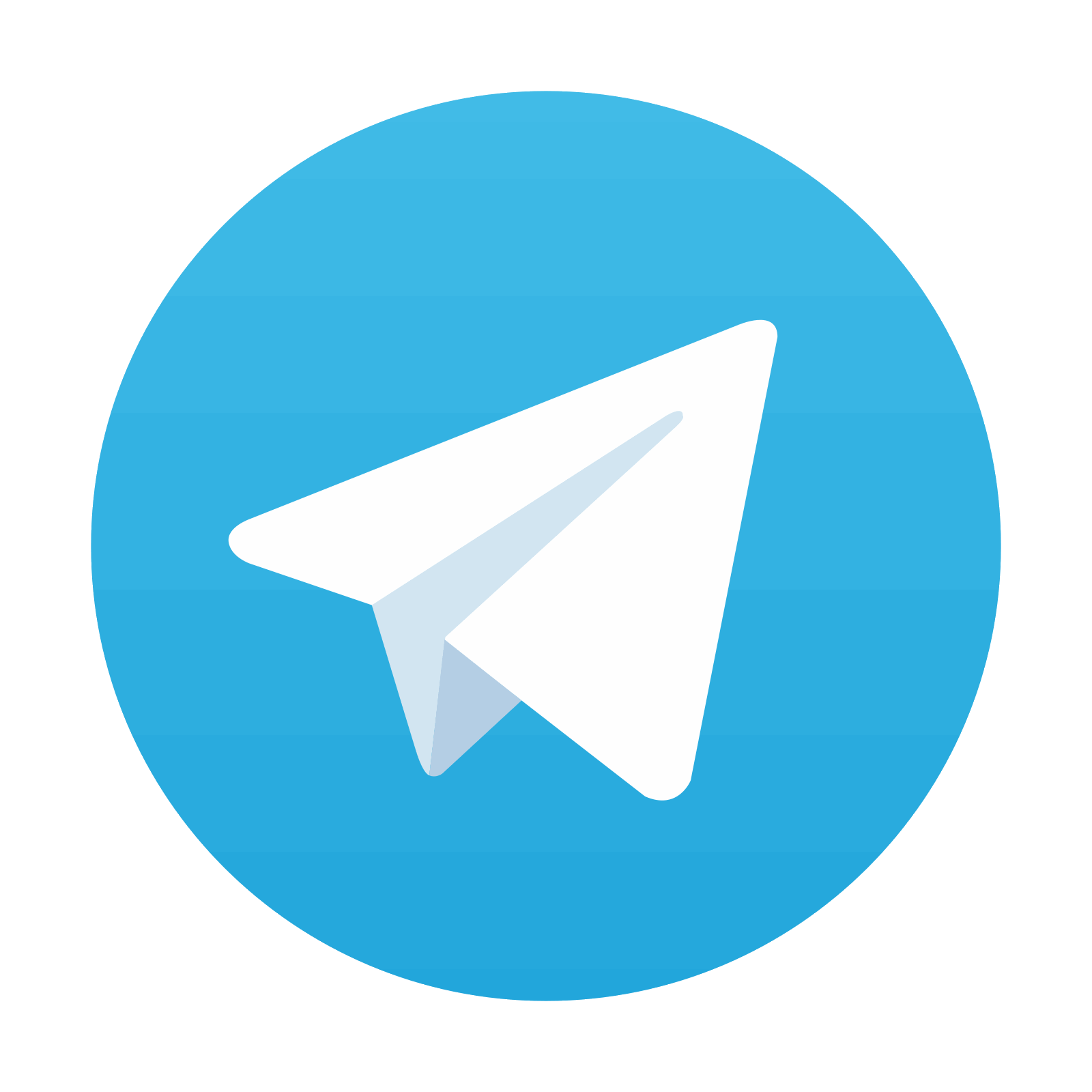
Stay updated, free articles. Join our Telegram channel

Full access? Get Clinical Tree
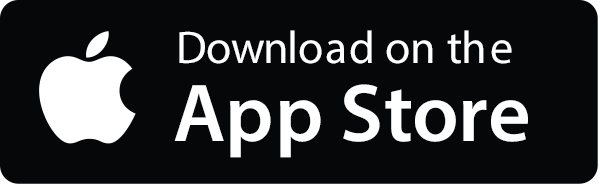
