Fig. 1.1
White-light microscope images of hematoxylin-eosin stained tissue section of selected brain tumors: (a) – gemistocytic astrocytoma (III grade); (b) – oligodendroglioma (II grade); (c) – glioblastoma multiforme (IV grade); (d) – anaplastic astrocytoma (III grade); (e) – meningothelial meningioma (I grade); (f) – fibrous meningioma (I grade)
Elemental “Signature” of Brain Tumors
The X-ray fluorescence (XRF) technique, especially based on synchrotron radiation (SRXRF), offers a nondestructive qualitative and quantitative analysis of chemical elements. The principles of the XRF technique can be found elsewhere (Markowicz 1993). The XRF technique including the SRXRF method has been used in a number of applications in biology and medicine (Szczerbowska-Boruchowska et al. 2011; Ducić et al. 2011; Ortega et al. 2007).
The usefulness of the SRXRF technique for classification (diagnosis) of brain tumors was studied by Szczerbowska-Boruchowska et al. (2011). The following tumor types were investigated: glioblastoma multiforme (11 cases), gemistocytic astrocytoma (2 cases), oligodendroglioma (4 cases), anaplastic oligodendroglioma (2 cases), ganglioglioma (1 case), fibrillary astrocytoma (1 case) and atypical transitional meningioma (1 case). The specimens, taken intraoperatively, were diagnosed histopathologically at the Department of Neuropathology at the Jagiellonian University Medical College in Krakow. The sample preparation procedures for both routine histopathological examination and spectroscopic studies were described in details by Szczerbowska-Boruchowska et al. (2011).
Elemental analysis of unstained tissue section representing various brain tumors was carried out using the SR-XRF technique. The measurements were performed at the bending magnet beamline L at HASYLAB (Hamburger Synchrotrostrahlunglabor, Hamburg, Germany). The primary X-ray energy was set to 17 keV. The incidence beam was focused to a size of 15 μm in diameter with the use of a half-lenses polycapillary. Each tissue section was scanned in two directions at a step size of 15 μm both horizontally and vertically. A typical size of the area of scanning was from 300 to 500 μm in one direction.
The SR-XRF technique allowed for the determination of elements such as P, S, Cl, K, Ca, Fe, Cu, Zn, Br and Rb in all analyzed neoplastic tissues. Based on the masses per unit areas of elements, determined for each point within the scanned area of the tissue sections, two-dimensional maps of elemental distribution were prepared. An example of XRF maps for oligodendroglioma case is shown in Fig. 1.2. For each sample data from 100 measurement points were selected for further statistical analysis. Multiple discriminant analysis (MDA) was used for two purposes: (1) detecting the variables (elements) that allow for discrimination between different tumor types, and (2) classifying cases into groups related to histopathological recognition. In the analysis, the samples were discriminated into groups based on their histopathological diagnosis. It was found that the most significant elements in the general discrimination of tumor type are as follows: S, Cl, Cu, Fe, K, Br and Zn. This means, that the contents of these elements and their relative ratios in neoplastic tissues may be a source of the unique elemental fingerprint of different types of brain tumors. Moreover, the largest difference in elemental composition was observed between atypical transitional meningioma and fibrillary astrocytoma (c.f. Fig. 1.3). As one can noticed from Fig. 1.3 the smallest differentiation, taking into account the elemental composition of neoplastic tissues, was noted between glioblastoma multiforme and oligodendroglioma. The usefulness of the calculated discriminant functions was examined via their ability to correctly classify each data point to their a priori groups. The mean percentage of correct predictions determined according to the a posteriori probabilities procedure was equal to 99.93 %. This means that almost 100 % agreement with histopathological diagnosis was achieved. Apart from the classification of tumor cases used previously for construction of discriminant function model the validation procedure was also carried out for ten new samples. In this case, the mean percentage of correct predictions obtained for the new cases of brain gliomas was 87.6 %. These results show that the elemental studies coupled with multiple discriminant analysis allowed for the classification of different types of brain tumors into separate groups and also even for classification/diagnosis of the unknown cases. Very high predictive accuracies of MDA that were achieved in the study described by Szczerbowska-Boruchowska et al. (2011) should be emphasized. As the authors suggest, the created elemental fingerprinting may be a very useful tool in assisting the process of histopathological diagnosis/classification of brain tumors. Moreover, it was pointed out that the elemental composition of a relatively small fragment of neoplastic tissue represents satisfactorily the elemental “signature” of cancer and using multiple discriminant analysis enables differentiation of various types of brain tumors.
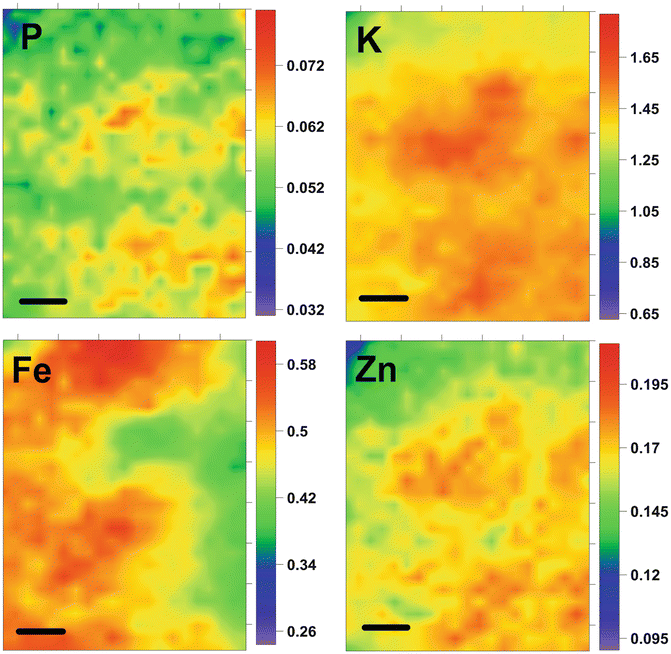
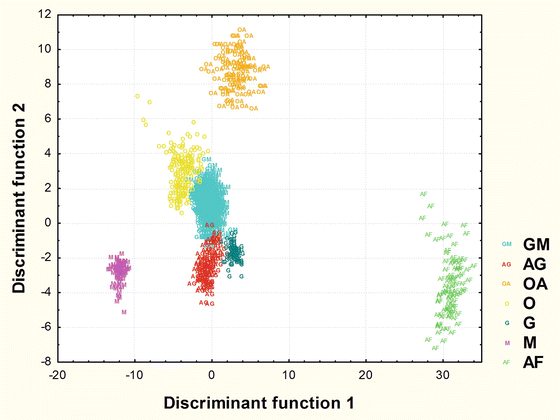
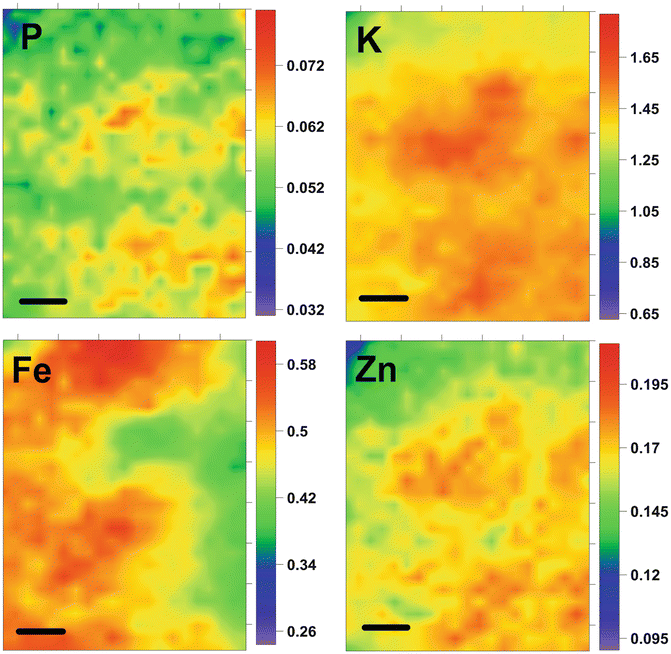
Fig. 1.2
Distribution of selected elements in oligodendroglioma (II grade) tissue section. Data presented in (μg/cm2). Scale bar: 50 μm
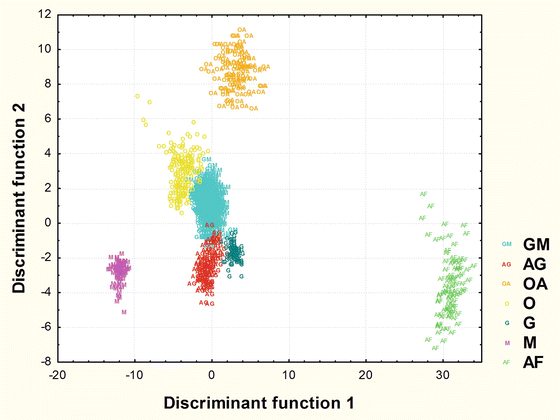
Fig. 1.3
The scatterplot of observations in the space of discriminant variables for different types of brain tumors (OA anaplastic oligodendroglioma, AG gemistocytic astrocytoma, AF fibrillary astrocytoma, GM glioblastoma multiforme, G ganglioglioma, O oligodendroglioma, M atypical transitional meningioma)
Macromolecular Fingerprint of Brain Tumors
Apart from elemental composition of brain tumors an organic components seem to be potential markers of various neoplastic tissues. The main biological macromolecules such as proteins, lipids, nucleic acids and carbohydrates can be satisfactorily identified with the use of infrared (IR) spectroscopy (Miller et al. 2002). Due to unique fingerprinting capabilities, this technique is a very valuable tool for biomolecular investigations. Moreover, IR spectroscopy allows for the probing of tissues and cells at the molecular level. Therefore, it was suggested as a diagnostic method for clinical applications. The selected advantages of IR spectroscopy such us minimal sample preparation required, non-destructive characteristic, no external markers required, and rapidity should be emphasized (Krafft et al. 2006). The IR imaging allows for the determination of two-dimensional distribution of biological macromolecules in tissues and cells. Moreover, molecular structure information can be also obtained from the same analysis, for protein secondary structure and fatty acyl chain peroxidation level. Therefore, several cancer markers can be identified based on IR spectroscopy. Potential applications of IR spectroscopy imaging coupled with linear discriminant analysis as a complement tool for diagnosis of brain tumors were discussed by Krafft et al. (2006, 2007). The authors (Krafft et al. 2006) used IR spectroscopic maps of multiple tissue samples from two patients (with an astrocytoma of III grade and a multifocal glioblastoma brain tumor) to construct a classification model. For this purpose linear discriminant analysis was applied. The chemical properties of training samples were described by the lipid-to-protein ratio (band intensity ratio 2,850–1,655 cm−1), two additional molecular descriptors identified at 1,545 cm−1/1,655 cm−1 (amide II to amide I intensities ratio) and (1,231 + 1,450) cm−1/1,655 cm−1, which are associated with hemoglobin and collagen, respectively. The model was applied to classify normal brain tissue, II grade astrocytoma, III grade astrocytoma, glioblastoma, hemorrhage, and leptomeninges. For each class, three IR spectra were taken as a training set from IR spectroscopic maps of two patients corresponding to the average spectrum, the minimum magnitude, and the maximum magnitude of the spectral features within the metric. Then the LDA model was used to whole IR spectroscopic images obtained for the analyzed tissue sections. The majority of the IR spectra from IR maps were correctly assigned according to their histopathological recognition. However, it was found that glioma tissues are inhomogeneous and might encompass several grades of malignancy. In the same work (Krafft et al. 2006) additional samples were used in the frame of an independent test of the previously constructed LDA classification model. For this purpose tissue sections taken from 15 patients with astrocytoma of III WHO grade, 31 patients with glioblastoma brain tumors, and 5 patients without brain tumor were analyzed. Single IR spectra from these sections were subjected to the LDA model. The results showed that for five normal samples 100 % accuracy was achieved. Fifteen III grade astrocytoma samples were assigned according to their histopathological recognition with 80 % accuracy. For 31 glioblastoma samples 74.2 % accuracy was achieved. Moreover, 20 % of III grade astrocytoma samples were assigned to glioblastoma and 19.4 % of glioblastoma samples were assigned to III grade astrocytoma. This may result from similar chemical composition of lipids and proteins. 6.4 % of glioblastoma samples were incorrectly classified as hemorrhage or leptomeninges. In general, 100 % of III grade astrocytoma and 93.6 % of glioblastoma were recognized as malignant gliomas. No malignant glioma was assigned to normal tissue or II grade astrocytoma. The high score (over 93 % of correct classifications) of the independent test samples shows that linear discriminant analysis based on molecular composition of tissue may be a potentially valuable method assisting classification of brain tumors. The usefulness of IR spectroscopic imaging coupled with linear discriminant analysis for classification of malignant gliomas was also studied by Krafft et al. (2007). In this work multiple IR images obtained for three tissue sections from one patient with a malignant glioma are acquired and assigned to the six classes normal brain tissue, II grade astrocytoma, III grade astrocytoma, glioblastoma multiforme (IV WHO grade), hemorrhage, and other tissue. For the diagnosis of new samples the LDA classification model trained as described by Krafft et al. (2006) was used. The usefulness of such procedure was verified additionally by using different IR detector (the focal plane array detector) for collecting new data than this (the single-channel detector) applied to probe the training samples. Based on the classification of IR spectra it was found that on average 95 % of the tissue cryosection was assigned to malignant glioma (that means III grade astrocytoma or glioblastoma) which points to a location within the tumor. From 12 % to 34 % of specimens classified as malignant gliomas and increased cell density were consistent with a location of the section near the tumor margins. Only single malignant glioma cells (less than 0.2 %) within mostly normal brain tissue of regular cellularity were detected in the cryosection which most likely originated from outside of the tumor. The presented results pinpoint the high accuracy of the LDA model which was trained by IR spectra from different patients and with different detectors. This demonstrates that the IR spectral patterns used to distinguish normal brain tissue and tumors are conserved among patients. Moreover, the LDA model is able to describe and recognize these spectral fingerprints. It should be also emphasized that the chemical information used in LDA model as the selected descriptors is identical in IR spectra which were recorded by single- or multi-channel IR detector. The work described by Krafft et al. (2007) pointed out that the chemical and molecular differences between normal brain tissue and brain tumors are sufficiently large and the appropriate LDA classification model may assist diagnosis/classification of brain tumors.
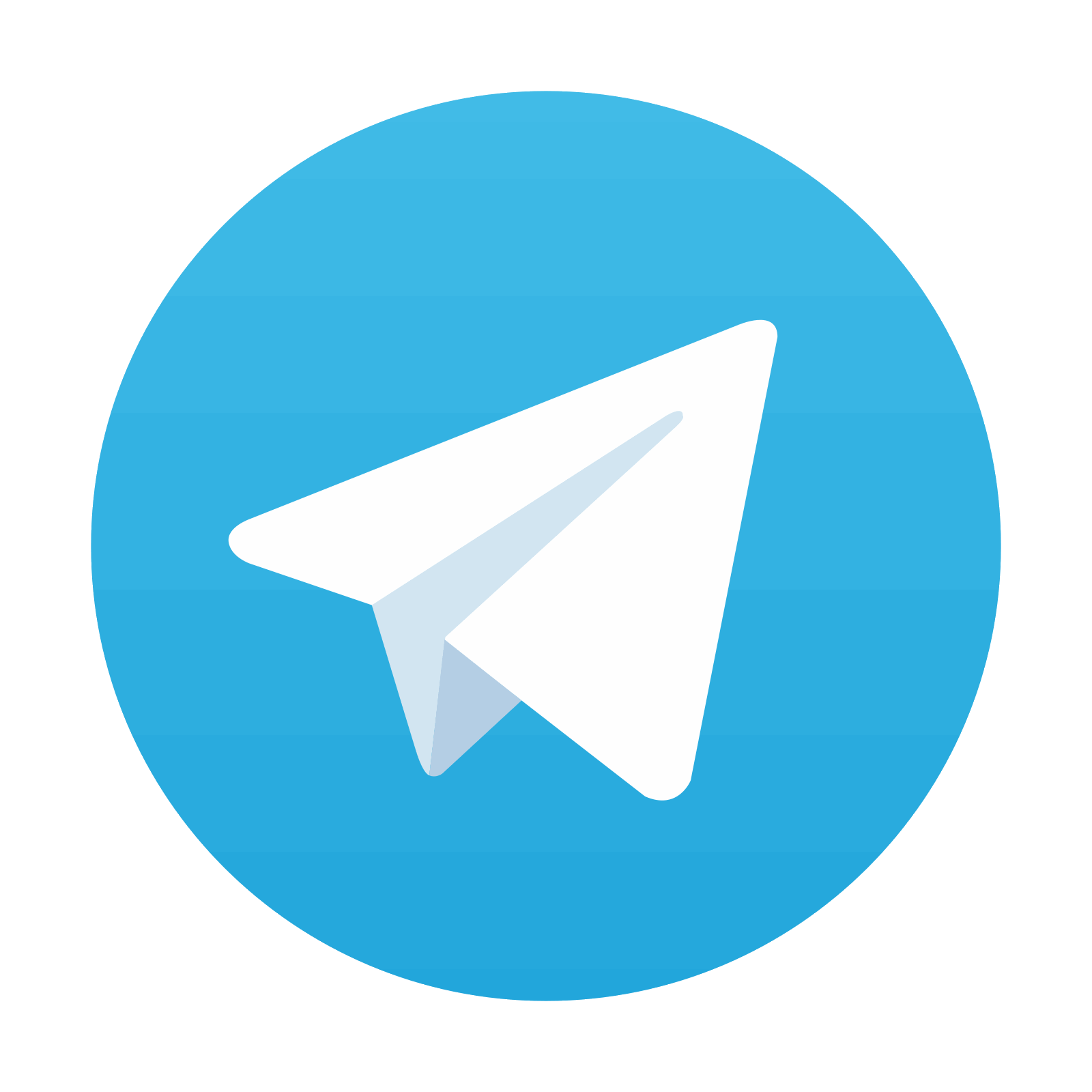
Stay updated, free articles. Join our Telegram channel

Full access? Get Clinical Tree
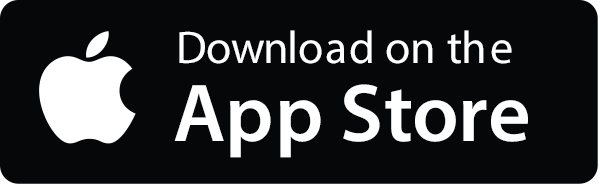
