The Purpose of Clinical Reasoning
It is through clinical reasoning that clinicians collect, weigh, and combine the information required to reach diagnosis; decide which treatment is required; monitor treatment effectiveness; and change their plans if treatment does not work. The study of clinical reasoning, therefore, concerns the cognitive processes that underlie diagnosis and the planning and implementation of treatment.
Diagnosis has three purposes: to aid research, to summarize information, and to guide treatment. For clinicians, the chief purpose of diagnosis is to summarize information in such a way as to guide treatment. In one approach to diagnosis, the clinician matches a pattern of clinical phenomena elicited from the patient against the idealized patterns of disease entities and chooses the diagnosis that best fits. In another approach, the clinician attempts to understand the particular environmental, biological, psychological, and existential factors that have both led to the current problem and perpetuated it. The first approach, therefore, seeks commonality and lends itself to generic treatment planning. The second approach stresses uniqueness and the adaptation of treatment to the individual. In good clinical practice the two approaches are complementary.
Clinical Reasoning & Actuarial Prediction
Diagnosis and treatment are risky ventures, fraught with the possibility of error that can have serious consequences. How can error be minimized? On the one hand are the clinicians who, having elicited information that is generally both incomplete and inferential, diagnose patients and use subjective probabilities to predict outcome. On the other hand are the psychological actuaries who regard natural clinical reasoning as so flawed as to be virtually obsolete and who seek to replace it with reliable statistical formulas.
A considerable amount of research has been conducted into the fallacies and biases that can lead clinicians astray. For several reasons, such research has had little effect on clinical practice. Actuarial experiments sometimes seem artificial, or even rigged (against the clinician), and may be dismissed as irrelevant. Clinicians are prone to concede that others may make a particular mistake in reasoning but they themselves are unlikely to do so. Indeed, clinicians often have a degree of self-confidence that enables them to survive in an uncertain world, and they are not likely to accept their defects unless they see a practical remedy. Finally, clinicians may fear that, if tampered with, their mysterious diagnostic skills will evaporate and be replaced by computing machines.
Research into Clinical Reasoning
There are three types of research into clinical reasoning: clinical judgment, decision theory, and process tracing. Clinical judgment research attempts to identify the criteria used by clinicians in making decisions. Decision theory explores the flaws and biases that deflect accurate clinical judgment. Process tracing elucidates the progressive steps of naturalistic reasoning. The first two types are statistical and prescriptive, the third is normative.
According to the “lens” model of clinical judgment, each patient exhibits a set of symptoms, signs, or criteria that the clinician weighs and combines to reach a decision (e.g., whether the patient is at risk for suicide or whether he or she should be hospitalized). Researchers attempt to “capture the policy” of the expert decision maker in order to construct mathematical models that replicate clinical judgment.
Given the fuzzy nature of clinical data, medical decisions have to be probabilistic. Accordingly, decision theorists base their research on Bayes’ theorem. This theorem states that P(D/F) (the probability that a diagnosis is present given a clinical finding) is a function of P(F/D) (the probability that a finding will be associated with that diagnosis), P(D) (the probability of the diagnosis in that population), and P(F) (the probability of the finding in that population). Thus,
The intuitive clinician gauges P(F/D) from theoretical knowledge and experience. However, P(F/D) must be combined with the local base rates for both the disease and the finding—base rates that are often unknown or ignored by the clinician. Unfamiliarity with Bayes’ theorem and other biases can introduce several errors into clinical reasoning (Table 1–1).
If a set of clinical findings (F) matches a textbook syndrome (D), clinicians are apt to diagnose it without sufficiently taking P(D) and P(F) into account. |
Clinicians must often rely on their subjective estimations of P(D) and P(F). They tend to be overconfident concerning the accuracy of these estimations. |
Clinicians may overestimate P(D) as a result of recent experience (e.g., reading a journal article). Thus, exotic disorders may be overdiagnosed. |
Clinicians are conservative. Despite corrective feedback, they are slow to correct their subjective base-rates. |
Clinicians are prone to rely too much on confirmatory data, whereas negative evidence is more powerful. |
Early preference for a particular diagnostic hypothesis may be hard to dislodge and can deflect the subsequent collection or evaluation of evidence. |
Decision theory has been applied most often to convergent problems, for example, whether or not to hospitalize a patient. The better choice should be the one that has the highest expected utility. Expected utility is the product of the probability of an outcome and its subjective utility (e.g., how highly the patient or physician values that outcome). Consider the following case:
A 14-year-old girl is evaluated in a pediatric hospital ward after she has taken an overdose of 50 acetaminophen tablets. She has made one previous suicide attempt. The recent suicide attempt occurred after she was rejected by a boyfriend. The patient is emotionally labile and clinically depressed. She is hostile to her mother and refuses to agree to a “no-suicide contract.” The consequences of hospitalizing the patient versus not hospitalizing her but referring her for outpatient treatment can be represented in Figure 1–1. The clinician is expected to opt for the choice that leads to the greater expected utility.
However, clinical decisions are usually more complex than can be represented by a simple decision tree of the type shown in Figure 1–1. Multiple branching yes–no decision trees have been constructed to aid diagnostic decision making (see, e.g., Appendix A in Diagnostic and Statistical Manual of Mental Disorders, fourth edition [DSM-IV]) and to encode expert treatment decisions. Utility and probability are also important considerations when cost–benefit analyses are undertaken, for example, concerning the desirability of mass screening procedures.
Research has shown that clinicians do not always follow the expected utility model. For example, decision making may be biased by the readiness with which a particular outcome can be remembered, particularly if it has had an emotional impact on the clinician (e.g., a patient’s recent suicide). Decision making is also affected by the way problems are presented. For example, a treatment that saves 800 lives out of 1000 may be preferred to one that sacrifices 200 out of 1000 (although the two situations are equivalent in risk). Furthermore, the probability of an outcome can sometimes affect the subjective estimation of its utility, whereas theoretically the two should be independent.
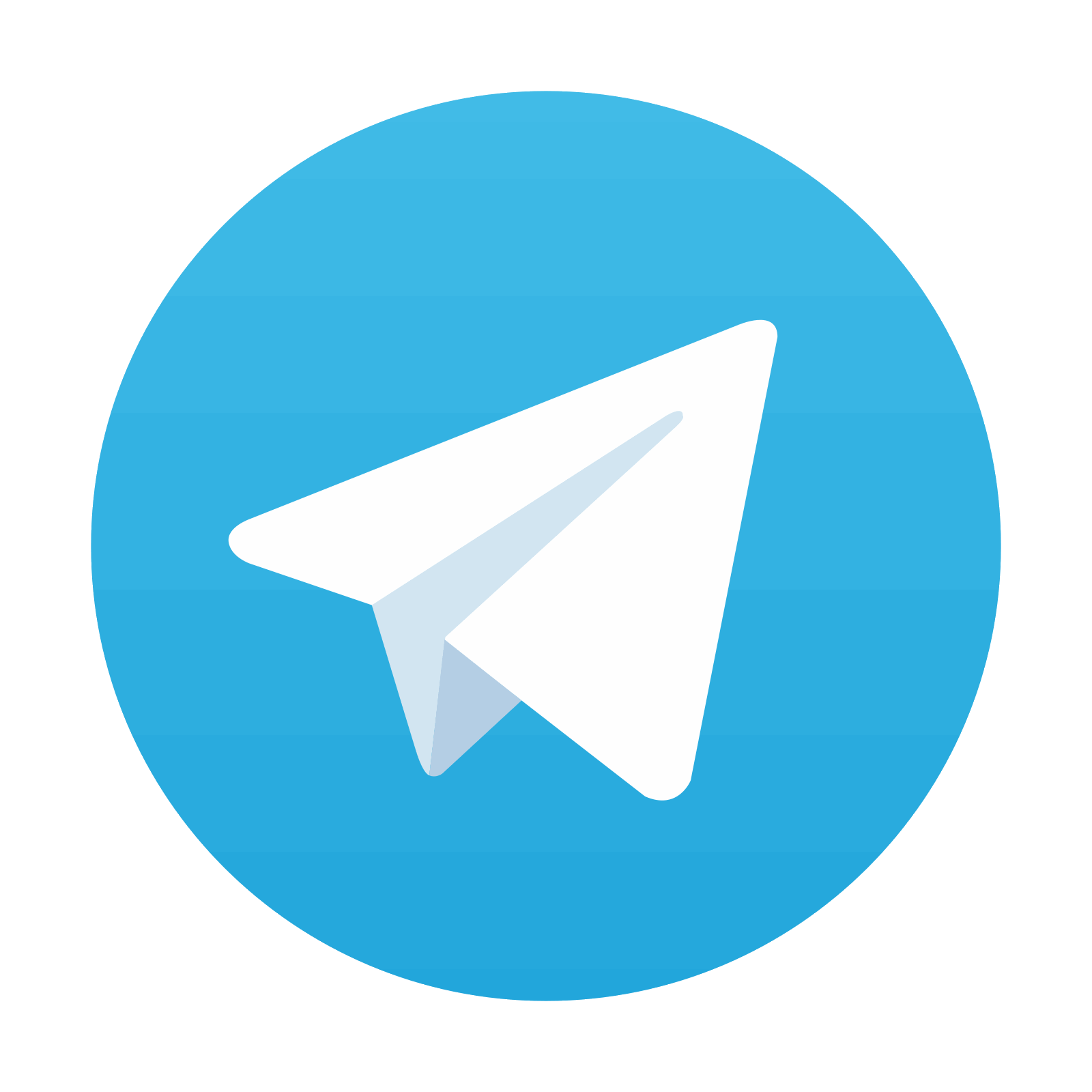
Stay updated, free articles. Join our Telegram channel

Full access? Get Clinical Tree
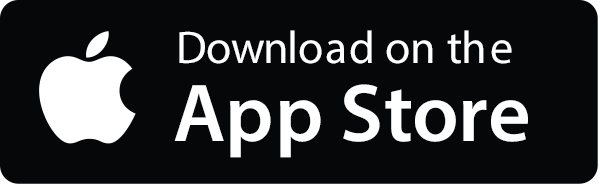
