Fig. 3.1
Natural endocast of the Taung child, an Australopithecus africanus fossil from South Africa. a Photograph of the original specimen. b Computed-tomographic (CT) scan of the original fossil. The frontal part of the endocast (red) was extracted virtually from the CT scan (see also Fig. 3.3)

Fig. 3.2
Segmentation of a virtual endocast from computed tomographic (CT) data. a CT scan of a modern human cranium visualized using the software package Avizo. b The air inside the endocranial cavity is filled on every CT slice. c Clipping the CT scan in the midsagittal plane reveals the virtual endocast (blue surface)
Automated segmentation, however, cannot be applied to the majority of fossil skulls, as they are typically (at least partially) filled with fossilized stone matrix. Figure 3.3 shows a CT scan of the facial fragment of the Taung skull (cf. Fig. 3.1). Unfortunately, the fossilized sediment that fills the endocranial cavity has almost the same density as the fossilized bone (Fig. 3.3b). In such cases the border between stone matrix and bone has to be drawn manually—anatomical expertise usually trumps automated algorithms, however manual segmentation for high-resolution CT data takes a lot of time.


Fig. 3.3
a CT scan of the Taung child’s facial fragment. b The endocranium is partially filled with fossilized sediment (white arrows). A clipping plane near the midsagittal plane shows that while it is possible to identify the bone, endocranial stone matrix and fossilized bone have very similar densities and therefore grey values. It was therefore impossible to extract the endocast automatically; instead the border between bone and matrix was traced manually on every CT slice (Gunz et al. 2009; Neubauer et al. 2012). c posterior view. CT Data from “digital @archive of fossil hominoids” (http://www.virtual-anthropology.com/3d_data/3d-archive)
Quantifying Size and Shape
Once the segmentation is complete, a skull’s endocranial capacity can be measured as the volume of the virtual endocast. Endocranial capacity is not the same as brain volume, as it comprises the brain as well as its surrounding tissues and the cerebrospinal fluid. Endocranial capacity, however, can be compared between fossils and extant species.
Figure 3.4 shows the virtual endocasts of a modern human (yellow) and a gibbon (blue). Brains and endocasts of gibbons and humans differ in shape as well as in size. These endocranial surfaces, however, also differ in position, and orientation because the crania were scanned on different CT scanners in different orientations. It follows that if you are interested in shape differences between a human and a gibbon endocast, you have to first standardize position and orientation and size. So as to compare these two endocasts, one therefore first aligns the two surfaces (Fig. 3.4b), and then scales them to the same size (Fig. 3.4c). Different methodological approaches rely on different registration algorithms as well as different methods of scaling—and these computational differences can have a major impact on the analysis and the interpretation of the results. Figure 3.5 illustrates how two different registration algorithms affect the visualization of shape differences. A human and a gibbon endocast were first scaled to the same size by dividing them by the cube roots of the respective endocranial volumes. The two surfaces were then aligned twice: once based on the cranial base (Fig. 3.5b), and once based on the entire endocranial surface (Fig. 3.5c). For the former registration all surface vertices were used, for the latter, the vertices on the cranial base were manually defined as a region of interest. Shape differences between the human and the gibbon endocasts were then plotted as color-coded distance maps; in blue areas the two surfaces are close to each other, in red areas they are far apart. These heat-maps were computed in the software package Avizo based on the distance between the surfaces. It is evident that the distance maps depend on the preceding registration step. There is nothing wrong, however, with the registration algorithms—both figures are technically correct. Ideally, the visualisation of shape differences should therefore not depend on a prior registration step. One option is to use thin-plate spline (TPS) deformation grids (Bookstein 1989, 1991) and surface morphs (Gunz et al. 2005) that are not dependent on any registration. This interpolation functions is central to a set of methods for the statistical analysis of shape called geometric morphometrics (GM). GM defines the shape of an object as the properties that are independent of its orientation, position, and scale. GM methods are based on landmarks—measurement points in either two or three dimensions that are homologous across all specimens of a sample (Bookstein 1991). A TPS deformation visualizes the shape difference between one reference form and one target form, based on a set of homologous coordinates measured on both forms. The space in-between the measured landmarks is interpolated by the TPS function “as smooth as possible” (Gunz and Mitteroecker 2013).



Fig. 3.4
How to compare two virtual endocasts? a Virtual endocasts of a human (yellow) and a gibbon (blue) in their original coordinate space. As they were scanned in two different orientations on different scanners, they are in different positions and orientations. b Standardizing for position and orientation by superimposing the centroids and aligning the endocasts along their principal axes. c Standardizing for scale, by dividing each endocast by the cube root of endocranial volume

Fig. 3.5
The impact of registration. Shape differences between the gibbon (a) and a human (b) endocast from Fig. 3.4c are visualized as a heat map of surface distance: Large differences between vertices are red; small differences are blue. I then used a popular surface registration algorithm (minimizing the root mean square) to align the two surfaces. In (c) the two surfaces were aligned based on the cranial base; in (d) based on the entire endocranial surface. The two registrations lead to very different heat maps. An alternative visualisation of the same shape difference is shown in Fig. 3.7
All GM methods require that all individuals be measured exactly the same, i.e. that all specimens have the same number of homologous landmarks in the same order. To convert the measured two- or three-dimensional landmark coordinates into shape variables, one has to remove information about position, orientation, and scale using a so-called “Procrustes superimposition” (Gower 1975; Rohlf and Slice 1990; Goodall 1991; Dryden and Mardia 1998). The Procrustes technique is named after a cruel figure in Greek mythology, who forced his victims to fit into his bed by either stretching them, or by cutting off their legs.
For each specimen a centroid is computed by averaging the respective landmark coordinates. This centroid is then subtracted from all landmarks; this standardizes the position as all centroids are moved to the coordinate origin. Dividing each specimen by its “centroid size”, a scalar computed as the square root of the summed squared distances between each landmark and the centroid, standardizes scale (Dryden and Mardia 1998). Finally, one iteratively optimizes the orientation of each specimen against the sample mean shape, by minimizing Procrustes distance—the square root of the summed squared distances between homologous landmarks (Rohlf and Slice 1990). Procrustes distance is a metric for shape difference between two specimens. Procrustes coordinates are shape variables that can be analysed using multivariate statistical methods.
Unfortunately, the number of landmarks that can be reliably identified on endocasts is very limited. Moreover, these anatomical landmarks do not capture the entire endocranial morphology as most of these landmarks lie on the cranial base. To address this problem several research teams have developed methods to quantify and compare entire endocranial surfaces (Table 3.1): “spherical meshes” (Specht et al. 2007), and “semilandmarks” (Neubauer et al. 2009). A related alternative method to these geometric morphometric approaches is called “spatiotemporal deformations” (Durrleman et al. 2011).
Table 3.1
Methods for comparing endocasts quantitatively
Method | Data input | Processing | Pros | Cons |
---|---|---|---|---|
“Sliding semilandmarks” (Neubauer et al. 2009) | For every specimen Surface 29 landmarks; 60 curve semilandmarks For a single template specimen 29 landmarks 60 curve semilandmarks 218 surface semilandmarks | 1. Map surface semilandmarks to each surface 2. Slide all semilandmarks to mean shape 3. Register based on all landmarks and semilandmarks 4. Scale to unit centroid size | Established geometric morphometric method—software available. Can be applied to partially complete specimens | Cannot handle more than approx. 2,000 semilandmarks Data collection is fairly time consuming |
“Spherical mesh” (Specht et al. 2007) | For every specimen Surface 7 landmarks Tessellation scheme 163,842 vertices | 1. Register surfaces based on 7 landmarks 2. Scale to same volume 3. Map surface to sphere 4. Remesh surfaces according to tessellation scheme | Fast automated processing of complete specimens | Entirely dependent on the 7 anatomical landmarks Application to partially complete specimens is not developed (yet) |
“Spatiotemporal deformations” (Durrleman et al. 2012) | For every specimen Surface | 1. Register surfaces 2. Measure correspondences between continuous surfaces | Fast automated processing of complete specimens Does not assume direct point correspondence among specimens | Dependent on initial registration step Unclear whether this can be extended to partially complete forms |
Specht et al. (2007) use a spherical mesh to distribute more than 150,000 points on the surface of every endocranium. On every endocast they measure the 3D coordinates of 7 homologous landmarks; these landmarks are then used to register the endocranial surfaces. All specimens are scaled by the cubic root of the respective endocranial volumes. The scaled and registered surfaces are then mapped to a sphere, and remeshed according to a pre-defined tessellation scheme. Whereas the number of vertices for each specimen is very large, the initial entire algorithm depends on the initial registration of the surfaces based on only 7 points.
In Neubauer et al. (2009) we presented an endocranial measurement protocol based on 29 endocranial landmarks and several hundred three-dimensional sliding semilandmarks (Gunz et al. 2005). Semilandmarks (Bookstein 1997) quantify curves and surfaces using corresponding 3D coordinates. After measuring anatomical landmarks, the same number of semilandmarks is placed equidistantly along curves. In a second step a regular mesh of surface semilandmarks is measured on a single template individual. This template mesh is then warped to every specimen in the dataset using a thin-plate spline interpolation function computed from the landmarks and curve semilandmarks. These warped surface points are then projected onto the actual endocranial surface of each specimen. This process ensures that every endocast is covered by the same number of landmarks and semilandmarks in roughly corresponding positions. In a final computational step the semilandmark positions are optimized with respect to the sample mean shape: all semilandmarks are allowed to slide along the respective curves and surfaces until the thin-plate spline bending energy between each specimen and the mean shape is minimal. This sliding step removes the influence of the arbitrary initial spacing of the semilandmarks and establishes a geometric homology. All technical details of the semilandmark algorithm are described in Bookstein (1997) for 2D curves and Gunz et al. (2005) for 3D curves and surfaces; for a review see also Gunz and Mitteroecker (2013).
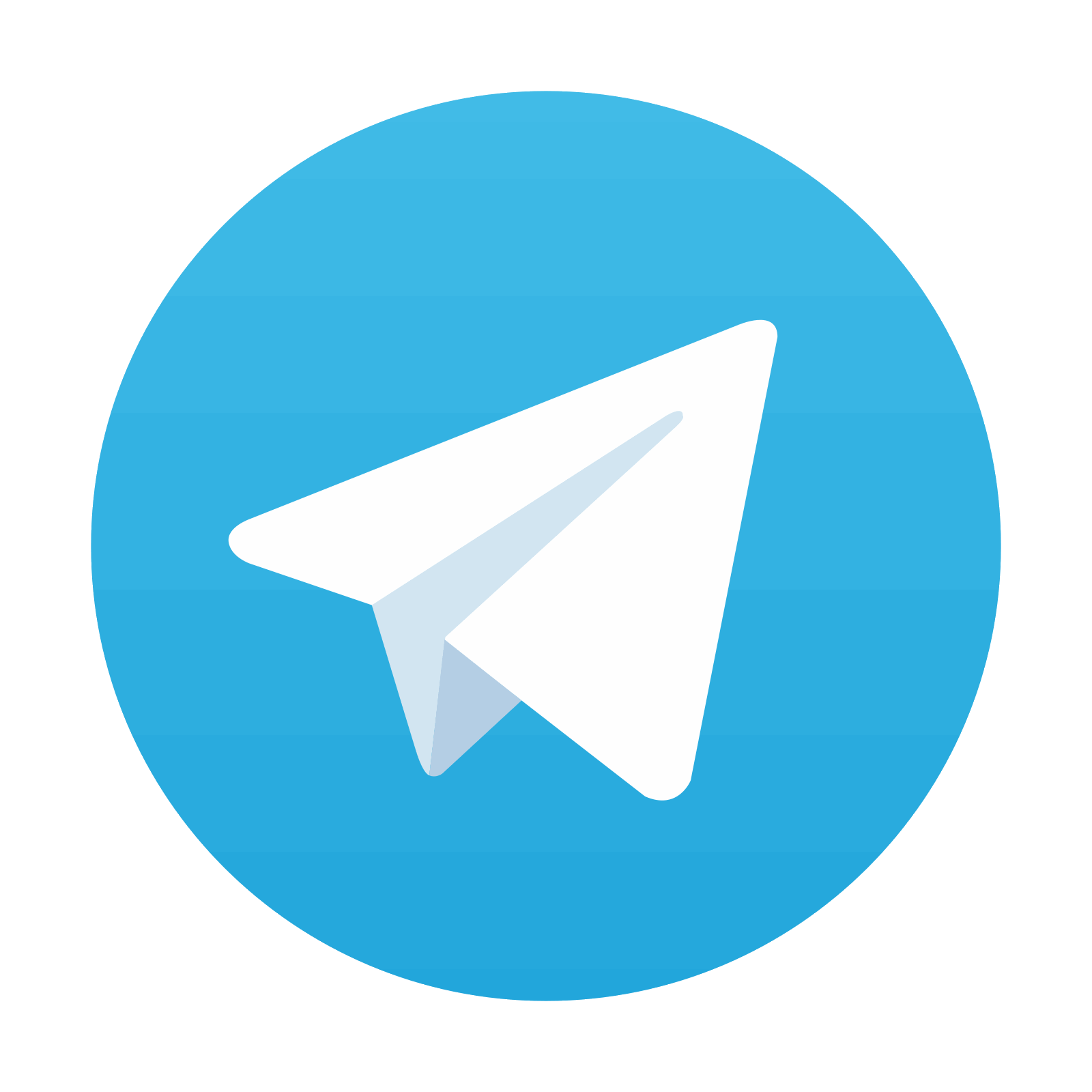
Stay updated, free articles. Join our Telegram channel

Full access? Get Clinical Tree
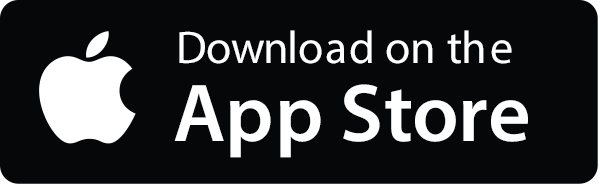
