The idea of surgical intelligence is finally coming to fruition. The use of both computer and robotic assistance, and increasing use of the two together, has already demonstrated the promise of surgical intelligence to transform spine surgery. Offering potential improvements in surgical accuracy, reductions in postoperative complications, and decreased revision surgeries, computer assistance provides the novel ability to base decision-making support on hundreds of thousands of prior cases. Poor measures of predictive power have been found for the surgeon’s ability to predict risk and benefit of surgery. The use of patient demographic and operative characteristics when creating complex analytical models allowed for the development of frailty scores that can better predict risks and benefits for a patient. Coupling such decision-making support for surgical uses, such as rod bending angles, with the precise ability of robotics to carry out the task, continues to improve patient outcomes. To date, the use of robotics in surgery has resulted in improved screw placements and improved navigation and reduced radiation dose, length of stay, and need for revision surgeries. However, as with many novel applications of technology, an array of challenges exists. These include reliance on single-centered retrospective data and limited data sharing, limited data for rare diseases and uncommon procedures, straight line trajectories of robotic movements, high costs of computer and robotic assistance (infeasible for small centers), high learning curves, a limited set of procedures for the use of such technologies (currently mainly used for screw placements), and legal issues regarding who is at fault in case of adverse events.
Recently, robotic uses for spine surgery are expanding from screw placements to include tissue-focused uses such as tumor resections, spurred by developments in haptic feedback systems, 3D printing, and mechanical advances. Further expansion of procedure types arose for microscopic-based surgeries with augmented reality support, visualizing tool orientation with respect to the anatomy of interest with no disruptions to the surgical view. Similar technological improvements, catalyzed by 5 G, gave rise to huge advances in telesurgical robotics, with the successful demonstration of the first 12 cases in 2020. With these advances, four dominant uses for computers and robotics in spine surgery have become apparent: telesurgical robotic surgery, shared-control robots with computer navigation, augmented reality systems, and machine learning decision-making support.
Telesurgical Robotic Surgery
While previously limited by bandwidth and speed of networks, the implementation of 5 G eliminates such concerns with its high speed, high bandwidth, and low latency. Demonstrations of telesurgical spine procedures, using a one-to-many workflow, open the door for the possibility of state-of-the-art surgeons being able to reach populations otherwise unable to present to the hospital. The workflow for the first 12 cases of successful spinal robotic telesurgery is presented below.
The telesurgery (demonstrated for pedicle screw implantation on patients with fracture, spondylolisthesis, or stenosis) involved a local operative team responsible for the peripheral tasks. First, the patients were anesthetized and the robotic system registered and positioned. A motorized C-arm took 3D images that were then transmitted to a control room (in a different city) over a 5 G system. The surgeon using the robotic software chose the entry point, screw orientation, and surgical path under navigational guidance. However, the K-wire and screw placement was done by surgeons on the patient side along with all image acquisitions, positioning of tools, equipment and patient preparation, and patient tracker installation. Furthermore, any bone resection and nerve decompressions would be done on the patient side. In this way, for full telesurgical uses, further innovations to technology, including advanced robotic movement capabilities, are necessary.
Necessary future innovations to telesurgery for spine surgeries include better control, increased dependence on the master surgeon, and expansion of procedure types. Haptic feedback presents the potential for improvements to master surgeon control of the robot by mimicking hand movements. Soft tissue surgery appears to be accomplishable by the current telesurgical systems, although data does not conclusively support this; soft tissue procedural safety, however, is supported by case reports using the da Vinci Surgical System.
Shared-Control Robots and Computer Navigation
Most of the current uses of robotics for spine surgery involve shared-control robots, including the Mazor X, ExcelsiusGPS, and ROSA One. Allowing both the surgeon and robot to control instruments and motions, these technologies improve placement, bending, and cutting. With the ability to position the robotic arms along pre-planned trajectories, the surgeon is able to preoperatively plan procedures, thereby reducing complex movements and decision-making during the operation for the surgeon. The five main steps involved in most shared-control procedures include the surgeon marking the trajectory on preoperative CT scans in the robotic system, a mounting frame being attached to the patient for image registration, fluoroscopic imaging being performed to facilitate synchronization with the preoperative imaging, robotic attachment to the mounting frame, and screw placement with guide wires.
Shared-control screw fixation surgical procedures have been shown to result in improved screw placement and navigation, and reduced radiation dose, length of stay, and need for revision surgeries. Furthermore, robotic use during surgery allows for data collection that does not require the surgeon to be involved. Such intraoperative data is already being collected and shared to develop better decision-making support for cage fitting and rod bending based on the patient outcomes of prior cases.
However, such workflows suffer from limitations. One such limitation is that minor patient movements during surgery can desynchronize the robotic path from the pre-planned trajectories; to address this, a reliance on intraoperative imaging is needed. Such reliance poses significant radiation concerns to both the patient and surgeon. Furthermore, the linear restrictions of the robot allow for limited paths with the inability to perform some tissue procedures. However, advances in current state-of-the-art shared-control robots include mechanical advantages such as movement stabilization (no-fly zones, restriction of movements based on pre-planning, tremor filtering) as well as significant reductions to radiation exposure. The latter is accomplished through the use of computer navigation.
With robotics relying on minimally invasive procedures to prevent excess tissue damage, surgeons lost the ability to visualize the key anatomical landmarks as was possible in open surgery. Thus, a reliance on intraoperative repetitive fluoroscopy was created. To eliminate this, computer navigation began to make use of advances in machine learning. Machine learning is the ability for the computer to make predictions based on its knowledge of past data. In the lens of spine surgery, with the input of a camera-captured (no radiation) image of a patient’s spine, the desired outcome may be to locate a specific landmark and synchronize it to a similarly located landmark in the preoperative CT image. To predict this desired outcome, a computer may be trained by repeated exposure to past cases where the desired output is known. Thus, with every case, a computer may learn how to predict better. Using such technology, advances in computer navigation have reduced radiation exposure by 80%; this method takes low-quality, low-dose intraoperative imaging and predicts how best to construct a higher quality image from this. Similar computer vision advances have used non-radiation-based photography to capture patient landmark features and predict synchronization to preoperative imaging, thereby addressing patient movement desynchronization during the course of surgery. While further research is required to develop synchronization and navigation based on optic cameras, such technology suggests a radiation-free intraoperative future workflow and thus illustrates the benefits computer navigation brings to shared-control and minimally invasive surgery.
Augmented Reality Systems
All the above procedures involve intraoperative imaging being displayed on a screen. This requires continuous disruption of the surgeon’s workflow to match the orientation of their surgical equipment and tools with the anatomy displayed on the nearby screen. Such separation in the display increases surgical time, decreases the surgeon’s understanding of the orientation of tools to anatomy, and requires more radiation exposure for continued intraoperative imaging. Augmented reality systems such as XVision, which is already cleared by the FDA, can superimpose the position of surgical tools on the CT imaging in real time and display the resulting augmented reality on a surgical headpiece that is worn by the surgeon. A similar use of augmented reality involves projecting the pre-planned surgical path onto the patient in a surgical headpiece to guide navigation better. Furthermore, segmentations of vertebrae have been combined with augmented reality visualizations of screw orientations to guide placement accuracy. By superimposing O-arm imaging with the live view from the microscope, superimposed videos can guide surgeons during the course of tumor surgeries and degenerative spine disease procedures.
Augmented reality uses are not limited to screw planning. Augmented reality can be used to project necessary anatomical landmarks into a surgeon’s microscopic view during minimally invasive anterior cervical discectomy and fusion, posterior cervical laminotomy, and foraminotomy. Furthermore, augmented reality display of resection planes has been used in combination with microscopic eyepieces during osteotomy for deformity.
Leveraging augmented reality systems for spine surgery may also serve as an educational tool. Allowing complex surgical procedures to be recreated in augmented reality environments can aid in the training of surgeons. Advanced future uses could expand such recreation to include simulation of a pre-planned technique and allow the surgeon to revise their plan based on the patient’s anatomy and visualization with the augmented reality system before the surgery itself.
Machine Learning and Decision-Making Support
We have already discussed the applications of machine learning for spine surgery including lower-dose imaging, anatomic landmark synchronization to preoperative imaging during patient movements, cage selection, and rod bending. All these technologies rely on a shared infrastructure: they take input information relevant to the patient (demographic variables, operative characteristics, preoperative imaging, and intraoperative imaging) and output a prediction (best cage shape, best rod angle, simulated high-dose imaging, anatomical orientation and synchronization). This infrastructure is built upon each machine learning-driven system being trained on thousands of cases from past surgeries.
However, the use of machine learning in spine surgery extends to include the conversion of imaging information (MRI to CT), algorithmic decision-making support for path planning, and risk prediction. Systems have already been developed that help with trajectory planning, avoiding at-risk tissue, and aiding the surgeon in obstacle avoidance during complex navigation. However, it is risk prediction that poses an immediate potential benefit to the current operating workflows. Using the available demographic and operative characteristics (including comorbidity status, procedural history, preoperative imaging, and operative plan), it is difficult for the surgeon to predict, based on their medical experience, the success of the planned surgery and risk of complication to the patient. With high-risk and high-cost procedures, high predictive capabilities in this domain hold incredible value. Machine learning allows for increased predictive power by learning from similar patients’ outcomes. A surgeon is unable to compare the current patient to hundreds of thousands of others and consider how similar the patient is; however, the current algorithms allow for such techniques. Recent systems aimed to support surgical decision-making, rather than simply being a black box to output a prediction, resulted in the surgeon seeing the 5 or 10 past cases most similar to the current patient along with their respective outcomes. Such similarity determination is based on complex information including personality type and genetic information in the most recent work. Such algorithms to be used for risk prediction have been instrumental in identifying risk factors. One such study found risk factors for sustained postoperative opioid use by leveraging a machine learning workflow.
To train better and more predictive machine learning algorithms requires high amounts of data. This poses a problem in rare and more specialized cases; however, an increasing effort is resulting in better methods and availability of such data. Robotics allows continuous data collection without surgeon assistance being necessary. Companies have developed data sharing technology to be coupled with robotic systems for the simulation of surgical plans.
Conclusion
Computer and robotic technologies are presenting clinically significant improvements to surgery, allowing them to make their way into state-of-the-art practice. However, there exists a need for formal cost-benefit analysis of the integration of these spine robotic systems into practice. There are potential high costs associated with the technology itself, high setup time, training curve, and increased surgical times as users are not familiar with the system; these may be offset by improved outcomes leading to decreased revision surgeries, readmission, and length of stay.
Further remaining to be studied is the potential for dissimilar distributions used for machine learning training. As data is scarce for many spine surgery tasks, such data may be taken from only one clinical center. For example, a machine learning system to segment the vertebrae may use the training data of CT scans from only one hospital. The CT scans of other hospitals may not closely resemble those from the first hospital, either in their distribution of anatomical abnormalities or even their imaging format. Such distribution shifts may pose a threat to the successful application of machine learning systems across varied users in spine surgery. As the field progresses, it will become necessary to use data for training that is obtained across a multitude of centers, capturing a wide range of types of conditions, imaging, and formats. We may soon begin to see the possibility for semi-, and even full, automation of surgical subroutines by robotics. As decision-making shifts toward increased reliance on the robotic and computer system predictions, standardized and regularized development of the deployment of such algorithms to patient care is imperative.
Computers and robotics offer, and have already partially achieved, a revolution in geographical reach, surgical precision, decision-making support, and decreased radiation in spine surgery.
References
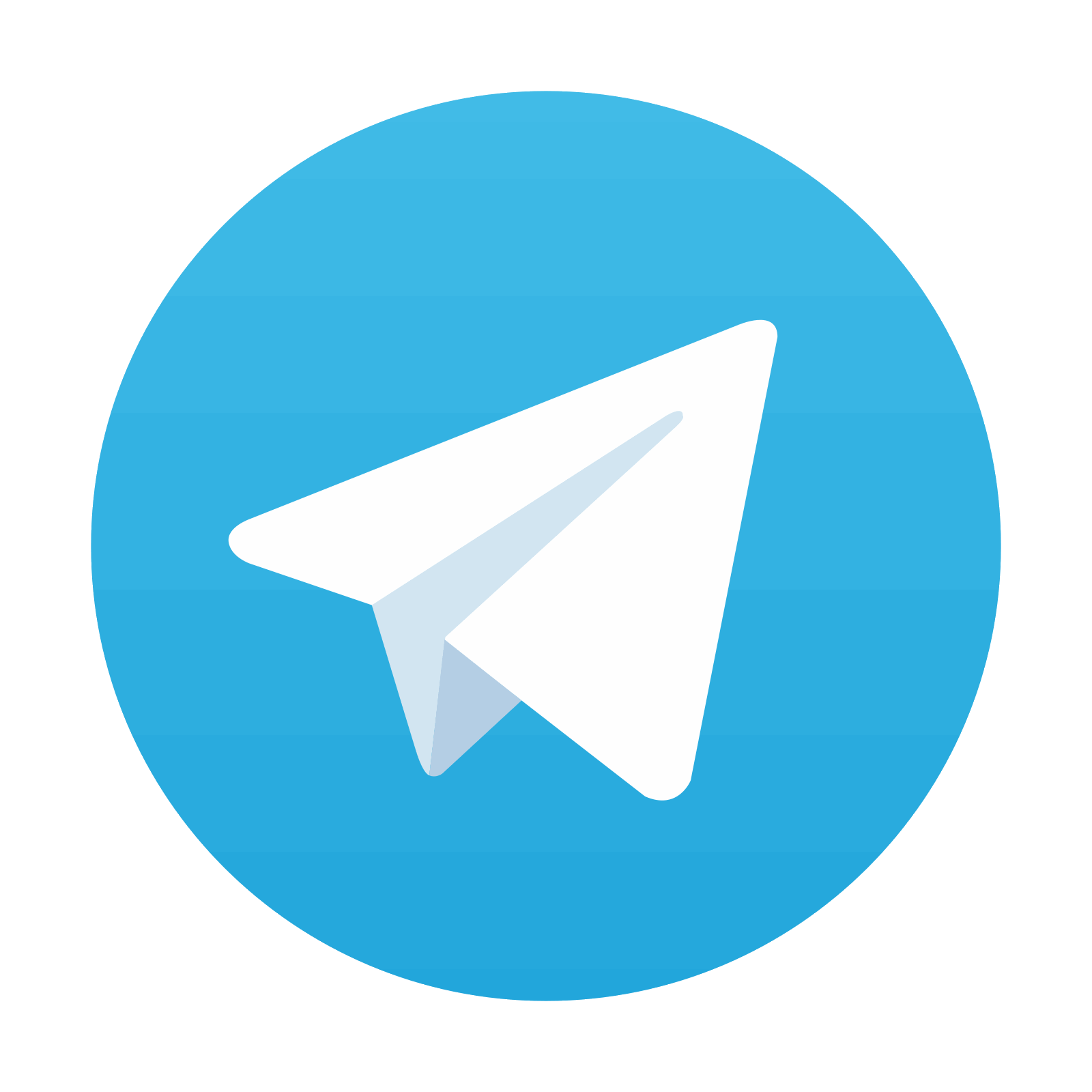
Stay updated, free articles. Join our Telegram channel

Full access? Get Clinical Tree
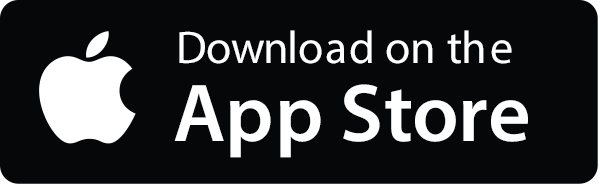

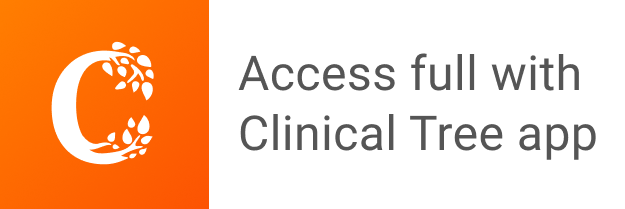