Resolving Controversy and Uncertainty with Evidence in Neurovascular Medicine
Objectives: After reading this chapter, the reader should be able to describe the types of clinical studies (case series, case-control studies, cohort studies, and randomized trials) and judge the quality of data derived from those studies.
Accreditation: The AANS* is accredited by the Accreditation Council for Continuing Medical Education (ACCME) to sponsor continuing medical education for physicians.
Credit: The AANS designates this educational activity for a maximum of 15 credits in Category 1 credit toward the AMA Physician’s Recognition Award. Each physician should claim only those hours of credit that he/she spent in the educational activity.
The Home Study Examination is online on the AANS Web site at: http://www.aans.org/education/books/controversy.asp
* The acronym AANS refers to both the American Association of Neurological Surgeons and the American Association of Neurosurgeons.
If every clinical decision was supported by solid, irrefutable evidence, there would be little controversy in clinical practice. Appropriate treatment decisions would be obvious. In reality, treatment decisions are based on imperfect evidence. Physicians receive bits of data from a variety of sources, including study results, expert opinion, and experience. These data must be combined and applied to a specific clinical situation.1
Ideally, every decision should be based on the highest standard of evidence available—the results of a randomized trial. However, very few informative randomized trials are undertaken. They are expensive and may be impractical, with delayed results.2 Further, the results of a randomized trial apply to the average patient enrolled in the study; they may not apply readily to the particular patient for whom a treatment decision is being made.3 In the foreseeable future, it still will not be possible to base clinical decisions entirely on the results of randomized trials. Therefore, it is important to understand the strengths and limitations of other sources of evidence.
Entirely rational clinical decision-making is not achievable. Subjectivity is unavoidable. Among other influences, our instinct for self-preservation is too powerful and important. For example, the decision to delay endovascular repair of a ruptured aneurysm may be influenced by a child’s Saturday morning birthday party. Personal financial gain also influences physician behavior.4 Some degree of physician self-interest is natural and essential. Few would join or survive the practice of medicine if every decision made were entirely altruistic. Our current monkish lifestyle would become intolerable. Nonetheless, it is essential to examine personal motivations in clinical decision-making and to view our interpretation of evidence with suspicion, always on the lookout for our own biases.5
In this chapter, I provide a framework for interpreting and generating evidence, with the goal of focusing attention on key sources of bias. Such a framework may be useful in identifying the boundaries between objective evidence and subjective bias to improve the quality of clinical decision-making.
Levels of Evidence
Clinical decisions generally involve synthesizing data from a variety of sources. Quality of the data source should influence the weight of the data in the final decision.1,6 Quality is dependent on the study type—case series, case-control study, cohort study, or randomized trial—and on the details of its execution. Standard methods for ranking quality of data have been proposed and are often used in developing clinical guidelines (Table 26-1).7,8 These rankings provide a rough template for assessing evidence.
Physician Experience and Opinion
The experience of the treating physician may be the most influential evidence used in making clinical decisions. Others may rely on the experience and opinions of experts to guide decisions. Textbooks frequently contain phrases such as “our experience has shown…” and “It is quite clear from our experience….”
Generally, this is the least reliable level of evidence. Any individual’s observations are subject to biases, regardless of years of experience and prominence in the field. These biases may be completely unrecognized by the individual.
Experience and opinion may be the only source of data. In this instance, developing consensus based on opinions of practitioners from various backgrounds may reduce the influence of bias. The loudest voice may have the greatest influence, so it is important to ensure that the opinions of a dominating personality are not weighted disproportionately. The RAND Corporation (Santa Monica, CA) has developed standardized methods for combining the experience and opinions of multiple practitioners.9 The method incorporates expert opinions with respect to specific, well-defined cohorts of patients.
Table 26-1 Levels of Evidence and Grading of Recommendations9A,9B
Level of Evidence | |
Level I |
Data from randomized trials with low false-positive (α) and low false-negative (β) errors |
Level II |
Data from randomized trials with high false-positive (α) or high false-negative (β) errors |
Level III |
Data from nonrandomized concurrent cohort studies |
Level IV |
Data from nonrandomized cohort studies using historical controls; data from case-control studies |
Level V |
Data from anecdotal case series |
Strength of Recommendation | |
Grade A |
Supported by Level I evidence |
Grade B |
Supported by Level II evidence |
Grade C |
Supported by Level III, IV, or V evidence |
Case Series
The next level of evidence is the description of a series of cases. A case series may produce more reliable evidence than opinion because the details of the experience are explicitly described. The opinion drawn from the cases can be questioned because its source can be examined by the reader.
Case series are particularly susceptible to bias, and details of study design must be considered carefully. Case selection is one major source of bias; designs that establish systematic methods of inclusion reduce this risk. For example, a study may describe five patients with unruptured aneurysms who died after coil embolization, and this may influence physicians by suggesting that endovascular treatment is unsafe. However, these five patients may have part of a series of 500 patients. Then the selection of only those cases that died has produced a misleading impression of the safety of coil embolization.
Choosing a consecutive series of cases may reduce the risk of bias in case selection. However, a bias can still be introduced if the beginning or ending of the collection period is influenced by outcome. For example, the occurrence of a death with coil embolization could trigger the initiation of a prospective study period, and the occurrence of five deaths among 15 treated patients could trigger preparation of a manuscript and publication. However, these 15 patients may not be representative of the entire experience at the center. Similarly, describing the initial experience with a technique and comparing it to prior results with a different technique may also be misleading because expertise will not be developed with the new procedure.
Practitioners with greater experience and prominence often publish case series. Further, those with poor results of treatment are less likely “to air the dirty laundry.” Therefore, case series tend to be biased toward underreporting of adverse events from treatment. This form of publication bias is not unique to case series and may influence reporting of all study designs.
Case series often do not include a comparison group. Centers with expertise in surgical clipping report a series of surgically treated unruptured aneurysms. Centers with experience in endovascular surgery report results for coil embolization. Comparing therapies described in different case series is problematic. First, outcome measures are variable and their assessment is influenced by the method of ascertainment, as discussed below. A poor outcome may be defined as death or vegetative state in one study, and as inability to return to work in another, and these results are not readily comparable. Second, the patients selected for treatment in different case series may not be comparable. For example, a surgical series may be derived from a referral population with a disproportionate number of giant aneurysms, and an endovascular series may be more representative of the community. Comparing these series could produce the misleading impression that differences in outcome are because of the greater safety of coil embolization.
Case series are a mainstay of the surgical and interventional literature. They are required to recognize priorities for further study but are seldom useful for establishing standards of care.
Case-Control Studies
Case-control studies are rarely used to evaluate treatment. By definition, a case-control study first classifies subjects by the outcome—such as dead/not dead—and compares all other characteristics in the two outcome groups. The design is useful when the outcome is rare and when it is difficult to define the study group prior to treatment. For example, if an investigator were interested in the risk factors for rerupture after coil embolization and had not been keeping track of details of all treated patients, a case-control study could be useful. A collection of 20 cases with rerupture could be compared with a random sample of 20 patients who had been treated but did not rerupture. A difference in aneurysm neck size in the two groups could implicate this characteristic as a risk factor for rerupture. The case-control design is more efficient than collecting neck size for all treated patients (which would be a cohort study): there would be fewer films to review with only marginal loss of statistical power. However, the case-control design is susceptible to biases if controls are not representative of the population from which the cases arose. For example, smoking may appear to be a risk factor for rerupture, but this could be due to a greater likelihood of refusal to participate in a control group with smokers, a phenomenon that is well-recognized. Difficulty in identifying an appropriate control group severely limits the utility of the case-control design.
Cohort Studies
Cohort studies are simply systematic case series. All patients are studied who meet specified entry criteria. Contrary to case series, cohort studies are always fully inclusive of all qualifying patients treated over a given time period. Contrary to case-control studies, subjects are identified by characteristics at the time of or prior to treatment rather than by the outcome, and outcomes are compared between groups treated in different ways. Though it is possible to study only a single therapy in a cohort study, the method is frequently used to compare therapies. The cohort study is a great improvement over case series when comparing therapies because it guarantees that outcomes are measured on the same scale and, preferably, by the same investigators.
The inclusion criteria of a cohort study must be explicit and should be established before outcomes are tallied. If the outcome influences the decision to include a case, bias will result. For example, if we are comparing surgery and endovascular therapy of unruptured aneurysms, and in preliminary analyses, we discover that new disability occurred in 5 of 10 middle cerebral artery (MCA) aneurysms treated by coil embolization, we would introduce a bias if we redefined entry criteria to restrict the cohort to aneurysms in other locations. It would be reasonable to report that outcomes were different with MCA aneurysms, but it would be misleading to omit them from the analysis after noting the outcomes. Establishing inclusion criteria prior to tallying outcomes prevents this form of bias. If we are convinced that MCA aneurysms should be treated with surgery, we can make the decision to omit this subgroup a priori, but we cannot alter our initial hypothesis and entry criteria once the outcomes have been evaluated.
The validity of cohort studies is strengthened by their standardized and explicit entry criteria established a priori. Further, the entry criteria can be used to define a group that could have received either therapy. This is essential because the appropriate treatment decision is known for those who could only receive one of the two therapies being compared, and these patients would not be included in a randomized trial. Including results of patients who could only receive one of the therapies could result in bias because their outcomes may be entirely different from those who could receive either therapy—the group in whom treatment decisions are made. Systematic inclusion criteria can be crafted to reduce the likelihood of including those who are not candidates for both procedures.10 For example, it might be reasonable to exclude cavernous carotid aneurysms from a study of unruptured aneurysm treatment because many would not be appropriate candidates for surgical clipping. When indications for treatment are unclear, physicians can be asked to determine whether a case could receive both treatments; blinding the reviewing physicians to actual treatment assignment and outcome further reduces the potential for bias when selecting cases that are candidates for both procedures.11
Applying identical entry criteria to both treatments does not ensure that there are no systematic differences in the risk profiles of the treatment groups. For example, a hospital may triage older patients to endovascular therapy because of the perception that the elderly do poorly with surgery. Unless the cohort is limited to patients in a certain age group, there will be an imbalance in age in the treatment groups, and this may result in poorer outcomes in the endovascular group. Evaluating and reporting other risk factors for the outcome of interest is essential in any cohort study. In this way, systematic differences in known risk factors can be identified.
Identifying systematic differences in risk profile between treatment groups does not invalidate a study. Multivariable analysis is one tool for reducing the potential for imbalances in risk that influence the relative outcomes of the compared treatments. Logistic regression is the standard form of multivariable analysis used to evaluate binary outcomes (such as dead/alive), and linear regression is used for continuous outcomes (such as infarct volume). By adjusting for other risk factors, multivariable analysis isolates the independent effect of treatment on outcome. However, multivariable analysis only adjusts for other risk factors that are measured. If there are unmeasured factors that may be different in the treatment groups, a difference in outcome could still be due to risk profile rather than actual treatment. For example, the configuration of certain aneurysms may result in more-difficult procedures, and we may not be able to capture this in a standard multivariable analysis because configuration is too poorly defined to measure. One solution is to have physicians review the films and judge the difficulty in treatment, reducing the potential for bias by blinding to actual treatment selection and outcome.11 Then, normally immeasurable factors can be incorporated into multivariable models. Such an analysis prevents one group from later blaming poorer outcomes on a patient-risk profile. However, it is always possible that a risk factor of either procedure, unknown to practitioner, is unbalanced between the treatment groups. This is the greatest inherent limitation of cohort studies and of all other studies in which treatment assignment is not random.
Randomized Trials
Randomized trials generally produce the most reliable evidence. Because only the randomization determines which treatment is received, all other risk factors are generally balanced between the treatment groups as long as the study sample size is adequate.12 Even unmeasured factors should be distributed evenly between treatments.
Double blinding is the ideal in randomized trials. By blinding the patient to treatment assignment, one reduces the likelihood that the patients’ preconceived notions of treatment will bias their responses when outcomes are assessed. This is important because patients tend to favor newer and more technical therapies and receive psychological benefit from these.13 Blinding the physician or the assessor of outcome reduces the likelihood that subject biases will influence assessment of outcomes. Though blinding is straightforward for most medical therapies, which can be compared with placebo, it is much more difficult with invasive therapies. Sham surgeries are used, but some argue that they are unethical because an unnecessary risk and discomfort are undertaken. An alternative approach is to blind the assessor of outcome to treatment assignment and to choose an outcome measure that is as objective as possible. Such an approach is common in modern trials of neurosurgical interventions.14
Randomized trials are difficult to perform.2 They require extensive planning and substantial resources, and results are often delayed. Some clinical questions cannot be ethically studied with randomized trials. For these reasons, most clinical decisions are made without the benefit of randomized trials. The results of randomized trials should always be considered in making clinical decisions, but other sources of evidence are essential.
Elements of Study Design
Prospective versus Retrospective
Studies are defined as prospective if patients are identified before the outcome has occurred and are retrospective otherwise.15 Case-control studies are always retrospective because outcome defines the groups being compared. Randomized trials are always prospective because inclusion and randomization occur before the outcome. Case series and cohort studies can be either prospective or retrospective.
Prospective studies have several advantages. First, it is not possible for outcome to influence inclusion of a case because outcomes are unknown when the study begins. Second, baseline factors can be assessed more completely to evaluate changes with therapy and to ensure a more complete balance. For example, a prospective study may allow measurement of cognitive function prior to treatment so that change with therapy can be assessed. Third, some pretreatment variables may be inaccurately or incompletely recalled, and these factors can be measured before treatment in a prospective study. For these reasons, prospective studies are often more likely to produce valid results.
However, prospective studies are inefficient. Often results are delayed for years while an adequate number of cases are generated. The results of a retrospective study are generated as rapidly as an analysis is performed. This is a tremendous advantage, but only if the potential pitfalls of retrospective studies are overcome by mimicking the design of prospective studies. First, to reduce the likelihood of outcome influencing case inclusion, strict entry criteria should be established before beginning the analysis, as discussed above. Second, the outcome chosen should be robust without a measure of pretreatment condition. For example, a retrospective study of unruptured aneurysm treatment with a cognitive test score as an outcome may be acceptable because patients are often unimpaired prior to treatment, but a similar outcome measure in a study of subarachnoid hemorrhage would be much more problematic in a retrospective study. Third, retrospective studies should only be undertaken if the measurable pretreatment risk factors are expected to allow for adequate adjustment of prognostic differences between treatment groups. Although we may have a Hunt and Hess score in a retrospective study of subarachnoid hemorrhage, this measure could not be expected to capture important differences in pretreatment prognosis in a study of subarachnoid hemorrhage treatment. In this instance, even a prospective study may not allow complete adjustment for important differences in prognosis. Compared with prospective studies, retrospective studies are clearly more efficient and, if performed carefully, are not necessarily more susceptible to bias.
Size and Power
Study size is often determined by convenience—the number of available cases. This strategy does not produce bias in a retrospective study because the size is fixed. In prospective studies, bias is produced if the results are evaluated at intervals and the study is stopped when a significant effect is seen. This strategy is more likely to produce significant results when the treatments are the same.
Study size determines the power to find a significant effect if one is truly present. Studies that are too small may fail to find clinically important differences in outcomes but should not be interpreted as showing no difference. A recent small, randomized trial of clipping versus coiling of ruptured aneurysms included 109 patients.16 Severe disability or worse occurred in 25% of surgical cases and 21% of endovascular cases, and the difference was not significant. However, the 95% confidence intervals were consistent with a threefold lower risk with coil embolization or a twofold lower risk with surgery, which demonstrates the limitations of study power. The International Subarachnoid Aneurysm Trial (ISAT) will include 2500 patients to answer the same question and was designed to detect a 25% difference in outcome between the two therapies.17
Necessary sample size and power can be calculated from tables, equations, or software programs (such as Epi-Info, which is available without charge from the Centers for Disease Control and Prevention Web site: www.cdc.gov). All prospective studies should include some calculation and consideration of power if the goal is to generate significant results when a true, clinically important difference is present. Further, all studies should acknowledge the limitations in the precision of treatment effect estimates. This can be done most readily by reporting the 95% confidence intervals.
Measuring Outcomes
Choosing the outcomes of a study requires careful consideration. The measure used by colleagues in recent publications may not be the ideal measure. Before beginning a study, it is preferable to define a single primary outcome measure for the main study hypothesis. Any number of secondary outcome measures can be evaluated, but committing to a single primary measure prevents bias produced by “cherry-picking” the outcome that happens to be significant. For example, if the Glasgow Outcome Scale,18 Barthel Index,19 and Rankin Scale20 are measured as outcomes but only the Rankin Scale shows a significant effect, an investigator is likely to focus on the Rankin Scale results to stress the importance of the findings. However, it is more likely that a single outcome will be positive if more outcomes are measured. Therefore, a study that measures multiple outcomes and does not commit to a primary outcome measure is more likely to find an effect even when none exists. If the Glasgow Outcome Scale results were defined a priori as the primary outcome measure, the study would be negative, though other results from secondary outcome measures could suggest that a smaller or more specific effect was present. If a primary outcome measure is not chosen a priori, all the study results should be examined carefully to confirm that findings are consistent in all the outcomes measured.
Outcome measures should be reproducible, valid, measure clinically meaningful results, and allow comparison of results between studies. The simplest way to define such an outcome measure is to utilize an existing scale. Many investigators bow to the temptation to create a new scale—such as excellent, fair, poor outcome—but this creates several problems. First, such a scale is not generalizable. What one physician calls an excellent outcome, another may define as unacceptable. This makes it impossible to compare results of one study with another. Second, vague definition of the outcome states encourages bias. It increases the likelihood that classification will be subjective with the potential to underrepresent the true impact of therapeutic complications. Third, homemade scales do not necessarily measure outcomes that are important to patients or to the health care system in general. For example, an excellent outcome may be defined as anything better than a vegetative state. However, patients may have significant and important disabilities that would not be captured in this scale. Fourth, a nonstandard scale may be difficult to reproduce over time or between investigators, introducing random misclassification that reduces the likelihood of finding an effect when one is present. If two patients with identical outcomes are classified differently because the scale definitions are vague, this introduces random variation that makes it harder to find a true effect.
Mortality is perhaps the cleanest outcome measure. There is little argument about whether an event has occurred or whether the outcome is important. However, mortality may be rare, which results in reduced power. Important differences in outcomes may be ignored if mortality is chosen as the primary outcome measure. For example, two therapies may have identical mortality rates but disability may be quite different. The difference in the therapies would be missed if only mortality were measured.
Scales with additional gradations increase the likelihood of finding a significant effect if one is present and may more accurately reflect the health impact of the therapies being studied. There are many standard, validated, and reproducible outcome measures available. These scales can measure functional states (such as the Barthel Index, Rankin Scale, Glasgow Outcome Scale, cognitive scales21), quality of life (such as the Sickness Impact Profile22), or health perceptions (such as the SF-3623).
Choosing between these and other scales can be difficult. From the perspective of statistical power, the best outcome measure is one that spreads out results evenly. For a binary outcome, this means that 50% of patients are in each category. The Glasgow Outcome Scale and Barthel Index do not measure minor deficits well. They are weighted toward differentiating very poor functional states. Therefore, in a study in which poor functional states are uncommon, such as a study of treatments for unruptured aneurysms, only a few patients would be expected to show impairment in these scales, and power would be low relative to a scale that included cognitive impairment. This was illustrated in the International Study of Unruptured Intracranial Aneurysms in which the inclusion of cognitive impairment nearly doubled the number of patients classified as disabled.24 If surgery and endovascular therapy were compared in this study, including cognitive impairment would increase the likelihood that a significant difference would be detected if one were truly present.
It is important to standardize a chosen outcome scale’s or scales’ measure to reduce the potential for bias. First, it is best to define a specific time point for assessment of the outcomes. In this way, differences in the timing of the evaluation between groups will not influence the results. For example, if an outcome is measured at hospital discharge and length of stay is longer for one therapy than another, one group will have longer to recover from treatment, potentially introducing a bias. Determining the timing of measurement requires careful consideration. The outcome should be measured after recovery is largely complete if differences in lifelong outcomes are expected but could be measured sooner if the rate of recovery is important in distinguishing therapies. Further, if patients have other medical problems, a delayed measure of outcome could introduce additional random variation or bias due to complications from other diseases unrelated to the therapies studied.
Second, who will be measuring the outcome must be carefully considered. The assessor can affect the sensitivity and reproducibility of the outcome measure. For example, studies have shown that neurologists detect complications much more frequently after carotid endarterectomy than neurosurgeons.25 In case series, this may affect the perceived risks of treatment. The assessor can also introduce his or her own biases into the assessment. For example, it is not realistic to expect a neurointerventional radiologist to evaluate outcomes of surgery in a study comparing clipping to coil embolization unless he is blinded to the actual treatment received. Even the best intentions and the highest integrity are challenged by subtle biases to favor one’s own practice. Therefore, it is best to obtain outcomes blinded to the actual treatment received. If this is not possible, other alternatives include choosing an assessor with no clear bias, such as a study nurse, or choosing a team of assessors with equal representation from both the procedures being compared.
Conclusion
Medical knowledge is constantly evolving. Physicians are responsible for integrating information of varying quality from multiple sources with varying applicability to a given clinical situation. The task is impossible to perform without error. Understanding and addressing biases in our own integration of evidence is important in making the most rational clinical decisions and in improving the quality of the evidence. There is a natural tendency to defend one’s own current practice, and this may influence the acceptance of new evidence and performance of studies. Recognizing potential sources of bias in clinical studies is important in weighing the evidence and improving it.
References
1. Guyatt GH, Haynes RB, Jaeschke RZ, et al. Users’ Guides to the Medical Literature: XXV. Evidence-based medicine: principles for applying the Users’ Guides to patient care. Evidence-Based Medicine Working Group. JAMA 2000;284(10):1290–1296
2. Feinstein AR. Current problems and future challenges in randomized clinical trials. Circulation 1984;70(5):767–774
3. Dans AL, Dans LF, Guyatt GH, Richardson S. Users’ guides to the medical literature: XIV. How to decide on the applicability of clinical trial results to your patient. Evidence-Based Medicine Working Group. JAMA 1998;279(7):545–549
4. Swedlow A, Johnson G, Smithline N, Milstein A. Increased costs and rates of use in the California workers’ compensation system as a result of self-referral by physicians. N Engl J Med 1992;327(21):1502–1506
5. Cabana MD, Rand CS, Powe NR, et al. Why don’t physicians follow clinical practice guidelines? A framework for improvement. JAMA 1999;282(15):1458–1465
6. Guyatt GH, Sinclair J, Cook DJ, Glasziou P. Users’ guides to the medical literature: XVI. How to use a treatment recommendation. Evidence- Based Medicine Working Group and the Cochrane Applicability Methods Working Group. JAMA 1999;281(19):1836–1843
7. Wilson MC, Hayward RS, Tunis SR, Bass EB, Guyatt G. Users’ guides to the Medical Literature. VIII. How to use clinical practice guidelines. B. what are the recommendations and will they help you in caring for your patients? The Evidence-Based Medicine Working Group. JAMA 1995;274(20):1630–1632
8. Adams HP Jr, Brott TG, Crowell RM, et al. Guidelines for the management of patients with acute ischemic stroke. A statement for healthcare professionals from a special writing group of the Stroke Council, American Heart Association. Stroke 1994; 25(9):1901–1914
9. Normand SL, McNeil BJ, Peterson LE, Palmer RH. Eliciting expert opinion using the Delphi technique: identifying performance indicators for cardiovascular disease. Int J Qual Health Care 1998;10(3):247–260
9A. Cook DJ, Guyatt GH, Laupacis A, et al. Rules of evidence and Clinical recommendations on the use of antithrombotic agents. Chest 1992; 102(suppl 4):S305–S311.
9B. Mayberg MR, Batjer HH, Dacey R, et al. Guidelines for the management of aneurysmal subarachnoid hemorrhage: a statement for healthcare professionals from a special writing group of the stroke council, American Heart Association Stroke 1994;25:2315–2328.
10. Horwitz RI, Viscoli CM, Clemens JD, Sadock RT. Developing improved observational methods for evaluating therapeutic effectiveness. Am J Med 1990;89(5):630–638
11. Johnston SC, Wilson CB, Halbach VV, et al. Endovascular and surgical treatment of unruptured cerebral aneurysms: comparison of risks. Ann Neurol 2000;48:11–19
12. Piantadosi S. Clinical Trials–A Methodologic Perspective. New York, NY: John Wiley & Sons; 1997
13. Kaptchuk TJ, Goldman P, Stone DA, Stason WB. Do medical devices have enhanced placebo effects? J Clin Epidemiol 2000;53(8):786–792
14. Executive Committee for the Asymptomatic Carotid Atherosclerosis Study. Endarterectomy for asymptomatic carotid artery stenosis. JAMA 1995;273(18):1421–1428
15. Kleinbaum DG, Kupper LL, Morgenstern H. Epidemiologic Research: Principles and Quantitative Methods. New York, NY: Van Nostrand Reinhold; 1982
16. Koivisto T, Vanninen R, Hurskainen H, Saari T, Hernesniemi J, Vapalahti M. Outcomes of early endovascular versus surgical treatment of ruptured cerebral aneurysms: a prospective randomized study. Stroke 2000;31:2369–2377
17. Molyneux A, Kerr R, Bacon F, Shrimpton J. The need for health technology assessment in the management of unruptured intracranial aneurysms [abstract]. Annu Meet Int Soc Technol Assess Health Care 1999;15(1):129
18. Jennett B, Bond M. Assessment of outcome after severe brain damage. A practical scale. Lancet 1975;1:480–484
19. Mahoney FI, Barthel DW. Functional evaluation: the Barthel Index. Md State Med J 1965;14:61–65
20. Rankin J. Cerebral vascular accidents in patients over the age of 60: II Prognosis. Scott Med J 1957;2:200–215
21. Folstein MF, Folstein SE, McHugh PR. “Mini-mental state.” A practical method for grading the cognitive state of patients for the clinician. J Psychiatr Res 1975;12(3):189–198
22. Buck D, Jacoby A, Massey A, Ford G. Evaluation of measures used to assess quality of life after stroke. Stroke 2000;31(8):2004–2010
23. Ware JE Jr, Sherbourne CD. The MOS 36-item short-form health survey (SF-36). I. Conceptual framework and item selection. Med Care 1992;30(6):473–483
24. The International Study of Unruptured Intracranial Aneurysms Investigators. Unruptured intracranial aneurysms—risk of rupture and risks of surgical intervention. N Engl J Med 1998;339(24): 1725–1733
25. Rothwell PM, Slattery J, Warlow CP. A systematic review of the risks of stroke and death due to endarterectomy for symptomatic carotid stenosis. Stroke 1996;27(2):260–265
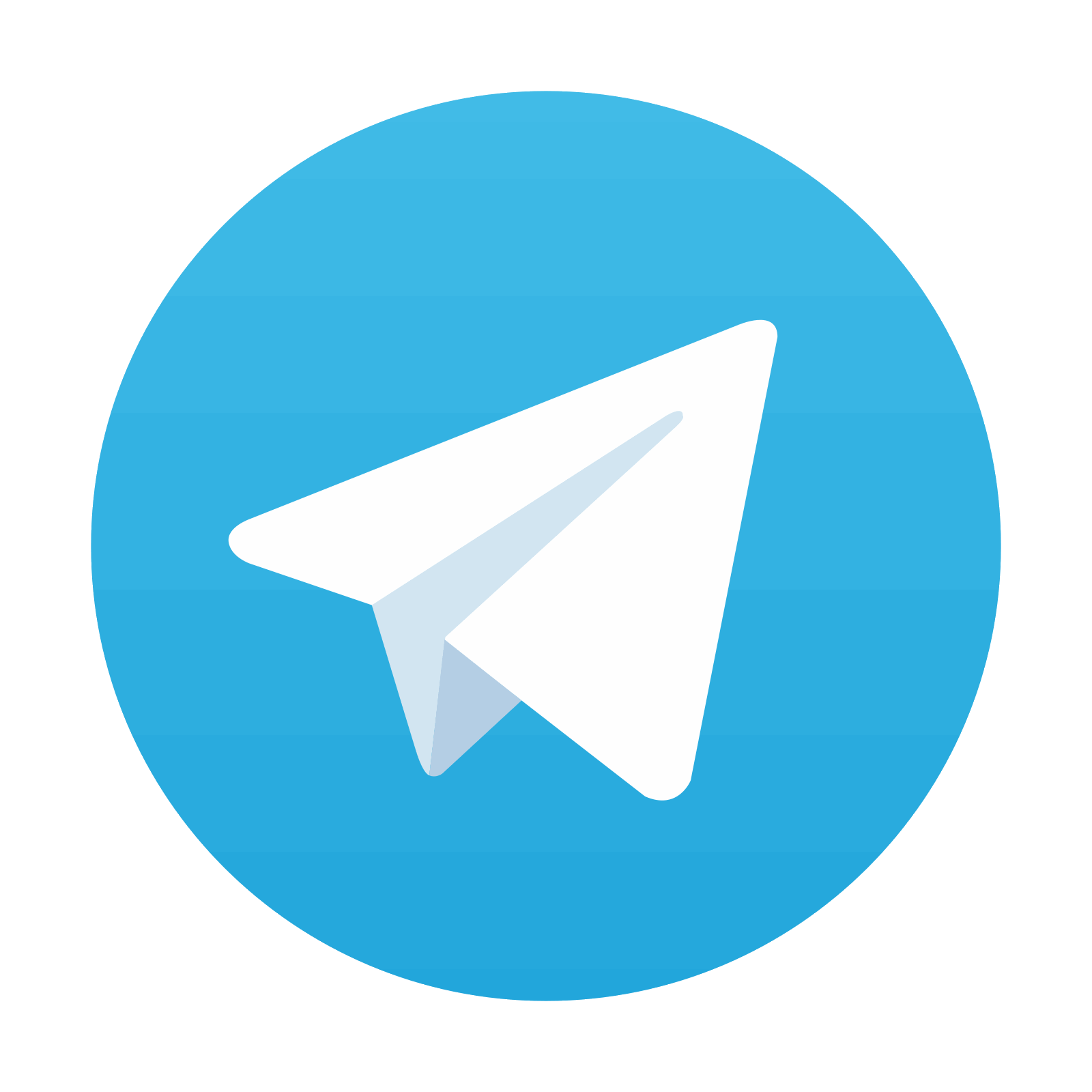