Fig. 5.1
(a) Location of the seed region (brown) and the three exemplary voxels for which co-activation maps are illustrated in panel B, displayed on a surface rendering of the MNI single subject template. The yellow colored exemplary seed voxel 1 is located at −4/−6/+68, seed voxel 2 is located at −2/0/+60, and seed voxel 3 at −6/+12/+48 (all coordinates in MNI space). (b) Brain-wide co-activation maps of three voxels indicated by the yellow numbers in panel A as revealed by meta-analytical connectivity modelling using ALE meta-analysis on the brain-wide foci reported in those experiments in BrainMap that featured the closest activation peaks to the respective seed voxels. (c) Co-activation matrix summarising the co-activation likelihood (ALE values) of all seed voxels to the rest of the grey matter. The grey matter mask is based on at least 10 % probability according to the ICBM (International Consortium on Brain Mapping). This matrix containing the brain wide co-activation pattern of each individual seed voxel served as the basis for co-activation based parcellation of the medial premotor seed region (Reprinted from Neuroimage, 57, Eickhoff, S.B., Bzdok, D., Laird, A.R., Roski, C., Caspers, S., Zilles, K., Fox, P.T., “Co-activation patterns distinguish cortical modules, their connectivity and functional differentiation”, 938–949, 2011, with permission from Elsevier)
As described above, the brain-wide co-activation pattern of each seed voxel was first computed using the BrainMap database. Hierarchical cluster analysis was performed on the seed region’s co-activation matrix to create a stratification of cluster solutions and similarity measures (Fig. 5.2). This revealed a clear-cut separation of the seed region into two co-activation defined clusters. Hierarchical clustering of the seed region was reiterated for a variety of different parameters as an acid test for the robustness of the co-activation derived sub-regions. This assignment of individual seed voxels to specific clusters was stable across all explored analysis parameters such as the similarity-measure between two co-activation profiles or the criterion for cluster-merger. Importantly, the MACM connectivity derived segregation in the seed region corresponded exactly to the two activation clusters obtained from the two different contrasts of the original fMRI study. Cluster formation driven by dissimilarity in co-activation patterns thus recovered the two original activation blobs from the combined seed region in a reliable manner and without a priori constraints imposed on the analysis.
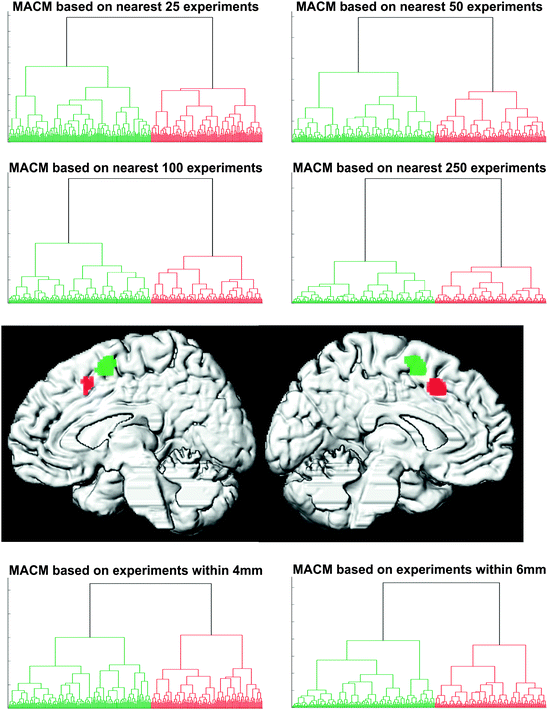
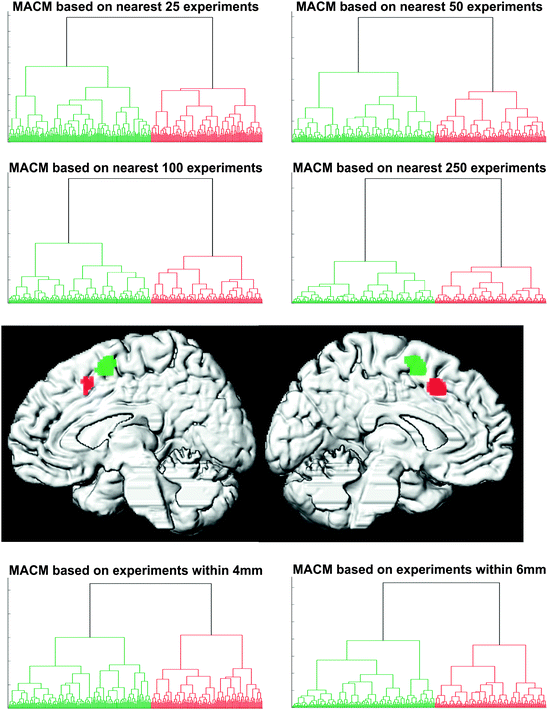
Fig. 5.2
Hierarchical cluster analysis of the co-activation profile matrix (cf. Fig. 5.1c) revealed a highly reliable separation of the seed voxels into two distinct clusters independent of filter criterion and cluster parameters. Projecting the voxels back onto their brain location revealed that these clusters were spatially continuous and corresponded to an anterior and posterior cluster in the medial premotor cortex (Reprinted from Neuroimage, 57, Eickhoff, S.B., Bzdok, D., Laird, A.R., Roski, C., Caspers, S., Zilles, K., Fox, P.T., “Co-activation patterns distinguish cortical modules, their connectivity and functional differentiation”, 938–949, 2011, with permission from Elsevier)
Task-based co-activations of each cluster were then delineated by performing an ALE meta-analysis across all experiments featuring at least one activation in that region, i.e., cluster. The conjunction analysis (Fig. 5.3a) revealed an overlap between the individual co-activation maps of the two clusters in dorsal and ventral lateral premotor cortex, BA 44, primary motor and somatosensory cortices, anterior insula, basal ganglia (particularly putamen), thalamus, superior cerebellum as well as intraparietal sulcus and adjacent inferior parietal lobule. That is, those brain areas are functionally connected to both the posterior and anterior cluster. The difference analysis (Fig. 5.3b) of the co-activation maps for the posterior and anterior cluster showed the clear distinction in functional connectivity pattern that actually drove parcellation of the seed region. The posterior cluster showed higher co-activation probabilities in dorsal premotor cortex, primary motor and somatosensory cortices, cerebellum and basal ganglia (putamen). In contrast, the anterior cluster showed significantly higher co-activation probabilities in ventral premotor cortex, middle frontal gyrus and BA 44, anterior insula and intraparietal sulcus/inferior parietal cortex. The stable distinction of the two clusters in the above analyses thus seems to originate from different co-activation likelihood of the posterior and anterior seed region with areas implicated in sensory-motor functions and cognitive control, respectively.
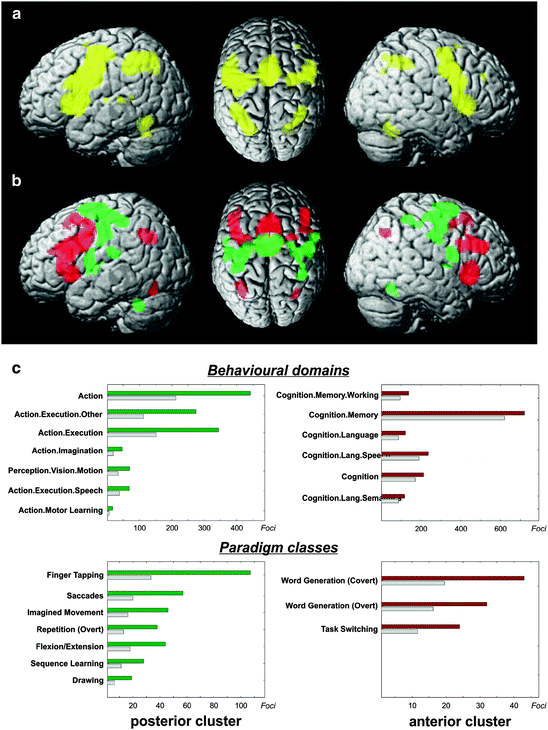
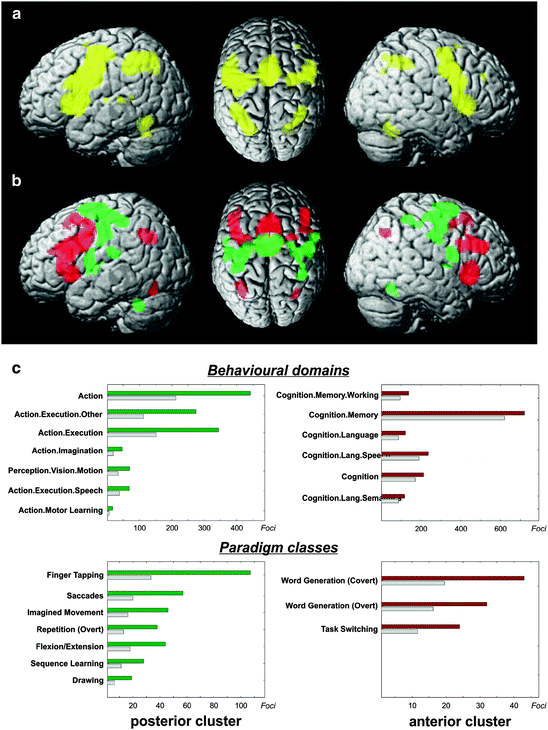
Fig. 5.3
(a) Conjunction analysis over the MACM maps for the two main clusters indicates that several fronto-parietal regions show significant co-activation with both medial premotor regions. (b) Contrasting the MACM maps revealed that the anterior cluster showed significantly higher co-activation probabilities with ventral premotor, inferior frontal and posterior parietal cortices. The posterior cluster showed significantly higher co-activation probabilities with dorsal premotor cortex, primary sensory-motor cortices, cerebellum and basal ganglia. It should be noted that, at the given threshold, many brain regions appear both in the conjunction as well as the contrast analysis. The reason behind this observation is that the connectivity maps of the two clusters differ mainly quantitatively (i.e., the target-voxel-wise connectivity likelihood) and hardly qualitatively (i.e., the topographical pattern of the target voxels). That is, although the two clusters interact with a number of same brain regions, the connection strengths with those target brain areas are different for each cluster. (c) Functional characterisation by behavioural domain and paradigm class metadata from BrainMap. The red/green bars denote the number of foci for that particular behavioural domain and paradigm class within the anterior/posterior cluster. The grey bars represent the number of foci that would be expected to hit the particular cluster if all foci with the respective behavioural domain or paradigm class were randomly distributed throughout the cerebral cortex. That is, the grey bars denote the by-chance frequency of that particular label given the size of the cluster. This analysis indicated that the posterior cluster was strongly related to motor functions whereas the anterior cluster showed lower specificity but was activated predominantly by more cognitive processes, such as language, working memory, and task switching (Reprinted from Neuroimage, 57, Eickhoff, S.B., Bzdok, D., Laird, A.R., Roski, C., Caspers, S., Zilles, K., Fox, P.T., “Co-activation patterns distinguish cortical modules, their connectivity and functional differentiation”, 938–949, 2011, with permission from Elsevier)
Interestingly, there seemed to be a profound topographical correspondence between the conjunction (Fig. 5.3a) and the difference analysis (Fig. 5.3b) of the task-based functional connectivity for the derived clusters. This is related to the fact that the connectivity maps of the two clusters differ mainly quantitatively (i.e., the connectivity likelihood different for the individual target-voxels) and less qualitatively (i.e., the topographical pattern of the target voxels). Put differently, although the two clusters interact with a similar set of brain regions, the connection strengths with those target brain areas were significantly different for the two cluster. In fact, the difference analysis revealed a quantitatively higher connectivity likelihood of the anterior cluster with anterior brain regions, and vice versa, in spite of highly similar network nodes. Importantly, this differentiation was additionally supported by a resting-state functional connectivity analysis in an independent sample of subjects. This analysis revealed, that all voxels indicated as local maxima in the MACM difference analysis also showed significantly stronger resting-state functional connectivity with the respective cluster.
After connectivity-based parcellation and MACM connectivity analysis, the ensuing clusters were functionally characterized by quantitative analysis of the underlying experiments’ meta-data (Fig. 5.3c). This step introduced a first link between the obtained clusters to functional differentiation, i.e., task-relatedness or task-specificity. All behavioural domains (BDs) and paradigm classes (PCs) that were significantly over-represented in experiments activating the posterior cluster were related to motor functions (execution, imagery and learning, overt speech and saccades). The only exception was a significant over-representation of visual motion experiments that may be attributable to the high prevalence of (reflexive) eye movements in these tasks. In contrast to the posterior cluster, conspicuously fewer PCs were over-represented in the anterior cluster indicating lower specificity to particular cognitive processes. Notably, none of the BDs and PCs over-represented in the anterior cluster related to motor behaviour. Rather this region was primarily activated in experiments assessing “higher” cognitive processes, such as working memory, language and task switching. In sum, functional characterisation of the connectivity-derived sub-regions linked the posterior cluster to motor function and the anterior cluster to higher associative processes.
This example thus showed a highly robust distinction in task-based functional connectivity between two medial premotor regions that could be linked to differences in functional properties. The posterior cluster co-activated with (pre-) motor areas and was activated by action-related tasks. The anterior cluster in turn co-activated with pre-frontal and parietal cortices and was associated with cognitive functions. Indeed, the pre-SMA is conceptualised to be more strongly involved than the SMA in more complex, “cognitive” aspects of motor behaviour, such as motor selection or inhibition (Picard and Strick 1996; Rizzolatti and Luppino 2001; Vogt et al. 2007). Supporting this view, connectivity tracing in non-human primates revealed that the pre-SMA receives afferences from the inferior parietal lobule (Luppino et al. 1993) and the prefrontal cortex. The described functional characterisation and co-activation pattern of the anterior cluster relate very well to these pre-SMA properties. Invasive tracing, moreover, provided no evidence for direct connections towards the primary motor cortex from pre-SMA but only from SMA proper (Luppino et al. 1993; Rizzolatti and Wolpert 2005). Taken together, earlier observations converge with the presented findings, and allow identification of the anterior cluster as pre-SMA and the posterior one as SMA proper.
5.5 Perspectives and Future Directions
Research in animals suggested that functional specialization in the cerebral cortex is strongly driven by local differences in brain-wide connectivity patterns. This relation between borders of (functional) cortical modules and brain connectivity is exploited by recent additions to the neuroscientist’s toolbox. Connectivity-based parcellation allows for “blind” discrimination of cortical modules by regional connectional heterogeneity in a given seed region without any prior knowledge.
From a neurobiological perspective, it is possible that more than one clustering solution for a given region of interest can be biologically meaningful. That is because a region of interest may feature a multi-layered functional hierarchy, which can be captured and described at more than one level. To illustrate this idea by an example: The amygdala is usually discussed as a monolithic functional unit in the neuroimaging literature. However, this brain region has been divided into three main nuclei groups based on cytoarchitectonic assessment (Amunts et al. 2005). It is therefore likely to be informative to study the amygdala at both the macroscopically defined and cytoarchitectonically defined hierarchical levels. Indeed, some in vivo connectivity studies focused on the human amygdala as a whole (Roy et al. 2009; Robinson et al. 2010), whereas other authors studied its connectional properties on a sub-regional scale (Saygin et al. 2011; Solano-Castiella et al. 2011). From a global perspective, limitation to a single parcellation scheme of a given target region might thus entail potential loss of biologically plausible information. Even more so, it would mitigate one of the actual strengths of connectivity-based parcellation, namely, the segregation of brain regions at different neurobiological levels.
Indeed, connectivity-based parcellation relying on task-based measures of functional connectivity enables the identification of cortical functional modules at coarse and finer grained scales, ultimately methodologically constrained by the spatial resolution of fMRI and PET measurements. Although different algorithms are conceivable to determine an “optimal” number of parcellation clusters, applying a single automated method seems sub-optimal in the authors’ opinion. Technically, there is no unequivocally accepted approach for this matter. Automated algorithms represent up to now mere attempts that often rely on subjectively pre-specified parameters and are therefore still far from representing reliable all-purpose solutions for clustering similarity matrices. In particular, determination of the “optimal” number of clusters (cluster validity problem) is generally acknowledged to be an unresolved issue in bioinformatics and pattern recognition (Handl et al. 2005; Jain et al. 1999). There is no universal clustering method that works excellent for all types of data and any number of clusters. Existing automated clustering algorithms thus tend to produce unstable parcellation schemes across similarity measures and other pre-specified parameters. Consequently, an informed and confident choice about the most reasonable cluster number in a seed region should build upon consistency across different clustering mechanisms and methodological modalities.
A variety of such clustering methods have already been employed to segment brain regions into functional modules. Spectral reordering lends itself as a semi-automated approach that suggests a specific number of clusters contained in the dataset, i.e., the seed-voxel-brain-connectivity matrix. This approach differs from the hierarchical clustering and k-means clustering by primarily providing a representation of the dataset that allows visually identifying the number of contained distinct components, i.e., potential functional modules. It is noteworthy that spectral reordering does not depend on any choices of parameters or association-measures, in contrast to other approaches. Hierarchical cluster analysis has the advantage of allowing parcellation of the seed regions at various coarseness levels. A set of clustering solutions can thus be obtained from a dendrogram that represents the nested seed voxel assignments to clusters and the similarity levels at which groupings change. K-means clustering, in turn, represents a non-hierarchical approach that is computationally attractive and provides an allocation of all voxels to a pre-specified number of clusters. Both hierarchical and k-means clustering depend on pre-existing domain knowledge, as they require (subjective) input parameters chosen by the investigator.
Taken together, there is currently no gold standard for the clustering mechanism in connectivity-based parcellation of brain regions as each approach comes with its own advantages and inconveniences and the very mechanics of a given clustering approach inherently bias the grouping process. Combing different clustering methods can improve the reliability of the results by consecutive application or by cross-validation. Up to now, the majority of CBP implementations in the neuroimaging field allocated each seed voxel definitively to one, and only one, cluster in a particular cluster solution. Future CBP approaches should shed more light on probabilistic versus absolute (in technical terms, “fuzzy” versus “hard”) clustering methods, which can reveal membership degrees of seed voxels to multiple clusters. Moreover, graph theory might prove to be useful addition to the methodological arsenal. Ultimately, the development of cluster validity measures for post-hoc assessment of the clusters’ meaningfulness (Handl et al. 2005) deserve further improvement as this was neglected so far in neuroimaging, comparing to other bioinformatic disciplines, such as broad-scale gene expression analysis.
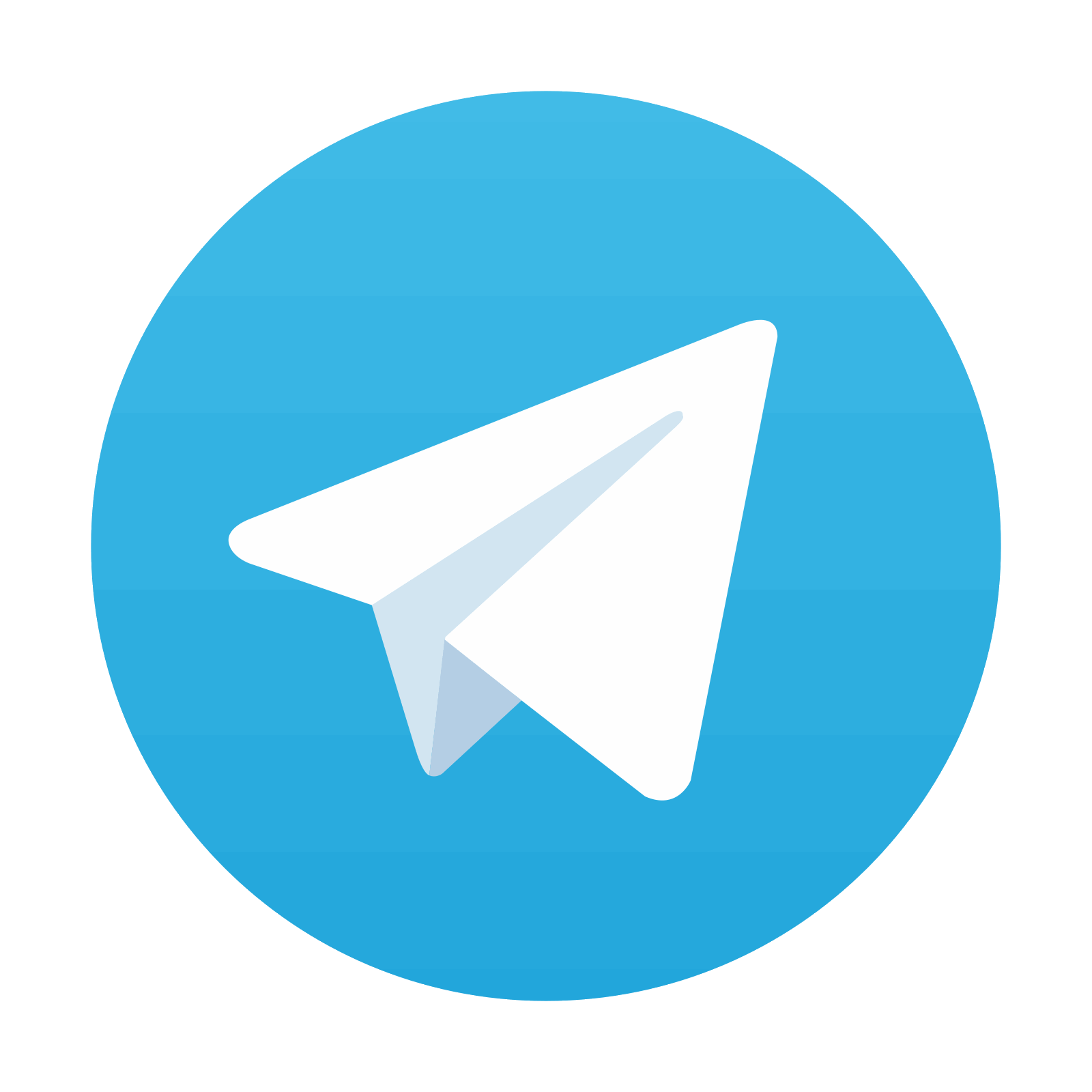
Stay updated, free articles. Join our Telegram channel

Full access? Get Clinical Tree
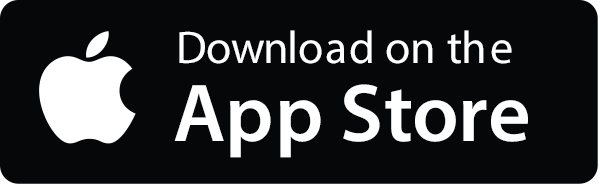
