Abstract
In this chapter, all aspects of electroencephalography (EEG) clinical applications in anesthesia and neurocritical care are reviewed. A large panel of EEG-based monitors is described, with emphasis on their basic principles, indications, and contraindications in clinical practice, and effects of anesthetic agents. Aside from a description of each type of equipment, cues for adequate reading and interpretation of the information provided by those monitors are given, as well as their main advantages and disadvantages. The chapter finishes with a few words on the current opinion of the authors on the clinical utility of all those monitors, and with a few suggested readings in the domain of EEG applied to anesthesia and critical care.
Keywords
Electroencephalography, Monitoring, Neuroanesthesia, Neurocritical care, Technique
Contents
Introduction 78
Basic Principles 79
Electroencephalography 79
Processed Electroencephalography 79
Depth of the Hypnotic Component of Anesthesia Monitoring 84
The Bispectral Index (BIS ™ ) 84
Spectral Entropy of the EEG (GE Datex-Ohmeda Entropy ™ , GE Healthcare, Milwaukee, WI, USA) 84
The Narcotrend ™ 85
The Patient State Index (PSI) 85
The Cerebral State Index (CSI) 85
The Wavelet-Based Anesthetic Value for Central Nervous System Monitoring (WAV CNS ) 85
The aepEX ™ 86
The qCON 86
The Cortical State and the Composite Cortical State 86
Evoked Potentials 86
Nociception–Antinociception Balance Monitoring 87
The Composite Variability Index 88
The Cortical Input 88
The Gradient Between State and Response Entropy 88
The qNOX 89
The Gamma-Band Oscillation Power and High Frequencies of the Electroencephalogram 89
Auditory Evoked Potentials 89
Advanced Analyses 90
Transcranial Magnetic Stimulation 90
Understanding the Equipment 91
Raw and Processed Electroencephalography 91
Depth of Anesthesia Monitoring 92
Transcranial Magnetic Stimulation 93
Indications and Contraindications 93
Raw and Processed Electroencephalography 93
Depth of the Hypnotic Component of Anesthesia Monitoring 94
Nociception–Antinociception Balance Monitoring 94
CVI 94
Cortical Input 95
The Gradient Between State and Response Entropy (RE-SE) 95
The qNOX 96
Gamma-Band Oscillation Power 96
Transcranial Magnetic Stimulation 96
Readings and Interpretation 97
Raw and Processed Electroencephalography 97
Depth of the Hypnotic Component of Anesthesia Monitoring 97
Transcranial Magnetic Stimulation and Perturbational Complexity Index 98
Effect of Anesthetics 99
Raw and Processed Electroencephalography 99
Depth of the Hypnotic Component of Anesthesia Monitoring 100
Advantages and Disadvantages 100
Raw and Processed Electroencephalography 100
Depth of the Hypnotic Component of Anesthesia Monitoring 101
Nociception–Antinociception Balance Monitoring 102
CVI 102
Gradient Between State and Response Entropy (RE-SE Gradient) 103
The qNOX 103
The Gamma-Band Oscillation Power 103
Current Opinion 103
Raw and Processed Electroencephalography 103
Depth of the Hypnotic Component of Anesthesia Monitoring 104
Nociception–Antinociception Balance Monitoring 104
Suggested Readings 104
References 104
Introduction
Through the recording of scalp surface electrical activity, electroencephalography (EEG) is unique as a noninvasive tool to explore the functioning brain. The obtained complex tracings correspond to the summation of the activity of several underlying neural assemblies, and they contain a huge amount of information. The time resolution of this tool is excellent, allowing an almost immediate assessment of brain function, while the space resolution is acceptable when multiple sites of recording are used. The main difficulty resides in extracting the contained information. Several modes of tracing analysis exist, from simple to highly complex, providing corresponding basic to system-integrated information. EEG can also be combined with other techniques, such as transcranial magnetic stimulation (TMS), or functional magnetic resonance imaging (fMRI), to further enhance the understanding of communication between brain regions and information integration by the brain. EEG repercussions of peripheral sensory stimulation can also be studied in the form of evoked potentials, which can be evidenced after repeated stimuli and averaging of EEG waves. Their amplitude and latency can provide very useful information on peripheral afferences processing by the central nervous system. Several of those EEG modalities have given birth to commercially available monitors that are of current use in clinical practice. We will here review all those aspects of EEG, except for the evoked potentials that are extensively described in Chapter VI.
Basic Principles
Electroencephalography
EEG consists in recording electric potential differences between electrodes placed on the scalp surface. Those potentials differences are usually said to be generated by the activity of cortical pyramidal neurons, oriented perpendicularly to the cortical surface ( Fig. 4.1 ). The synchronous firing of large groups of neurons generates detectable potential differences. These activities are influenced by cortico-cortical or cortico-subcortical interactions, notably with the thalamus. The obtained final complex wave corresponds to the summation of all those activities.

Processed Electroencephalography
After digitalization (see Depth of Anesthesia Monitoring section), EEG can be analyzed through several ways ( Table 4.1 ). The most common technique is the visual inspection of the tracing by experienced electrophysiologists, leading to a syntactic or semantic description of the wave shape, amplitude, and frequency. Several EEG patterns can be visually recognized that correspond to different clinical conditions, including sleep stages, focal or generalized seizure, focal or global brain suffering, encephalopathy, brain death, or anesthesia. Such a visual analysis necessitates strong experience, and it is not within reach of all clinicians. In addition to the easy storage of information, the digitalization of the EEG offers several advantages, including post hoc mathematical and statistical manipulation of the tracing to improve filtering, extracting nonvisible parameters that are potentially interpretable by the nonelectrophysiologist, identifying sources of activity, characterizing interactions between brain regions, and making mechanistic inferences regarding brain functions.
Type | Name | Domain | Possible clinical applications | |
---|---|---|---|---|
Visual inspection | EEG alone | Wave shape, frequency, amplitude | Pattern recognition | Sleep stages Focal or generalized seizure Focal or global brain suffering Encephalopathy Brain death DHOA |
Digitized EEG | Zero crossing frequency | Time | Rare | |
α, β; θ, δ; and γ power | Frequency | DHOA | ||
Peak frequency | Frequency | DHOA | ||
Median frequency | Frequency | DHOA | ||
Spectral edge frequency | Frequency | DHOA | ||
Discrete wavelet transform, eigenvector analysis | Time and frequency | DHOA | ||
Coherence | Frequency | DHOA | ||
Bispectrum or phase synchrony | Frequency | DHOA | ||
Shannon or symbolic entropy (amplitude) | Disorder | DHOA | ||
Spectral entropy (power spectrum) | Disorder | DHOA | ||
Approximate, sample, and permutation entropy | Stability in time | DHOA | ||
Autoregressive modeling | Stability/complexity of activity | Mechanistic | ||
Granger causality and symbolic transfer entropy | Functional interactions between brain regions, effective connectivity | Mechanistic | ||
Dynamic causal modeling, mean-field modeling | Effective connectivity, mechanistic | Mechanistic | ||
Phase-amplitude coupling of frequency bands | Connectivity | Mechanistic | ||
Lempel-Ziv complexity, amplitude coalition entropy, synchrony coalition entropy | Effective connectivity and activity complexity | Mechanistic | ||
EEG + TMS | Perturbational complexity index | Effective connectivity, information differentiation and integration | Mental content assessment | |
EEG + fMRI | Functional and effective connectivity | Mechanistic |
Time and Frequency Domain Analyses
Time and frequency domain analyses of the complex EEG wave are considered first-level analyses of the processed EEG. Time domain analysis describes the tracing in time, with parameters such as the zero-crossing frequency, which is the number of times the EEG wave crosses the baseline per unit of time. Frequency domain analyses first require the dissection of the complex EEG wave into all its individual sinusoidal components. This can be done using a Fourier transformation. The result is given in terms of several frequency bands and their relative contribution to the tracing total power, namely the power spectrum of the EEG. Classical frequency bands encompass the alpha (α; from 8 to 12 Hz), beta (β; 12–30 Hz), delta (δ; 0.5–4 Hz), and theta (θ; 4–8 Hz) bands. The gamma (γ; 25–100 Hz) band corresponds to higher frequencies. Several parameters can be derived from the power spectrum. For example, the peak frequency is the one with the highest power, the median frequency corresponds to the frequency where 50% of the power is achieved by lower frequencies, and 50% by higher ones, and the spectral edge frequency corresponds to the frequency where a specified percentage of the power (generally 90% or 95%) is achieved by lower frequencies.
Discrete wavelet transform and eigenvector analysis are other modes of EEG tracing dissection that allow describing the tracing in the time and frequency domain. The method consists in extracting coefficients that represent the correlation between a standard or “mother” wavelet and a localized section of the signal. It is a generalization of Fourier transformation that adds time elements to the frequency analysis, using signal windows of variable size. Wavelet analysis facilitates the analysis of rapidly changing signals.
Time and frequency domain analyses of the EEG of higher complexities have been proposed. For example, coherence measures synchronization between brain regions at a given frequency range, such as the gamma band. Other examples are the bispectrum or phase synchrony, corresponding to phase correlation between different frequency components.
Disorder Within the Electroencephalogram
The EEG is a complex signal, and one approach to its analysis can be the estimation of the disorder within the signal, or, in other words, its entropy. This disorder will vary as a function of brain state, being lower when brain activity is lower and vice-versa. EEG entropy can concern the amplitude (Shannon or symbolic entropy) or the power spectrum (spectral entropy). EEG entropy can also correspond to the stability of the EEG in time. When highly stable, such as during anesthesia, future amplitudes can be predicted with accuracy from previous amplitudes, and entropy is low. Approximate, sample, permutation, and multiscale entropies are examples of such parameters estimating EEG stability. Another way of estimating EEG stability/complexity uses autoregressive modeling methods.
Depth of the Hypnotic Component of Anesthesia Monitoring
The fact that anesthetic agents have effects on the EEG has been known for a long time, but changes in the EEG pattern as a function of anesthesia depth are not necessarily easy to recognize visually. In the 1990s, EEG-derived indexes were proposed to simplify EEG interpretation. Those indexes were initially designed to assess the hypnotic component of anesthesia, or importance of consciousness alteration. The basic principle governing the calculation of such indexes is the extraction of processed EEG parameters, and their introduction into a mathematical algorithm whose output is a dimensionless number between 0 and 100 or a letter. Usually, 0 corresponds to the deepest stage, or isoelectric EEG, and 100 to the wake state. Several depth of the hypnotic component of anesthesia (DHOA) monitors are currently commercially available.
The Bispectral Index (BIS ™ )
BIS™ (Medtronic, Covidien, Minneapolis, MN, USA) is the first ever commercialized DHOA monitor, and the most widely studied. Its algorithm takes account of parameters such as the activity in the β frequency range, synchronized fast-slow activity, bispectrum, and burst suppression (alternating bursts and flat signal).
Spectral Entropy of the EEG (GE Datex-Ohmeda Entropy ™ , GE Healthcare, Milwaukee, WI, USA)
As described in Disorder Within the Electroencephalogram , spectral entropy measures the disorder into the power spectrum of the EEG. The obtained value is then normalized on a 0 to 100 scale, and is thus independent of the frequency and amplitude scale of the EEG. The mathematical algorithm of the GE Datex-Ohmeda Entropy module generates two numbers, the Response Entropy (RE), and the State Entropy (SE). SE is calculated over a frequency range between 0.8 and 32 Hz, which grossly corresponds to EEG activity. RE covers frequencies between 0.8 and 47 Hz, which involves the electromyographic activity of facial muscles in addition to EEG, although with overlap. Normalized SE values range between 0 and 91 and are presumed to reflect the hypnotic component of anesthesia. RE is always higher or equal to SE, and it ranges between 0 and 100. The occurrence of noxious stimulation in the absence of adequate antinociception provokes a facial muscles’ jerk, hence an increase in RE. Consequently, the gradient between RE and SE is proposed to reflect the intraoperative balance between nociception and antinociception (see also Basic Principles , Nociception-anti-nociception Balance Monitoring , The Gradient Between State and Response Entropy ).
The Narcotrend ™
The development of Narcotrend™ (Narcotrend-Gruppe, Hannover, Germany) started with the identification of 15 different EEG patterns recorded during corresponding deepening stages of anesthesia. Each of those patterns was then further characterized by a set of processed EEG parameters extracted from the power spectrum, evaluating entropy, or derived from autoregressive analyses. Again, the output is a number between 0 and 100, in addition to a letter specifying the EEG pattern. The letter A indicates a wake state, B0 to B2 sedation, C0 to C2 light anesthesia, D0 to D2 general anesthesia, E0 to E2 general anesthesia with deep hypnosis, and F0 to F1 general anesthesia with increasing burst suppression.
The Patient State Index (PSI)
The PSI (SedLine™ monitor, Masimo Corp., Irvine, CA, USA) output is also a number between 0 and 100, derived from the relative activity in specific frequency bands, interhemispheric coherence, and anterior to posterior frequency and phase relationships. It necessitates 4 EEG channels, and its development was mainly based on the anesthesia-induced frontal changes in the EEG spectrum.
The Cerebral State Index (CSI)
CSI (Cerebral State Monitor™, Danmeter, Odense, Denmark) calculation involves the activity in the α and β frequency range, the difference between those two activities, and the importance of burst suppression.
The Wavelet-Based Anesthetic Value for Central Nervous System Monitoring (WAV CNS )
For the WAV CNS (NeuroSENSE™ monitor, NeuroWave Systems Inc., Cleveland Heights, OH, USA), time and frequency domain information contained in the EEG is transposed into wavelet coefficients (see Time and Frequency Domain Analyses ). It is highly stable during a steady-state anesthesia and has the shortest time needed for calculation among the commercially available DHOA monitors. Hence, it has a very short reaction time when conditions are changing during anesthesia.
The aepEX ™
The aepEX™ monitor (Medical Device Management Ltd., Essex, UK) is slightly different from the other available DHOA monitors regarding the parameters that enter its algorithm. Following earphones-delivered auditory 7 Hz clicks, the device extracts middle latency auditory evoked potentials using a moving averaging window, and it calculates a 0 to 100 index based on their latency and amplitude. Indeed, amplitude decreases and latency increases with depth of hypnosis.
The qCON
The qCON (qCON2000™, Quantium Medical S.L., Mataro (Barcelona), Spain) uses an Adaptive Neuro Fuzzy Inference System applied to a combination of a set of EEG frequency bands. The monitor also calculates the qNOX index, based on the same technology but devoted to the monitoring of the nociception–antinociception balance (see Basic Principles , Nociception-anti-nociception Balance Monitoring , The qNOX ). The output number ranges between 0 and 99 .
The Cortical State and the Composite Cortical State
The development of the cortical state and its more robust derivative, the composite cortical state (CCS), was based on a mathematical EEG model, which aims at describing the influence external input on cortical activity. CCS is proposed to assess the response of the cortex to an arbitrary stimulus, hence DHOA, in combination with another index, the cortical input (CI, see The Cortical Input .), which quantifies the magnitude of the input to the cortex, and hence should better reflect the effect of antinociceptive medications. Up to now, CCS has been relatively poorly evaluated in clinical practice, but it seems to nicely correlate with DHOA.
Evoked Potentials
Whereas the EEG is the measurement of the spontaneous electrical activity of the cerebral cortex, the evoked potentials are the measurement of the EEG response to a stimulus. They can be measured without patient cooperation and are therefore useful for diagnosis in the operating room under general anesthesia or in the intensive care unit. Several evoked responses can be used for the assessment of the nervous system integrity, including somatosensory-evoked responses (SSEP) obtained following repeated stimulation of the somatosensory system, auditory evoked responses, and visual evoked responses. Other modalities do not look at the EEG response, but rather to the peripheral response after central stimulation. For example, motor-evoked potentials are recorded in muscles following the transcranial electrical or magnetic stimulation of the motor cortex that activates the descending motor pathways. All those evoked responses modalities are discussed in detail in Chapter 6 and will not be covered here, except for some of them that are useful for the assessment of DHOA and the nociception–antinociception balance.
Nociception–Antinociception Balance Monitoring
During anesthesia, individually tailoring the balance between nociception, corresponding to the unconscious consequences of noxious stimulation on the body, and antinociception, corresponding to the administration of antinociceptive medications such as opioids, is important to avoid unwanted movements or excessive autonomic responses to surgical stimulation, and to avoid overdose and its potential side effects, such as prolonged ventilation, escape phenomenon, or postoperative hyperalgesia. Several devices have recently been proposed to better assess this nociception–antinociception balance, or NAN balance. Most of them are based on parameters that reflect changes in the autonomic nervous system in response to noxious stimulation. By nature, those parameters may be influenced by factors that have nothing to do with nociception, such as the intravascular volume status, temperature, or vasoactive medications. Some authors have looked at more central witnesses of nociception processing, to bypass the autonomic nervous system confounding factors. Although nociception is mainly mediated by subcortical circuits located in the spinal cord and brain stem, it has also direct and indirect consequences on cortical activity. At the present time, there is no clear consensus on which EEG frequency bands or features best correlate with the NAN balance, but several EEG-derived NAN balance indices have been proposed. They include the composite variability index (CVI), the CI, and the CCS, the gradient between state and response spectral entropy (RE-SE), the qNOX, the gamma-band oscillations, and the A-line autoregressive index (AAI). In this chapter devoted to EEG-derived monitors, we will limit the description of NAN balance indices to those derived from the EEG.
The Composite Variability Index
The development of CVI was based on the fact that BIS™ value is influenced both by the cortical EEG and by the facial electromyogram. Although BIS ™ value before the occurrence of a noxious stimulation is not a reliable predictor of patient movement, its variability in response to this kind of stimulation is attenuated by antinociceptive medications. Facial EMG is also known to react to noxious stimulation when antinociception is not deep enough. CVI integrates the variability in BIS™ (sBIS) and in facial electromyogram (sEMG). Both sBIS and sEMG can be calculated from frontal EEG tracings. They correspond to the standard deviation of BIS and EMG over a 3 minute time period. The algorithm of CVI is based on a linear combination between sBIS and sEMG, and it was developed from a retrospective multicenter study dataset comprising 307 patients. The parameters entering the algorithm were chosen as a function of their ability to predict the occurrence of somatic events in response to noxious stimulation. The output is a dimensionless number between 0 and 100.
The Cortical Input
As mentioned The Cortical State and the Composite Cortical State , the CI is intended to quantify the magnitude of input to the cortex. It is proposed to assess the effect of antinociceptive medications or NAN balance. When combined with CCS, it seems to perform better than the combination of BIS ™ and CVI to predict response to stimulation, but it still needs further clinical validation.
The Gradient Between State and Response Entropy
The rationale behind using the RE-SE gradient (see Spectral Entropy of the EEG ) for assessing the NAN balance resides in the observation that noxious stimulation modifies facial electromyographic activity, which in turn can be modulated by opioids. The clinical utility of the RE-SE gradient at assessing the NAN balance is still a matter of debate (see Indications and Contraindications , Nociception-anti-nociception Balance Monitoring , The Gradient Between State and Response Entropy ).
The qNOX
The qNOX is the index of the NAN balance displayed by the qCON2000™ monitor (Quantium Medical S.L., Mataro (Barcelona), Spain), and is based on four EEG frequency bands. Its value is also dependent on the qCON value. When qCON is below 25, it is assumed that, due to very deep DHOA, the patients will very unlikely respond to a noxious stimulation. Similarly to qCON, qNOX ranges between 0 and 99.
The Gamma-Band Oscillation Power and High Frequencies of the Electroencephalogram
Noxious stimulation elicits an increase in the power of frequencies in the γ range (30–100 Hz) within the primary somatosensory cortex. The magnitude of this increase in γ-band oscillation power (GBOp) correlates with the perceived intensity of pain in conscious individuals. This does not mean that GBOp is directly related to pain experience, insofar as stimuli perceived as more painful are also more intense, and thus more prone to trigger mechanisms of attentional capture or arousal. GBOp could only reflect unspecific stimulus-triggered attentional processing. However, GBOp does not fade with stimulation repetition, remaining predictive of subjective pain intensity despite novelty reduction resulting from stimulus repetition. GBOp is therefore promising for assessing pain, but it has not been tested during general anesthesia yet to assess the NAN balance.
Analyses of the EEG very high frequencies (105–145 Hz) combined with analyses in the classical frequency range have also been proposed to predict nociceptive responses during sedation and DHOA. Using power spectral density and auto-mutual information measures yielded parameters with acceptable predictive ability. They have not been evaluated in the clinical setting yet.
Auditory Evoked Potentials
Middle latency auditory evoked potentials that correspond to the transfer of auditory information from the medial geniculate body to the primary auditory cortex have been shown to provide information on the NAN balance when combined with the use of BIS™. When maintaining a constant DHOA level through a BIS™-guided sevoflurane administration, the AAI (an index derived from middle latency auditory evoked potentials and close to the aepEX™) response to surgical incision is able to distinguish deep and lighter antinociception. When used alone, AAI is not a better predictor of the response to noxious stimulation than BIS.
Advanced Analyses
The highest sophistication in EEG analysis consists in looking at functional interactions between brain regions. Increasing the number of electrodes placed on the scalp surface improves the space resolution of such analyses. Interactions between brain regions are witnesses of information processing by the brain, and understanding them has the potential to provide insights into the mechanisms of complex phenomena such as the perception of external information and consciousness.
Using powerful statistical tools, it is possible to measure causality between signals recorded at different sites. Such causality relationship is named directed functional connectivity or effective connectivity. For example, Granger causality establishes a statistical link between the activity observed in a specific region and the previous activity of another region. Symbolic transfer entropy is an oversimplification of Granger causality. A mechanistic dimension can be added to the exploration of effective connectivity using dynamic causal modeling and mean-field modeling. In that case, mechanistic models are a priori constructed to describe coupling between brain regions, and the one that best fits recorded data is retained. Phase-amplitude coupling within and between frequency bands across the brain is another way of looking at connectivity, but all types of coupling are not necessarily linked to the consciousness function. Some analytical methods combine the study of effective connectivity and activity complexity using parameters such as Lempel-Ziv complexity, amplitude coalition entropy, and synchrony coalition entropy.
The possible combinations between those different kinds of EEG analyses are almost infinite. Intense research currently combines the use of EEG with other techniques such as fMRI or TMS (see Indications and Contraindications , Transcranial Magnetic Stimulation ) to further improve our understanding of high-level cognitive functions and their perturbation during altered states of consciousness such as anesthesia.
Transcranial Magnetic Stimulation
TMS consists in applying a magnetic field at the contact of the scalp to induce electrical current within the cortex. This current depolarizes the neural tissue, giving rise to action potentials that are transmitted to other brain regions. The response and its transmission can be recorded through an EEG montage, with good spatial resolution when the density of electrodes is high. The characteristics of the stimulation vary as a function of magnetic field rise and decay times, and frequency of repetition. The depth of penetration essentially depends on coil geometry and stimulus strength. When guided by a structural magnetic resonance imaging-based navigation system, TMS can be applied on precise cortical regions. When repeated, the stimulation may have several neurobiological aftereffects, including changes in synaptic plasticity, modulation of neurotransmitters, second messenger systems, and immediate early genes, among others.
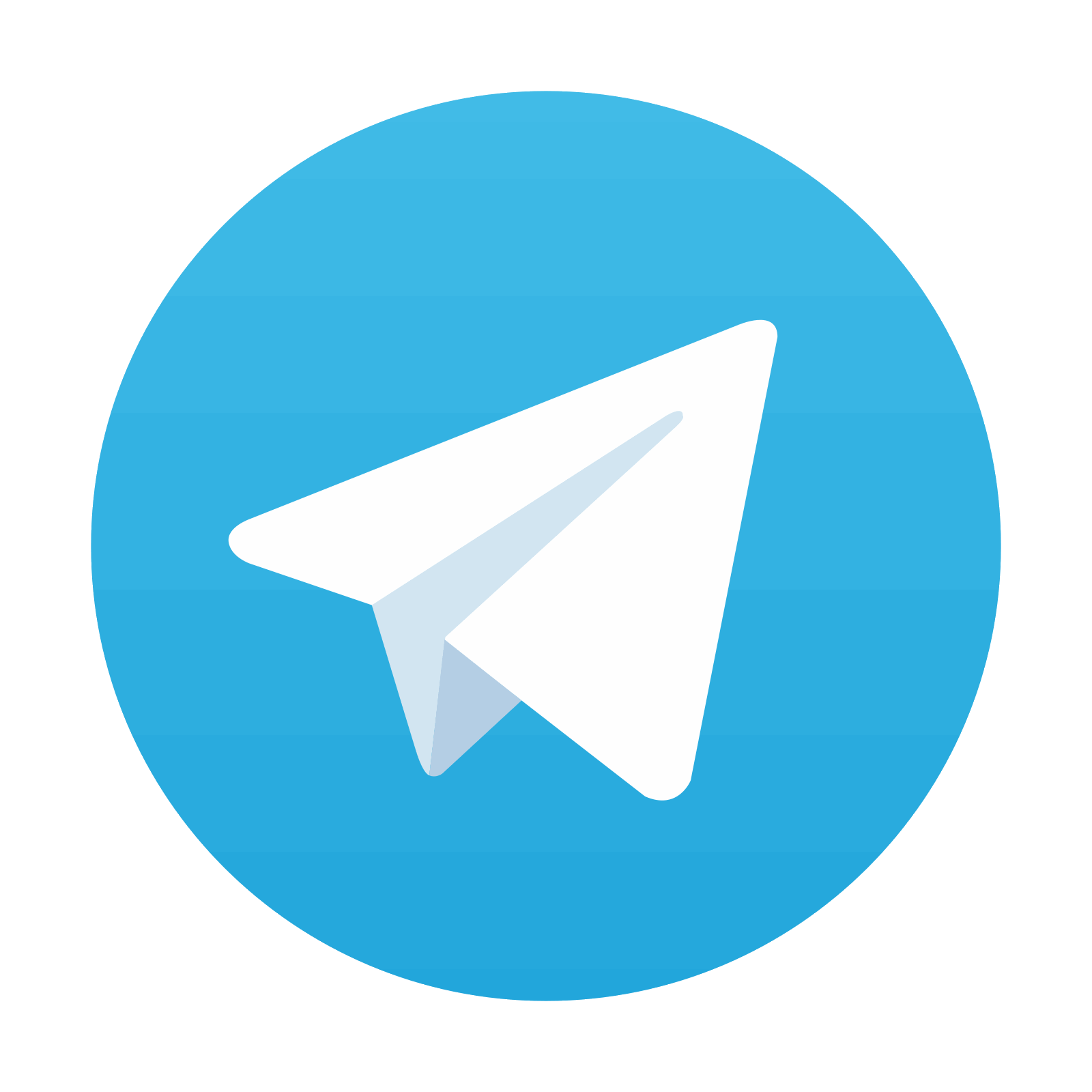
Stay updated, free articles. Join our Telegram channel

Full access? Get Clinical Tree
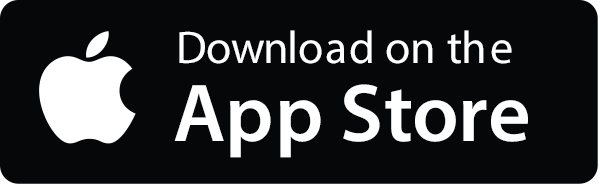
