Fig. 14.1
Electrode positions in international 10–20 system
Conventionally, considerable time was required to quantitatively examine slow-wave activity by means of visual observation. Using NAT technology, however, it is possible to quantitatively check the features of large sets of data in a short time by comparing the patterns with representative patterns (templates) of certain disorders prepared in advance.
In this article, we shall first describe the EEG features of DLB patients in an awake, resting, and eyes-closed state that are visually recognizable and then discuss those features quantitatively obtained by NAT analysis.
14.2 EEG Visual Inspection
14.2.1 EEG of Healthy Aged Subject in Awake, Resting, and Eyes-Closed State
Figure 14.2 shows an example of the EEG data of a healthy aged subject with no cognitive function disorders. The EEG is that of a female subject, aged 70, who scored 29 on the Mini-Mental State Examination (MMSE). Alpha waves of about 12Hz appear prominently in the occipital region and display some waxing and waning. This is considered as a normal EEG. However, such clean EEG rhythms are not seen often among subjects aged 60 and over, who tend to display the slowing or diffusion of alpha waves, increase of theta waves, or slowing of waves in the temporal regions [2, 3].


Fig. 14.2
Example of EEG of aged subject with normal cognitive functions in an awake, resting state with eyes closed (age 70, female, MMSE = 29. Alpha waves of about 12Hz appear dominantly in the occipital region and display waxing and waning)
14.2.2 EEG of DLB Patient in Awake, Resting, and Eyes-Closed State
Figure 14.3 shows an example of the EEG data of a DLB patient. The EEG is that of a female patient, aged 87, who scored 10 on MMSE. It displays a moderate slow abnormality with a predominance of theta waves and irregular, sustained alpha waves in the frontal region. The EEG is clearly different from that of Fig. 14.2, but it would be difficult for non-experts.


Fig. 14.3
Example of EEG of DLB patient in an awake, resting state with eyes closed (age 87, female, MMSE = 10. Moderate slow abnormality with theta waves predominating. Irregular sustained alpha waves are seen in the frontal region)
14.3 NAT (Neuronal Activity Topography)
To obtain NAT, two markers, sNAT and vNAT, which characterize the brain activity are defined from the power spectrum of the EEG potential recorded with electrodes and then analyzed [6]. This yields two types of information: (1) images of brain activities (i.e., the NAT images) and (2) a numerical index (i.e., likelihood) that indicates to what degree the obtained EEG is similar to those representing the average brain activity patterns specific to certain disorders (Fig. 14.4).


Fig. 14.4
NAT analysis flow
The details of sNAT and vNAT are given below.
14.3.1 sNAT
The EEG potentials are divided into 0.64 s segments, each of which is Fourier transformed, so that the power spectrum is discretized into frequency bands expressed by mf 0, i.e., integer multiples of the basic frequency f 0 (=1/0.64 s = 1.5625 Hz). The average power for the mth frequency bin mf 0 for the jth electrode shall be denoted S j,m . Since the frequency band we wish to analyze is in the range 4–20 Hz, i.e., theta, alpha, and low beta, which normally appear when the subject is awake, resting and with eyes closed, the ten frequency bins which are subjected to analysis are 4.7, 6.3, 7.8, 9.4, 10.9, 12.5, 14.1, 15.6, 17.2, and 18.8 Hz, corresponding to m = 3~12. The components of the normalized power spectrum are defined as follows:


(14.1)
This value represents the distribution ratio of the power of the frequency bin in question within the EEG spectrum.
To emphasize the variation of the distribution ratio of the EEG power for the frequency bin mf0, we subtract the average value for all electrodes from the above and call it marker “sNAT.” This marker consists of the ten submarkers, sNAT j,m , which are expressed as follows:


(14.2)
Since there are 21 electrodes, there are 210 submarkers in total. This value represents “the degree to which the distribution ratio of the power for a certain frequency bin differs from the average of that frequency bin for the entire scalp” and is related to the level of neuronal activation.
14.3.2 vNAT
Brain activities are a manifestation of biological information being transmitted within the brain. Biological information is encoded by the manner (frequency) in which neuronal activation is turned on or off. As neuron groups are locally switched on/off in response to biological signals, this causes changes in the potential of each frequency bin, and the level of the potential power will be different for each frequency bin. This causes the power levels of adjacent frequency bins to differ. In order to quantify this effect in a simple manner, the ratio of the power levels of two adjacent frequency bins is defined as:


(14.3)
And NPV j,m is defined, so that it will have the range 0–1, as follows:


(14.4)
As in the first marker, when the average value of all electrodes is subtracted from this to emphasize the variations, we obtain the second marker which we call vNAT. This marker consists of ten submarkers, vNAT j,m , defined as follows:
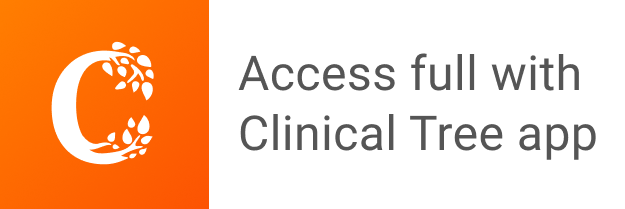