Evaluating the Data: Limitations of Research and Quality Assurance Issues Regarding Natural Remedies
Maurizio Fava
David Mischoulon
How do we know whether a natural remedy—or any remedy, for that matter—is effective? Unlike registered medications, which typically require years of extensive testing before they are allowed on the market, natural remedies are freely available without much regulation or scientific data to support their efficacy and safety. Consumers and clinicians are therefore in the difficult situation of evaluating the appropriateness of these medications, without the security provided by the endorsement of the medical establishment. Doctors and patients need a reliable method to reach their own decisions when faced with the choice of whether or not to recommend or use a particular alternative medicine.
Positive anecdotal experience, as seen when therapeutic successes outnumber therapeutic failures, is a common way for an alternative remedy to earn support. Consumers who get better by trying a particular natural medication or other therapy may recommend it to friends. Clinicians who see their patients improve with such a treatment may recommend it to other patients, as well as to professional colleagues. Marketing, especially direct-to-consumer advertisements, such as ads that feature testimonials of satisfied customers, may also have a significant impact on choice of and requests for a particular remedy. The popular media’s frequent stories on natural products may also influence the public as well as clinicians.
For medical professionals, the scientific literature and academic conferences remain the most trusted sources of information—scant as it may be in some cases—on alternative treatments. The growth of the Internet has facilitated public access to scientific articles and proceedings of symposia, allowing more ambitious consumers to peruse the same sources of information available to physicians. Increasing numbers of consumers can now bring questions about the literature into their clinical appointments, calling upon clinicians to assess research data from the standpoint of making recommendations to their patients. This chapter provides a framework for clinicians to critically and effectively assess the data in the scientific literature, with emphasis on some issues specific to complementary and alternative medicine.
EVALUATING THE SCIENTIFIC LITERATURE: OVERVIEW
By and large, clinicians are more likely to place their trust in a given treatment when the medical literature supports its efficacy and safety. When evaluating, say, a report of a clinical trial of an herbal remedy, several general factors about the article should be considered. First, was the article published in a peer-reviewed or a non-peer-reviewed journal? The former generally has more credibility than the latter because the data are more carefully scrutinized before publication is offered to the authors. Second, who sponsored the publication or the research? If a manufacturer or distributor of natural remedies is the sponsor, this could suggest a possible conflict of interest for the authors. Academic editors nowadays are demanding
more thorough disclosures on the part of study authors who seek publication in their journals. The reader should therefore pay close attention to whether any potential conflicts of interest were adequately disclosed and discussed. If the National Institutes of Health or other federal agencies were involved in funding the study, this typically means higher standards in the conduct of the study and fair reporting of its results. Federally funded studies have Data Safety and Monitoring Boards that provide independent oversight on the project, and also have a number of other elements in place aimed at improving the quality of the science. Third, readers should consider whether the efficacy findings are statistically significant. Statistically significant outcomes are generally considered more credible, and thus have a greater chance of being fully reported, compared with nonsignificant outcomes for both efficacy and harm data (1). Statistical significance can sometimes be deceiving; however, small, underpowered studies that yield statistically significant results are often not replicated in subsequent, larger studies.
more thorough disclosures on the part of study authors who seek publication in their journals. The reader should therefore pay close attention to whether any potential conflicts of interest were adequately disclosed and discussed. If the National Institutes of Health or other federal agencies were involved in funding the study, this typically means higher standards in the conduct of the study and fair reporting of its results. Federally funded studies have Data Safety and Monitoring Boards that provide independent oversight on the project, and also have a number of other elements in place aimed at improving the quality of the science. Third, readers should consider whether the efficacy findings are statistically significant. Statistically significant outcomes are generally considered more credible, and thus have a greater chance of being fully reported, compared with nonsignificant outcomes for both efficacy and harm data (1). Statistical significance can sometimes be deceiving; however, small, underpowered studies that yield statistically significant results are often not replicated in subsequent, larger studies.
GENERAL CONCEPTS IN CLINICAL TRIALS
Double-blind, placebo-controlled, randomized clinical trials (RCTs) have been the gold standard of clinical research for several decades (2). These studies typically have an “arm” for the investigational treatment, as well as one or more comparator arms, which may include placebos, as well as active comparators—usually a standard drug that is Food and Drug Administration (FDA)-approved for treatment of the disorder under investigation. The standard medication allows us to compare the new treatment against an established one, while the placebo allows us to determine the degree to which the observed benefit from the medication tested is due to the medication itself rather than to other factors. A two-arm study demonstrating that a new agent has comparable efficacy to that of a standard comparator does not necessarily prove that the new agent is effective, since the improvements in both arms of the study could have been related to nonspecific, placebo-like effects. Multiarm studies that include both a placebo comparison and an active comparator, however, are usually costly since they require large numbers of participants and may therefore take more time to complete. In addition, studies with three or more arms typically involve less than a 50% chance of receiving placebo, and this has been shown to actually enhance the placebo effect (3), presumably by increasing the patients’ expectations of improvement. For these reasons (cost, time, and risk of placebo response enhancement), most RCTs will have only 2 arms, one for the experimental treatment and one for placebo.
Assignment of treatments in a double-blind manner means that that neither the investigators nor the study subjects know what treatment the subject is receiving, thus minimizing biases secondary to expectations from a known treatment. Randomization, meaning that treatments are assigned based on chance, reduces the risk of selection biases, for example, the repeated assignment of patients with certain characteristics to a particular treatment arm. The patient population, or study sample, should, as much as possible, reflect the population at large with regard to gender, age, and ethnicity. The validity of the outcome measures and statistical analyses should also be carefully scrutinized. And, particularly with regard to natural remedies, we need to consider factors in the study that may affect placebo response.
Unfortunately, many natural remedy studies are uncontrolled, meaning that they lack a placebo or an active comparator arm, and this makes it difficult to separate true drug effects from placebo-like effects. Even well-designed double-blind RCTs may have significant limitations. One frequent concern is whether the statistical power is adequate to test the study’s hypotheses. Statistical power refers to the likelihood of observing a predicted effect size under certain prespecified conditions. Usually, the desired statistical power of a clinical trial is equal
to or greater than 80%. Underpowered controlled studies are those that are conducted with sample sizes that, given an expected difference in the degree of response across study arms (e.g., effect size), yield less than 80% power. Effect size refers to the difference in mean change in score (from baseline to end point) of the outcome-measuring instrument (e.g., a symptom questionnaire) for patients treated with drug versus patients treated with placebo, divided by the pooled standard deviation of the two groups. Mathematically, this is expressed as follows:
to or greater than 80%. Underpowered controlled studies are those that are conducted with sample sizes that, given an expected difference in the degree of response across study arms (e.g., effect size), yield less than 80% power. Effect size refers to the difference in mean change in score (from baseline to end point) of the outcome-measuring instrument (e.g., a symptom questionnaire) for patients treated with drug versus patients treated with placebo, divided by the pooled standard deviation of the two groups. Mathematically, this is expressed as follows:

The key message of this equation is that the greater the difference in scores between active treatment and placebo, the larger the effect size, and hence the better the chance of obtaining adequate statistical power with a given patient sample. Effect sizes are usually classified as follows: small, 0.2 to 0.39; moderate, 0.4 to 0.59; or large, 0.6 or greater. In order to detect small effect sizes, it is ideal to have at least 300 patients per arm; large effect sizes, on the other hand, can be detected with much smaller samples. When planning for a controlled study, it is essential to estimate its statistical power. Dr. David Schoenfeld, an authority on statistical analyses of clinical trials, has developed a very useful Web-based tool (http://hedwig.mgh.harvard.edu/sample_size/size.html) that allows investigators to quickly estimate the statistical power of their study, once the assumptions about the efficacy of the treatment arms are available.
ESTABLISHING DIAGNOSIS AND SUBJECT ELIGIBILITY IN CLINICAL TRIALS
A reliable and valid diagnosis of the patients enrolled into a clinical trial is a key requirement for meaningful results. In particular, clinical interviews are the most common way in which psychiatric investigators evaluate interested patients in order to decide whether they are qualified to enter a particular clinical trial. Structured interviews refer to those that are based on an established diagnostic questionnaire, such as the Structured Clinical Interview for the Diagnostic and Statistical Manual (4) and the Mini International Neuropsychiatric Interview (5). The use of such instruments is generally considered the most accurate and reliable means of establishing the presence of a psychiatric disorder in a potential study subject.
In unstructured interviews, where no rigorous diagnostic instruments are used, psychiatric diagnoses may be subject to clinician biases, and inter-rater reliability may be poor, meaning that two or more clinicians may significantly disagree on the diagnosis and/or severity of illness of a particular study subject. Unstructured diagnostic criteria may increase the risk of placebo-like effects (i.e., a perceived “improvement”) in study participants who did not meet formal diagnostic criteria in the first place. Even in cases where structured interviews and severity questionnaires are used, artificial inflation of scores may result from pressure to recruit or financial incentives from study sponsors (such as bonuses for sites that meet recruitment quotas). Unfortunately, the body of published natural remedy studies in psychiatry is characterized by wide variability of diagnostic techniques compared to studies of synthetic drugs, and this has hindered interpretation of the available data as a whole.
Clinical trials usually have a set of predetermined inclusion and exclusion criteria for potential study participants. Some studies will recruit “all comers” with a particular disorder, while others recruit specific subpopulations of patients—usually those with minimal comorbidity, such as other psychiatric diagnoses, medical illnesses, and substance use problems. In addition to a firm diagnosis, clinical studies usually require a threshold of severity of illness, such as a
minimum Hamilton Depression (HAMD) rating scale for score in depression (6). Over the years, there has been a tendency to progressively elevate such thresholds, under the assumption that this approach would yield the enrollment of subjects with relatively more true forms of illness and would minimize the placebo response. However, it is now well established that the opposite is true, in that the number of patients responding to placebo in depression trials is positively related to the minimum HAMD score required for entry into such studies (7).
minimum Hamilton Depression (HAMD) rating scale for score in depression (6). Over the years, there has been a tendency to progressively elevate such thresholds, under the assumption that this approach would yield the enrollment of subjects with relatively more true forms of illness and would minimize the placebo response. However, it is now well established that the opposite is true, in that the number of patients responding to placebo in depression trials is positively related to the minimum HAMD score required for entry into such studies (7).
Another assumption that was often made in the past was that “purer” forms of a given psychiatric disorder would lead to greater separation between active treatment and placebo. This myth was also dispelled over time, as patients without comorbid illnesses turned out to be more likely to respond to placebo than those with such comorbidities (8,9). Whether or not concomitant medications or psychotherapies are allowed in the study is also important to consider, since these can impact on psychiatric symptoms and confound medication-related effects. Tighter inclusion/exclusion criteria may produce more reliable data, but such studies may be criticized for being less reflective of the “real world” of practice, thus limiting generalizability of the findings (10).
THE STUDY SETTING: LOCATION, LOCATION, LOCATION
Most psychiatric clinical trials are conducted in outpatient settings, though many are still carried out in inpatient units. The latter tend to have more severely ill patients compared to outpatient studies, and generally better treatment adherence, thanks to closer scrutiny of the patients. These differences may impact on the quality of the data as well as on the generalizability of the findings. Some studies are conducted in a single clinical center while others may employ a multicenter design, recruiting patients from several different venues. The latter may provide for larger patient samples with greater ethnic, socioeconomic, and geographic diversity, but may be more cumbersome and costly to administer. Likewise, studies may be conducted in an academic site or a nonacademic site (or both). With well-trained clinicians and adequate oversight from the study principal investigators, both academic and nonacademic sites can perform well in a psychiatric clinical trial. In general, the degree of experience and sophistication that the site clinicians have in conducting clinical trials, and whether they are compliant with Good Clinical Practice (GCP) will influence the quality of the results obtained.
Leading academic centers in psychiatric research used to play a key role in providing oversight to the implementation of multicenter clinical trails in psychiatry and in ensuring compliance with GCP. However, Dr. Miriam Shuchman (11) in a recent review pointed out that, “in a trend that has received surprisingly little attention, contract research organizations (CROs) have gradually taken over much of academia’s traditional role in drug development over the past decade.” Private, for-profit CROs have claimed to offer greater speed and efficiency in conducting clinical trials than academic groups, but questions have been raised about their qualifications, ethics, accountability, and degree of independence from their industry clients (11). In fact, annual CRO-industry revenues have increased from about $7 billion in 2001 to an estimated $17.8 billion (11). In an effort to bring academia back to the center of psychiatric drug development, the Massachusetts General Hospital (MGH) recently established an academic, non-for-profit CRO focused on psychiatric drug trials, the MGH Clinical Trials Network and Institute.
HOW ARE TREATMENT OUTCOMES DEFINED AND MEASURED?
Treatment outcomes often depend on the definition of the outcome. Outcome measures are usually chosen a priori, during the design phase of the study, but they may be modified as the
study progresses (when the investigators are still blind to the outcome) or even after the study is complete (post hoc). The a priori outcome definition may reduce the likelihood of “data massaging” in cases where the findings were not as expected, while post hoc decisions to change outcome measures are often viewed as carrying a great risk of bias. In any case, outcome measures should be based on established criteria that have been previously validated, so as to ensure integrity of the study findings and the conclusions drawn. Outcome measures may be categorical (e.g., response or nonresponse) or continuous (e.g., a decrease in the score of a depression rating scale or the amount of time to response), and studies often use both, depending on the range of questions the investigators wish to answer. In depression studies, for example, outcome measures should ideally assess both response and remission, and these in turn should be based on standard criteria, such as “response” meaning a decrease of 50% or more in the score on the HAMD-17 scale and “remission” meaning a final HAMD-17 score of 7 or less (12).
study progresses (when the investigators are still blind to the outcome) or even after the study is complete (post hoc). The a priori outcome definition may reduce the likelihood of “data massaging” in cases where the findings were not as expected, while post hoc decisions to change outcome measures are often viewed as carrying a great risk of bias. In any case, outcome measures should be based on established criteria that have been previously validated, so as to ensure integrity of the study findings and the conclusions drawn. Outcome measures may be categorical (e.g., response or nonresponse) or continuous (e.g., a decrease in the score of a depression rating scale or the amount of time to response), and studies often use both, depending on the range of questions the investigators wish to answer. In depression studies, for example, outcome measures should ideally assess both response and remission, and these in turn should be based on standard criteria, such as “response” meaning a decrease of 50% or more in the score on the HAMD-17 scale and “remission” meaning a final HAMD-17 score of 7 or less (12).
There are two basic types of instruments used to assess outcome. Clinician-rated (as opposed to patient self-administered) instruments are often considered the most reliable and the most sensitive to change, though these do not always prevent investigator and/or patient biases, and may still produce unsatisfactory inter-rater reliability, especially when clinicians do not have consistent and adequate research training. Self-rated instruments are especially subject to patient biases, test-retest reliability issues, and therapeutic sensitivity issues. However, in some cases, certain self-rating scales have outperformed clinician-rated scales such as the HAMD. For example, in a study comparing 300 mg of imipramine with 150 mg of imipramine, the self-rating scale Symptom Rating Test (SRT) discriminated significantly between the two doses, but not the total HAMD score and the SRT had been developed specifically to increase the sensitivity of outcome measures of depression and anxiety (13,14).
Whatever instruments an investigator uses, we need to understand whether they are truly appropriate and have adequate therapeutic sensitivity for detecting illness and changes thereof in the study. Their psychometric properties, e.g., internal and concurrent validity and reliability, must be well understood. Multidimensional instruments, for example, may be less appropriate for natural remedies with selective effects.
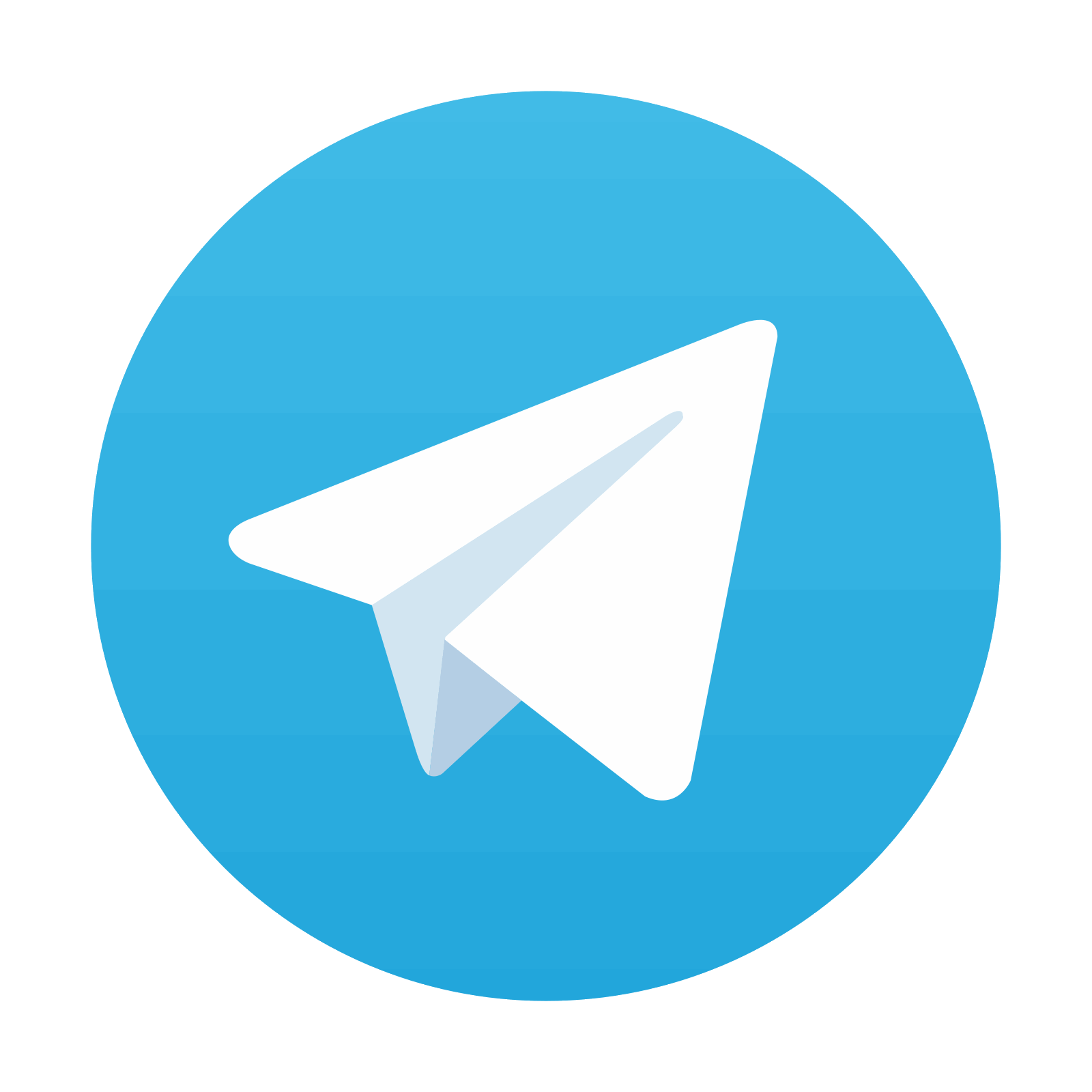
Stay updated, free articles. Join our Telegram channel

Full access? Get Clinical Tree
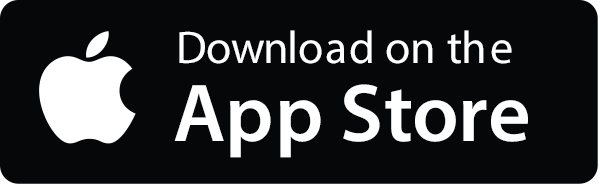
