Total scale raw score
T-scores based on comparison to others with ASD
T-scores based on comparison to national normative group
150
54
77
140
52
74
130
50
71
120
48
69
110
46
66
100
44
63
90
42
60
80
40
57
70
37
55
60
35
52
50
33
49
40
31
46
Mn
117.8
53.1
SD
50.3
36.1
Table 3.1 provides a raw score to T-score conversion table based on the means and standard deviations (SD) for the national and ASD reference groups. What is most remarkable about the results is that a raw score of 80 on the ASRS would yield a T-score of 40 if the reference groups were individuals who had previously been identified as having ASD. This would suggest that a child who earned this score would be one SD below the mean for the reference group of individuals with ASD. It would be reasonable to conclude that an individual who obtained such a score was not like those with ASD. The raw score of 80, however, is equal to a T-score of 57 in relation to the national norm, nearly one SD above the national mean. These results illustrate how different conclusions may be reached when the same rating scale is calibrated against two different samples. We suggest that the comparison with the national norm is more informative and the score that should be used to understand the extent to which an individual evidences behaviors associated with ASD. The use of a national norm also has considerable impact on evaluation of behavior change as a function of intervention.
Calibration of Change
In recent years, practitioners and researchers in educational and psychological settings have compared raw scores from a test to evaluate the effectiveness of some academic instruction or other treatment. Naglieri (2012) illustrated how the comparison of raw scores over time can be misleading when students’ progress over time was monitored using words read per minute as a measure of reading skill. The issue is that some tests of skills, like reading or vocabulary, show a very strong age-to-age progression of raw scores. This progression reflects the typical changes in skills that occur as a child grows older as a combination of factors including maturation, learning from the environment as well as school. In order to calibrate change as a function of some specific instruction or intervention, the amount of change should be calibrated to the normal growth curve, not only the pretreatment level. This is a particularly important issue in treatment programs for individuals with ASD.
Expressive vocabulary is one variable that is often studied as a way to demonstrate improvement over time (e.g., Kasari et al. 2008). The choice of which expressive vocabulary test and score to use, has a profound impact on the result. Using the Kasari et al. study results as a guide, we obtained the standard scores using the normative tables from the Expressive Vocabulary Test (Williams 1997). The results shown in Fig. 3.1 suggest that there were changes in performance from Time 1 through Time 4 (12 month interval). Both treatment groups appear to have higher scores at Time 4. The interpretation of these data could lead to the conclusion that the treatments were effective but examination of the standard scores associated with these raw scores suggests a different conclusion as shown in Fig. 3.2.
When the raw scores are converted to standard scores (mean of 100 and SD of 15) shown in Fig. 3.2, the results suggest that although the raw scores increased over the 12-month interval the standard scores associated with these raw scores actually showed no improvement. That is, even though the two treatment (as well as the control) groups’ raw scores increased, the difference between those scores and the mean for the standardization group remained large. In fact, the average raw scores for the four age groups are 41, 48, 52, and 56. Therefore, we suggest that raw score improvement alone is insufficient to show treatment effectiveness. Standard score improvement provides an additional reference point that must be taken into consideration in order to determine if a treatment is sufficiently effective.

Fig. 3.1
Performance from time 1 through time 4

Fig. 3.2
Conclusion on the basis of examination of standard scores associated with the raw scores
Reliability of Measurement
Consideration of the role reliability plays in evaluation of treatment change is essential because all measurements have some degree of error. In classical test theory, an obtained score is comprised of the true score plus error (Crocker and Algina 1986). For this reason, we should always report an obtained score with a range of values within which the person’s true score likely falls with a particular level of confidence. The size of the range is determined by the level of confidence and the reliability of the measurement; the higher the reliability the smaller the range. When reporting a T-score, for example, we state that a child earned a score of 50 (± 7); meaning that there is a 95 % likelihood that the child’s true IQ score falls within the range of 43–50. The range of scores (called the confidence interval) is computed by first obtaining the standard error of measurement (SEM) from the reliability coefficient and the SD of the score in the following formula (Crocker and Algina 1986):

The SEM is considered the average SD (68 % of the normal curve is in this range) of the theoretical distribution of a person’s scores around the true score. If one SEM is added to and subtracted from an obtained score, there is a 68 % chance (the percentage of scores contained within ± 1 SD) that the person’s true score is contained within that range. The SEM is multiplied by a z value of, for example 1.64 or 1.96, to obtain a confidence interval at the 90 or 95 % levels, respectively. The resulting value is added to and subtracted from the obtained score to yield the confidence interval. For example, the 95 % confidence range for a test score with a reliability of .95 and an obtained T-score of 60 (recall that a T-score is set to have a mean of 50 and SD of 10) is 57 (60 – 3) to 63 (60 + 3). It is important to note that the higher the reliability the smaller the interval of scores that can be expected to include the child’s true score. The smaller the range, the more precise the practitioners can be in their interpretation of the results, resulting in more accurate decisions regarding the child. The relationships between reliability and size of the confidence interval is provided in Fig. 3.3 for T-scores (M = 50; SD = 10). Confidence intervals should always be used for interpretation of all scores because they take measurement error into account at a specific level of probability.

Fig. 3.3
Relationship between reliability and confidence intervals for T-scores
The method of computing confidence intervals described above has been modified in recent years by some test authors (e.g., Wechsler and Naglieri 2006) to be theoretically more accurate than the approach of basing confidence intervals around obtained scores (Nunnally 1978). The modification involves centering the confidence interval on the estimated true score. This approach accounts for measurement error associated with the scores to provide a band of error that is centered on the estimated true score and thereby takes into account regression to the mean (Salvia and Ysseldyke 1981). Figure 3.4 shows the relationships between obtained scores from 30 to 70 and the confidence intervals associated with those scores using estimated true score-based method. This is the method used in most ability tests and some rating scales.

Fig. 3.4
Upper and lower values of estimated true score-based confidence intervals for T-scores
Reliability and Comparison of Scores
The SEM is also particularly important when scores from different raters are compared. When comparing scores earned on the same scale, it is critical to recognize that the lower the reliability the larger the SEM, and the more likely scores will differ as a function of measurement error i.e., the lower the reliability, the more likely there will be differences among scores. Inconsistent results that reflect measurement error can complicate the interpretation of pre- and postfindings, and make a clear understanding of an individual’s treatment progress more difficult to interpret. For example, when a researcher or practitioner is attempting to determine whether the several scores an individual has received are similar or significantly different, the answer to that question is directly related to each score’s reliability because the calculation of the SEM is based on reliability. In fact, the formula for the difference between two scores earned by an individual is calculated using the SEM of each score.

Applying this formula to T-scores, as shown in Fig. 3.5, we see that as the reliability goes down, the differences needed when comparing two scores increases dramatically. This means that scores from measures with reliability of .70 from two different teachers would have to differ by 15 T-score points to be significant at the 95 % level. This means that test scores with higher reliability reduce the influence of measurement error on the different scores. Clearly, in both research and clinical settings, variables with high reliability are needed for precision of measurement, but how are these coefficients evaluated?

Fig. 3.5
Relationship between reliability and the difference required for significance when comparing two T-scores
Evaluation of Reliability Coefficients
Bracken (1987) provided suggested levels for acceptable for test reliability. He stated that scales that comprise a complete instrument should have at least an internal reliability estimate of .80 or greater and total test scales should have an internal consistency of .90 or greater. These guidelines should be further considered in light of the decisions being made. For example, if a score is used for screening purposes where over identification is preferred to under identification, a .80 reliability standard for a Total Score may be acceptable. If, however, scores from a scale contribute to important decisions then a higher (e.g., .95) standard should be deemed more appropriate (Nunnally and Bernstein 1994). We suggest that professionals evaluating the treatment of symptoms related to ASD use scores that have internal reliability estimates of .80 or higher. For scores comprised of several variables that have been combined, an internal reliability estimates of .90 or greater. Clinicians are advised not to use measures that do not meet these standards because there will be too much error in the measurement to allow for confidence in the result. This is especially important because the decisions clinicians make can have significant impact on the life of a child. Therefore, we urge the reader to carefully examine the reliability findings of any measure they choose to use.
Implications
We have stressed the need for norm-referenced measurement of symptoms related to ASD as well as the advantages of using measures that have high reliability so that greater accuracy can be achieved. The overarching goal is to use well-developed psychological tools when assessing individuals with ASD and particularly when evaluating the effects of any treatment. We will now illustrate how these measurement issues can be operationalized with a rating scale. Although all the issues related to reliability are well established, the issues surrounding psychometrics of treatment effectiveness are much more complex. There are many questions and an evolving set of possible solutions. Typically, researchers have studied both global changes in symptoms associated with a particular condition as well as specific behaviors, some of which may or may not be associated with a particular condition. In clinical practice, treatment goals are rarely set at the disorder level, instead, the focus is typically on general symptoms (e.g., improve peer socialization) and specific behaviors associated with a general symptom (e.g., increase ability to initiate conversation with peers).
Treatment Evaluation Illustration
In this section, we present a way to evaluate symptoms related to ASD on both global and specific levels, identify areas for treatment, and evaluate the effects of treatment. To do so, we will illustrate using information from the ASRS (Goldstein and Naglieri 2009). We choose to illustrate using this tool because it is nationally normed and provides several different types of global scores as well as measures of specific behaviors. In addition, the reliability of the scales is well documented and guidelines for assessing treatment change are also provided. We will begin with a brief explanation of the ASRS and then describe the steps needed to determine the current status of the individual who was rated, which scales and individual behaviors warrant attention, and how to assess treatment effectiveness.
Autism Spectrum Rating Scale
The ASRS (Goldstein and Naglieri 2009) is a rating scale for assessing behaviors associated with ASD. Children aged 2–5 years (N of items = 70) and youth aged 6–18 years (N of items = 71) can be rated by parents and teachers. Each of the items is scaled using a 5-point Likert scale (0 = Never, 1 = Rarely, 2 = Occasionally, 3 = Frequently, 4 = Very Frequently) and scored so that higher scores are indicative of behaviors associated with ASD. Initial item generation was based on a comprehensive review of both current theory and literature on the assessment of ASDs (autistic disorder, Asperger’s disorder, and Pervasive Developmental Disorder, Not Otherwise Specified), the DSM-IV-TR (APA 1994), and ICD-10 (World Health Organization 2007) diagnostic criteria, as well as the authors’ clinical and research experiences. The ASRS scale structure includes three factorially defined scales (Social/Communication, Unusual Behaviors, Self-Regulation), eight content-derived Treatment Scales (Peer Socialization, Adult Socialization, Social/Emotional Reciprocity, Atypical Language, Stereotypy, Behavioral Rigidity, Sensory Sensitivity, Attention), a DSM-IV-TR Scale based on the DSM-IV-TR symptomatic criteria for autistic disorder and Asperger’s disorder, and a Total Score. The structure of the ASRS scales is shown in Fig. 3.6.

Fig. 3.6
Structure of the autism spectrum rating scale
The ASRS was normed using samples obtained from parents (N = 1,280) and teachers (N = 1,280). The normative samples closely match the US population according to age, sex, race/ethnicity, parental educational level (for parent raters), and geographic region. The ASRS internal reliability coefficients are summarized in Table 3.2. The median reliabilities for the Total Scale (.97) and the empirical scales (range from .92 to .94) are all high. The median reliabilities for the Treatment Scales range from .69 to .91, suggesting that some of these scale which were built according to the content of the items have higher reliability than others. This is important to recognize when comparing scores across raters or over time. There is considerable evidence about validity of the ASRS which can be found in the test manual (Goldstein and Naglieri 2009). The goal of this section was to provide a brief overview of the ASRS so that the sections which follow will be more easily understood.
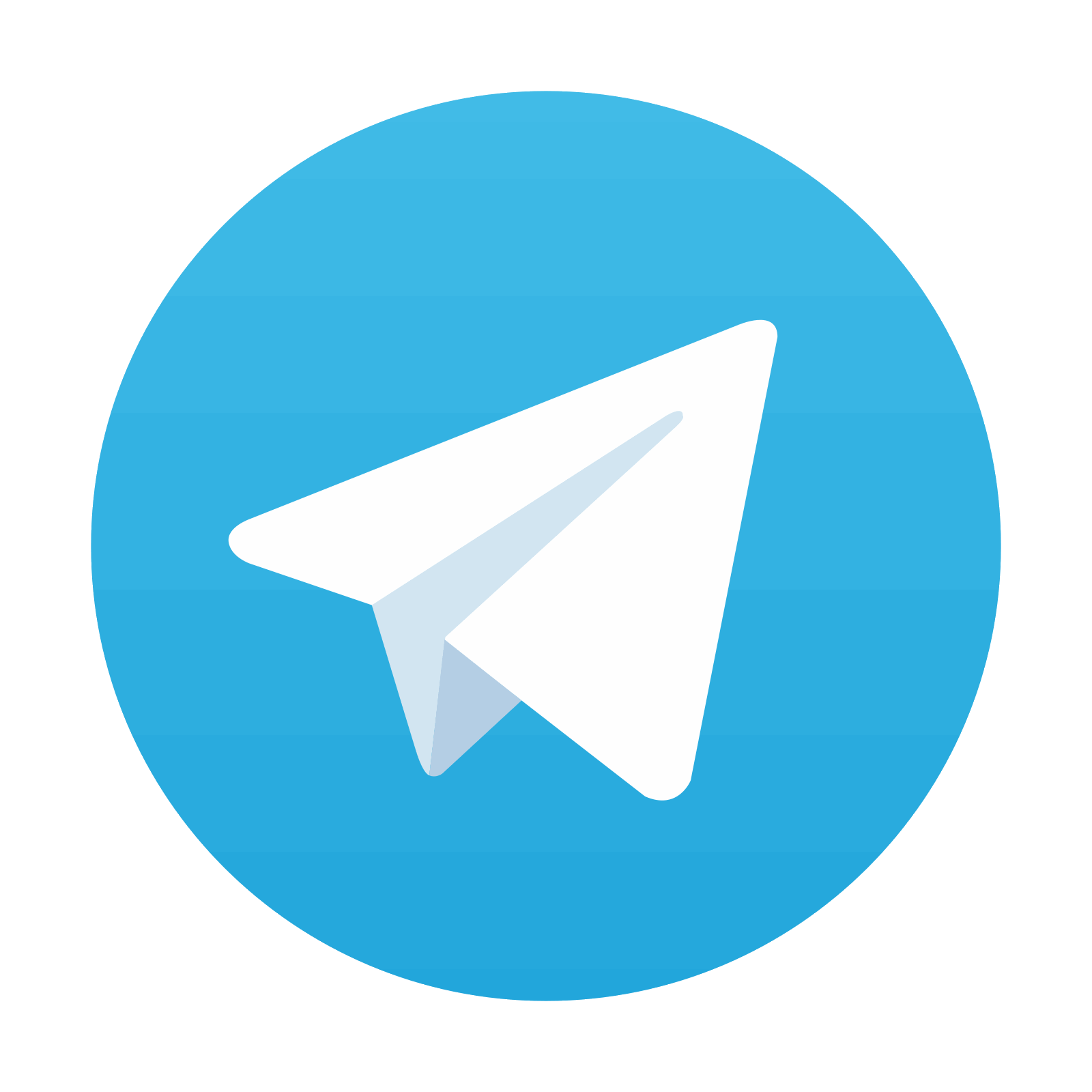
Stay updated, free articles. Join our Telegram channel

Full access? Get Clinical Tree
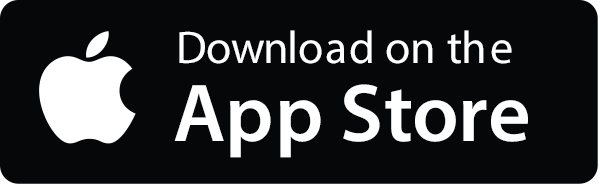
