Trait/disease
Family studiesa
National datab
GWAS hitsc
All GWAS SNPsd
Schizophrenia
0.81e
0.65f,g
0.02h,i
0.23j
Bipolar disorder
0.85k
0.60f,g
0.02i,l
0.22j
Major depression
0.37m
0.31g
0n
0.22j
Autism
0.75o
–
0.17j
ADHD
0.76p
–
0q
0.29j
1.
Overestimation of heritability from family studies.
2.
Underestimation of effect sizes of identified variants.
3.
Causal variants are rare so they are not in linkage disequilibrium (LD) with genotyped SNPs.
4.
Causal variants are common but their effect sizes are too small to achieve genome-wide significance.
We will consider these explanations in turn.
Explanation 1. Overestimation of Heritability from Family Studies
Heritability is estimated from partitioning phenotypic variance into genetic and non-genetic components (Visscher, Hill, & Wray, 2008). The genetic component can be further divided into additive and non-additive variations and the environmental component can be partitioned into shared environment and unique environment. However, sources are often unavoidably confounded in the experimental design, so that their contribution to the overall variance cannot be separated. For example, in the classical twin design of monozygotic (MZ) and dizygotic (DZ) twin pairs, there are only three essential statistics that can be estimated from their phenotypes, the MZ resemblance (for example, covariance or correlation), the DZ resemblance and the overall phenotypic variation in the sample. Therefore, only three variance components can be estimated, although many more genetic and non-genetic causal components of variance can be postulated to influence MZ and DZ resemblances (Lynch & Walsh, 1998). It is well recognised that estimates of heritability may be biased and that it is difficult to separate additive from non-additive components and as a result additive genetic variances may be overestimated. Since relatives with additive coefficient of relationship u have additive*additive coefficient of relationship u 2, one way to investigate evidence for non-additive variation is by checking consistency of estimates from different types of family relationships, i.e. genetic clones (monozygotic twins), first-degree relatives (parent–offspring and full-sibs, who differ in their coefficients of dominance relationship), second-degree relatives (half-sibs, grandparent–grandoffspring, avuncular). However, degree of relationship is often confounded with degree of shared environment and separation of these factors remains difficult. Moreover, more distant relationships are subject to greater sampling variance and achieving large enough sample sizes without biases is difficult. The national registries with known family relationships matched to hospital records provide an opportunity to achieve large sample sizes, although such data sets come with their own potential problems such as false linkages, potential non-paternities and diagnostic inaccuracies. Nonetheless, it is noteworthy that for schizophrenia (SCZ) and bipolar disorder (BPD) estimates are lower than traditionally reported from more carefully collated but smaller studies (Table 11.2), which from meta-analyses are 0.81 (95 % CI 0.73–0.90 (Sullivan et al., 2003)) for SCZ and 0.85 (95 % CI 0.73–0.93 (McGuffin et al., 2003)) for BPD. However, heritabilities estimated from Swedish (Lichtenstein et al., 2009) and Danish (Wray & Gottesman, 2012) national cohorts are SCZ: 0.64 (95 % CI 0.62–0.68) and 0.67 (95 % CI 0.64–0.71) and BPD: 0.59 (95 % CI 0.56–0.62) and 0.62 (95 % CI 0.58–0.65), respectively. These lower estimates may be more representative of the samples brought together for GWAS that necessarily combine cohorts with different collection protocols (e.g. diagnostic criteria and their local applications), and therefore reflect greater phenotypic variance than samples traditionally used in heritability estimation.
Table 11.2
Exploring frequency and effects size consistent with causal variants in LD with schizophrenia associated SNP rs2021722, risk allele frequency (RAF) 0.78 and odds ratio (OR) 1.15 (Ripke et al., 2011)
LD r 2 | Min RAF | Max RAF | OR @ min | OR @ max | V % |
---|---|---|---|---|---|
1.0 | 0.78 | 0.78 | 1.15 | 1.15 | 0.09 |
0.8 | 0.73 | 0.82 | 1.16 | 1.19 | 0.12 |
0.5 | 0.60 | 0.90 | 1.18 | 1.34 | 0.19 |
0.2 | 0.32 | 0.99 | 1.29 | >10 | 0.46 |
Lastly, it has been suggested that epigenetic mechanisms that play a pivotal in plasticity of gene expression may contribute to overestimation of heritability or missing heritability (Labrie, Pai, & Petronis, 2012). There is now robust evidence to suggest that some epigenetic marks are stably inherited despite the resetting of epigenetic marks during gametogenesis and early development. If the factors controlling the inherited epigenetic mechanisms act additively, then they would only contribute to missing heritability if inherited signatures were not tagged by common SNPs. While there is no doubt that overestimation of heritability may contribute to missing heritability for some traits and disorders, there is sufficient evidence (including from adoption studies (Bouchard, Lykken, McGue, Segal, & Tellegen, 1990)) to suggest that, even if overestimated, missing heritability would remain.
Explanation 2. Underestimation of Effect Sizes of Identified Variants
Since the experimental design of GWAS is that 80 % of the variation in the genome is represented by less than 10 % of the polymorphic sites, it is known, a priori, that identified associated variants have little chance of being the causal variants and are much more likely to be a variant in LD with them. To illustrate this point consider SNP rs2021722. This SNP explains the most variation in liability (0.09 %) out of all those reported in the Psychiatric Genomics Consortium GWAS mega-analysis for SCZ (Ripke et al., 2011). In Table 11.2 we list the range of risk allele frequencies and odds ratios consistent with causal variants in LD with the associated SNP. In reality, the most associated SNP is expected to be the one most closely correlated (in highest LD) with the unknown causal variant. Most likely the causal variant is in relatively high LD, in which case its frequency is similar to that of the associated SNP and the variance explained is only slightly more than estimated from the associated SNP. However, in some regions of the genome the LD coverage by genotyped SNPs could be less, so that if the causal variant is only in weak LD, its frequency could be quite different from the associated SNP and the variance explained would be higher for a causal variant with r 2 = 0.2 with the genotyped SNP, in this example fivefold higher. Despite the high-fold differences that can be inferred between effect sizes of associated and causal variants, the impact of underestimation is limited by that fact that very large effect sizes would already have been discovered in other experimental paradigms such as linkage.
Explanation 3. Causal Variants Are Rare so They Are Not in Linkage Disequilibrium with Genotyped SNPs
All evolutionary models imply a great excess of rare causal variants compared to more common variants (Eyre-Walker, 2010). Selection implies a negative correlation between effect size and allele frequency, but still the individual contribution to variation in the population made by the vast majority of rare variants may be expected to be negligible. For example, a variant of frequency 0.2 % and odds ratio 4 explains only 0.13 % of the variance in liability of a disease of lifetime risk of 1 %. Since the experimental design of GWAS is to genotype common variants, it is well recognised that variation represented by less common and rare variants is not represented since such variants cannot generate high r 2 LD with common variants (VanLiere & Rosenberg, 2008; Wray, 2005). However, the genome-wide SNP chips have allowed the study of large rare copy number variants (CNVs). Association of CNVs with SCZ, autism and ADHD have been reported (summarised in (Sullivan et al., 2012)), and more recently for early-onset BPD (Priebe et al., 2012) as well as a general increased burden of large (>500 kb) CNVs for SCZ (International Schizophrenia Consortium, 2008) and developmental delay (Cooper et al., 2011). The CNVs identified for ADHD and autism mostly are de novo mutations through exome sequencing of trios (probands and their parents), for which further research is usually required to attribute causality. De novo mutations are not shared by family members (except in monozygotic twins or if structural qualities of the parental genome confer increased probability of the same de novo mutation occurring in multiple offspring). Therefore, de novo mutations are unlikely to contribute to missing heritability as these cases would not contribute to estimates of heritability from family studies (since the contribution from de novo mutations in MZ twins is small (Gratten, Mowry, Visscher, & Wray, 2013)). The lack of reported CNVs for other disorders reflects, in part, that CNVs have not been studied (since large sample sizes are needed for comparison of rare events in cases and controls and early GWAS chips did not carry probes that allowed CNV detection). It is notable that for the most severe of psychopathologies, mental retardation, hundreds of rare mutations of high penetrance in a large number of genes have been identified, perhaps implying a severity spectrum correlated with the impact of individual genetic mutations.
It is likely that we have discovered all but a tiny proportion of less common and rare variants underlying psychiatric disorders. This is clear from the biologically implausible discontinuity in the frequency of associated risk alleles illustrated for SCZ in Fig. 11.1. The new generation of SNP chips will help identify associated variants with minor allele frequencies in the range 0.01–0.05. Whole exome and genome sequencing will allow identification of rarer variants, but only if sample sizes are large (Kiezun et al., 2012). Based on lessons from Mendelian disorders, it is likely that once causal genes are identified, many associated rare variants will be found in those genes. Rather than focussing on individual variants, sequencing studies will seek to identify genomic regions (e.g. genes or subunits of genes) that carry an increased load of rare mutations in cases than controls (Hoffmann, Marini, & Witte, 2010).
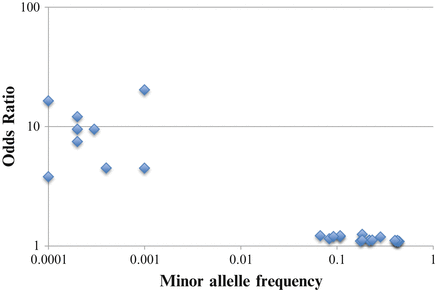
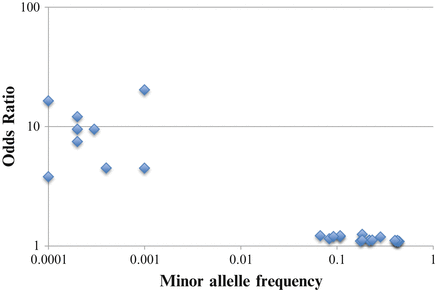
Fig. 11.1
Relationship between minor allele frequency and odds ratio of identified variants associated with schizophrenia shows evidence for missing variants in the spectrum
Explanation 4. Causal Variants Are Common but Their Effect Sizes Are Too Small to Achieve Genome-Wide Significance
The significance of association in GWAS is declared at a stringent threshold selected to ensure that variants taken forward into functional analysis are true positives. However, many studies show a great excess of associated variants at less stringent thresholds consistent with effect sizes too small to achieve genome-wide significance. This implies that with increasing experimental sample size more variants should be detected and this is supported by empirical data (Visscher, Brown, McCarthy, & Yang, 2012). New statistical methods that combine quantitative and population genetic concepts to allow better understanding of the nature of complex trait variation and evaluate the contribution to variance of common SNPs across the whole genome without identifying them individually have been developed (Deary et al., 2012; Lee et al., 2012; So, Li, & Sham, 2011; Yang et al., 2010; Yang, Lee, Goddard, & Visscher, 2011; Yang, Manolio et al., 2011). These methods use people unrelated in the conventional sense of the word, but given the finite global population size, share a proportion of their DNA by descent. The proportion of sharing between pairs of individuals can be estimated using genome-wide marker data, and that genomic similarity can be correlated with phenotypic similarity to estimate genetic variation (Lee et al., 2012; Lee, Wray, Goddard, & Visscher, 2011; Powell, Visscher & Goddard, 2010; Yang et al., 2010). By using distantly related individuals, the heritability tagged by common SNPs estimated from these methods should not be inflated by non-additive genetic effects (as the coefficients of non-additive relationships are so small) nor by shared (nuclear) family environment. The methodology has been extended to binary disease traits in which genetic variance attributable to SNPs is detected if case–case pairs and control–control pairs have higher genomic similarity than case–control pairs (Lee et al., 2011). When applied to quantitative traits and fitting ancestry principle components as covariates, confounding of genotype and trait in these analyses seems unlikely. However, inherent to case–control studies is a potential for artefacts to impact on genomic differences between case–controls. Stringent quality control (QC) of genomic data can minimise such artefacts but in some applications such stringent QC may remove real signal and, in others, may not be sufficient to remove biases, and the truth for any data set is unknown (Lee et al., 2013). Estimates from single cohorts (e.g. for SCZ (Lee et al., 2012), BPD (Lee et al., 2011) and major depressive disorder (MDD) (Lubke et al., 2012)) are often higher than estimates from combined cohorts (Cross Disorder Working Group of the Psychiatric GWAS Consortium et al., 2013). The use of bivariate models, in which cases and controls from one study represent the first trait and cases and controls from another study represent the second trait, allows estimation of a genetic covariance between the studies through the pairwise relationships of individuals across the two case–control sets. A positive genetic covariance is estimated if the cases of one trait are more closely related to the cases of the other trait (and also controls of one trait more related to the controls of the other trait), than cases of one trait are related to controls of the other trait. A negative covariance is estimated if the cases of one trait are more closely related to the controls of the other trait than they are to cases of the other trait. A zero covariance is estimated if there is no difference in the relationship between cases and controls in one trait with cases and controls in the other trait. Application of bivariate methods to case–control cohorts of the same disorder allow estimation of the genetic variance of the disorder as the genetic covariance tagged by SNPs estimated between studies and this should be free from artefactual biases, as it seems less likely that the same systematic differences apply to cases and controls from independently collected cohorts. The covariance is then a lower limit on the variance explained by SNPs. These methods have been applied to psychiatric disorders and between 17 and 28 % of the variance in liability are attributable to SNPs (last column Table 11.1) (Cross Disorder Working Group of the Psychiatric GWAS Consortium et al., 2013). The results imply that for psychiatric disorders as for other complex traits and diseases additional associated variants will be identified as sample sizes increase. These methods have also provided evidence for a shared genetic aetiology between SCZ, BPD and MDD, between SCZ and autism spectrum disorders and between MDD and ADHD.
Section Summary
Most likely, all four explanations listed above contribute to missing heritability. The variation tagged by common SNPs that have not individually achieved genome-wide significance has been termed the “hiding heritability” (Yang, Lee et al., 2011), and as shown in Table 11.1 (h 2 all GWAS SNPs) this is considerably greater than the variance explained by individually associated SNPs (h 2 all GWAS hits). For quantitative traits one third to one half of the heritability estimated from family studies has now been accounted for (Visscher, Brown et al., 2012). For the psychiatric disorders studies, less variance is explained, about one quarter to one third. This may be consistent with theories of mutation-selection balance theories for disease, but it is not yet possible to draw strong conclusions. The estimates of h 2 from genome-wide SNPs provide empirical support that investment in genotyping of larger samples will identify more individually associated common loci. Identification of a more complete portfolio of risk variants, risk genes and risk pathways is an important step in progressing the understanding of complex genetic disorders and in clinical translation of this knowledge. It is clear that causal variants have frequencies across the allelic spectrum and, in the absence of evidence for fully penetrant individually causal risk loci, many variants of small effect are likely to contribute to psychiatric disorders. The consequence of such a genetic architecture is that each individual could harbour a unique profile of risk alleles. Such a spectrum of risk profiles could be consistent with phenotypic symptom profiles. Understanding the genetic architecture of psychiatric disorders provides some boundaries on our understanding of the etiological complexity of these disorders. Applications of genomic medicine require this fundamental knowledge to progress fully. However, this understanding merely marks the end of the beginning, rather than the beginning of the end. The passing of the baton from the statistical geneticist to the molecular functional geneticist has, to date, been incomplete. The functional genetics community must contemplate studying variants that individually contribute little to the variation in the population. On the other hand, these are variants that do, indeed, contribute to variation in human populations and we would hypothesise that studies of these variants in the more controlled genetic backgrounds of model species will generate much larger effects at the functional level. As more variants of small effect are identified, the underlying metabolic pathways will become clearer, and time will tell if these are the same as the candidate pathways that have been long studied. The culminating results from the genomics era have the potential for generating a paradigm shift in our thinking.
Response to Treatment Genomics
While understanding and defining the genetic variants that contribute to risk of pathological disorders is an important step in the understanding of their complex aetiology, the road to translation is expected to be long. Direct investigation of the genetic contribution to the efficacy and adverse drug reactions (ADRs) of the current generation of drugs may impact the lives of those affected more quickly, particularly given pharmaceutical company reluctance to develop new drugs (Abbott, 2010). Individual differences in response to treatment contribute to the high failure rate of new drugs, which in turn has led to concerns about the future of the pharmaceutical industry (Munos, 2009), since although novel drugs may improve upon the current gold standard for some individuals, surpassing this standard for most may be unlikely. Data from clinical trials of new drugs for oncology show that only 3 % of drugs entering phase I trials reach market with 45 % failing because of toxicity and 46 % failing because of lack of efficacy (BIO industries analysis. Oncology clinical trials—Secrets of success, 2012). Failure rates of new drugs for psychiatric disorders may be even higher (Cressey, 2011). Recognising the contribution of genetic variation to these statistics has led to calls (Feero, Guttmacher, & Collins, 2010) that DNA should be collected and stored from those enrolled in clinical trials of drugs and devices, generating a resource to explore the contribution of genetic variants to toxicity and efficacy. The application of genetic technologies to the study of the effects of drugs in the patient population is termed pharmacogenetics. Continuation of treatment when patients do not experience a reduction in severity of symptoms or remission, delays the time to remission. Moreover, continued use of inefficacious drugs increases the chances of the patient experiencing harmful side effects or that symptoms worsen. The ability to predict which drug will be most effective for each individual patient would help to reduce time to remission and medical costs.
To illustrate the extent of the potential impact of identifying genetic variants that contribute to treatment response and ADRs for psychiatric disorders, consider the example of antipsychotics, particularly Clozapine. A large-scale trial called the Clinical Antipsychotic Trials of Intervention Effectiveness (CATIE) that was designed to compare the efficacy of five antipsychotics over 18 months showed that 74 % of patients discontinued their treatment prior to the end of the trial due to lack of efficacy of the treatment or significant side effects (Lieberman et al., 2005). One of the CATIE antipsychotics was Clozapine, widely accepted as the most efficacious antipsychotic, despite the development of other “atypical” antipsychotics (Citrome, 2011; Kane, 2011). Clozapine is significantly better at ameliorating symptoms than first-generation (Leucht et al., 2009) and other second-generation (Lewis et al., 2006) antipsychotics and has demonstrated superior efficacy for treatment-resistant SCZ (Essali, Al-Haj Haasan, Li, & Rathbone, 2009; Kane, Honigfeld, Singer, & Meltzer, 1988), a common clinical problem. In addition to its high response rate and unique efficacy, it possesses anti-suicidal, anti-aggression and anti-substance misuse properties (Farooq & Taylor, 2011). However, Clozapine’s affinity for several neurotransmitter receptors (Falkai et al., 2006) elicits a wide range and heavy burden of ADRs (Nielsen, Damkier, Lublin, & Taylor, 2011) (apart from extrapyramidal side effects which are generally improved (Flanagan, 2008)), more than other medications (Taylor, Douglas-Hall, Olofinjana, Whiskey, & Thomas, 2009), with a substantial percentage of patients (17 % (Young, Bowers, & Mazure, 1998) to 35 % (Taylor et al., 2009)) having to be withdrawn from Clozapine because of severe ADRs including those that can be grouped as haematological, cardiac, neurological/psychiatric and others. As a consequence of the ADRs, guidelines of professional bodies such as the Royal Australian and New Zealand College of Psychiatrists and the US National Institute for Health and Clinical Excellence state that treatment of Clozapine should only be initiated in patients who do not respond adequately to treatment of at least two different antipsychotics including one other second-generation antipsychotic. Once initiated, treatment with Clozapine must be accompanied by a demanding routine of clinical monitoring. Weekly blood samples are required during the first 18 weeks of treatment to monitor white blood cell counts (associated with neutropenia and agranulocytosis). This routine, in combination with unwanted side effects, leads to a high rate of non-compliance withdrawals (Pai & Vella, 2012). While Clozapine’s efficacy is not in doubt, its potential life-threatening ADRs significantly constrain and delay its use (Farooq & Taylor, 2011). Clinicians are often reluctant to commence Clozapine because of severe ADRs (Kane, 2011). Taylor, Young and Paton (2003), for example, reported that Clozapine prescription was delayed by 5 years compared with guideline recommendations.
Similar variation is seen in the treatment for other disorders. For example, the Sequenced Treatment Alternatives to Relieve Depression (STAR*D) study set up by the National Institutes of Mental Health in the USA showed that only 30 % of patients experienced remission after a 12-week treatment course with the antidepressant citalopram (Trivedi et al., 2006). A further 15 % of patients had a significant response as measured by a >50 % reduction in depression severity. No therapeutic benefit was obtained from a first course of antidepressants for more than half of the sample. Likewise, lithium has been used as a treatment in BPD for decades, yet the reasons as to why some patients respond and others do not, have not yet been elucidated (Schulze et al., 2010).
There have been few large twin and family studies conducted to investigate the heritability of drug response in psychiatric disorders. This is not surprising as such studies require large samples of family members to have undergone treatment. Those studies that have investigated genetic sources of variation invariably have shown familial clustering of response (Arranz & de Leon, 2007; Franchini, Serretti, Gasperini, & Smeraldi, 1998; Grof et al., 2002; Mata, Madoz, Arranz, Sham, & Murray, 2001) and side effects (Theisen et al., 2005), but notably also of symptom profiles (Korszun et al., 2004). Studies in other disorders provide a wealth of evidence that genetic variation plays a significant role in treatment response and there are several examples of polymorphisms explaining a significant amount of the variance in patient response. Owing to their large effects, these variants have not required large sample sizes to detect them in association studies. One of the first associations discovered was between the maintenance dose of the anticoagulant drug warfarin and polymorphisms in the vitamin K epoxide reductase complex I (VKORC1) gene, which is the active target of the drug which was discovered in a genome-wide associations study of 181 patients (Cooper et al., 2008). Further studies have identified polymorphisms in the CYP2C9 gene as also influencing dose response to the anticoagulant and increasing risk of haemorrhage (Takeuchi et al., 2009). In fact, an emerging principle from GWAS of treatment response in other medical fields is that effect sizes of variants associated with drug response are larger than those observed for complex disease phenotypes (Daly, 2012). Such results seem plausible since variants are unlikely to have been subject to selection pressure; thus, we might expect quite a different genetic architecture to underpin response traits compared to disease. For example, the HLA-B*57:01 allele is associated (risk allele frequency (RAF) = 5 %, odds ratio (OR) = 45) with drug-induced liver injury in the context of flucloxacillin, a relationship initially identified in a GWAS limited to only 51 cases (Daly et al., 2009). This allele is also a strong predictor of abacavir hypersensitivity in treatment for HIV (Hetherington et al., 2002). GWAS have also identified the association of the IL28B locus to the efficacy of anti-hepatitis C virus therapy (RAF = 75 %, homozygote OR = 7.1) (Ge et al., 2009) and the SLC01B1 locus to the toxicity of simvastatin (RAF 15 %, OR = 4.5) (Link et al., 2008).
Replicable genetic associations that explain a significant proportion of the variation in efficacy or side effects have been limited in pharmacogenetic studies in psychiatry. Before genome-wide association studies became feasible, a large number of candidate gene studies were initiated to investigate genetic variants in treatment response in major depression, bipolar and SCZ. Most of these studies have focused on polymorphisms in genes involved in the serotonin or dopamine metabolism pathways, as these are the site of action of many antipsychotics and antidepressants. Comprehensive reviews of the candidate gene literature have been undertaken for lithium response (Serretti & Drago, 2010; Smith, Evans, & Craddock, 2010), antipsychotic response and side effects (Zhang & Malhotra, 2011) and antidepressant response (Kato & Serretti, 2010). The most studied variants are the serotonin receptor short/long polymorphism (5HTTLPR) and a 48 bp tandem repeat in the DRD4 gene. While many studies have reported associations with candidate genes, in the case of lithium and antipsychotics, there have been no replicated findings. A meta-analysis of studies that evaluated response or intolerance to antidepressant treatment found that the 5HTTLPR-long allele, the BDNF 66Met variant and the TPH1 218C/C variant were all significantly associated with a positive response (Kato & Serretti, 2010). However, not all of the studies used the same study protocol. There were differences in the antidepressant used, the length of time it was prescribed for, the time that remission or response was evaluated, the criteria used to assess remission and response, the setting of the study (inpatients vs. outpatients), and the ethnicity of the participants. It is therefore difficult to draw inference on the meta-analysis results. Study design differences also create difficulties in assessing non-replication in lithium and other studies. Furthermore, most of the candidate gene studies were retrospective studies that genotyped participants long after treatment was administered. These studies could therefore not control for confounders such as non-compliance with the treatment regimen (Smith et al., 2010). Moreover, the sample sizes of most studies were too small to detect variants all but very large effects on response.
The weaknesses in the candidate gene studies have led to the establishment of a number of prospective studies to investigate variation in treatment response in which genome-wide genotypes have been obtained. The substantially larger sample sizes and consistent protocol for treatment and assessment of response in these studies offer hope of finding genetic variants influencing response. Genome-wide association studies have been performed in most of these studies. A summary of the results of the largest GWAS published to date in psychiatric pharmacogenetics is provided in Table 11.3. Several studies identified variants influencing response at genome-wide significance in spite of relatively small sample sizes. None of these findings has been replicated in an independent sample (through lack of availability) and hence should be considered preliminary findings. It is also worth noting that the findings from different studies did not converge, with no two studies highlighting the same gene or metabolic pathway. These differences could be explained by variation between studies in the type of drugs used, the length of treatment, the assessment of response or other confounders. Even within studies, the dose administered was not consistent across patients, reflecting professional opinion of individual needs.
Table 11.3
Summary of genome-wide association studies for response to treatment of mood and psychotic disorders
Disorder/symptoms | Cohort | Sample | Response measure | Drugs | Array | Min p-value | Gene(s) implicated | Reference |
---|---|---|---|---|---|---|---|---|
Response to antidepressants in MDD patients | Munich antidepressant response signature (MARS) + replication in STAR*D | 339 inpatients, replication 361 inpatients, replication 832 outpatients | Reduction in Hamilton depression rating | Multiple | Illumina HumanHap 300 | 7.6 × 10−7 | CDH17 | Ising et al. (2009) |
Response to antidepressants in MDD patients | STAR*D | 883 responders, 608 non-responders | Reduction in quick inventory of depressive symptomatology | Citalopram | Affymetrix 500 K | 4.65 × 10−7 | UBE3C | Garriock et al. (2010) |
Response to antidepressants in MDD patients | Genome-based therapeutic drugs for depression (GENDEP) | 706 individuals | Reduction in Montgomery–Asberg depression rating scale | Escitalopram, nortriptyline | Illumina 610-quad | 3.82 × 10−7 | Intergenic, IL11 | Uher et al. (2010) |
Response to lithium treatment in bipolar disorder | Systematic treatment enhancement programme for bipolar disorder (STEP-BD) + replication in University College London sample | 1,177 individuals + 359 replication | 2 or less symptoms DSM-IV mood episode | Lithium | Affymetrix 500 K | 5.5 × 10−7 | GLURB | Perlis et al. (2009) |
Response to antipsychotics in schizophrenia patients | CATIE | 738 patients | Reduction in positive and negative symptoms scale | Olanzapine, perphenazine, quetiapine, risperidone, ziprasidone | Affymetrix 500 K | 9.8 × 10−8 | Intergenic, ANKS1B, CNTNAP5 | McClay et al. (2011) |
Extrapyramidal symptoms caused by antipsychotics | CATIE | 738 patients | Simpson–Angus scale and abnormal involuntary movement scale | Olanzapine, perphenazine, quetiapine, risperidone, ziprasidone | Affymetrix 500 K | 1.2 × 10−10 | Intergenic, ZNF202 | Aberg et al. (2010) |
Metabolic side effects of antipsychotics | CATIE | 738 patients | 12 Indicators of metabolic side effects | Olanzapine, perphenazine, quetiapine, risperidone, ziprasidone | Affymetrix 500 K | 1.2 × 10−8 | MEIS2, GPR98 | Adkins et al. (2011) |
Response to antipsychotics in schizophrenia patients | Clinical trial of iloperidone | 407 patients—210 given iloperidone | Reduction in positive and negative symptoms scale | Iloperidone | Affymetrix 500 K | 2 × 10−5 | NPAS3 | Lavedan et al. (2009)
![]() Stay updated, free articles. Join our Telegram channel![]() Full access? Get Clinical Tree![]() ![]() ![]() |