SNP ID
Locus name
Chromosome (bp)
Odds ratio
rs35749011
GBA/SYT11
1 (155,135,036)
1.824
rs114138760a
GBA/SYT12
1 (154,898,185)
1.519
rs823118
RAB7L1/NUCKS1
1 (205,723,572)
1.122
rs10797576
SIPA1L2
1 (232,664,611)
1.131
rs6430538
ACMSD/TMEM163
2 (135,539,967)
0.875
rs1474055
STK39
2 (169,110,394)
1.214
rs12637471
MCCC1
3 (182,762,437)
0.842
rs34311866
TMEM175/GAK/DGKQ
4 (951,947)
0.786
rs34884217a
TMEM175/GAK/DGKQ
4 (944,210)
1.232
rs11724635
BST1
4 (15,737,101)
1.126
rs6812193
FAM47E/SCARB2
4 (77,198,986)
0.907
rs356182
SNCA
4 (90,626,111)
0.760
rs7681154a
SNCA
4 (90,763,703)
0.854
rs9275326
HLA-DQB1
6 (32,666,660)
0.826
rs13201101a
HLA-DQB1
6 (32,343,604)
1.185
rs199347
GPNMB
7 (23,293,746)
1.110
rs591323
FGF20
8 (16,697,091)
0.916
rs117896735
INPP5F
10 (121,536,327)
1.624
rs329648
MIR4697
11 (133,765,367)
1.105
rs76904798
LRRK2
12 (40,614,434)
1.155
rs11060180
CCDC62
12 (123,303,586)
1.105
rs11158026
GCH1
14 (55,348,869)
0.904
rs2414739
VPS13C
15 (61,994,134)
1.113
rs14235
BCKDK/ STX1B
16 (31,121,793)
1.103
rs11868035
SREBF/RAI1
17 (17,715,101)
0.939
rs17649553
MAPT
17 (43,994,648)
0.769
rs12456492
RIT2
18 (40,673,380)
0.904
rs8118008a
DDRGK1
20 (3,168,166)
1.111
As with most GWA, the effect sizes identified in this recent GWA are in the range of an odds ratio of ~1.3 per allele (the odds ratio at a locus may change considerably once the effect allele is determined). A common question that is levied is “what is the use of identifying low-risk alleles.” There are two aspects of this criticism, and these are both worth consideration: what is the value of these alleles in the context of understanding risk for disease, and what can these loci tell us about the etiology of disease? As noted above, the individual risk conferred by these alleles is relatively modest; however, on a population level, because the alleles are so common, they contribute far more to the risk of PD than, for example, synuclein, parkin, pink1, and dj1 mutations combined. Also, the risk attributed to these alleles is cumulative; thus, they can be combined, and for any individual, it is possible to generate a risk score based on their possession of multiple risk alleles. When this type of risk profile is performed, we can then rank individuals in a population based on the amount of total known genetic risk they possess. If we then look at those individuals with the highest 20 % of genetic risk, and compare them to the group with the lowest 20 % of risk, the former group are about 4 times as likely to get PD. This, by any measure, is a substantive amount of risk. The second aspect of this criticism, that low risk signifies low etiologic importance, is a logical fallacy. The odds ratio tells us about the risk conferred by an allele, and when we have confidence in this signal, we can be sure that this allele is altering the regulation of a gene and that this gene is involved in the disease process. The effect size tells us nothing about the importance of this gene in the disease process. Again, we will provide an illustrative example of this point later, in the description of work that represents a major step forward in PD research and which leveraged GWA results.
So in the context of GWA, is there an appetite for further studies? It is likely that a substantive increase in sample size will continue to identify additional risk loci, and this is certainly an approach that has been successfully applied to diseases such as type II diabetes and schizophrenia, where risk loci number in the hundreds. There are two primary challenges to taking such a route in PD; first significant advances are likely to require substantive increases in sample size, certainly more than double the size of current studies. Collecting these samples, both for discovery and replication stages, represents a significant challenge. Unlike (e.g., type II diabetes), hundreds of thousands of DNA samples from PD patients are not currently available. Second, because the sample size increase will need to be so large, the financial cost associated with sample collection and genotyping is extremely large. It is unlikely that any single funding body will support such an endeavor outside of a large philanthropic donation.
Outside of expanding current genotyping, there are several approaches that may be used to garner more information from the current datasets. In perhaps the most simple, the current GWA data can be mined more deeply, taking forward alleles that did not meet the stringent level of genome-wide significance but that were suggestive in nature. Additional typing of these in replication series is warranted and is certainly ongoing. This represents a fairly cost-efficient approach to wringing more from the current data; however, this approach alone will not identify all of the common risk alleles associated with disease.
Beyond Simple Association for Common Variants
There are many things outside of simple association that can be accomplished with dense genotype data of the type produced by GWA studies. One approach that has become increasingly popular over the last 2 or 3 years is the use of GWA data to estimate the heritable component of disease [29, 69]. The method GCTA (genome-wide complex trait analysis) estimates the proportion of phenotypic variance explained by common SNPs for complex traits, including disease. This is essentially achieved by estimating the degree of genetic relationship between individuals at a very fine genetic scale and comparing this metric between cases and controls, effectively determining how much more cases are related to each other than controls. This method has been applied to PD and the results are revealing. First, this work establishes an autosome-wide heritable component of PD of 0.3 [25]. While this is a substantial number, it is worth noting that this is likely an underestimate of the total heritable component of PD and certainly an underestimate of the total genetic component of this disease. The underestimate of the heritable component is because this method does not effectively capture the impact of very rare variants; a large number of individually rare mutations could account for a substantive proportion of the heritability of PD, but would not be reflected by this method. The genetic component of this disease would also not be completely reflected by this method, as it fails to capture the contribution of de novo mutations to disease. Thus the true heritable component is likely to be higher than 0.3 and the genetic component certainly so. Another notable aspect of this GCTA work in PD was a calculation of the proportion of the identified heritable component where the gene or locus is already known. The authors showed that when they repeated the analysis including only the genetic regions already associated with disease, including those identified by GWA and those identified by linkage and positional cloning, the heritable component was ~0.03 [25]. This data suggests that although a large number of loci and genes have been identified, only ~10 % of the heritable component of the disease has thus far been explained; there is much more to find.
In addition to a straightforward approach of association testing, there have been a number of other attempts to garner more etiologic understanding from GWA data. A fairly popular approach centers on pathway-based analysis. In this general scheme, investigators take significant SNPs (and here, significance may be defined quite loosely) and look for a pattern in the associated genes. This can be performed using data from existing pathway-based databases, for example, the Kyoto Encyclopedia of Genes and Genomes (KEGG; http://www.genome.jp/kegg/), and looking for an enrichment of genes associated with the GWA signals in a particular functional group of genes. Broadly, this is the type of approach that was used by Holmans and colleagues, which suggested an enrichment of immune-related genes within the PD identified loci [21]. While this type of pathway-based approach is not ideally suited to identifying individual risk alleles/genes with confidence, it does have the benefit of implicating functional networks/pathways in the disease process and thus may broadly indicate therapeutic opportunities.
As discussed above, the net effect of risk allele burden for each individual can be calculated using genetic risk profiling to give an estimate of an individuals cumulative known genetic risk. It is unlikely that this genetic risk profile alone will be sufficient to predict disease likelihood, onset, or course. It is, however, likely that this risk profile will be a critical component of a multifactorial disease prediction model that may include other easily accessible phenotypic and biological markers. Thus, the development of programs such as the Parkinson’s Progression Markers Initiative (http://www.ppmi-info.org) and the Parkinson’s Disease Biomarkers Project (https://pdbp.ninds.nih.gov) has included a substantive genetic component. It is also worth considering that, as we move from risk loci, to identifying the actual biologically relevant risk allele, the effect size at these loci will increase, in some instances substantially; this advance will have a significant impact on risk prediction models.
Next Approaches
A much-lauded tool in our efforts to understand the genetic risk underlying complex disease is second-generation sequencing (also called next-generation sequencing or massively parallel sequencing). This method allows the production of extremely large-scale nucleotide sequence data, of sufficient size to sequence whole genomes or whole exomes. Whole-exome sequencing is a method that centers on taking a genomic DNA sample and enriching that sample for the protein-coding regions of the genome, which represent about 1 % of the total genome or ~30 million base pairs of sequence. This exon-enriched “exome” sample can then be readily sequenced revealing both common and rare variants within an individual’s genetic makeup. Thus, this method has been suggested as an answer to find the missing heritability of complex disorders, which is believed in part to exist in the space of rare protein-coding variants. One would expect that, given the variants are individually rare, sample sizes for simple association would need to be extremely large, likely in the tens of thousands. This high bar can perhaps be lowered somewhat by assessing the gene as the unit of association, rather than the variant, for example, by assessing the cumulative number of rare variants in a gene in cases compared to controls. There are several proposed approaches for this type of gene burden test, but no consensus as to the best approach has been established to date. While large unbiased “exome-wide” sequencing efforts have not yet borne fruit in the search for rare risk variants in PD, some success has been had in Alzheimer’s disease, for example, with the identification of TREM2 mutations as moderate-risk alleles [17, 24].
Exome sequence offers speed, reduced cost per individual, and to a certain extent reduced data storage and analytical burden, when compared to whole-genome sequencing. It is inevitable that the field will turn toward whole-genome sequencing as the discovery engine for genetics in complex disease and traits. In many ways, the sample preparation is more simple, and of course more can be gleaned from the data, not only because the whole-genome sequence is generated rather than just the protein-coding exons but also because it is less challenging to analyze structural genomic variants in whole-genome data. While the current cost remains high, at ~ $1,200 for a mid-coverage genome sequence, this is a somewhat accessible price point, and it is to be expected that for many diseases and cohorts, population level genome sequence data will be produced. There are currently no public genome sequencing projects of a large scale in PD at the time of writing, but this will likely change over the next few years.
The Challenge of Understanding Risk
As outlined above, GWA has been very successful in identifying risk loci, with 28 independent risk loci identified to date for PD [38]. It is important to note though that GWA identifies loci, not genes. There are two immediate challenges that follow from the identification of a risk locus: determining the variant at that locus that is mediating the biological effect and establishing which gene is being altered by that locus and how it is being influenced. As with most disease, this effort in PD is still in fairly early days. There are some loci where the likely gene is immediately apparent, for example, it is highly likely (though not 100 % proven) that the risk variants at the SNCA and LRRK2 loci exert their effects through SNCA and LRRK2 rather than some other proximal gene. For the majority of loci such a candidate is not immediately apparent. A common approach to identify the likely effector gene is the use of expression quantitative trait locus mapping (eQTL) [19]. For the most part, GWA signals are not linked to protein-coding variants, and thus it is clear that these risk alleles must be exerting their effect through transcript expression (here expression is defined quite broadly to include transcript splicing, half-life of the transcript, basal amount of the transcript, or induced transcript levels). Several reference sets have been devised that compile genetic data and gene expression data and correlate individual variants with levels of proximally encoded transcripts. Within our own laboratory, we have generated such a set in human brain tissue, and this resource allows us to interrogate risk variants from PD GWA for their association with gene expression levels [15, 19]. Within PD GWA loci, several expression QTLs have been identified, and some of these make biologic sense, for example, the demonstration that PD risk alleles at SNCA appear to increase SNCA expression fits entirely with our notion of increasing SNCA and increasing risk [52, 53]. For the majority of loci, however, an eQTL is not apparent, and even for those where an eQTL exists, association does not imply causation; the transcript could be correlated with genotype by chance, or it could be biologically related to the genotype but irrelevant to the disease process. Clearly, careful functional characterization of proteins encoded by genes within GWA loci is an essential next step in our understanding of the disease process.
One such study was recently performed which sheds some light on the pathologically relevant proteins at two GWA loci. Beilina and colleagues performed a screen that looked for protein interactors of Lrrk2 [3]. The initial screening phase of this effort identified a large number of potential protein interactors with Lrrk2 from a screened pool of ~10,000. The large number of positive hits was too great to take forward through the essential steps of reductionist validation; however, a comparison of these interactor data with GWA data from PD revealed that two of the key proteins were under GWA peaks. This group went on to show that the proteins Rab7L1 and Gak form a complex with Lrrk2, the former of which had been independently shown previously [33]. We predict that the application of unbiased high-content screening methods integrated with the results from broad-scale genetics efforts such as GWA will be the initial key to the identification of proteins critical to the pathogenesis of PD; this work, in our opinion, should be a priority for the field.
Identifying the biologically relevant variants within GWA is challenging. Because genomic regions are inherited in chunks even within a population, extensive linkage disequilibrium exists in the human genome. Thus, it is extremely difficult to separate the true disease-related variant from those variants that are benign and coinherited with the causal variant because they are physically close. Again, we believe that success in this regard will rely on a combination of dense genetic data and functional efforts. One key observation that has been made within PD is that of the pleomorphic risk locus (PRL); this posits that for some genes, many types of disease-related genetic variants will exist, including mutations that cause disease, common noncoding variants that impart risk for disease, and coding variants that impart risk for disease [54]. Within PD thus far the phenomenon of PRL seems to be true for SNCA, LRRK2, and perhaps also GBA. It is reasonable to suggest therefore that some of the GWA-identified loci will contain genes that not only harbor common risk variants but that will also contain rare disease causing mutations or rare risk variants. In this regard resequencing of GWA-identified loci in many thousands of samples would be predicted to provide data for fine mapping of the risk signal and may also identify rare causal mutations, which in effect would both nominate the disease-related gene within the locus and expand the known risk architecture. While this approach is likely being pursued by many laboratories, no systematic investigation of GWA loci through extensive resequencing has been published to date.
Another important step in understanding the causal variant and its effect involves overlaying functional mapping data. The EnCODE project (Encyclopedia Of DNA Elements) is a decade long effort that aims to identify all functional elements in the human genome sequence (http://www.genome.gov/encode/). These functional elements are mapped using a variety of approaches including the mapping of histone modifications, transcription start points, and transcription factor binding sites, each performed in a variety of cell types (for more information, see http://www.genome.gov/26524238). Thus, the data identifying key functional elements can be overlaid on risk SNPs with GWA loci in an attempt to identifying the key DNA element and the critical nucleotide. This approach has been used successfully to both identify the critical variant and the gene effected, although not yet in PD [55]. Again, we believe using this type of data, and indeed creating a catalog of functional elements in PD-related cell types, is a critical need for the field.
Conclusion
The past 10 years has been an incredible period of discovery for Parkinson’s disease genetics, particularly in the context of understanding the architecture of genetic risk in this complex disease. Many challenges remain, the most apparent of which is the application of methods to identify the remaining risk loci and determining the effector allele responsible for mediating this risk. The successful translation of this knowledge into an understanding of the etiology and pathogenesis of PD presents new challenges to both our paradigm of disease etiology and to the traditional framework of disease gene investigation. With these challenges lie substantial opportunity however, and this opportunity lies not only in understanding etiology but also in the investigation of genetics in other areas such as prognosis, onset, subtyping, and response to treatment. We predict that genetics will continue to serve as the foundation of our investigation into PD but that progress will rely on extensive collaboration and the integration of disparate data types.
References
1.
1000 Genomes Project Consortium, Abecasis GR, Altshuler D, Auton A, Brooks LD, Durbin RM, Gibbs RA, Hurles ME, McVean GA. A map of human genome variation from population-scale sequencing. Nature. 2010;467(7319):1061–73. doi:10.1038/nature09534.CrossRef
2.
Aharon-Peretz J, Rosenbaum H, Gershoni-Baruch R. Mutations in the glucocerebrosidase gene and Parkinson’s disease in Ashkenazi Jews. N Engl J Med. 2004;351(19):1972–7. doi:10.1056/NEJMoa033277.CrossRefPubMed
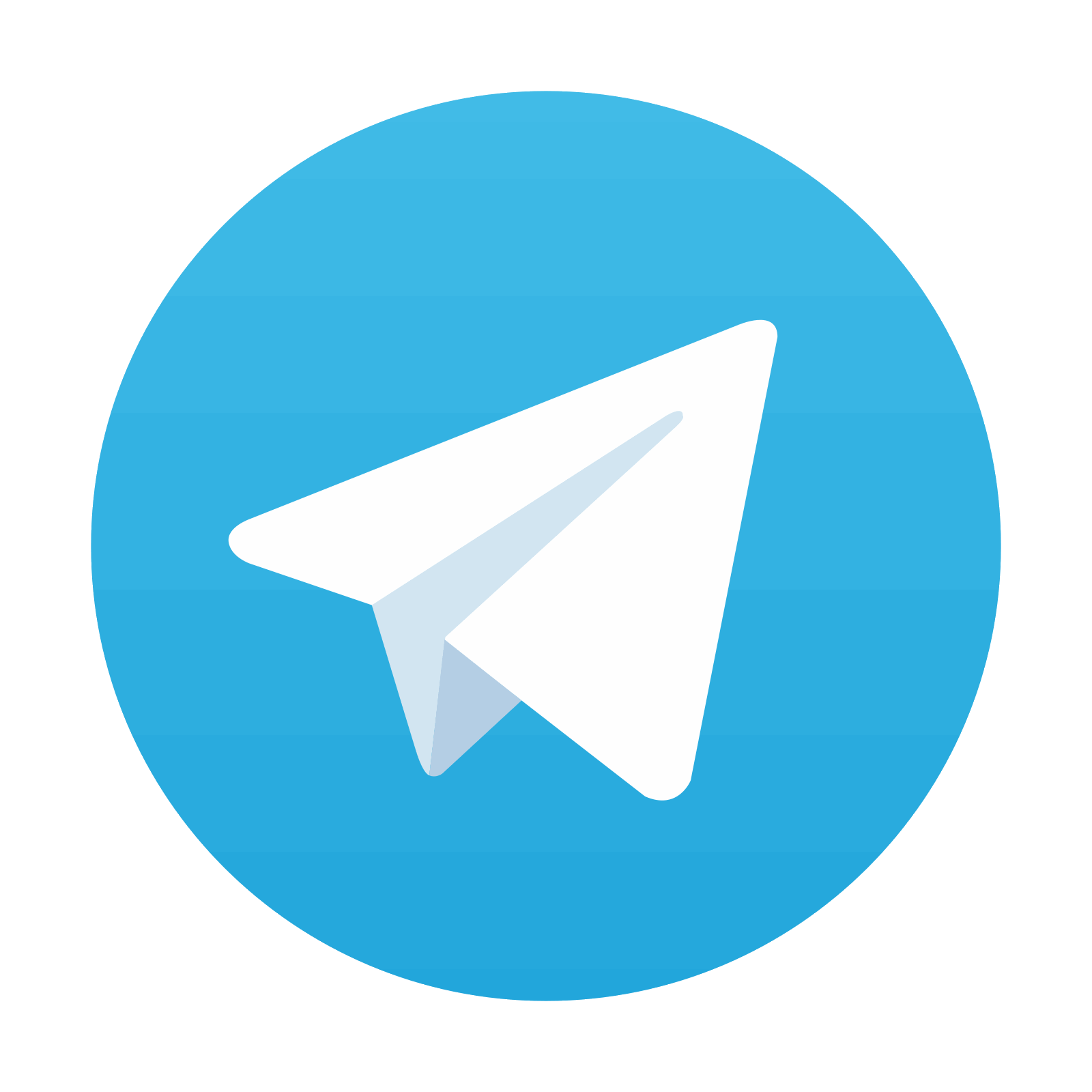
Stay updated, free articles. Join our Telegram channel

Full access? Get Clinical Tree
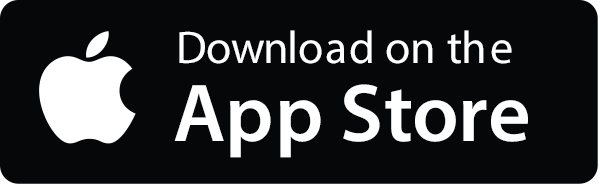
