DSM-5
DSM-IV
Failure to fulfill major role obligations
Y
Y (abuse)
Recurring use in hazardous situations
Y
Y (abuse)
Use despite interpersonal problems
Y
Y (abuse)
Use despite recurring legal problems
N
Y (abuse)
Tolerance
Y
Y (dependence)
Withdrawal
Y
Y (dependence)
Using more or longer than intended
Y
Y (dependence)
Giving up important activities to use
Y
Y (dependence)
Spending a lot of time using
Y
Y (dependence)
Use despite recurring physical/psychological problems
Y
Y (dependence)
Persistent, failed quit attempts
Y
Y (dependence)
Craving—strong urge or desire to use drug
Y
N
DSM–IV
Abuse—1 or more of 4 criteria
Dependence—3 or more of 7 criteria occurring in the same 12-month period
Substance use disorder
Unaffected—0 or 1 of 11 criteria
Mild affected—2 to 3 of 11 criteria occurring in same 12-month period
Moderate affected—4 to 5 of 11 criteria occurring in same 12-month period
Severely affected—6 or more of 11 criteria occurring in same 12-month period
Substance use disorders are also commonly diagnosed under the subheading of Mental and Behavioral Disorders using the International Statistical Classification of Diseases and Related Health Problems (most recently, ICD-10) (World Health Organization, 2007). The ICD-10 dependence syndrome (or drug addiction) is quite similar to the dependence criteria outlined by the DSM; however, the current ICD-10 classification of dependence already includes craving. Also in contrast, abuse or harmful use is codified somewhat differently, being largely limited to damage to physical or mental health due to substance involvement. An independent category also allows for the diagnosis of abuse of non-dependence-producing substances, such as laxatives, antacids, folk or herbal remedies, or over-the-counter analgesics. This category is more indicative of harmful use (i.e., use despite health problems) but is not accompanied by the hallmark features of dependence, such as withdrawal.
Addictive Substances
Alcohol, tobacco, cannabis, cocaine, and opiates are amongst the most recognized psychoactive substances with potential for misuse. These substances are also most commonly studied from a genetic perspective. Other psychoactive substances that produce addiction syndromes do exist and are commonly misused. For instance, the nonmedical use and misuse of prescription drugs, particularly analgesics, tranquilizers, sedatives, and amphetamines/stimulants, continues to be a public health problem. Use of designer drugs, such as ecstasy (3,4-methylenedioxymethamphetamine), appears to be on the rise in the US teenagers and is a source of particular concern (Johnston, O’Malley, Bachman, & Schulenberg, 2011). Hallucinogens, including the recently popularized, naturally occurring psychoactive substance Salvia (Perron et al., 2012), are also known to be addictive. Other substances that bear potential for misuse include steroids, diet pills, inhalants (e.g., paints, glue), and synthetic preparations of naturally occurring substances (e.g., synthetic marijuana, such as Kronic, K2) (Vandrey, Dunn, Fry, & Girling, 2012). In addition to the illicit use of these drugs, caffeine also bears the potential of being used excessively and producing caffeine intoxication, which was diagnosed in DSM-IV as excess consumption producing clinically significant impairment (American Psychiatric Association, 1994b). Caffeine Use Disorder appears in Section III of DSM-5 (i.e. measure meriting further research). There is growing interest and progress in the study of these substances and their addictive potential and genetic underpinnings.
Addictive or Abuse Potential
Some substances are considered more addictive than others. The likelihood that someone who experiments with a drug will become dependent on it is referred to as its addictive or abuse potential. For instance, not everyone who drinks alcohol develops an alcohol use disorder. In the 2010 National Survey of Drug Use and Health (NSDUH) (Substance Abuse & Mental Health Services Administration [SAMHSA], 2011), while only 2.6 % of cannabis users met criteria for cannabis dependence in the past 12 months, 8 % of those who used heroin were diagnosed with heroin dependence. Thus, it is often argued that heroin has a higher addictive potential than cannabis (Nutt, King, Saulsbury, & Blakemore, 2007). The addictive potential of a drug depends on multiple factors that can be broadly defined as drug specific and individual specific. Drug-specific factors include (a) the biochemical and neurochemical action of the drug that facilitates addiction, including its pharmacokinetic and pharmacodynamic properties, (b) dosage to achieve desired effect and its route of administration, and (c) potential for harm, including development of problems and difficulty ceasing use (e.g., severe withdrawal symptom), its availability, price, and other policies surrounding its legality or taxation (Nutt et al., 2007). Drug-specific addictive potential is typically computed by regulatory agencies using multiple criteria, including the controversial scheduling of drugs. For example, in the USA, the Controlled Substances Act (United States Department of Justice, D. E. A., 2012) classifies Schedule I drugs as those with a high addictive potential, with no known medical use in treatment and with a lack of accepted safety in administration; cannabis and heroin, for example, are classified as a Schedule I controlled substances. However, research often diverges from these regulatory classification schemes. For instance, using a complex, research-based classification system that included harm to self and others, an independent panel of scientists in the UK found that heroin was significantly more harmful to self than other drugs while alcohol was classified as the drug most harmful to others, even more so than heroin (Nutt et al., 2007).
Individual-specific factors are equally important and include (a) individual drug use patterns and (b) genetic and environmental vulnerability to repeated and excessive use. This chapter focuses on the important role of these individual factors on the etiology of substance use and substance use disorders.
Current Issues
Evidence for Genetic Influences
Family Studies of Substance Use Disorder
The first evidence for the potential genetic underpinnings of SUDs comes from a number of large-scale family studies that support the notion that SUDs “run in families.” These family studies are typically designed around a proband—an individual with substance-related problems or an SUD who is initially identified and via whom the entire family is ascertained into the study. Using alcoholic probands, studies such as the Collaborative Study of the Genetics of Alcoholism find that rates of alcohol dependence are considerably elevated in siblings of probands (50 % of male siblings and 22–25 % of female siblings) when compared with the siblings of non-proband control individuals (20 % of male and 9 % of female siblings) (Begleiter et al., 1995; Bierut et al., 1998). That alcohol use disorders are more common in brothers, sisters, as well as parents and offspring of probands indicates a familial basis for alcoholism. Bierut and colleagues (Bierut et al., 1998), with these data, also reported elevated rates of habitual smoking, cannabis, and cocaine dependence in relatives of alcoholic probands. For example, 47 % of male probands relative to 10 % of control individuals, met criteria for cannabis dependence, suggesting comorbidity between alcohol and cannabis dependence. In addition, rates of cannabis dependence were considerably elevated in male (and female) relatives of probands (29 %) when compared with male (and female) relatives of control individuals (11 %). This elevated vulnerability to drug dependence in relatives of probands indicates familial co-aggregation (or that alcohol and cannabis dependence run together in families) of SUDs. Similarly, with data on 149 probands with drug dependence (87 probands with opioid, 27 probands with cocaine, and 35 probands with cannabis dependence), 89 probands with alcohol dependence, and 61 control probands with no evidence of a lifetime history of any diagnosis in DSM–III–R, Merikangas, Stolar et al. (1998) examined the familial basis for alcohol and drug dependence. Examining 1,267 first-degree relatives, primarily composed of parents and siblings (42 % parents, 51 % siblings, and 7 % offspring), these authors found that relatives of probands with drug and alcohol dependence were more likely to report drug and alcohol (9.1 % vs. 3.1 % in relatives of controls), drug only (9.1 % vs. 0.8 %), and alcohol only (15 % vs. 11.8 %) dependence, while in probands with alcohol dependence alone, the most elevated risk of psychopathology in the relatives was alcohol dependence alone (27.7 %). These results, while consistent with the study by Bierut et al., did suggest the possibility that certain forms of alcohol dependence (that occur in the absence of co-occurring drug problems) may “breed true” and not elevate risk for drug problems in relatives, indicating familial specificity.
These studies provided some of the earliest evidence that familial factors that influence vulnerability to alcohol dependence may also affect liability to problems with and dependence on other drugs. The principal limitation of such family studies is that they cannot, without information from extended pedigrees, disentangle the extent to which this familial clustering is due to genetic factors versus shared family environment. It is well known, for instance, that in addition to genetic factors that are shared, to varying degrees, by members of a family, there are also environmental factors, such as religious affiliation and cultural context that are also common to families. Disentangling these effects from each other can be challenging in family studies, so adoption studies provide one possible avenue for delineating the extent to which familial environment and genetic transmission influence SUDs.
Can Adoption Studies Inform the Genetics of Substance Use Disorder?
The premise of an adoption study is straightforward yet elegant. It involves the similarities between the adopted individual (adoptee) with (a) their biological relatives (parents or siblings) and (b) their adoptive family (parents or siblings). Under a series of simplifying assumptions, any correlation with biological relatives is ascribed to genetic sources while any similarities with the adoptive family are attributed to rearing environment. Therefore, for disorders such as schizophrenia that have a strong genetic basis, it is expected that correlations between adoptees and their biological family will be particularly strong (Gejman, Sanders, & Kendler, 2011). On the other hand, for SUDs, there is accumulating evidence that both the biological and the adoptive family play important roles. One of the first adoption studies that looked at 55 male adoptees found higher rates of drinking problems in those with one biological parent who had a history of alcoholism, supporting the role of genetic vulnerability in alcoholism (Goodwin, Schulsinger, Hermansen, Guze, & Winokur, 1973). Later studies, though, have identified important effects of the adoptive environment. In one of the earliest adoption studies (termed a cross-fostering study), Cloninger, Bohman, and Sigvardsson (1981) found that temperance board registration (indicating alcohol-related problems) of male adoptees was associated with two somewhat distinct forms of biological and adoptive family history. These included (a) a milder form (also severe, in some cases) which involved adult-onset alcohol abuse without criminality in the biological parents and also environmental vulnerability as indexed by the low socioeconomic status of the adoptive father and (b) a moderate form of alcohol abuse which involved early-onset and recurrent alcohol problems with criminality in the biological parents but less influence of the characteristics of the adoptive father (for female adoptees, see Bohman, Sigvardsson, & Cloninger, 1981). Using an independent cohort of adoptees, Sigvardsson, Bohman, and Cloninger (1996) found that mild or severe (Type I, or 1 as in the original study) and moderate (Type II, or 2 as in the original study) alcohol abuse could be classified by both genetic (based on onset age, severity of parental alcohol abuse, comorbid criminality) and environmental risks (based on socioeconomic status of the adoptive father or prolonged preplacement with diminished access of medical care). Further delineating the effects of adoptive family characteristics of adolescent drinking, McGue, Sharma, and Benson (1996) found that adoptive parent drinking only influenced the drinking of their biological, but not their adopted, offspring. Parenting influenced both biological and adopted offspring, though the latter to a considerably lesser extent. However, the drinking behaviors of the biological and adopted offspring were correlated when they were demographically matched, thus indicating the role of age-delimited (sibling) shared environment.
In addition, adoption studies can yield mechanistic insights into the multiple pathways that underlie SUDs. The potential link between criminality in the biological parent and SUD in the adoptive offspring was further clarified as an independent risk pathway in the Iowa adoption study. Cadoret, Yates, Troughton, Woodworth, and Stewart (1995) found that in addition to increased risk for alcohol and drug dependence in adoptees with biological relatives who had SUDs, antisocial behavior in the biological relatives was correlated with antisociality in the adoptees and that this, independently, contributed to an increased risk for drug use disorders in the adoptees. Therefore, adoption studies can be highly informative from both a genetic and environmental perspective. They are, however, hindered by limitations, such as negative correlations between the characteristics of the biological (typically, lower socioeconomic status and greater psychopathology) and adoptive (higher socioeconomic status and lower psychopathology) parents (McGue et al., 2007). Prenatal environment is another important factor—even when adoptees are separated from their biological mother at birth, the prenatal environment shared with the biological mother can confound the posited exclusivity of the genetically governed correlation between the adoptee and the biological mother (Bouchard & McGue, 2003).
Twin Studies and the Gold Standard of Heritability
Classical twin studies rely on similarities and differences in pairs of monozygotic (MZ, or identical) and dizygotic (DZ, or fraternal) twins (Neale & Cardon, 1992a). Total variance in a measure, such as SUDs, is decomposed into 3 sources—these typically include additive genetic factors (A) and individual-specific environment (E, including measurement error) as well as either nonadditive genetic influences (D) or shared environmental influences (C). Additive genetic influences reflect the net effect of all segregating genes that are shared, on average, 100 % by MZ and 50 % by members of DZ pairs—the presence of A is indicated by an MZ correlation (rMZ) that is substantially greater than a DZ correlation (rDZ). Individual-specific environment, by definition, is uncorrelated across members of twin pairs, even MZ pairs, and may include the influence of measurement error. Evidence for E comes from an MZ correlation that is less than 1.0. Thus in the presence of A and E alone, rMZ < 1 and rDZ = 0.5(rMZ), for instance, rMZ = 0.7 and rDZ = 0.35. C reflects those environmental factors that make members of twin pairs more similar to each other and, under the equal environments assumption, are shared equally (100 %) by members of MZ and DZ pairs. Thus, when C is present, rDZ > 0.5(rMZ) (e.g., rMZ = 0.7 and rDZ = 0.55). In contrast, nonadditive genetic effects are shared to a lesser degree by members of DZ (25 %) than MZ (100 %) pairs, and hence are evidenced by rDZ < 0.5(rMZ) (e.g., rMZ = 0.7 and rDZ = 0.25). That proportion of the total variance (A + C + E or A + D + E) that is attributed to A (i.e., A/A + C + E) is termed (narrow) heritability. In the presence of D, (A + D)/(A + D + E) can also be used to calculate broad-sense heritability.
We now know that SUDs are heritable—about 40–60 % of the variation in liability to SUDs can be attributed to additive genetic influences (A) (Dick, Prescott, & McGue, 2009). While there is evidence for C on certain stages of substance involvement, there is very limited evidence for the role of nonadditive (D) genetic factors. About 48–66 % of the variation in alcohol dependence (Heath et al., 2001; Heath & Martin, 1994; Kendler, Heath, Neale, Kessler, & Eaves, 1992; Prescott & Kendler, 1995) is heritable. For nicotine dependence, 33–71 % (Edwards, Maes, Pedersen, & Kendler, 2011; Kendler et al., 1999; Lessov et al., 2004; Lyons et al., 2008) of the variance can be attributed to heritable influences. A recent meta-analysis of eight twin studies reported heritability estimates of 51 % for males and 59 % for females for cannabis addiction (Verweij et al., 2010). Heritability estimates for cocaine use disorders range from 42 to 79 % with the lower estimates reported for females (Kendler, Karkowski, Neale, & Prescott, 2000; Tsuang, Bar, Harley, & Lyons, 2001; van den Bree, Johnson, Neale, & Pickens, 1998). Two large-scale studies have also examined opiate addiction. Kendler et al. (2000) reported that 23 % of the variation in opioid addiction in men was attributable to genetic factors while Tsuang et al. (1998) report a considerably higher estimate of 54 % in male Vietnam Era Twins (see also Agrawal et al., 2012a).
A majority of the early twin research into substance use behaviors and SUDs were based on samples of predominantly Caucasian descent collected in the USA and Australia. As heritability estimates can be context specific, the addition of studies from Europe, the UK, and China has provided considerable insights. In general, the longitudinal CAStANET study (van den Bree et al., 2007) from the UK indicates comparable heritability estimates for SUDs (Fowler, Lifford et al., 2007). A similar longitudinal Finnish sample has provided numerous insights into the genetic etiology of the development of substance use (Kaprio, Rose, Romanov, & Koskenvuo, 1991). In these Finnish twins (FinnTwin), for example, heritability of problem drinking at age 16 is estimated to be 42–50 % (Dick, Meyers, Rose, Kaprio, & Kendler, 2011) while heritability of smoking and cannabis initiation was approximately 60 % (Huizink et al., 2010). Studying Dutch twins (mean age 27 years), for example, Vink and Boomsma (Vink, Wolters, Neale, & Boomsma, 2010) reported a heritability of 44 % for cannabis initiation (compared with 56 % and 48 % in the USA (Agrawal, Neale, Jacobson, Prescott, & Kendler, 2005) and Australian samples (Agrawal et al., 2007)) with an additional 31 % attributable to shared environment. The elevated estimate of shared environment in the Dutch sample may be attributed to tolerance in the Netherlands towards possession of small amounts of cannabis for personal use. In China, where cigarette smoking is alarmingly common in males (Mackay et al., 2006) but rare in females, examinations of substance use in adolescent twins from Qingdao have found heritable influences (68 %) on alcohol use but not in tobacco experimentation (Unger et al., 2011). However, in a sample of adults from the same region, current (75 %) and heavy (66 %) smoking were highly heritable while evidence for heritable influences on alcohol consumption (42–60 %) was modestly lower and quite consistent with estimates from Caucasian samples (Lessov-Schlaggar et al., 2006).
One disadvantage of these more recent studies is that they are largely restricted to substance use, initiation, and problem use, but not SUDs, due to either the relatively low rates, smaller sample size, or the developmental stage of the twin participants. Nonetheless, they inform the etiology of SUDs as abuse and dependence might be considered one of the several stages in a complex multistage process that underlies the development of addictions.
The Multistage Nature of SUDs
Clinically, SUD is conceptualized as present (affected) or absent (unaffected). Research indicates that the development of SUDs is a multistage process (Fig. 7.1) involving (a) experimentation, (b) regular use, (c) heavy use, characterized by increasing quantity and frequency of use, (d) onset of abuse and dependence (or drug use disorders), and (e) remission and relapse. Early stages of substance involvement, including whether one uses a substance (e.g., onset of alcohol use or experimentation with cigarette smoking), tend to be less heritable and more sensitive to familial environmental factors (Rose, Kaprio, Winter, Koskenvuo, & Viken, 1999). For example, the heritability of alcohol initiation ranges from 0 to 34 % (Pagan et al., 2006; Rose et al., 1999) and for frequency of alcohol use from 30 to 45 % while the heritability of alcohol use disorders ranges from 55 to 65 % (Prescott, Aggen, & Kendler, 1999). Thus, heritability varies across the stages that culminate in SUDs. For genetic studies, this produces a particularly interesting confound. For example, consider a study where never users of cannabis are combined with those who use cannabis regularly but do not develop abuse/dependence symptoms and further, with those who report 1–2 dependence symptoms but do not meet criteria for a cannabis use disorder. None of these individuals meet criteria for cannabis use disorder and, therefore, are unaffected, but are they equally unaffected? Do we expect never users, regular nonproblem users, and problem users without dependence to have identical genetic vulnerabilities? Combining these different types of unaffected individuals can contaminate heritability estimates—when never or even light users are combined with users who do not develop addiction, the consequent mixture of liability distributions can dilute estimates of heritability (Heath, Martin, Lynskey, Todorov, & Madden, 2002). Determining how never users or occasional users will be dealt with in any genetic study of addiction is a crucial first step.
There are several methods for examining genetic influences on SUDs while accounting for its earlier stages. One method, the common-causal-contingent (CCC) approach (Kendler et al., 1999), argues that liability to SUDs is (a) contingent on substance exposure (e.g., those who don’t use a substance cannot develop SUDs); (b) a causal consequence of liability to substance use (e.g., substance use causes SUDs); and (c) that the liability to SUDs partially overlaps, or is in common, with the liability to substance use, such that a portion of the genetic influences on SUDs also influence substance use. Using this method, Kendler and colleagues found that 69 % of the genetic influences on nicotine dependence were shared with genetic vulnerability to initiating cigarette smoking (Kendler et al., 1999). In subsequent analyses extending to all classes of illicit drugs, a considerably high degree of genetic overlap, ranging from 30 % for sedative use disorders to 75 % for cannabis use disorders, was found (Agrawal et al., 2005). Fowler, Lifford et al. (2007), in a sample of UK twins, reported similarly high genetic correlations for tobacco and cannabis but less so for alcohol, perhaps due to the low heritability of alcohol initiation. An alternative method examines the precise degree to which genetic and environmental factors individually correlate across substance initiation or earlier stages of substance involvement and later SUDs (Heath et al., 2002). A variation on the Cholesky decomposition (Neale & Cardon, 1992b) method, results from these models have also consistently shown significant overlap of genetic factors across stages of substance involvement. For example, one study reported correlations of 0.70–0.90 between genes influencing duration of cannabis use and those influencing cannabis use disorders (Lynskey et al., 2006). These studies indicate that, at least, in population-based twin studies, genes that influence liability to substance use often also contribute substantially to genetic vulnerability to SUDs.
The Typical Developmental Course of Substance Use
SUDs follow a natural developmental course. Most substance use begins during adolescence with patterns of use becoming more regular during late adolescence and adulthood. For instance, reports from developed and developing countries participating in the World Mental Health Surveys suggest that 50–80 % of first alcohol use occurs by age 15, with estimates as high as 99 % by age 21 (Degenhardt et al., 2008). In Europe (see ESPAD reports for more detailed estimates (Hibell et al., 2012)), 40–80 % of alcohol use is initiated by age 15; however, some other countries show more marked developmental change—for instance, in Israel, rates of alcohol use rise from 15 to 63 % when examining onset ages of 15 versus 21 years. Likewise, in Japan, a similar steep rise from 30 to 93 % is noted (Degenhardt et al., 2008). Similar 2–3-fold increase in the cumulative incidence of cannabis use is also noted across these ages (Degenhardt et al., 2008). SUDs can develop during adolescence but are more likely to evolve during adulthood. For example, in the 2010 report of the US-based National Household Survey of Drug Use and Health, rates of SUDs were highest in those aged 18–25 years (20 %) relative to those aged 12–17 years (7.3 %) and those aged 26 and older (7 %) (SAMHSA, 2011).
The heritability of these individual stages of substance use and SUDs varies by developmental epoch. For measures of quantity/frequency of use (e.g., drinks/day, cigarettes/day) (Pagan et al., 2006; Rose, 1998) and the number of substances used, heritability estimates appear to have a modest influence during adolescence (11–17 years) and increase with emerging adulthood (Derringer, Krueger, McGue, & Iacono, 2008). During adolescence, shared environmental factors appear to contribute maximally to familial resemblance; however, with the emergence of adulthood, genetic influences are unveiled and heritable variation begins to explain up to 75 % of these individual differences. For problem use, however, there has been consistent evidence for heritable influences, even during adolescence (Rhee et al., 2003).
Gender Differences
These can reflect variations in the magnitude (e.g., is alcoholism more heritable in men than in women?) or the nature of heritable influences (e.g., do different genetic factors influence alcoholism in men and women?). Despite early studies suggesting the absence of heritable influences on alcoholism in women, Heath et al. (1997) concluded that the magnitude of heritable variation in alcohol dependence was similar (64 %) across men and women. Subsequently, Madden and colleagues (1999) reported a similar finding for smoking persistence where heritability ranged from 39 to 49 % in women and 42 to 45 % in men. For cannabis dependence, one study (Lynskey et al., 2002) reported evidence for no heritable influences in women, but this model could not be distinguished from one without sex differences.
Changes in Heritability via Environmental Modification
While there is clear evidence for genetic influences on SUDs, these genes do not act in isolation. Older models focusing on either environment or genetic influences (nature vs. nurture) have now largely been discredited, and it has become widely accepted that important developments in our understanding of the etiology of SUDs will only emerge from research integrating both genetic and environmental influences in a unified framework (Dick, 2011a; Rutter, Pickles, Murray, & Eaves, 2001)—correspondingly, the last decade, in particular, has witnessed an explosion in studies of gene-environment interplay. In addition to studying main effects of genetic liability and environmental exposures, these studies take the novel approach of contextualizing genetic liability as a function of environment and vice versa. We briefly review two important mechanisms by which genetic vulnerability and environmental influences operate in concert.
First, genetic predisposition to SUDs might correlate with an increased or decreased likelihood of exposure to certain environments. Twin studies have substantiated both that there are heritable influences on these “environments” and that observed genetic variation in these environments correlates with genetic susceptibility to the respondent’s own behavior (e.g., peer selection). Such heritable influences on environmental measures that also influence behavior reflect gene–environment correlation (rGE)—the likelihood of exposure to an environment based on one’s own genetic predisposition (Plomin, DeFries, & Loehlin, 1977; Scarr & McCartney, 1983). rGE can be passive (e.g., parents provide enriched environment to offspring with high IQ, genetic predisposition for which is also inherited from parents), evocative (e.g., teacher response to individual’s behavior that is genetically influenced creates advantageous school environment), or active (e.g., selection of niches/peer groups that favor one’s genetic propensity to drug use) in nature.
In contrast, gene–environment interaction (GxE) refers to activation or suppression of genetic vulnerability in a particular environmental context. In some instances, high-risk environments (e.g., exposure to harsh abuse) may render genetic vulnerability redundant while in others it may serve as a trigger for genetic expression (Dick, 2011b; Shanahan & Hofer, 2005). For substance use, environments reflecting reduced social control and increased permissiveness often accentuate or unmask heritability, and twin studies afford some of the most elegant conceptualizations of GxE while accommodating rGE.
In a sense, rGE and GxE correspond to changes in the mean and the variance (and its components), respectively. In the presence of rGE, such as the situation where similar genetic influences connect the choice of and affiliation with deviant peer groups to substance use, it is expected that rGE will contribute to an increased mean level of substance use in respondents who affiliate with deviant peers relative to those who do not. Once this mean difference is accounted for, any differences in the variance that are attributable to the heritable component of variance can be seen as evidence for GxE. Well-powered twin studies can illustrate not only how mean levels or rates of SUDs may be elevated in environments of reduced social control (e.g., respondents with more deviant peers typically report greater substance use) but also whether net variance and heritability differ across levels of environmental exposure such as a range of environments that vary from being restrictive (e.g., legal sanctions on smoking, parental monitoring, high religiosity, low urban development) to being largely permissive (e.g., openness towards drug use, peer delinquency, high migration).
Peers and Heritability of SUDs
There is no doubt that peer substance use, whether perceived or objectively measured, has a potent influence on onset and regular use of substances (Creemers et al., 2010; Fergusson, Swain-Campbell, & Horwood, 2002; Guxens, Nebot, Ariza, & Ochoa, 2007; Korhonen et al., 2008). A wealth of literature has documented that individuals who affiliate with deviant peers are more likely to use and misuse substances, indicating a mean difference. To some extent, this mean increase in substance use due to deviant peer affiliations is attributable to rGE. Evidence for rGE is derived from observations that, first, peer drug use and deviance is heritable (Agrawal, Balasubramanian et al., 2010; Button et al., 2009; Kendler, Jacobson et al., 2007) and, second, due to an overlap between these genetic influences and those impacting respondent self-report of substance use and misuse. Kendler and colleagues have reported that genetic influences on peer deviance range from 30 % at ages 8–11 years increasing up to 50 % at ages 20 and older (Kendler, Jacobson et al., 2007). In fact, a study of peer networks found that a genotype in the gene encoding the dopamine receptor 2 (DRD2) is correlated across unrelated peers, further adding support for genetic influences on peer choice (Fowler, Settle, & Christakis, 2011). Multiple studies find commonality in the genetic factors influencing respondent reports of peer and personal substance use (Button et al., 2009; Gillespie, Neale, Jacobson, & Kendler, 2009; Harden, Hill, Turkheimer, & Emery, 2008). For instance, a study by Agrawal, Balasubramanian et al. (2010) found that 18 % of the genetic influences on respondent reports of peer drug involvement were shared with genetic influences on their own substance use behavior. Reporting even higher genetic correlations of 0.6–0.7, Fowler, Shelton et al. (2007) find strong evidence for the role of shared genetic influences on peer and personal alcohol use. This genetic overlap may be viewed as support for peer selection or affiliation with peer groups that espouse one’s own views on substance use, as indicated by the shared genetic liability to use substances and affiliate with peers who do so as well.
Peer socialization, on the other hand, implies that prolonged affiliation with a peer group modifies the respondent’s behavior. To test the socialization hypothesis in a genetically informed manner, Gillespie and colleagues (2009) used a direction-of-causation model. In this framework, uncorrelated genetic and environmental factors were allowed to influence peer group deviance and cannabis use, and the relationship between the two (reflecting socialization/transactional and selection/contagion processes) was quantified by causal paths going in either direction. However, instead of socialization, that study found evidence for peer selection (respondent’s cannabis use influenced their choice of peers).
Evidence for GxE can also be considered a form of peer socialization—as an individual aggregates more substance-using peers (or peers who approve of substance use), the respondent’s genetic propensity to use substances is unveiled. In support of this hypothesis, two independent studies have found that the total variance and the proportion of heritable variance in substance use change with changes in exposure to substance-using peer. One study found that heritability (and total variance) of a measure representing repeated alcohol, cigarette, and cannabis use was as high as 60 % in those who perceived a majority of their peers to be substance users and as low as 10 % in those who perceived low levels of peer substance use (Agrawal, Balasubramanian et al., 2010). In the other study, Button and colleagues (2009) found that heritability (and total variance) of substance dependence was high in both those with very high and very low levels of peer delinquency but declined sharply in those with intermediate levels of affiliation with deviant peers.
Reduced Social Control as an Environmental Moderator
Drug-using or delinquent peers are an index of environments representing reduced social control (Rose & Dick, 2010). Such environments, by minimizing prohibition on drug use behaviors, allow for manifestation of genetic propensity. Socio-regional characteristics that typify reduced social restriction (e.g., urban environments with higher numbers of youth, easier access to substances, and increased migration) have also been found to have a similar effect on heritable influences on alcohol involvement (Rose, 1998; Rose et al., 2003). In an influential paper, Rose et al. (1999) reported the importance of socio-regional context (such as residential region and urbanicity) in twin similarity for drinking initiation. While there was an overwhelming influence of shared environment on initiation, analyses revealed that MZ within-pair correlations in twins residing in Greater Helsinki (classified as an urban “wet” area) were nearly twice as high as those in twins from Northern Finland (classified as a rural “dry” area). Extending these analyses, these investigators found that individual differences in alcohol use in young Finnish twins living in regions with most young adults (h 2 = 0.63 vs. 0.13), most migration (h 2 = 0.60 vs. 0.16), and high alcohol sales (h 2 = 0.49–0.61 vs. 0.25–0.32) were attributable to a significantly greater degree to heritable factors when compared to residential regions with fewer youth, lower migration, and lower alcohol sales (Dick, Bernard et al., 2009; Dick, Rose, Viken, Kaprio, & Koskenvuo, 2001; Rose, Dick, Viken And, & Kaprio, 2001). The authors posited that regions of greater urbanicity allow for a pro-drinking milieu, which contributes to the unveiling of individual genetic propensity to consume alcohol. Similarly, Koopmans, Slutske, van Baal, & Boomsma (1999) reported increased heritability of drinking in those with reduced church attendance (i.e., less subjected to religious proscriptions on drinking) while Heath, Jardine, and Martin (1989) found that variation in alcohol problems in unmarried (vs. married) women was more significantly attributable to heritable factors, potentially because marriage, by establishing social roles, potentially buffered genetic vulnerability to alcoholism.
Same Genes, Different Drugs?
Thus far, we have focused on genetic influences on individual substances. However, multiple lines of research show that SUDs co-aggregate. For instance, in the large family-based Collaborative Study of the Genetics of Alcoholism, siblings of the alcohol-dependent index cases were not only more likely than siblings of control individuals to be at increased risk for alcohol use disorders but they were also more likely to meet criteria for cannabis and cocaine dependence and for habitual smoking (Bierut et al., 1998). However, this across-substance co-aggregation has not been uniformly reported. Merikangas and colleagues found that rates of illicit drug use disorders were elevated in relatives of individuals with opioid, cannabis, or cocaine use disorder but not in relatives of individuals with alcohol use disorders alone (Merikangas, Stolar et al., 1998). Nonetheless, evidence that genetic factors are strong contributors to this co-aggregation arises from numerous twin studies that show that a preponderance of the genetic influences on one form of SUD is shared with others.
Three important conceptualizations of this genetic overlap have been studied. In their study of Vietnam Era Twin males, Tsuang and colleagues found that underlying cannabis, stimulant, sedative, opiate, and hallucinogen use disorders was a common genetically influenced vulnerability (Tsuang et al., 1998). According to this study, a majority of the genetic influences on SUDs were shared with this common vulnerability, which had a heritability of 31 %. The exception was heroin and opiate use disorders for which 70 % of the heritable influences were drug specific. Reporting a similar result, while testing a somewhat differing rationale for co-aggregation of SUDs, Kendler, Jacobson, Prescott, and Neale (2003) found that a single genetic factor was responsible for a majority of the heritable variance in cannabis, cocaine, stimulant, sedative, opiate, and hallucinogen use disorders. Extending this work to include alcohol, nicotine, and, interestingly, caffeine dependence, Kendler, Myers, and Prescott (2007) found evidence for a model where two genetic factors were responsible for a majority of the genetic variation. The first genetic factor captured vulnerability to cannabis and cocaine dependence while the second genetic factor was responsible for genetic influences on alcohol, nicotine, and caffeine dependence. However, these genetic factors were highly correlated (r = 0.82) indicating that a common set of genes might contribute to licit and illicit SUDs. A third model of shared influences was examined by Young, Rhee, Stallings, Corley, and Hewitt (2006) where common and specific genetic influences were allowed to explain the co-aggregations of alcohol, tobacco, and cannabis use and problem use. Intriguingly, they noted that while shared genetic etiologies were important to problem use of alcohol, cannabis, and tobacco, they played a less prominent role in substance use. Based on this work as well as prior evidence from sibling correlations (Stallings et al., 2003), these authors developed a single measure representing dependence vulnerability (average number of dependence symptoms across substances) which was subsequently used quite successfully in genomic analyses.
Same Genes, Different Psychopathologies
In addition to similar genetic influences acting across SUDs, these genetic factors also influence other forms of externalizing psychopathology, such as antisocial personality disorder, conduct disorder, and impulsivity. In an early study of adolescent twins, Krueger and colleagues (2002), and more recently Hicks, Schalet, Malone, Iacono, and McGue (2011), found that a common heritable predisposition to externalizing psychopathology connected these behaviors accounting for most of the heritable influences on them. This is consistent with studies of adolescent samples that have identified significant increases in disinhibitory and problem behavior accompanying adolescent substance use and misuse (Iacono, Carlson, Taylor, Elkins, & McGue, 1999; McGue, Iacono, & Krueger, 2006). Based on a similar degree of genetic overlap across antisocial behaviors and substance use problems in adolescents (Rhee et al., 2006), Stallings et al. (2005) have developed indices of antisocial drug dependence that have been quite fruitful in linkage studies. In a sample of adult males and females, Kendler, Prescott, Myers, and Neale (2003) similarly reported that a single genetic factor was primarily responsible for the heritability of adult antisocial behavior, conduct disorder, and alcohol and illicit drug use disorders. However, the study of adults also identified genetic influences that were specific to alcohol and illicit drug use disorders and did not overlap with other forms of externalizing psychopathology. This indicates that, perhaps, as individuals’ age, some may mature out of disinhibitory behaviors and conduct problems but develop SUDs via other comorbid pathways, including those that relate to internalizing psychopathology.
While a majority of genetic research has focused on the comorbidity between externalizing psychopathology and substance use and SUDs, there is also considerable comorbidity between SUDs and internalizing disorders, including anxiety disorders and major depressive disorder. That internalizing disorders are more common in those with SUD and vice versa is well recognized. Family studies indicate that these disorders also co-aggregate—for example, Merikangas, Stevens et al. (1998) reported that alcohol dependence (but not abuse) may share genetic etiologies with panic disorder (but not social phobia). Two large-scale twin studies (Prescott, Aggen, & Kendler, 2000) have found considerable genetic overlap between major depressive disorder and alcohol use disorders with a recent study reporting that genetic influences on depressive symptoms and alcohol use correlate about 0.26–0.59, even during adolescence (Edwards, Sihvola et al., 2011). One hypothesis linking SUDs to internalizing problems relates to self-medication (Khantzian, 1997)—individuals with anxiety or depressed mood may misuse substances to medicate their symptoms. However, another line of research indicates that the genetic overlap between major depressive disorder, cannabis, and alcohol use disorders may be attributable to comorbid co-occurrence of antisocial personality disorder (ASPD). In the Vietnam Era (male) Twin sample, Fu and colleagues (2002) found that after controlling for ASPD, the residual genetic overlap between major depressive disorder and both SUDs was negligible. In addition to accounting for genetic influences on SUDs, ASPD also explained 15 % of the total variance in major depression, indicating that disorders conventionally classified as part of the internalizing and externalizing spectrum may not be that orthogonal after all.
What Does Heritability Mean?
Thus far, we have presented compelling evidence supporting the role of heritable influences on substance use and SUDs. This heritability represents the aggregate effects of multiple genetic variants that exert a polygenic effect on liability to SUDs. Over the past 2 decades, research has accelerated the pace of gene identification strategies that have allowed investigators to isolate genomic regions and gene variants that contribute to this heritable variation. These gene finding methodologies can be broadly divided into linkage, candidate gene association, and genome-wide association studies.
Linkage Studies
Linkage analysis is used to isolate large segments of the genome that segregate with the behavior of interest. Despite being low density, linkage studies can be used to systematically scan the human genome to identify regions that potentially warrant a more fine-grained genotypic investigation (Sham, 1998). Underlying the most common form of linkage analysis is the concept of identity by descent (IBD) or allele sharing. IBD refers to the extent to which a pair of related individuals inherits the same ancestral allele. The simplest example is of allele sharing in pairs of siblings. Imagine that a polymorphic (a variation along the genome that naturally exists in varying states in the population) marker X exists in 4 forms (A, B, C, and D, such that, perhaps, 20 %, 30 %, 40 %, and 10 % of the population carry one or more copies of alleles A, B, C, and D, respectively). In the absence of linkage, the mating of two fully informative heterozygous parents (say, one with genotype AB and the other with genotype CD) will produce, with equal likelihood, offspring with genotypes AC, AD, BC, and BD. For any pair of these offspring (i.e., siblings), it is expected that by chance alone, 25 % of the sibling pairs will share no alleles IBD (e.g., sibling 1’s genotype is AC and sibling 2’s genotype is BD) and an equal proportion will share both alleles IBD (e.g., sibling 1 and 2 are AC). The remaining 50 % are expected to inherit 1 of these 2 alleles from the same parent (e.g., sibling 1’s genotype is AC and sibling 2’s genotype is BC, so they share allele C). Now, imagine that proximal to marker X is a gene variant that increases vulnerability to SUDs such that during meiosis (gametic cell division) marker X and the disease gene variant are transmitted from parent to affected offspring in tandem. Examining pairs of siblings with SUDs, we may now expect an excess of allele sharing for marker X—in other words, we might expect more than 25 % of the SUD sibling pairs would share both alleles IBD—or that allele sharing, on average, would exceed 50 %. This deviation from the expected likelihood of allele sharing (i.e., 25 % IBD = 0, 50 % IBD = 1, and 25 % IBD = 2 for siblings) in the direction of excess sharing indicates that marker X may be linked to a gene variant that potentially modifies vulnerability to SUDs. Using this strategy, one of the first and largest multisite genetic studies of alcohol dependence, the Collaborative Study of the Genetics of Alcoholism (COGA), utilized alcohol dependence data from 105 multigenerational families and examined linkage across 291 such polymorphic markers. Regions on chromosome 1, 2, and 7 were identified. For example, the strongest signal in 382 alcohol-dependent sibling pairs was for D1S532 and D1S588 with 55–56 % allele sharing on average (Reich, 1996; Reich et al., 1998).
There have been numerous linkage studies of SUDs with considerable progress in the area of alcohol use disorders. Results of linkage studies are often viewed as LOD scores (LOD is the Logarithm (base10) Of Odds; an LOD of 3 indicates a 1,000:1 odds in favor of linkage) and these LOD scores often span a significant genetic distance. For example, the linkage peak on chromosome 1 referenced above spanned 100 cM (centiMorgans, where 1 cM roughly equals 1,000,000 base pairs of DNA). Thus, while linkage studies narrow the search for SUD genes to a chromosomal region, there is often much to be done to systematically identify these genes. In some cases, as for alcohol, linkage produces exciting new leads that translate into gene-finding efforts. For example, utilizing data on families from the Southwestern American Indian tribes, Long et al. (1998) were amongst the first to identify a region on the short arm of chromosome 4 for alcohol dependence. This region harbors a cluster of genes encoding receptor subunits of the gamma-aminobutyric acid receptor, which have since been found in multiple independent studies to be associated with SUDs (Agrawal et al., 2006; Covault, Gelernter, Hesselbrock, Nellissery, & Kranzler, 2004; Drgon, D’Addario, & Uhl, 2006; Edenberg et al., 2004; Fehr et al., 2006; Lappalainen et al., 2005). Another region on the long arm of chromosome 4 was identified in a large study of Irish families densely affected for alcohol use disorders (Kuo et al., 2006). This region, also implicated in COGA when studying a quantitative index of alcohol consumption (maximum drinks consumed in a single 24-h period) (Saccone et al., 2000), is intriguing because of its proximity to genes that form the alcohol dehydrogenase (ADH) cluster of genes (ADH7, ADH1C, ADH1B, ADH1A, ADH6, ADH4, ADH5). These linkage findings were instrumental in narrowing the genomic search space for genes associated with alcohol use disorders.
Linkage studies for nicotine dependence and smoking–related phenotypes have also begun to produce exciting leads. In one of the earliest attempts to home in on genomic regions for smoking, Straub and colleagues (1999) examined linkage for nicotine dependence (defined using scores on the Fagerstrom Tolerance Questionnaire) in a sample of 308 nicotine-dependent individuals from 130 families from Christchurch, New Zealand with replication in a US sample. Regions of chromosomes 2 and 10 showed possible evidence for linkage; however, support for these regions was considerably lower in the replication sample. Since that study, Sullivan and colleagues (2004) identified multiple genes of putative etiologic importance to nicotine dependence in those regions. These included a family of cytochrome P450 genes, involved in drug (but not nicotine) metabolism on chromosome 10; however, as is discussed later, these genes have not emerged as the most promising vulnerability loci for nicotine dependence. Perhaps the strongest linkage signal for a smoking phenotype was obtained for maximum number of cigarettes smoked in a 24-h period in a sample of Australian and Finnish adults ascertained for heavy smoking—a region on chromosome 22 reported an LOD score of 5.41 for a marker (D22S315) in ADRBK2, encoding the beta-adrenergic receptor kinase 2 (Saccone, Pergadia et al., 2007). Recently, a meta-analysis of published linkage studies of nicotine dependence and heavy smoking phenotypes was conducted (Han, Gelernter, Luo, & Yang, 2010). A promising and genome-wide significant signal was identified on the long arm of chromosome 20 while suggestive evidence for linkage was noted on the short arm of chromosome 20, chromosome 22, and chromosome 17. Of particular note, this region on chromosome 20 harbors the gene encoding the alpha 4 subunit of the cholinergic nicotinic receptor (CHRNA4).
In addition to alcohol and tobacco, multiple linkage studies have also been conducted of cannabis–related phenotypes. Of these, three independent studies have isolated a region on the long arm of chromosome 3 which harbors the gene encoding monoglyceride lipase, the enzyme involved in metabolism of certain endocannabinoids (Agrawal, Pergadia et al., 2008; Ehlers, Gizer, Vieten, & Wilhelmsen, 2010; Hopfer et al., 2007). Other signals have been modest (Agrawal, Hinrichs et al., 2008; Agrawal, Morley et al., 2008) and largely inconsistent, with the exception of two studies by Ehlers and colleagues (Ehlers et al., 2010; Ehlers, Gilder, Gizer, & Wilhelmsen, 2009). In the first, a study of Type II cannabis dependence in Native American families, LODs of 4.4 and 6.4 were identified on chromosomes 16 and 19, as well as a region on chromosome 14 which was consistent with a prior linkage signal for cannabis dependence in COGA (Agrawal, Hinrichs et al., 2008). In a subsequent family study of alcoholism, these authors studied specific aspects of cannabis dependence, specifically craving and withdrawal. For cannabis craving, including during withdrawal, an LOD of 5.7 was noted on chromosome 7 while for cannabis withdrawal, a previously identified region on chromosome 9 showed an LOD of 4.2 for the withdrawal symptom of sleeplessness (Ehlers et al., 2010).
Other illicit drugs have also been sporadically investigated within the context of linkage analysis. Gelernter and colleagues (2005) conducted linkage analysis of cocaine dependence and cocaine-induced paranoia—while results were speculative for the diagnostic measure of cocaine dependence, a region on chromosome 9 (LOD = 3.65) was isolated for cocaine-induced paranoia in African-American subjects. Furthermore, when these investigators classified individuals based on their patterns of cocaine and other drug use behaviors, an LOD of 4.66 on chromosome 12 was identified but only in the cluster of European-American individuals that were predominantly cocaine users. In addition to the use of a novel method of grouping individuals with SUDs, this study had two additional features—first, it was amongst few studies that specifically examined linkage signals that were unique to European-American and African-American families; and second, it utilized a parametric linkage model. While quite successful for the study of genes responsible for Mendelian disorders, parametric linkage, which does not rely on IBD but instead requires the specification of mode of inheritance, disease allele frequency, and penetrance (likelihood of observing the phenotype in the presence of the risk genotype), has not been widely used in linkage studies of SUDs. However, the linkage signal for predominantly cocaine use was identified by specifying a dominant mode of inheritance, disease allele frequency of 0.01, and a penetrance of 0.85. Gelernter and colleagues also examined linkage regions for opioid dependence and cluster of opioid users (Gelernter, Panhuysen et al., 2006). A region on chromosome 17, with an LOD of 3.06, was identified for the cluster of heavy opioid users.
In addition to individual substances, Stallings and colleagues have used a composite measure of dependence vulnerability (average number of dependence symptoms across drug classes) for linkage analysis (Stallings et al., 2003). This measure captured LOD scores on chromosomes 3 and 9, which could be further enhanced by the addition of antisociality to the assessment (Stallings et al., 2005). At least one independent study has identified this peak on chromosome 3 as well (Agrawal, Pergadia et al., 2008).
Despite providing a whole-genome perspective, linkage studies can only home in on broad segments of the genome, which then require further careful study. Association analyses, within families or with unrelated individuals, allow the unique opportunity for gene identification, of either genes within a linkage region or genes of putative biological interest, or to explore genes across the entire genome. Unlike linkage, which focuses on an overabundance of allele sharing in related individuals, association can be conducted with both related (family-based) or unrelated (case-control) individuals. The simplest method for conducting family-based association is to examine whether a risk-conferring allele is transmitted from a heterozygous parent to an affected offspring—this is referred to as the transmission disequilibrium test (Spielman, McGinnis, & Ewens, 1993). It bears some similarities to linkage; however, in addition to multiple technical differences, family-based association is often extended to examine not only transmission of risk within families but also whether the risk-conferring allele is more commonly noted, across families, in affected versus unaffected individuals. In fact, when association is conducted with unrelated individuals, it simply focuses on whether this risk-conferring allele is more prevalent in cases (affected) or controls (unaffected).
Association Studies with Candidate Genes
The earliest and, perhaps, most popular association studies are candidate gene studies. Here, the investigator selects genes of biological significance to stages of substance involvement, including substance-related problems or SUDs. These genes can be broadly divided into (a) genes encoding receptors whose activity is modified by the drug binding to it or that influence the subjective experience of the psychoactive effects of the drug and (b) genes that are involved in drug metabolism. Not surprisingly, receptor systems appear to influence liability to various SUDs while genes associated with metabolism tend to be fairly drug specific. Here we review genes that can be classified into these two categories for alcohol, nicotine, and cannabis, as well as a family of genes that have been associated with a number of SUDs as well as with externalizing and internalizing disorders, potentially explaining some of the genetic overlap noted in twin studies between SUDs and these psychopathologies.
Alcohol: Motivated by human and animal genetic research, extensive gene association analyses have been conducted for alcohol use disorders. The receptor gene that has been most consistently implicated in the etiology of alcohol use disorders is GABRA2, the gene encoding the α2 subunit of the gamma-aminobutyric acid receptor A. GABA is a major inhibitory neurotransmitter, associated with the sedative and anxiolytic effects of drugs. It binds to the pentameric GABA-A receptors, which commonly include two α, two β, and a γ subunit (Barnard et al., 1998). In experimental models, some key effects of ethanol are GABA-A receptor mediated (Buck, 1996). A fairly large number of independent human genetic studies have implicated single nucleotide polymorphisms (SNPs) in GABRA2 in the etiology of alcohol and other SUDs. The first of these studies, in the COGA sample (Edenberg et al., 2004), examined 49 SNPs in GABRA2, as well as 20 SNPs in the neighboring GABRG1, GABRA4, and GABRB1 genes. Thirty-one SNPs that were correlated with each (in moderate to high linkage disequilibrium) in or near GABRA2 were associated with alcohol dependence, and 26 SNPs were also associated with beta wave brain activity, an electroencephalogram measure that is considered to be an endophenotype (an intermediate index of alcoholism vulnerability) for alcohol use disorders. Some of the most significantly associated SNPs (rs279871, rs279826, rs279845, rs279836, or rs279858) were associated with alcohol dependence in independent Russian (Lappalainen et al., 2005) and German (Fehr et al., 2006) samples. Since these studies, a number of additional investigations have revealed that the association between GABRA2 SNPs and alcohol dependence may extend to comorbidity between alcohol and other SUDs. For example, revisiting the COGA findings, Agrawal and colleagues (2006) found that association between GABRA2 and alcohol dependence was most pronounced in those with comorbid illicit drug use disorders, with association being nonsignificant in those with alcohol dependence alone. However, the stronger effect for comorbid alcohol and substance use disorder has not been consistently replicated (Covault et al., 2004; Lind et al., 2008; Soyka et al., 2008). In addition to its links with SUDs, GABRA2 SNPs have been found to contribute to higher risk for impulsivity and conduct disorder (Dick, Bierut et al., 2006), both of which developmentally co-aggregate with SUDs. Recently, several non-replications of the GABRA2 association have also emerged, urging the need for a meta-analytic approach (Lind et al., 2008; Soyka et al., 2008).
None of these well-studied GABRA2 SNPs have known functional relevance. Yet, experimental studies have begun to link these SNPs to alterations in brain activity. Carriers of the risk-conferring allele in rs279858 (a synonymous SNP, i.e., a variant where alterations in base pairs do not produce a coding change) and rs279826 (an SNP in a noncoding/intronic gene region) not only were found to be at increased risk for alcohol dependence and impulsiveness but also showed greater insula activation during the reward anticipation phase of a monetary incentive task (Villafuerte et al., 2012). This does not imply that these SNPs themselves modify insula activity but that they may be highly correlated with other variants that might contribute to this variation.
There have also been numerous animal studies exploring the role of the GABAA receptors and the GABRA2 gene in the context of ethanol treatment. In Xenopus oocytes, ethanol treatment modified GABA currents as a function of change in the number of α2 subunits in GABAA receptors supporting the role of α2 subunits in the potentiation of GABAA by ethanol (Hurley, Ballard, & Edenberg, 2009). Another elegant study of alcohol-preferring rats trained to binge drink found that injection of silencing/short interfering RNA that suppresses α2 expression in the central nucleus of the amygdala resulted in short-term cessation of ethanol intake (Liu et al., 2011).
GABAA genes tend to occur in chromosomal clusters (McLean, Farb, & Russek, 1995) and while there has been limited evidence for association with other genes in the chromosome 4 GABRA2 cluster, genes encoding other GABAA subunits have been linked to alcohol dependence (Dick et al., 2004; Dick, Plunkett et al., 2006; Ittiwut et al., 2012; Xuei et al., 2010). Another family of genes that tends to occur in clusters and is of clear relevance to alcohol use disorders is the alcohol and aldehyde dehydrogenase genes which play a role in metabolism of alcohol (Edenberg, 2007; Thomasson, Crabb, Edenberg, & Li, 1993). In particular, rs1229984 in ADH1B and rs671 in ALDH2 are widely recognized for their role in eliciting the flushing response upon alcohol exposure noted in certain Asian populations (Thomasson et al., 1993). Upon ingestion, alcohol is first converted to acetaldehyde, and subsequently this acetaldehyde is converted to acetate. The rate of production of acetaldehyde is modified by ADH1B (rs1229984) while its clearance is regulated by ALDH2 (rs671) with its accumulation resulting in flushing (facial reddening, nausea, dizziness). Thus, those who carry the protective variant in rs1229984 tend to rapidly convert alcohol to acetaldehyde, experience flushing, and therefore drink less. Likewise, those who carry the protective variant in rs671 experience difficulty converting acetaldehyde to acetate and, due to the aversive effects of accumulating acetaldehyde, drink less. There are numerous association studies of ADH1B and ALDH2 in Asian cohorts (Li, Zhao, & Gelernter, 2011); however, while this protective variant in rs1229984 is fairly common (approx 70 %) in certain Asian populations, it is relatively rare in non-Asians (about 1–3 % in European and African-American subjects). Despite its low prevalence, multiple independent studies have found strong effects of rs1229984 on drinking behaviors in non-Asians. In fact, MacGregor and colleagues (2009) reported that non-Asian carriers of the protective rs1229984 allele also exhibit flushing and drink less. Another study showed that, in European-Americans, those who carry the protective variants are at 0.34 decreased odds of developing alcohol dependence with a similar effect in African-Americans as well (Bierut et al., 2012). On the other hand, while rs671 is recognized to have clear effects in Asians, the prevalence of its protective allele is low in Asians (11–15 %) but is vanishingly rare and unlikely to be of considerable relevance in non-Asians. Other SNPs are likely to exert an influence in these non-Asian cohorts. Within the ADH cluster, SNPs in ADH1C, ADH4, and ADH5 have been found to be associated with alcohol dependence. Of note, the functional and common SNP rs1693482 (and rs698, which is correlated with it) in ADH1C has also been implicated (Agrawal, Lynskey et al., 2010; Frank et al., 2011; Luo et al., 2006; Macgregor et al., 2009).
Intriguingly, it has been noted that rates of alcohol problems in heterozygotes (carriers of one copy of the protective variant) of rs671 have undergone increase (Higuchi et al., 1994)—this implies that despite the aversive nature of the flush response, social pressures may drive individuals to drink—yet another illustration of the incredible complexity underlying even the simplest (i.e., metabolism) aspects of SUDs.
Additional gene variants have been frequently studied in the context of alcohol dependence as well as other SUDs and psychopathology in general. The Taq1A promoter polymorphism in the gene encoding the D2 dopamine receptor (DRD2) is recognized as a risk factor for alcoholism (Noble & Blum, 1993). The functional significance of the Taq1A polymorphism remains unknown although it has been correlated with reduced D2 receptor density (Jonsson et al., 1999; Pohjalainen et al., 1998; Thompson et al., 1997). Meta-analyses find odds ratios ≈ 1.2 (p < 0.001) (Munafo, Matheson, & Flint, 2007) −1.4 (p < 0.00001) (Smith, Watson, Gates, Ball, & Foxcroft, 2008), for the A1 allele; however, considerable across-study heterogeneity exists. While linked to DRD2, the Taq1A polymorphism (also known as rs1800497) is actually in the ANKK1 (ankyrin repeat and kinase domain containing 1) gene (Neville, Johnstone, & Walton, 2004) and recent research has expanded the search for risk effects previously associated with DRD2 to its neighboring genes, including ANKK1 as well as NCAM1 (neural cell adhesion molecule 1) and TTC12 (tetratricopeptide repeat domain 12). SNPs in exon 3 of TTC12 and exon 12/intron13 of NCAM1 are associated with alcohol, nicotine, and drug dependence with additional evidence for association with SNPs in ANKK1 that are distinct from Taq1A (Gelernter, Yu et al., 2006; Yang et al., 2007). Some studies have also implicated Taq1A in smoking behaviors, including initiation, dependence, and cessation, but meta-analyses have yielded inconclusive results (Munafo, Timpson, David, Ebrahim, & Lawlor, 2009).
Nicotine: In addition to Taq1A, multiple other candidate SNPs have been examined in the context of nicotine dependence and smoking-related behaviors. These can also be broadly divided into SNPs in genes encoding the cholinergic nicotinic receptors (CHRNs) and the cytochrome P450 2A6 (CYP2A6) involved in the metabolism of nicotine and cotinine. There is now convincing evidence that polymorphisms in a cluster of CHRN genes on chromosome 15 (CHRNA5/CHRNA3/CHRNB4: cholinergic receptor, nicotinic, alpha 5 and 3, and beta 4) are associated with cigarettes smoked per day (Bierut, 2011). The earliest evidence for association of these polymorphisms with nicotine dependence is from a study of 1,050 nicotine-dependent cases (Fagerstrom Test of Nicotine Dependence (FTND) score of 4 or greater) and 879 smoker controls (FTND score of 0 but smoked 100 or more cigarettes during the lifetime) (Saccone, Hinrichs et al., 2007). A non-synonymous (functional) variant rs16969968 in CHRNA5 was amongst the top 5 association signals—the effect of this polymorphism has now been replicated in multiple large genome-wide association studies (Liu et al., 2010; Thorgeirsson et al., 2010; Tobacco and Genetics Consortium, 2010). This SNP has also been shown to be associated with cocaine dependence (Grucza et al., 2008; Sherva et al., 2010), although paradoxically in the opposite direction from its effects on nicotine dependence. Other studies have implicated variants in CHRNA4 (Han et al., 2011; Saccone et al., 2010), a gene that resides in the region identified by a meta-analysis of linkage studies, and in conjunction with the receptor subunit encoded by CHRNB2, the target for smoking cessation drug varenicline (Gonzales et al., 2006). Minor allele carriers of multiple CHRNB2 variants have also been shown to experience greater nausea and dizziness upon use of varenicline (Swan et al., 2012).
The first step in the metabolism of nicotine occurs via its conversion to cotinine in the liver by the enzyme encoded by CYP2A6 (Benowitz & Jacob, 1994). Analysis of cotinine levels has led to classification of smokers as slow and fast metabolizers (Benowitz, Swan, Jacob, Lessov-Schlaggar, & Tyndale, 2006). Slow metabolizers tend to have a lower ratio of 3′hydroxycotinine/cotinine, smoke less, and are relatively more successful at quitting smoking. Slow metabolism has been linked to the CYP2A6 gene, which has fully inactive deletion forms, such as CYP2A6 * 4A, CYP2A6 * 4B, and CYP2A6 * 4D and a SNP, CYP2A6*2 (Hukkanen, Jacob, & Benowitz, 2005). Multiple other allelic variants in CYP2A6 and in CYP2B6 also influence nicotine metabolism to varying degrees. Research shows that slow metabolizers may be better suited to nicotine replacement therapy (NRT)—slow metabolizers tend to have higher levels of plasma nicotine upon administration of NRT and, thus, need fewer doses. Fast metabolizers, who may have a more recalcitrant form of nicotine dependence, may respond better to non-nicotine treatments such as bupropion and varencline (Malaiyandi et al., 2006).
Cannabis: Two genes have dominated candidate gene studies of cannabis dependence. The gene encoding the cannabinoid receptor 1 (CNR1), a ubiquitous G-protein-coupled receptor and the target for the controversial antiobesity drug Rimonabant, has been associated with cannabis dependence in multiple independent studies (Agrawal et al., 2009; Haughey, Marshall, Schacht, Louis, & Hutchison, 2008; Hopfer et al., 2006). An AAT repeat was amongst the first variants to be studied for cannabis use disorders. Comings and colleagues (1997) reported that individuals with five or more repeats were more likely to meet criteria for SUDs, including cannabis dependence. SNPs most commonly studied in CNR1 include rs2023239, rs806368, and rs806380 and while a majority of studies have utilized general measures of SUD (Agrawal & Lynskey, 2009), Hopfer and colleagues (2006) found that rs806380 and haplotypes of rs6454674-rss806380-rs806377-rs1049353 (GGCC, TACC, GACC) were associated with cannabis problems specifically.
Another well-studied gene for cannabis use disorders is FAAH, encoding fatty acid amide hydrolase which catalyzes the conversion of both AEA (N-arachidonoylethanolamine, or anandamide) and 2-AG (2-arachidonoylglycerol) to arachidonic acid and ethanolamine (for AEA) or glycerol (for 2-AG) (Di Marzo, De Petrocellis, Bisogno, & Melck, 1999). In animal studies, inhibiting FAAH activity (and thereby reducing breakdown of endogenous cannabinoids) increases non-opioid-induced analgesia (Cravatt & Lichtman, 2003). In murine knock-out models, FAAH −/− mice are reported to be more responsive to exogenous cannabinoids and to have lower pain sensitivity (Lichtman, Hawkins, Griffin, & Cravatt, 2002). These effects of FAAH blockade on pain and inflammation have led to excitement surrounding its therapeutic role. A majority of these studies tested the effects of a missense (causing a coding change) mutation (C385A) that converts a conserved proline residue to threonine in exon 3 (rs324420, previously rs57947754). There is considerable evidence that this missense mutation is associated with risk for substance dependence in Caucasian and African-American, but not in Japanese or other Asian populations (Agrawal & Lynskey, 2009). Individuals homozygous for the minor A allele were nearly 5 times more likely to be street drug users or to report problems with alcohol or drugs (Sipe, Chiang, Gerber, Beutler, & Cravatt, 2002). The effects were strongest in those with comorbid drug and alcohol problems, but there were no statistically significant effects in individuals with alcohol or nicotine dependence in the absence of illicit drug use. In contrast, when examining cannabis dependence alone (and not general substance dependence), Tyndale, Payne, Gerber, and Sipe (2007) found that A/A individuals were at 0.25 odds of developing cannabis dependence (but, potentially more likely to try cannabis). The authors argue that the reduced risk for cannabis dependence may stem from diminished FAAH activity leading to increased levels of endogenous cannabinoids which may ameliorate craving and withdrawal.
Examining the effects of rs2023239 (CNR1) and rs324420 (FAAH) on cannabis withdrawal and craving, Haughey et al. (2008) reported that both SNPs were associated with craving upon cue exposure and negative affect upon withdrawal while the CNR1 SNP was associated with cannabis withdrawal upon abstinence—some of these effects were direct, while other genotype effects occurred via an interaction with abstinence. C allele carriers of rs2023239, for instance, reported significantly higher withdrawal scores after 5 days of abstinence. Likewise, CC homozygotes for rs324420 showed a significant increase in craving upon abstinence.
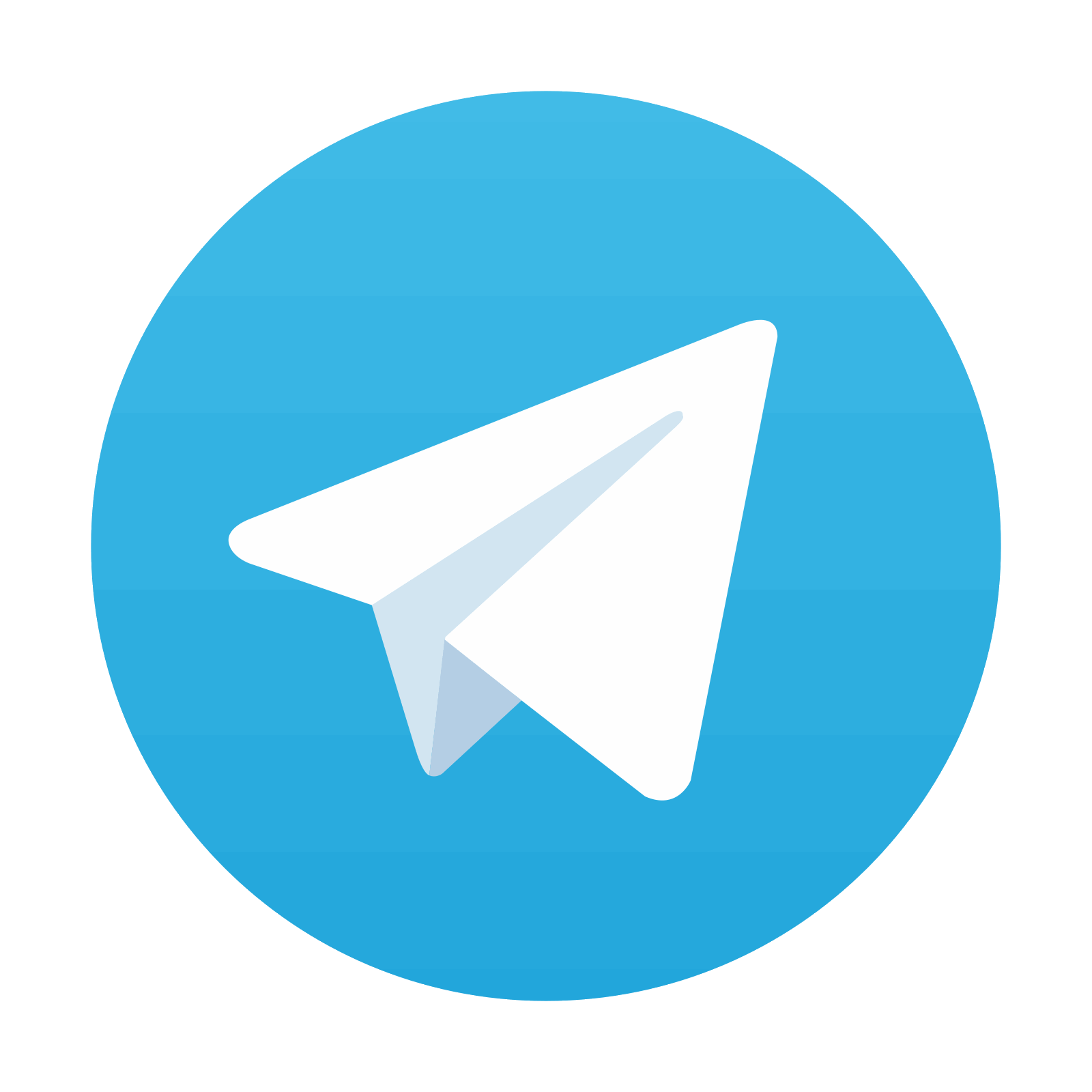
Stay updated, free articles. Join our Telegram channel

Full access? Get Clinical Tree
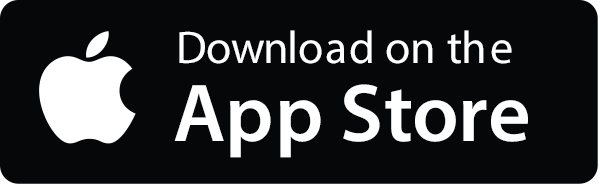
