Medication adherence
Adverse effects/intolerance
Adequate treatment duration
Outcome definition (improvement/response/remission)
Inclusion of non-antidepressant concomitant treatments
Phenocopies/medical comorbidity
Comparison groups for TRD cohort
A related problem is the range of medical comorbidities that may contribute either to persistence of MDD or to depressive symptoms. Two examples are hypothyroidism (regardless of etiology) and sleep apnea, both of which may contribute to apparent treatment resistance. While the importance of these factors in unselected clinical populations is difficult to estimate, unless specifically excluded, they may contribute heterogeneity to the population of patients with TRD. (As with adverse effects, these factors may themselves be genetic, of course.)
While these TRD phenocopies undoubtedly contribute to misclassification and thus diminish the power of association studies to identify risk variants, the greatest limitation in such studies remains the paucity of samples available for study. Indeed, across psychiatric genomics, after decades of argument about correctness of phenotypes and other strategies required for success, efforts to identify common genetic association have begun to succeed largely because of adequately powered sample cohorts combined via meta-analysis. As with antidepressant response genomics as a whole [30], it is fair to say such sample sizes have not yet been achieved in the study of treatment-resistant depression.
As such, a key next step in the study of treatment resistance is achieving such cohorts. (While the precise sample size needed for success in genome-wide study varies widely, nearly all phenotypes have yielded to cohorts of 10,000 cases or more.) Of course, if this process has proven challenging for disease phenotypes, collecting cohorts requiring establishment of diagnosis plus two (or more) treatment trials is likely to be substantially more so.
There are at least three possible next steps, and all of these may be required in order to find reliable, replicable associations. First, investigators will likely need to share data in the context of a consortium. Second, the largely untapped data from large randomized trials conducted by pharmaceutical companies will need to become accessible. And finally, novel means of identifying cases and controls from large-scale biobanks, registries, or repositories may be required to augment the data available from costly prospective clinical trials (Table 4.2). An example of such an initiative was the rare variant study of TRD described earlier, in which treatment response was characterized based on electronic health records [28]. This approach may also facilitate the development of integrated risk models incorporating both genetic and clinical risk predictors [27].
Table 4.2
Study designs for TRD
Source | Outcome data | Treatment data | Randomization | DNA | Sample size | Relative cost |
---|---|---|---|---|---|---|
Clinical trial | +++ | +++ | Yes | +++ | + | $$$ |
Cohort study or disease registry | ++ | ++ | No | +++ | +/++ | $$ |
Health claims data | + | + | No | Requires recontact | +++ | $ |
Electronic health record | ++ | ++ | No | Requires recontact or biobank | +++ | $ |
TRD remains an area of great clinical importance in psychiatry, contributing substantially to morbidity and healthcare cost. The lack of success in identifying reliable risk variants to date likely reflects a problem of engineering, not science: investigators will need to collect sufficiently large cohorts to afford adequate statistical power. In light of the potential benefit that early identification of TRD risk could afford, however, further efforts to efficiently characterize such cohorts are sorely needed.
References
1.
Alexanderson B, Evans DA, Sjoqvist F (1969) Steady-state plasma levels of nortriptyline in twins: influence of genetic factors and drug therapy. Br Med J 4(5686):764–768PubMedCentralCrossRefPubMed
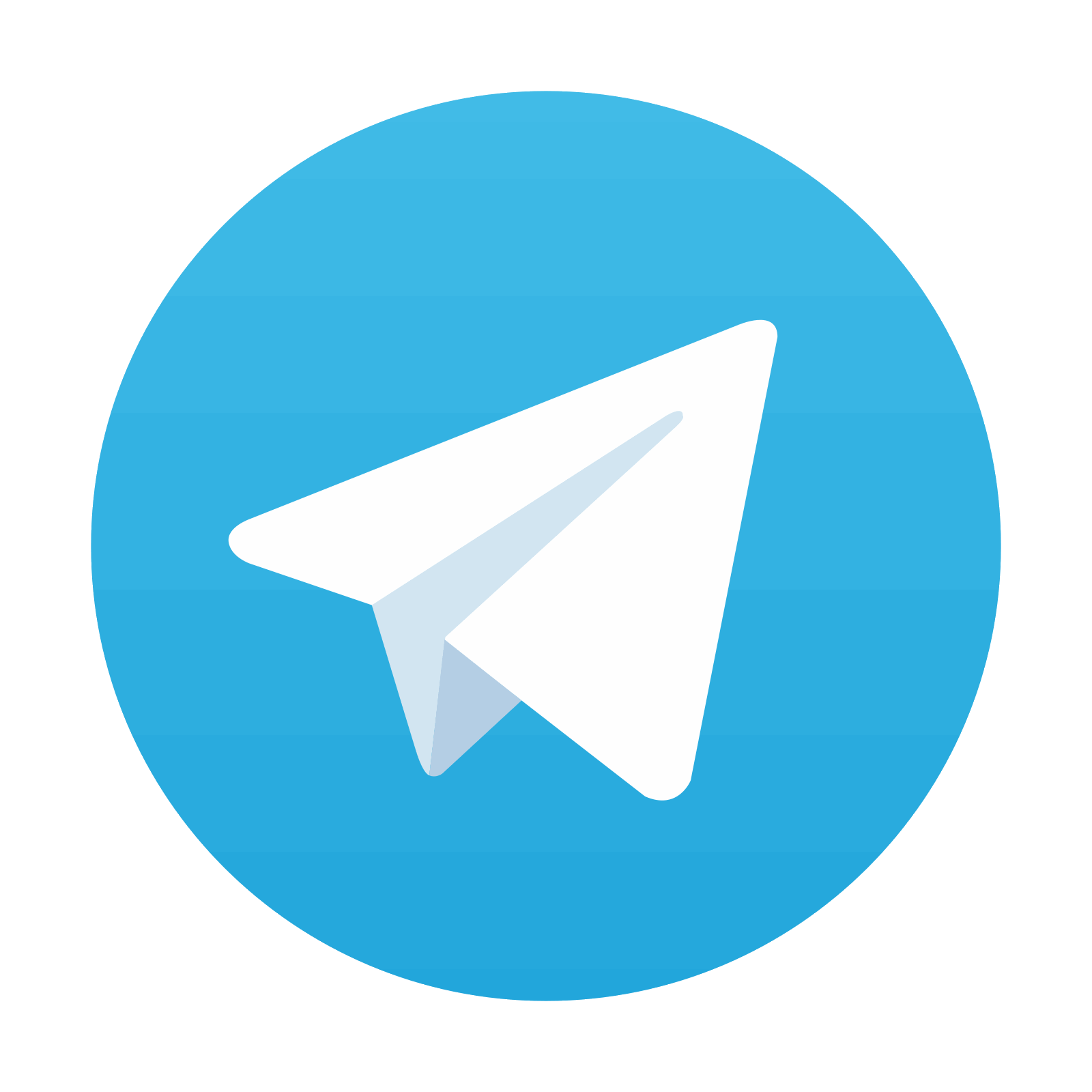
Stay updated, free articles. Join our Telegram channel

Full access? Get Clinical Tree
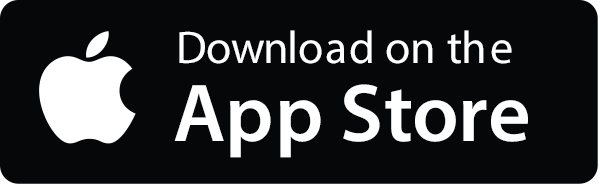
