Given the substantial growth in frequency and expense of spine deformity surgery, and the general economic landscape of the health care system, health economics research has an important role in the literature on adult spinal deformity (ASD). The purpose of this article is to provide an update on the current state of health economics studies in the ASD literature and to introduce areas in which health economics might play some additional role in future research on ASD.
Key points
- •
The last decade has witnessed a substantial increase in the frequency and expense of spine deformity surgeries.
- •
With increasing economic scrutiny, spine deformity surgeries must be done more efficiently, and maintaining quality of care in the face of such economic pressures requires continued development in health economics research in adult spinal deformity.
- •
This article reviews the literature on the costs and health-related quality-of-life outcomes after adult deformity surgery, as well as cost effectiveness and decision modeling.
- •
This article introduces future areas of health economics research in adult spine deformity, including bundled payments and demand for surgical treatment.
Introduction
Health economics is a broad field, covering a wide range of topics from single-center cost-effectiveness studies to large-scale health services research. The discipline primarily concerns questions of health policy, including the determinants and distribution of health and access to health care, optimal delivery of health care services, and optimal allocation of resources among competing health care uses. The field draws heavily from microeconomic theory as well as applied econometrics and statistics.
When considered in the context of specific medical conditions or treatments, health economics generally takes the form of cost- or comparative-effectiveness studies (including value-based insurance design and cost sharing), utilization and demand analysis, and bundled payment analysis (including calculation and guidance regarding implementation). In the general spine literature, health economics studies have traditionally focused on cost effectiveness and utilization of treatments for low back pain, with particular emphasis on the trends, expense, quality-of-life improvements, and cost effectiveness of spine surgery. However, only recently has health economics made its way to the literature specifically on adult spinal deformity (ASD).
The purpose of this article is 2-fold. First, we hope to introduce areas in which health economics might play some role in future research on ASD. Second, the article provides an update on the current state of health economics studies in the ASD literature. We do not intend for this article to be a comprehensive guide for how to effectively evaluate health care programs from an economic perspective. We leave this to the many health economics experts and texts that have already laid out such guides in much more detail and accuracy.
Introduction
Health economics is a broad field, covering a wide range of topics from single-center cost-effectiveness studies to large-scale health services research. The discipline primarily concerns questions of health policy, including the determinants and distribution of health and access to health care, optimal delivery of health care services, and optimal allocation of resources among competing health care uses. The field draws heavily from microeconomic theory as well as applied econometrics and statistics.
When considered in the context of specific medical conditions or treatments, health economics generally takes the form of cost- or comparative-effectiveness studies (including value-based insurance design and cost sharing), utilization and demand analysis, and bundled payment analysis (including calculation and guidance regarding implementation). In the general spine literature, health economics studies have traditionally focused on cost effectiveness and utilization of treatments for low back pain, with particular emphasis on the trends, expense, quality-of-life improvements, and cost effectiveness of spine surgery. However, only recently has health economics made its way to the literature specifically on adult spinal deformity (ASD).
The purpose of this article is 2-fold. First, we hope to introduce areas in which health economics might play some role in future research on ASD. Second, the article provides an update on the current state of health economics studies in the ASD literature. We do not intend for this article to be a comprehensive guide for how to effectively evaluate health care programs from an economic perspective. We leave this to the many health economics experts and texts that have already laid out such guides in much more detail and accuracy.
Trends in spinal deformity surgery
From a clinical perspective, ASD is defined simply as an abnormal curvature of the spine in patients who have completed their growth. The condition is distinct from more common spine problems such as lumbar spinal stenosis or herniated discs. However, despite clinical distinctions between ASD and other spine problems, there is some overlap in the treatment of spinal deformity and treatment of more common spine conditions such as herniated discs. In fact, the application of complex spinal fusion surgeries to more common spine problems (eg, incorporating spinal fusions with discectomies for patients with herniated discs and radiculopathy) has been under scrutiny over the last several years.
Overlap in treatment complicates the identification of patients with ASD based purely on diagnosis-related groups or procedure codes. To briefly review the trends in spinal deformity surgery, we therefore follow the literature and identify spine deformity surgeries based on the International Classification of Diseases, Ninth Revision, Clinical Modification (ICD-9-CM) principal diagnosis codes.
Fig. 1 presents total discharges by payor type undergoing surgical treatment of spinal deformity from 1993 through 2010. From 2000 to 2010, the figure illustrates a 4-fold increase in the number of spine deformity surgeries for Medicare patients and a 70% increase for managed care patients. The Medicare population, therefore, accounts for most of the growth in spine deformity surgeries of the past decade. Over this same period, the mean length of stay for Medicare patients decreased from 7.4 days to 6.2 days (16%), with a similar 16% decrease in length of stay for managed care patients (from 6.7 to 5.6 days). Yet, despite the apparent increase in efficiency based on reduced length of stay, the average charge per inpatient stay has increased more than 230% for Medicare patients and approximately 190% for managed care patients from 2000 to 2010, as illustrated in Fig. 2 .
Combining the average charge figures with the total number of discharges, we estimate total charges for spine deformity surgeries in Fig. 3 . Over the last decade, total charges for spine deformity surgery for Medicare patients increased from $56 million to more than $958 million, a 16-fold increase, whereas total charges for the managed care population increased nearly 4-fold from $344 million to more than $1.7 billion. Of course, charges are a flawed measure of either costs or payments, but trends in charges over time are still informative and relevant to the growing health economics literature in this area. Together, Figs. 1–3 indicate a substantial increase in the frequency and expense of spine deformity surgeries over the last 10 years.
As a reference point, the total number of discharges for all spine nondeformity primary diagnosis codes increased from 675,470 in 2000 to 813,849 in 2010 (20% increase). For the Medicare and managed care populations, respectively, total discharges increased from 220,793 to 328,750 (49%) and from 320,274 to 332,935 (4.0%) over the same period. These figures are dwarfed by the 400% and 70% increase in spine deformity discharges among the Medicare and managed care populations, respectively. Average charges for nondeformity spine surgery have increased more than average charges for deformity surgeries; however, when adjusting for the decrease in length of stay in spine deformity surgeries over the same period, the average charge for deformity surgeries has increased at a faster rate than that for nondeformity surgeries among Medicare patients. These data are summarized in Table 1 .
Nondeformity | Deformity | |||
---|---|---|---|---|
Medicare | Managed Care | Medicare | Managed Care | |
Average Charge | ||||
2000 | $15,167 | $16,278 | $49,399 | $56,730 |
2010 | $52,415 | $59,133 | $164,538 | $164,285 |
Length of Stay | ||||
2000 | 4.4 | 2.9 | 7.4 | 6.7 |
2010 | 4.0 | 2.9 | 6.2 | 5.6 |
Charge per Day | ||||
2000 | $3447 | $5613 | $6676 | $8467 |
2010 | $13,104 | $20,391 | $26,538 | $29,337 |
% Increase in Charge per Day | 280.1 | 263.3 | 297.5 | 246.5 |
Health economics research in ASD
Given the intense economic scrutiny placed on spine fusion surgeries in general, the comparison above is particularly telling—in the likely near future, complex spine deformity surgeries must be done more efficiently, and maintaining quality of care in the face of such economic pressures requires continued development in health economics research in ASD.
There are 2 areas in the ASD literature in which health economics research has become relatively standard: (1) analyses of health-related quality-of-life (HRQOL) outcomes after surgery and (2) analyses of the cost of surgical treatment. Two additional areas in which health economics research in ASD is slowly emerging but not yet fully adopted include cost-effectiveness studies and decision modeling. We review the literature in each of these 4 areas in more detail below.
Health-Related Quality-of-Life Outcomes
Of the existing quality-of-life studies on surgical treatment of ASD, authors often compare pre- and postoperative HRQOL measures and analyze whether significant improvements in HRQOL were observed. Common instruments in the spinal deformity literature include the disease-specific 22-item and 30-item Scoliosis Research Society (SRS) questionnaires (SRS-22 and SRS-30, respectively), the regional-specific Oswestry Disability Index (ODI), and Medical Outcomes Study Short Form 36 and 12-item general health questionnaires (SF-36 and SF-12, respectively), all of which have been validated and applied in spinal deformity. Adopting these measures, Glassman and colleagues reported a significant improvement in patients’ SRS-22, SF-12 Physical Component Scores, and ODI scores after surgery. The authors also found that HRQOL measures improved the most over the first year after surgery, stabilizing thereafter with generally no significant change from 1-year to 2-year follow-up. Many other studies have similarly found significant improvements in HRQOL scores after surgical treatment for ASD.
Concerned that improvement in HRQOL scores does not necessarily translate into a clinically discernible qualitative improvement in HRQOL, investigators have also studied the proportion of patients reporting a minimum clinically important difference (MCID) in HRQOL improvement. To this point, however, there is little agreement in the literature regarding the appropriate MCID for different outcome measures. For example, in a comprehensive literature review covering both general spine and spine deformity, Yadla and colleagues cite 15 studies reporting pre- and postoperative ODI scores, with an average improvement of 15.7 points and MCID of 3.1–32.3 points. Bagó and colleagues reported the MCID in SRS scores of 0.6 points in the pain domain, 0.3 points in functional activity, 1.3 points in self-image, and 0.4 points in mental health.
The application of MCID in HRQOL research remains an area of debate, and although the general concept has been around for more than 20 years, much of the literature remains in flux. Conceptually, MCID avoids the use of statistically significant results that are qualitatively meaningless and dovetails with the general distinction between practical relevance and statistical significance. But despite its intuitive appeal, the appropriate estimation and use of MCID remain unclear. At least 2 general estimation strategies have been espoused in the literature: (1) the anchor-based approach and (2) the distributional approach. The anchor-based approach uses some external responses, such whether the patient reported feeling better or worse after treatment, and calculates the MCID by comparing the HRQOL of patients in the better group with those of the worse group. Conversely, the distributional approach calculates MCID based on the within-sample change in HRQOL scores relative to some measure of variability in the distribution. The distributional approach, therefore, bases its calculation of MCID on existing HRQOL responses and does not rely on an external criterion.
Each approach carries with it some potential flaws. For example, in practice, the external criterion used in the anchor-based approach is often some other subjective questionnaire. In such a case, it can be argued that the MCID is essentially converting one subjective measure to match another. Conversely, the distributional approach attempts to provide a statistical framework around the calculation of the MCID. For example, some investigators estimate MCID as one standard error above the mean score. Far from an alternative to statistical significance, this approach largely amounts to changing the threshold for statistical significance. The point here is not to argue for or against the MCID but rather to discuss some of the potential conceptual problems in its calculation. In spirit, the MCID is an excellent concept and could be an important part of HRQOL research; however, to date, many questions remain regarding how the measure is calculated and ultimately its appropriateness in identifying evidence-based practices.
Although there is increasing awareness in the literature regarding the importance of HRQOL research, few existing studies formally analyze the potential causal factors underlying patients’ self-reported HRQOL improvements after ASD surgery. The determinants of HRQOL improvements are important given the potential disconnect between common clinical and radiographic outcomes (eg, fusion status) and a patient’s quality of life after spine surgery. As such, HRQOL measures may provide clinically relevant information separate from radiographic measurements. A patient-centered, evidence-based decision analysis should, therefore, incorporate not only the standard radiographic measures but also the patient’s predicted HRQOL improvement from surgery. Improved HRQOL analyses can also be used to develop more formal cost-effectiveness studies regarding the incremental benefit and costs of surgical versus nonsurgical treatment.
Unfortunately, statistically modeling the causal factors of HRQOL improvements is far from straightforward. One difficulty lies in the multidimensional nature of existing questionnaires used in the ASD literature. For example, the SRS-22/30 questionnaire yields a summary score in each of 5 dimensions (functional activity, self-image, mental health, pain, and satisfaction) in addition to an overall summary score calculated as a weighted average of each individual domain. But an average across domains does not account for patient preference (ie, patient 1 may care only about functional activity and pain, whereas patient 2 may weigh more heavily their self-image and mental health). Researchers are then left to answer, on their own, what does it mean if a surgical patient improves in functional activity but deteriorates in pain? Is this patient better or worse off after surgery?
This problem does not emerge in all HRQOL questionnaires. For example, researchers using the EQ-5D, the SF-6D, or the Health Utilities Index (HUI1, HUI2, or HUI3) have developed scoring algorithms to collapse the HRQOL responses in each dimension into a single index (also termed a utility score ). Coupled with a time component, the index scores from the SF-6D, EQ-5D, or HUI are used to estimate quality-adjusted life-years (QALYs). As a simple example, assume a study has collected HRQOL responses at 6-month and 1-year follow-up examinations, which yield index scores of 0.66 and 0.75 and a QALY of (0.66*[6/12] + 0.75*[6/12]) = 0.33 + 0.375 = 0.705.
The index scores of 0.66 and 0.75 in the above example would be derived from published algorithms to convert HRQOL responses to a single index. These scoring algorithms are generally based on population-level studies, in which individual health states elicited from the HRQOL questionnaire of interest are valued by patients through a series of questions intended to measure patient preferences. The standard gamble (SG), time trade-off (TTO), and visual analog scale are some of the most common methods of measuring preferences over health states used in the literature. Economists are generally fonder of the SG or TTO because these methods are choice based, requiring patients to explicitly compare alternative health states with one another.
Most HRQOL questionnaires have far too many possible responses to measure each health profile explicitly. Therefore, many scoring methods culminate in a statistical model that can be used to estimate an index score from all possible responses to the HRQOL questionnaire. Published algorithms are currently available in the literature based on the EQ-5D, the SF-6D (derived from either the SF-36 or the SF-12), and the HUI questionnaires. Unfortunately, research in ASD has not consistently relied on generic health-profile questionnaires, such as the EQ-5D or the SF-36, focusing instead on disease-specific or regional-specific questionnaires, such as the SRS-22/30 or the ODI. Directly valuing responses to these questionnaires using the SG or TTO methods is somewhat unclear because the questionnaires do not elicit a general health profile but rather a specific measure of certain characteristics relevant to the patient population in question. As such, statistical models have been developed to convert ODI scores to SF-6D utility scores.
In applying statistical models to estimate index scores (whether scoring the SF-6D or EQ-5D directly, or converting the ODI to the SF-6D), researchers should be aware of at least 2 important issues:
- 1.
Although there may be exceptions, the standard scoring algorithms in the literature are based on population studies, and the algorithms will generally differ depending on the population for which the algorithm was based. For example, in comparing algorithms derived from a US versus UK population, investigators have found potentially significant differences in the conclusions regarding the estimated treatment effect. Although algorithms for the EQ-5D have been assessed in both US and UK populations, not all HRQOL questionnaires have been separately studied in different countries (including, for example, the SF-6D, for which there is not yet a published algorithm derived from a US population). Similarly, the algorithm developed to convert ODI scores to the SF-6D was not derived solely from spine deformity patients but rather a slightly wider range of patients undergoing lumbar fusion for degenerative disorders.
- 2.
In applying statistical models to estimate index scores, researchers should also take caution in interpreting predicted index scores for a given patient. This is because regression-based models are inherently estimating the conditional mean function and may on average provide accurate predictions, whereas for a given patient provide relatively inaccurate predictions. Researchers are, therefore, encouraged to focus on the average prediction rather than an individual prediction.
In response to these concerns, one additional approach worth mentioning involves the concept of dominant health states. For example, the EQ-5D responses are ranked from 1 to 3 in each of 5 domains, with 1 being no problems, 2 being some problems, and 3 being significant problems. The resulting health state for a given patient can then be easily characterized based on the patient’s response to each question (eg, 11211 or 22212, denoting the patient’s response of 1, 2, or 3 in each of the 5 domains). These health states can then be ordered, with 11111 dominating 21111. Other comparisons, such as 11211 versus 12111, would be considered unordered health states because both states have 4 responses of no problems and one response of some problems. This method, therefore, avoids assigning weight to each domain and relying on statistical models to estimate a single index, with the downside that many health state comparisons would be indistinguishable.
Still, whether the analysis considers a disease-specific questionnaire such as the SRS-22/30 or a generic profile such as the EQ-5D, the researcher may remain interested in analyzing individual HRQOL domains. Here, the most common statistical tools in current practice may fall short. For example, the EQ-5D responses are inherently discrete, ordered, and likely correlated across domains. With observational data, estimating the effect of surgery on HRQOL will require statistical tools that are robust to the complicated nature of the dependent variable. Although multivariate ordered logit or probit models can be estimated, these tools are not yet part of the standard toolbox for clinical researchers and may be considered unnecessarily complicated by some audiences. Future research in HRQOL may need to embrace these more complicated tools to accurately estimate the effects of interest.
One final point regarding HRQOL: the literature on condition-specific HRQOL questionnaires versus generic health profiles and the distinction between non–preference-based versus preference-based questionnaires is broad and well beyond the scope of this article. For the curious reader, many excellent texts are relevant to this area (often in the context of cost-effectiveness analyses).
Costs of Surgery
Although there have been several cost studies in the general spine fusion literature, only recently have investigators begun to study the cost of medical treatment of ASD. Glassman and colleagues found that among 68 ASD patients with nonoperative treatment, mean treatment cost over 2 years was $10,815. Mean treatment costs increased with symptoms—$9704 for low-symptom patients, $11,116 for midsymptom patients, and $14,022 for high-symptom patients. In a study of surgical treatment of adolescent idiopathic scoliosis, Kamerlink and colleagues reported mean costs of $32,836. To our knowledge, however, the costs of surgical treatment of ASD have not been reported and analyzed in the literature. Advances in this area will help determine the predictors of cost for surgical care of spinal deformity and allow comparisons among different centers treating ASD, ultimately identifying best practices in the treatment of ASD.
Because of the highly skewed nature of the distribution of costs for surgical treatment of spinal deformity, an appropriate analysis of the costs of surgery requires a careful statistical approach. The 2 most common statistical approaches to cost analyses include:
- 1.
Analyze a log-transformed model of costs, wherein the costs of surgery (dependent variable) are measured in logs such that the distribution is less skewed and relatively more bell shaped. The intention of such a transformation is to restore homoscedasticity and normality of the residuals. However, one drawback of a log transformation is that coefficients are then interpreted as the estimated effect on the geometric mean cost rather than the arithmetic mean. Although investigators have suggested retransformations that may address this issue, assumptions required for an accurate retransformation are not satisfied in many cases.
- 2.
Estimate a generalized linear model (GLM) of costs as a function of other covariates of interest. Unlike ordinary least squares with a log-transformed dependent variable, the use of GLM with log link function preserves the standard interpretation of regression coefficients in terms of the effect of an independent variable on average costs. Extensions to the GLM approach have also been proposed in which the regression and link parameters are estimated semiparametrically. Although more robust, this method carries with it a substantial loss of parity compared with the standard GLM and particularly ordinary least squares; however, the estimation technique proposed by Basu and Rathouz 34 should be considered if one is concerned about assumptions regarding the GLM link function and if advanced econometric tools are appropriate for the intended audience.
Aside from statistical modeling, another difficulty in the area of cost analysis involves how to define cost. Although seemingly simple, this is not a straightforward question. Providers may be most interested in the total or direct costs of surgery. Studies of direct costs may be particularly relevant to compare across hospitals because overhead and other indirect costs are not identified specifically with a given inpatient stay and instead are allocated to inpatient stays using general accounting algorithms. Such an allocation introduces additional noise into the total cost measure that is largely hospital specific and may not be reflective of actual costs incurred for surgical treatment.
Payors may also be interested in observed costs of surgery if they intend to negotiate lower rates; however, payors are generally interested in reimbursements or allowed amounts, because such costs are what the payors actually incur. Reimbursements may also be of interest from a policy standpoint because these amounts represent the dollar amount ultimately spent for the procedure in question.
Charges are sometimes used as a proxy for costs. Although many researchers do not consider charges to be an appropriate proxy for costs, charges still provide some level of information regarding the cost of care. Combined with actual cost data, an analysis of charges may still be useful, for example, as an indicator of a provider’s markup on medical devices. And combined with cost and reimbursement data, charges would provide a relatively complete picture of the provider’s negotiated rates with suppliers and payors. In a perfectly transparent system, such data would be extremely useful in synchronizing health care prices and reimbursement rates among comparable providers and payors. All measures of cost (direct costs, total costs, charges, or reimbursements), therefore, have some relevant information, particularly if analyzed together across providers.
In practice, researchers rarely have the luxury of choosing which measure of costs to adopt. Costs are instead determined by the best measure available in the data. Sometimes, this means estimating costs based on charges or utilization data, in which case estimating costs often can be done with one of 2 common methods—a bottom-up or a top-down approach. Although a thorough description of different costing methods is beyond the scope of this article, the appropriate estimation of costs is a common concern in large-scale studies (particularly when using publically available or restricted-use datasets), for which the Chapko and colleagues and Tumeh and colleagues studies, among others, should prove as valuable resources.
Cost-Effectiveness Studies
In a general sense, cost effectiveness has become a critical component in the economic evaluation of medical care, demonstrated by numerous initiatives encouraging more cost- and comparative-effectiveness research. For example, the United States recently created the Patient-Centered Outcomes Research Institute (PCORI) as part of the Patient Protection and Affordable Care Act. PCORI was specifically created to promote and ultimately fund the development of comparative effectiveness research in health care. PCORI and other similar initiatives illustrate a general movement toward a value-based health care economy and increasing focus on both costs and patient-reported outcomes in the economic evaluation of health care programs.
Cost effectiveness is generally measured by the dollar value of health care services. Operationally, researchers calculate cost effectiveness as the ratio of costs to some measurable outcome. The outcome of interest is often the improvement in QALYs but may also be some other metric such as symptom-free days or number of positive screenings. Investigators often reserve the term cost-utility analysis (CUA) to describe cost-effectiveness studies with a utility-based outcome measure (e.g., QALYs), as opposed to cost-effectiveness studies with a non–utility-based outcome, such as symptom-free days.
Although there is increasing awareness regarding the importance of cost-effectiveness research, few cost-effectiveness studies currently exist in the general spine literature. Of the few studies available, the measure of interest largely involves the cost effectiveness of surgical treatment in the lumbar spine, including disc herniation and degenerative spondylolisthesis. For example, as part of the Spine Patient Outcomes Research Trial study, Tosteson and colleagues studied the cost effectiveness of surgical versus nonoperative treatment of lumbar disc herniation. The authors estimated costs based on patient-reported utilization multiplied by Medicare per-unit allowable payment rates and measured outcomes as QALYs based on the EQ-5D. Based on total costs, the study reported an incremental cost-effectiveness ratio of $69,403 for all patients and a ratio of $34,355 for Medicare patients. Based on direct costs only, the incremental cost-effectiveness ratio increased to $72,181 for all patients and $37,285 for Medicare patients.
Glassman and colleagues analyzed the cost effectiveness of single-level posterolateral lumbar fusion for 93 patients, with cost data based on actual reimbursements from third-party payors and outcomes measured as QALYs based on the SF-6D. As part of the cost of surgery, the authors included outpatient visits up to 6 months after surgery. The authors reported an average reimbursement amount per QALY of $164,261 after 1 year and $154,865 after 2 years, although estimates varied depending on the patient cohort of interest.
Although interest in cost-effectiveness research seems to be growing in the spine literature, studies generally do not focus specifically on surgical treatment of ASD and often do not have precise cost information for the episode of surgical care, relying instead on outside data sources, including surveys of the existing literature, high-level Medicare payment rates applied to observed utilization, or total reimbursements from third-party payors. Additional costs incurred after initial treatment should also be considered (eg, costs of revision surgery, follow-up visits) as well as additional so-called indirect benefits, such as improved productivity. These additional considerations, however, are rarely explicitly quantified in existing cost-effectiveness studies. Regardless, the literature on the cost effectiveness of spine deformity surgery is new, and there are significant research opportunities in this area.
Decision Analysis
Decision analysis or decision modeling derives largely from statistical decision theory, drawing from Bayesian statistics as well as expected utility theory. Decision analysis also combines several sources of information (often involving multiple treatment options) into one analysis and is, therefore, distinct from pure cost analyses or cost-/comparative-effectiveness studies. Another area in which decision analysis is distinct from other analyses discussed above is in the explicit consideration of uncertainty and variability (including heterogeneity across patients or patient groups).
Few decision analysis studies currently exist in the general spine literature. For example, in 2 related studies involving degenerative spondylolisthesis, Kuntz and colleagues studied fusion with and without instrumentation, and Kim and colleagues analyzed decompression with or without fusion. Both studies relied on simulations to form expected benefits and costs of alternative treatments after 10 years. Kuntz and colleagues relied entirely on data collected from the literature, including average complication rates, fusion rates, and costs, whereas Kim and colleagues relied on a combination of observational data and a review of the literature. Both studies measured costs based on total hospital costs, including direct costs, such as implants, as well as indirect costs, such as administration and facility usage.
Kuntz and colleagues reported an incremental cost effectiveness of approximately $3.1 million per additional QALY for instrumented fusion compared with noninstrumented fusion; however, the results were sensitive to assumptions regarding the rate of patients with symptom relief after instrumented fusion. Kim and colleagues reported an incremental cost effectiveness of $185,878 per additional QALY for decompression with fusion compared with decompression alone, again with significant sensitivity regarding assumptions on the magnitude of QALY improvements over time, costs, and revision rates.
As is clear from the findings discussed above, there is some overlap in the characterization of cost effectiveness and decision analysis. We chose to label the Kuntz and colleagues and Kim and colleagues studies as decision models rather than pure cost-effectiveness studies for 3 primary reasons: (1) both studies relied, at least in part, on additional data from the literature (eg, on the average complication rate after specific types of surgery); (2) both studies considered multiple outcomes after surgery, the associated costs of such outcomes, and the probability associated with each outcome under consideration; and (3) both studies projected costs and benefits 10 years out. These features are fundamental aspects of decision analysis and easily differentiate the studies of Kim and colleagues and Kuntz and colleagues from the cost-effectiveness studies discussed previously.
As with our discussion of cost effectiveness, decision analysis studies remain relatively rare in the spine literature and have not yet caught on in spinal deformity. This is partly because of a lack of data and prior research on which to build an appropriate decision analytic model. For example, most studies of pseudoarthrosis or proximal junctional kyphosis (2 of the more commonly cited concerns among surgeons treating ASD) are still based on small patient cohorts. Progress is being made in this area with the collaboration of multiple centers, including the Spinal Deformity Study Group and the International Spine Study Group, with the goal of collecting data on larger cohorts of patients across several different geographic regions undergoing treatment from several different surgeons. As these datasets continue to grow, we can better estimate the HRQOL outcomes and costs of surgical and nonsurgical treatment for ASD, the rate of different complications after surgical treatment, the costs associated with such complications (eg, revision surgery), and eventually a breakdown of these variables by the type of surgical procedure or technique. Combining all such data would provide a solid foundation for a wide range of decision analytic modeling in spinal deformity.
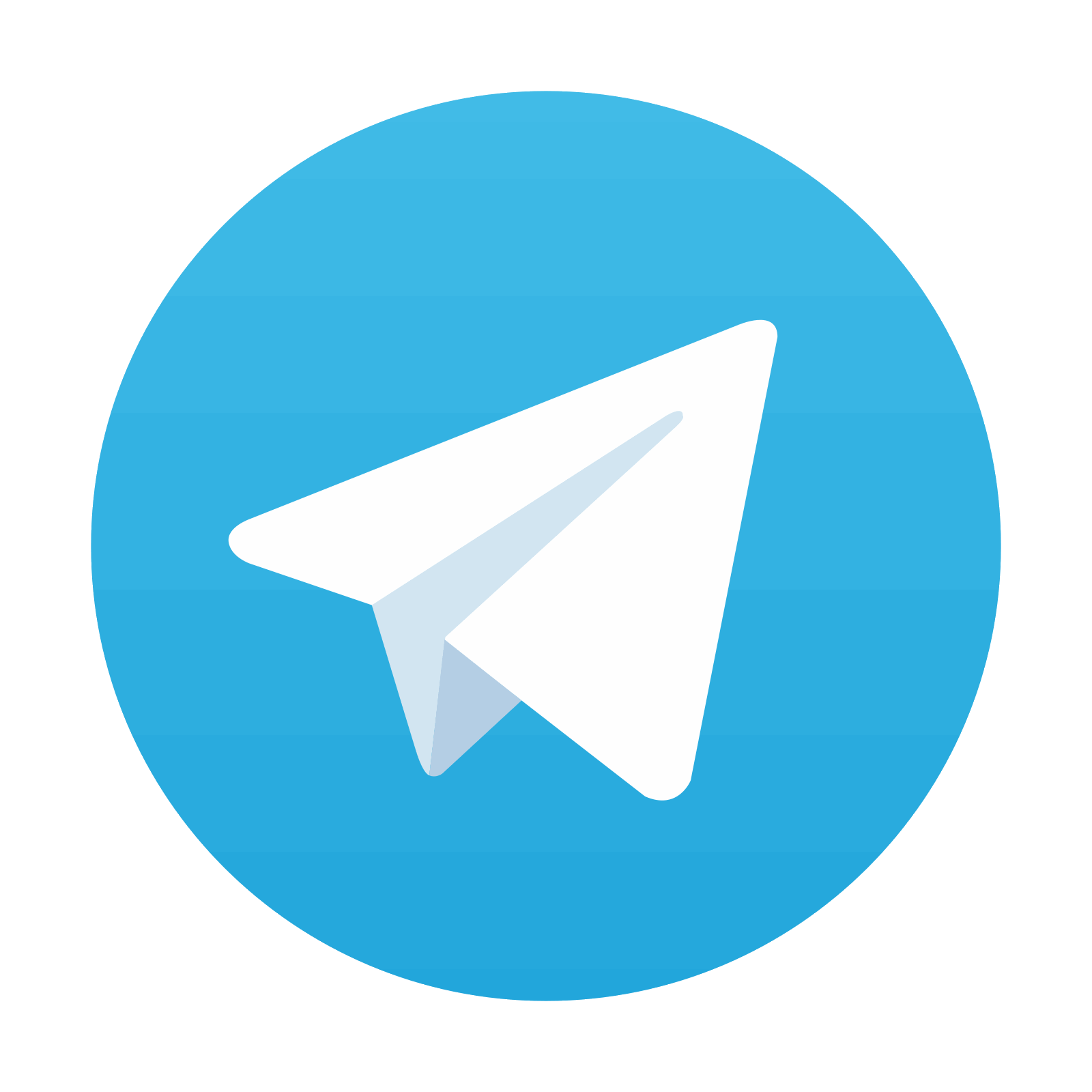
Stay updated, free articles. Join our Telegram channel

Full access? Get Clinical Tree
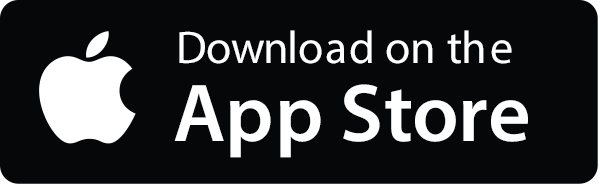
