Fig. 9.1
Frequency-domain HRV analysis. (a) Time series of R–R intervals in a 31-year-old male subject. The time series was resampled at evenly spaced intervals (2 Hz). (b) The decomposition of the same time series into high-frequency (HF: 0.15–0.40 Hz), low-frequency (LF: 0.04–0.15 Hz), and very-low-frequency (0–0.04 Hz) components. These three traces were obtained by inverse Fourier transforming the corresponding frequency bands. (c) Power spectral density (power spectrum) of the same HRV time series. HF and LF areas under the power spectrum are equivalent to the variance of the HF- and LF-component time series, respectively, shown in (b)
HF power is generally accepted to reflect RSA, which is mediated by parasympathetic activity. Therefore, HF power is taken as an index of cardiac parasympathetic tone. However, it has been shown that sympathetic cardiac blockade augments vagally mediated heart rate oscillations, including the HF component [59, 60]. Therefore, the HF power is, in some degree, affected by sympathetic tone and may not be a pure parasympathetic index [61].
In contrast, the interpretation of LF-power-related indices is controversial. Many studies have presumed that LF power reflects both sympathetic and vagal influences or that LF power in normalized units provides an index of cardiac sympathetic tone, and the ratio of LF to HF power (LF/HF ratio) indicates sympathovagal balance [35, 39]. In this view, an increase in LF power is assumed to indicate an increase in sympathetic activity, and a higher value of the LF/HF ratio indicates a shift of sympathovagal balance toward a sympathetic predominance. In short-term HRV recordings under experimental conditions affecting autonomic response, the relative power contribution of the LF component is considered a marker of sympathetic activity [39, 40]. However, many studies using pharmacological, physiological, or psychological manipulations affecting sympathetic activity and HRV have challenged the putative association of cardiac sympathetic activity with LF power [41, 42]. One important fact is that vagal blockade dramatically reduces LF power [62], whereas sympathetic blockade has no significant effect [42]. The available data suggest that over a wide frequency range including the LF and HF bands, the HRV power spectrum is mainly determined by the parasympathetic system [44]. It has also been suggested that LF power is an index of baroreceptor reflex gain (or sensitivity) because of the contributions from oscillations in blood pressure and vasomotor activity to this band [43, 63, 64].
More controversial is the interpretation of LF power and the LF/HF ratio in patients with a marked reduction of ventricular function. In such patients, whereas sympathetic activity is clearly elevated, a decrease in LF power and the LF/HF ratio is commonly observed and is associated with increased risk of mortality [47, 52]. Therefore, the clinical significance of the association of cardiac sympathetic overactivity with LF power-related indices is questionable.
9.3 Nonlinear HRV Indices and Sympathetic Activity
In the framework of linear stochastic processes as described by autoregressive models, the power spectrum provides a complete characterization of the process if the stationarity condition is fulfilled [65]. However, many real-world signals including HRV time series cannot be fully characterized based on the assumption of stationary linear processes and display much more complex behavior. To quantify such nonlinear properties, concepts and analysis methods developed in nonlinear dynamics and statistical physics have been applied to HRV analysis. As a result, various types of nonlinear HRV indices have been proposed: e.g., scaling exponents characterizing fractal and long-range correlation characteristics [51, 66], multifractal properties [67], Poincaré plot-based indices [68], entropy measures [69–71], symbolic pattern statistics [70, 72], and non-Gaussian properties [73]. Some nonlinear indices are expected to provide information on HRV characteristics complementary to that from traditional linear methods, as well as better diagnostic and prognostic information. However, their interpretations are not yet fully elucidated. Among nonlinear indices suggesting clinical significance, a few, such as the short-term scaling exponent α 1 [52, 53] and the non-Gaussianity index [54–56], are suggested to be associated with sympathetic activity. In this section, we discuss the association of sympathetic activity with these nonlinear indices.
9.3.1 Short-Term Scaling Exponent
The short-term scaling exponent α 1 is estimated by detrended fluctuation analysis (DFA; see Fig. 9.2 for details) [51]. The DFA is used to quantify long-range temporal correlations and fractal scaling properties of time series, as observed in, for example, fractional Brownian motion and fractional Gaussian noise. This method was developed to accurately quantify long-range temporal correlations embedded in nonstationary time series.


Fig. 9.2
Assessment of scaling exponents by detrended fluctuation analysis. The standard DFA procedure is as follows: (1) after subtracting the mean from each data point, (a) R–R interval time series {b(i)} is integrated (b); (2) the integrated time series {B(i)} is divided into equal-sized, nonoverlapping segments of length n (b); (3) in each segment, the mean squared deviation from the least-squares polynomial fit of degree k is calculated (k = 1 in c); the mean squared deviations are then averaged overall segments and the square root F(n) is calculated; this computation (steps [2] and [3]) is repeated over multiple time scales (window sizes) to characterize the relationship between F(n) and n (d); a linear relationship on a log–log plot of F(n) as function of n indicates the power–law scaling range; in this scaling range, the fluctuations can be characterized by a scaling exponent α, the slope of the linear relation between log F(n) and log n. In HRV analysis, short-term (<11 beats) and long-term (>11 beats) scaling exponents, α 1 and α 2, have commonly been calculated (e)
In HRV analysis, short-term (<11 beats) and long-term (>11 beats) scaling exponents, α 1 and α 2, have commonly been calculated [47]. Decreased short-term scaling exponent α 1 has been shown to have a more powerful prognostic power than traditional HRV indices in various post-AMI populations [74–76]. In the Danish Investigations of Arrhythmia and Mortality ON Dofetilide (DIAMOND-MI) trial, patients post-AMI with α 1 < 0.75 had a significantly increased risk of mortality (relative risk 3.0), and the new index was more strongly related to outcome than traditional HRV indices [47]. Additionally, in a study of consecutive patients with acute MI, reduced short-term fractal exponent was independently associated with recurrent nonfatal coronary events [77, 78].
Although the above findings suggest clinical significance for the short-term scaling properties of HRV, the physiological interpretation of α 1 remains unclear. In some studies, α 1 has been suggested to reflect the sympathovagal balance, similar to the LF/HF ratio [52, 53]. This is understandable in light of the mathematical relation between α 1 and the LF/HF ratio. Willson et al. showed mathematically that a scaling exponent estimated by DFA corresponds to standard spectral analysis [57, 58]. Based on the mathematical argument, it can be shown that α 1 is approximately related to the LF/HF ratio by the expression:
where the symbol “~” denotes proportionality. In agreement with this expression, a strong correlation between α 1 and the LF/HF ratio has been observed empirically. Therefore, α 1 reflects aspects similar to those characterized by the LF/HF ratio, although they are not exactly the same. As in the interpretation of the LF/HF ratio, the association of α 1 with sympathetic activity appears to be questionable.

9.3.2 Non-Gaussianity Index
Kiyono et al. proposed the non-Gaussianity index as potentially related to cardiac sympathetic over activity [54–56]. This index is calculated by multiscale probability density function (PDF) analysis to characterize the intermittent and non-Gaussian behavior of time series as shown in Fig. 9.3 [79, 80]. This type of multiscale analysis method was developed to characterize the intermittent fluctuations observed in hydrodynamic turbulence [81]. In this method, after detrending via least-squares polynomial fitting, the intermittent behavior of the HRV, such as the heterogeneity of the variance and burst-like patterns, is analyzed through the process of deformation of a non-Gaussian probability distribution (Fig. 9.3b, c) across scales of coarse graining (local averaging), and the observed non-Gaussian shape at each scale is quantified by the non-Gaussianity index λ s . The latter is defined as a parameter of a non-Gaussian distribution model (see Figs. 9.3 and 9.4 for details). The greater the λ s , the greater the proportion of large deviations over what is expected from the Gaussian distribution.



Fig. 9.3
Multiscale probability density function (PDF) analysis for intermittent time series. (a) An example of intermittent fluctuations generated numerically by a random cascade model [80]. (b) Probability-distribution deformation process across scales s of partial sums (s = 1, 4, 16 from top to bottom). Estimated PDFs (markers) from the time series (a) are plotted on log–linear scales. The observed non-Gaussian shape at each scale is quantified by the non-Gaussianity index λ s, which is defined as a parameter of a non-Gaussian distribution model (solid lines) [80]. A large value of λ s indicates a non-Gaussian shape with markedly fat tails and a peak around the mean value. For comparison, a Gaussian distribution is also shown (dashed lines). (c) Scale dependence of λ s 2. In this plot, a gradual decline in λ s 2 with increasing scale s indicates the process of convergence to a Gaussian distribution

Fig. 9.4
Representative examples of non-Gaussian heart rate fluctuations in a healthy subject and in a patient with congestive heart failure. Shown are time series of normal-to-normal R–R intervals (top row), standardized time series of coarse-grained heart rate variations {Δ25sec B(t)} (middle row), and standardized probability density functions (PDFs) of Δ25sec B(t) (bottom row). Estimated values of the non-Gaussianity index λ 25sec are shown in each panel in the bottom row. The solid lines represent the PDF approximated by a non-Gaussian model [79] with the parameter λ 25sec. The procedure used for this analysis is as follows: (1) time series of R–R intervals are interpolated and resampled at 4 Hz, yielding interpolated time series {b(t)}; after subtracting the mean from interpolated time series, integrated time series {B(t)} are obtained by integrating {b(t)} over the entire length; (2) the integrated time series {B(t)} are divided into overlapping segments of length 2 s with 50 % overlap, where s is the scale of coarse graining (s = 25 s in Fig. 9.4); in each segment, the local trend is eliminated by a third-order polynomial fit; (3) coarse-grained variation Δ s B(t) is measured as the increment with a time lag s of integrated and detrended time series; (4) {Δ s B(t)} is standardized by its standard deviation to quantify the probability density function; then the non-Gaussianity index λ s is estimated based on the q-th order moment of {Δ s B(t)} as
. In the bottom row, λ s is estimated based on the 0.25th order moment (q = 0.25) to emphasize the center part of PDF
![$$ {\lambda}_s^2(q)=\frac{2}{q\left(q-2\right)}\left[ \ln \left(\frac{\sqrt{\pi }E\left[|{\Delta}_sB(t)|{}^q\right]}{2^{q/2}}\right)-\mathrm{l}\mathrm{n}\Gamma \left(\frac{q+1}{2}\right)\right] $$](https://i0.wp.com/neupsykey.com/wp-content/uploads/2017/08/A332405_1_En_9_Chapter_IEq1.gif?w=960)
In a study using the non-Gaussianity index, Kiyono et al. reported that, in patients with chronic heart failure (CHF), increased non-Gaussianity in the 24-h ambulatory HRV predicted increased mortality risk, while none of the conventional HRV indices, including those reflecting vagal heart rate control, were predictive of death [54]. Moreover, Hayano et al. reported that, following acute myocardial infarction, increased non-Gaussianity index λ 25sec at a scale of 25 s was associated with increased cardiac mortality risk, with predictive power independent of clinical risk factors and other HRV predictors [55].
The aspects characterized by λ 25sec are related to the amplitude modulation properties of the LF component shown in Fig. 9.1b. The detrending procedure in the multiscale PDF analysis acts as a high-pass filter that removes low-frequency components below a given time scale, and the calculation of partial sums (or increments of integrated series) acts as a low-pass filter. In the calculation of λ 25sec, the combination of these procedures acts as a band-pass filter that passes frequency components mainly in the LF band. Increased λ 25sec indicates an increase in the heterogeneity of the amplitude of the LF oscillation as seen in Figs. 9.3a, and 9.4, middle right. Note that the λ 25sec can change independently of the value of LF power, the latter quantifying the variance of the LF component. Thus, the λ 25sec can provide information complementary to that provided by conventional frequency-domain indices.
The relationship between the autonomic nervous system and λ 25sec can be understood in light of the following facts: (1) heart rate fluctuations at the scales corresponding to the HF and LF bands are mediated almost exclusively by neural autonomic mechanisms [35, 82]; (2) the λ 25sec showed no substantial correlation with HRV indices reflecting vagal heart rate regulation [54, 55]; and (3) the λ 25sec was decreased in post-AMI patients taking β-blockers [55]. Based on these facts, Hayano et al. suggested that λ 25sec at least partly captures heart rate fluctuations mediated by intermittent activations of cardiac sympathetic input [55].
In addition, Kiyono et al. reported that, in a study of ambulatory HRV that included patients with multiple system atrophy (MSA) and patients with Parkinson disease (PD), the marked increase in λ 25sec observed in patients with CHF was not observed in the patients with MSA or PD who had sympathetic dysfunction [56]. Both MSA and PD are progressive neurodegenerative disorders, although the autonomic lesion in MSA is preganglionic sympathetic failure [83], and that of PD is ganglionic and postganglionic [84, 85]. As shown in Fig. 9.5a, compared with the age-matched healthy control group, the mean values of λ s in patients with CHF display marked increases at the relatively small scales covering the LF band. On the other hand, as shown in Fig. 9.5b, c, such marked increases were not observed in patients with MSA or PD. This result also supports the view that an increase of λ s at scales corresponding to the LF band (s ≤ 25 s) could be a hallmark of cardiac sympathetic overdrive.


Fig. 9.5
Scale dependence of the non-Gaussianity index λ s . (a) Results for patients with congestive heart failure (CHF), both for survivors (n = 69) and nonsurvivors (n = 39) within a follow-up period of 33 ± 17 months. (b) Results for patients with multiple system atrophy (MSA). (c) Results for patients with Parkinson disease (PD). Age-matched controls were selected from a database of healthy subjects. Error bars indicate the 95 % confidence intervals of the group means. The gray, shaded range corresponds to the low-frequency band (s ≤ 25 s). Marked increases in λ s in the gray shaded scales are observed only in patients with CHF, particularly nonsurvivors (a)
Although the above findings suggest an association of cardiac sympathetic activity with the non-Gaussianity of the HRV, most studies evaluating HRV using the non-Gaussianity index have been limited by relatively small cohorts. Therefore, to confirm the clinical and prognostic significance of the non-Gaussianity of HRV, additional prospective studies with larger cohorts will be required.
9.4 Conclusion
The majority of studies evaluating HRV in cardiac patients have shown that reduced and/or abnormal HRV is associated with an increased risk of mortality. In these studies, most HRV indices that predict mortality risk are considered to primarily reflect reduced or impaired vagal function. In contrast, few HRV indices are related to sympathetic function. In this chapter, we try to give a better understanding of the association between sympathetic nervous system activity and such HRV indices. Among HRV indices that have been suggested as markers of sympathetic activity, increased non-Gaussianity of HRV may reflect the deleterious effects of elevated cardiac sympathetic activity in cardiac patients. In addition, some other nonlinear indices not mentioned in this chapter, such as approximate and sample entropies [85, 86], have been suggested to be associated with sympathetic activation. However, to explore the physiological and clinical significance of such indices, a more extensive program of validation will be necessary in the future.
References
1.
Esler M, Kaye D. Sympathetic nervous system activation in essential hypertension, cardiac failure and psychosomatic heart disease. J Cardiovasc Pharmacol. 2000;35:S1–7.CrossRefPubMed
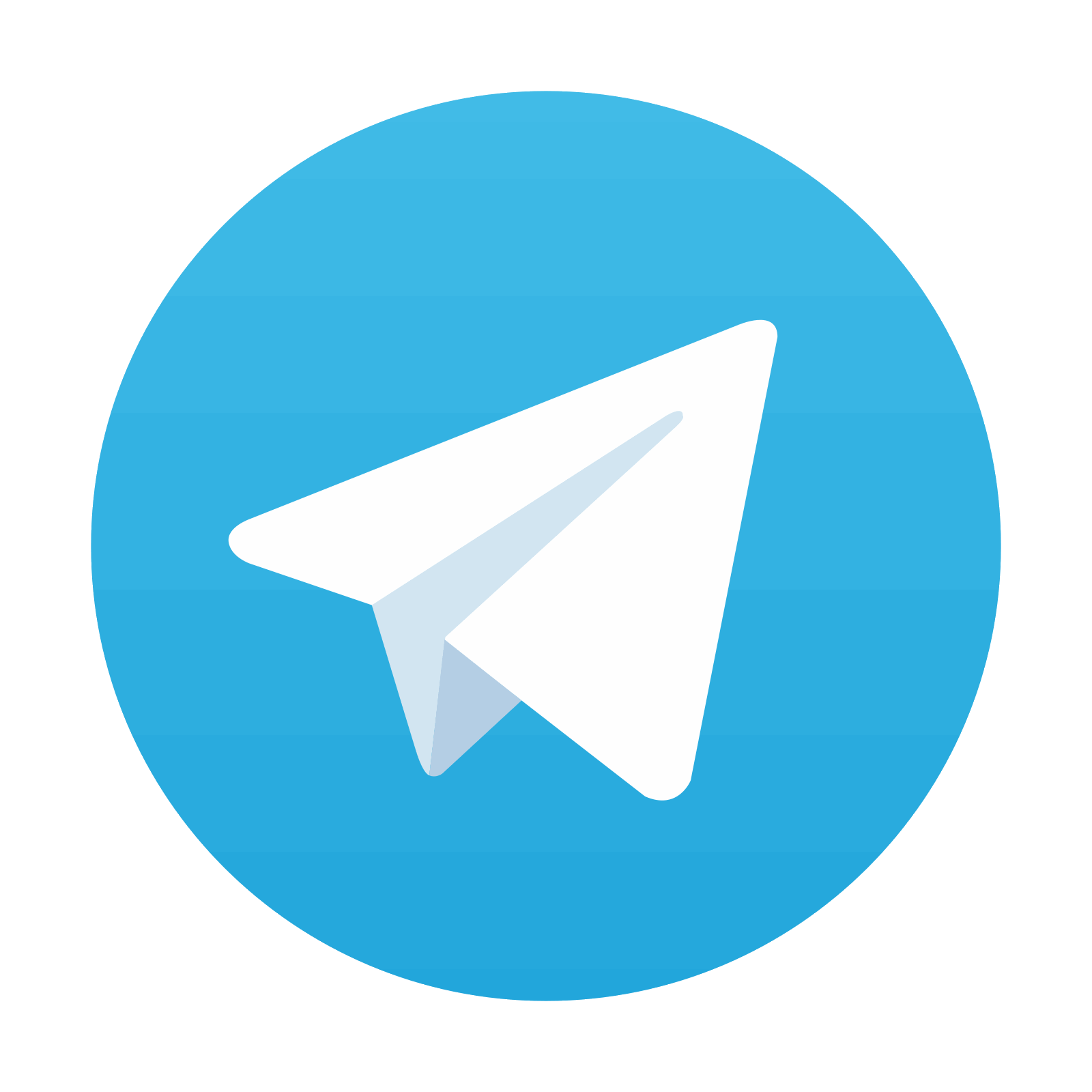
Stay updated, free articles. Join our Telegram channel

Full access? Get Clinical Tree
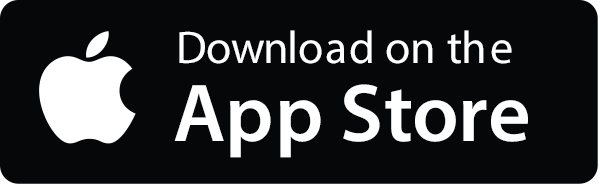
