Fig. 22.1
Schematic representation of cardiovascular circulation . Red, oxygen-rich blood; blue, desaturated blood
Each heart cycle consists of three phases . During the first phase, the atria contract and empty their contents into the ventricles. After a short delay, the second phase is initiated during which the ventricles contract and drive their blood content into the pulmonary artery and aorta, respectively. During the third phase, the myocardium recovers from contraction. After this period a new heart cycle can start.
Electrical Innervation of the Heart
The mechanical contraction of individual heart cells is initiated by action potentials (APs), electrical impulses that travel across a physiologically determined pathway across the cardiac muscle. In a healthy heart, the trigger for each cardiac cycle is in the upper right area of the right atrium, the sinoatrial (SA) node (Fig. 22.2). This area contains cells that spontaneously can generate APs. The frequency at which action potentials are generated by this natural pacemaker has a natural baseline value, but this frequency usually is controlled by the autonomic nervous system. From the SA node, APs travel across a pre-programmed pathway to adjacent cardiac cells; first, the atrial myocardial cells are activated in such a way that they contract from the top to the atrioventricular areas. Contrary to the mechanical barrier that prevents blood to flow from right to left, APs travel from right to left atria and ventricles. However, there is an electrical barrier between both atria and the ventricles: APs cannot cross the atrioventricular junction, except in one area called the atrioventricular (AV) node . This structure is located in the muscle wall between the two atria and consists of specialized tissue that introduces a delay in the conduction of APs. Once passed through the atrioventricular barrier, APs are conducted via the bundle of His and the bundle branches to the bottom of the right and left ventricles, respectively. Through small branches called Purkinje fibers, the ventricular myocardium is innervated, basically from bottom to the atrioventricular areas. After the electrophysiological innervation of cardiac muscle cells, there is always a resting or refractory period which needs to be finished in other to initiate a new AP.


Fig. 22.2
Neural innervation pathways across the heart (yellow)
Spreading of Electrophysiological Activity in the Surrounding Tissue
Action potentials not only act as a trigger for the mechanical contraction of cardiac muscle cells but also cause electrical currents that spread across all conductive tissues surrounding the heart. Most pericardial tissue can be considered as a bioelectrical universe that instantaneously conducts the local currents generated in and around individual cardiac cells toward the natural electrical conductive barrier that surround every subject: skin that is surrounded by air. Because air cannot conduct electrical current, the currents that are generated in the myocardium also cause currents that flow across the skin. Because skin has a finite electrical resistance, Ohms law applies, and voltage differences are generated across different locations at the skin. Because of the highly collective and synchronized manner, many cardiac cells are activated and deactivated during each heart cycle; all the small contributions of individual cells add up and generate a measurable signal at the skin surface, in particular at skin positions close to the heart. This signal, the electrocardiogram (ECG), varies over time because the activation of the various heart regions varies over time and consequently the distance between active and “silent” cardiac regions is time-varying. The basic rule of thumb is that the closer an active region is to any skin position, the larger its contribution in the net electrical voltage that can be measured at such a location. This typically is done using adhesive sensors called electrodes.
ECG: Morphology and Other Characteristics
Figure 22.3 shows an example of a 10-s episode of an ECG recording obtained at nine different skin positions . A detailed description is beyond the scope of this text, but one can easily see that the morphology of the various recordings differs significantly. Nevertheless there are a number of common characteristics: each recording shows a characteristic prominent sequence of sharp peaks and valleys, some recordings having the opposite polarity when compared to others. This is the QRS complex, which is associated with the electrical activation of the ventricles. Although the amplitude (peak-to-peak value) may differ remarkably, its time of occurrence for each recording is exactly the same. This is a result of the laws of physics.


Fig. 22.3
Example of a 10 s ECG recording from different electrode positions
A second characteristic that regularly appears in ECG recordings and is of relevance for HRV analysis is illustrated by the large, slow fluctuations that occur in all recordings around 5 s from the beginning of this trace and in the lower three recordings at the end of this epoch depicted in Fig. 22.3. This is an example of the many types of artifacts that may be encountered during ECG recordings: such large fluctuations are usually a consequence of movement, poor electrode contact, or even loose electrodes. Other manifestations of artifacts may be presence of irregular waveforms (usually referred to as noise), flat recordings, or extremely high amplitudes.
Because different skin areas have different relative positions to the bioelectric heart activity , the morphology of an ECG strongly depends on the position of an electrode. This becomes apparent from illustrated in Fig. 22.3. Two standard electrode configurations, or montages, are commonly used in clinical practice. The oldest electrode configuration was defined by the first pioneer in ECG recordings, Willem Einthoven, who in the early 1900s used four basins filled with saline in which the two lower arms and legs where immersed. The basins acted as electrodes and were connected to a galvanometer that recorded the voltage differences between the four “leads.” Today, adhesive electrodes are used that directly are wired to modern polygraphs, but the leads still are referred to as RA, LA, RL, and LL for right and left arm/leg, respectively (sometimes the term “foot” is used instead of “leg”). Any electrophysiological recording requires a reference voltage; often the right leg electrode is used for this purpose, but many alternatives can be found in literature. For standard extensive cardiological assessments , the wrists and ankles still are used, but for clinical studies the positions of the four Einthoven leads often are chosen at more convenient positions such as just below the left/right claviculae and at left and right upper legs or hips. In addition to the voltage difference between the “active” leads and the reference (RL) electrode, one also can determine the difference between two active leads, e.g., LA-RA, LL-RA, and LL-LA. In compliance with Einthoven’s naming conventions, these three signals are coded with roman numerals: I, II, and III, respectively.
The second type of electrode montage uses six electrodes which are placed at the so-called precordial positions, skin locations close to the heart at well-defined positions at the chest. The positioning is not only chosen such that they can be used for any gender but also allow observation of the electrical heart activity from different directions. The naming convention for these electrode positions is V1…V6. Figure 22.3 also shows example of the signals acquired from these electrodes.
In most clinical and health HRV assessments , a subset of the ten standard electrode positions is used; the precordial positions usually are preferred because these ECG recordings have the most prominent QRS complex and consequently allow the most robust R-peak detection. When a minimal number of electrodes is required, often lead LA-RA is used (positions at left and right front shoulders, just below the claviculae) or V1–V6, the latter having the largest amplitudes [22] but are not always practical considering client-friendliness.
R-Peak Detection for HRV
In cardiology, ECG recordings are indispensable for the initial diagnostic of a host of cardiac diseases. HRV, however, can only be done reliably and in a sensible manner when no clear deficits of heart rate control by the autonomic nervous system and AP conduction problems across the myocardium exist. Under these conditions, each QRS complex can always be associated with one single “trigger” in the SA node. Therefore, the first step in ECG-based HRV analysis is the detection of each individual and consecutive QRS complex and careful determination of its time of occurrence and detection of the next QRS complex.
From a signal analysis perspective, QRS detection is a pattern recognition task. Trained human experts are known to be extremely skilled in such a task, but automation using computer algorithms in practice turns out to be a very difficult task, in particular when dealing with long-duration and/or ambulatory conditions. A host of proposals have been made, but to our experience no algorithm has shown to be as good or even superior to human pattern recognition. A useful and recommended strategy is to preprocess the data by a modern QRS detection algorithm, such as the one based on filter banks proposed by Afonso et al. [1], and subsequently inspect, and if necessary correct, the algorithmically determined fiducial points.
Measuring Heart Rate Variability
Given accurate determination of the fiducial QRS complex points, the R-R (inter-beat) intervals can be calculated. These intervals are also referred to as normal-to-normal (NN) intervals or heart periods . Actually, a more correct term for what is usually referred to as heart rate variability would be heart period variability, because usually most calculations are performed on heart periods not rates.1 The heart periods can be plotted against time in the cardiotachogram (Fig. 22.4b). The calculations on heart periods can be classified into four categories: time domain measures, frequency domain measures, time-frequency domain methods, and nonlinear measures. Software for many of these measures is freely available in several packages such as Kubios (http://kubios.uku.fi/), Physionet (http://www.physionet.org/), and RHRV (http://cran.r-project.org/web/packages/RHRV/).


Fig. 22.4
Example of a raw ECG with fiducial R-peaks (a); the associated cardiotachogram (b); FFT-based (c) and autoregressive-based (d) spectra showing the VLF (pink), LF (blue), and HF (yellow) bands; Poincaré plot (e); and detrended fluctuation analysis (f)
Time Domain Methods
The most common time domain methods of HRV are listed in Table 22.1, along with normative values and some values that have been found in cases of PTSD. Mean NN intervals in seconds or milliseconds, or mean heart rate in beats per minute, are obvious global indicators of heart functioning that can be analyzed in various situations like rest, sleep, task performance, etc. The most straightforward measure of overall HRV is the standard deviation of NN intervals (SDNN). To obtain stable measures, a period of approximately 5 min is recommended, and the duration must be kept constant in case comparisons under different conditions are made, because the standard deviation depends on the length of the recording period. In case of 24-h recordings, the total duration is often divided into 5-min segments, and the standard deviation of the segment averages is reported (SDANN). This value reflects very slow periodicities, i.e., longer than 5 min. The most commonly used time domain measures for short (beat-to-beat) variations are the Root Mean Square of Successive Differences (RMSSD), calculated as the square root of the mean squared differences of successive NN intervals; NN50, the number of NN intervals greater than 50 ms; and pNN50, the proportion of number of intervals greater than 50 ms. Obviously, because the latter measures all reflect relatively fast fluctuations, they are highly correlated.
Table 22.1
Normative values for commonly used variables of HRV
Name | Description | Unit | Normative values |
---|---|---|---|
Mean R-R | Mean of R-R intervals | ms | 926 ± 90a |
SD R-R (SDNN) | Standard deviation of R-R intervals | ms | 50 ± 16a |
RMSSD | Root mean square of successive differences | ms | 42 ± 15a |
HRV triangular index | Triangular index of HRV histogram | 37 ± 15b | |
LF | Power in the low-frequency band (0.04–0.15 Hz) | log10(msb) | 5.01 ± 1.76a |
LFnu | LF power in normalized units (divided by total power – VLF power) | n.u. | 54 ± 4b |
HF | Power in the high-frequency band (0.15–0.40 Hz) | log10(ms2) | 4.76 ± 1.78a |
HFnu | HF power in normalized units (divided by total power – VLF power) | n.u. | 29 ± 3b |
LF/HF | Ratio between LF and HF power | 2.8 ± 2.6a; 1.5–2.0b |
A histogram of frequency distribution of all NN intervals forms the basis of geometrical time domain measures. If the frequency distribution is narrow and high, then all NN intervals lie within a small range of values, and HRV is low. If, on the other hand, the distribution is low and broad, then variability is high. Based on this notion, the HRV triangular index is the ratio between the integral of the NN density distribution (i.e., the total number of NN intervals) and its height. Because the resulting value depends on the bin width of the frequency histogram, this bin width has been standardized to 1/128 = 7.8125 ms). The triangular interpolation of the NN interval histogram (TINN) reflects the baseline width of the NN interval histogram in milliseconds. The HRV triangular index and TINN correlate with SDNN, as they reflect overall variability. Note that a sufficient number of NN intervals should be available for reliable calculation of the geometric measures of HRV. Therefore, these measures are usually only employed for 24-h recordings.
Frequency Domain Measures
The power spectral density function (PSD) relates variance (power) to specific frequencies in the heart period series. It can be calculated nonparametrically (usually by the fast Fourier transform; FFT), or parametrically by autoregressive (AR) methods. The nonparametric FFT-based approach is fast and straightforward, but may result in “spiky” spectra for relatively short recordings (although this can be alleviated somewhat by using Welch’s periodogram). In addition, the results may depend on choices made with respect to tapering of the raw series to avoid spectral leakage (a 10% cosine window is often used) and to the smoothing of the periodogram. AR spectra are smoother and more suitable for short recordings, but it is not straightforward to determine the optimal AR model order. Irrespective of the method used to calculate the PSD, its calculation assumes an equidistantly spaced time base, as opposed to an NN interval base. Therefore, the NN intervals should be interpolated to a real-time axis. Usually, cubic splines are used for interpolating values, and a sampling frequency of 4 Hz is recommended for the time base.
Overall power as calculated by the PSD corresponds to overall variation in the time domain as calculated by SDNN. If short (5-min) recordings are used, three sources of variance can be distinguished: very low frequency (VLF; <0.04 Hz; period >25 s), low frequency (LF; 0.04–0.15 Hz, period ~7–25 s), and high frequency (HF; 0.15–0.4 Hz; period 2.5 – ~7 s). If 24-h recordings are analyzed, the VLF band is usually further subdivided into an ultralow frequency band (ULF; <0.003 Hz, period >333 s ~5 min) and a VLF band (VLF; 0.003–0.04 Hz, period 25 s – 5 min). An example of the PSD in which these frequency bands were marked is presented in Fig. 22.4c (FFT method) and Fig. 22.4d (AR method); normal values are given in Table 22.1. Power in the HF band correlates with the RMSSD, NN50, and pNN50 in the time domain, because these variables all reflect relatively fast fluctuations in the NN series. There is no clear correlate of the LF and VLF bands in the time domain, but the ULF band in 24-h recordings is related to SDANN.~
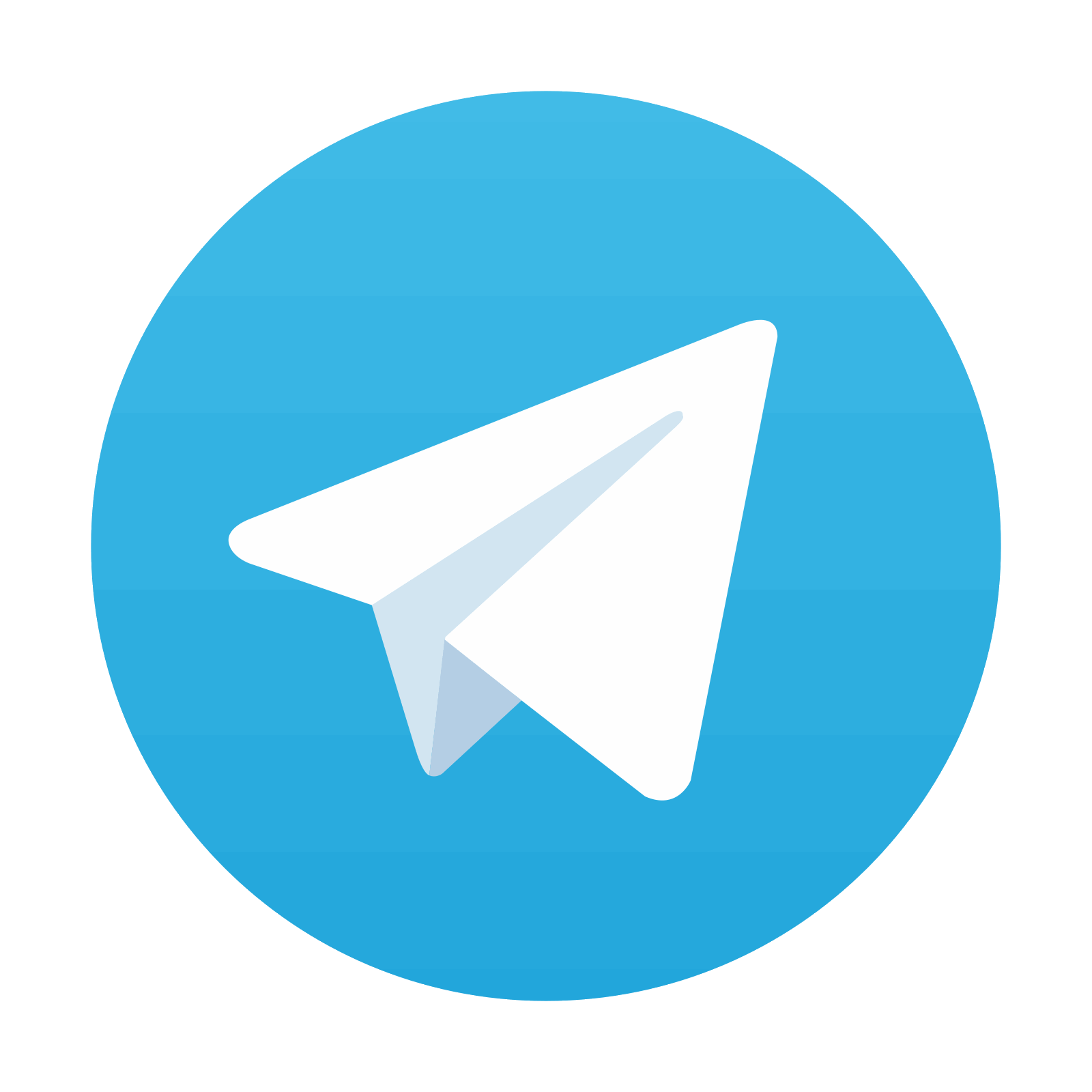
Stay updated, free articles. Join our Telegram channel

Full access? Get Clinical Tree
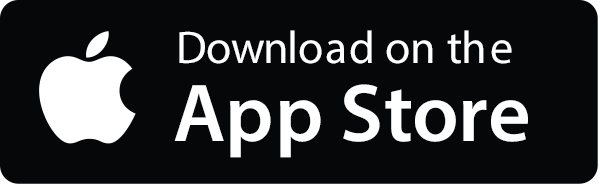
