(2.1)
where S is the DW signal, S0 is the signal without any DW gradients (but otherwise identical imaging parameters), D is the apparent diffusion coefficient, and b is so-called “b-factor”. MRI signals are proportional to the sum of magnetization components from all water molecules in a voxel, which is closely associated with the area of the diffusion gradient pulses defined by the amplitude of the magnetic field gradient pulses, G, and the temporal duration, δ, and the temporal spacing between the pulses, Δ. The effects of all these parameters are indeed ascribed to a coarse term “b-factor” as below:
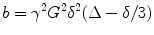
(2.2)
By applying the appropriate magnetic field gradients, MR imaging may be sensitized to the random, thermally driven motion (diffusion) of water molecules in the direction of the field gradient. Diffusion is anisotropic (directionally dependent) in WM fiber tracts, as axonal membranes and myelin sheaths present barriers to the motion of water molecules in directions not parallel to their own orientation. The direction of maximum diffusivity has been shown to coincide with the orientation of WM fiber tracts [44].
In short, the fundamental concept behind DTI is that water molecules diffuse differently in the tissues heavily depending on its type, integrity, architecture, and presence of barriers, generating information about its orientation and quantitative anisotropy embodied by the diffusion tensors [9, 14, 44]. DTI may be used to map and characterize the three-dimensional diffusion pattern as a function of spatial location, which can further be taken advantage of estimating the connectivity properties of the whole brain WM networks , using the diffusion anisotropy and the principal diffusion directions [23].
Undoubtedly, DTI holds a unique and unparalleled sensitivity to water movements of painting the blueprint of brain architecture [26, 33, 43, 56], as uses existing MRI technology without the necessity of investing new equipment, contrast agents, or even radiochemical tracers. However, there remain many technical issues with regard to the performance of the tool, practical considerations of working on biological specimens, and the interpretation of DTI tractography images. For instance, within each voxel of diffusion-encoded images, it can only resolve the single (the most dominant) fiber direction and cannot differentiate the kissing/crossing/branching fibers in the complex cerebral regions [1, 71]. Consequently it estimates the fiber orientation to be the mean of the underlying fiber directions, even though the mean direction will not be representative of the true fiber directions [3]. Nevertheless, it is not capable of determining with accuracy the origin and destination of fibers, which requires further comprehensive evidence to corroborate, even in combination with employing other advanced technologies [5, 58].
2.3 Diffusion Spectrum MRI (DSI)
Recent technological development of MRI methods has been devoted to solving the aforementioned issues, so as to better characterize the complicated fiber patterns and discern fiber orientations. As matter of a fact, either model-based or model-free methods have been acknowledged that have the capacity to resolve heterogeneity of fiber orientations in each resolved volume of tissue (voxel) [65, 68], and provide detailed views into the precise organization of cerebral white matter tracts [69]. Here we will focus on the model-free derivations of diffusion MRI technique.
Model-free methods, also called q-space imaging methods [11, 16, 59], measure the microscopic diffusion function directly without any assumptions on the form of the underlying diffusion function, even though they still have to count on the Fourier transform relation between the diffusion MR signals and the underlying diffusion displacement [31]. Firstly the probability density function (PDF) or orientation distribution function (ODF) of the diffusion displacement in the three biophysical dimension are obtained. One can then calculate a common quantitative scalar measure, generalized fractional anisotropy (GFA) in DSI, physiologically equivalent to FA [19], so as to implicate the microstructure property. Worthy to mention, FA, an index derived from the diffusion tensor that reflects the degree of directional coherence, myelination, and diameter of axonal fibers has been widely used to examine the integrity of white matter tracts in DTI [4, 26]. Simply put, higher FA or GFA values imply higher coherence of fiber directions, more myelination or larger axonal diameters in the white matter at the microstructural level.
More frequently the connections of each ODF will be explored to infer the underlying linking patterns of the fibers [22]. Here in after, we briefly discuss two commonly used q-space (diffusion-encoding space) reconstruction methods to estimate the ODF from the acquired diffusion MR signals, with particular emphasis on the DSI method.
Q-ball imaging (QBI): Tuch and his co-workers introduced q-ball imaging (QBI) [64], which usually used the Funk–Radon transform (also known as the spherical Radon transform) to reconstruct ODF at the cost of large pulsed field gradient and time-intensive sampling. It is also feasible to resolve intravoxel fiber crossing through using a high angular resolution diffusion imaging (HARDI) scheme, which samples data on a shell in the diffusion encoding space. The Funk–Radon transform relation forms the core basis of the QBI reconstruction method to avoid any assumptions on the diffusion process like Gaussianity or multi-Gaussianity and to achieve better accuracy and efficiency [17].
Diffusion spectrum imaging (DSI): Wedeen and his colleagues proposed to acquire the diffusion MR signals by using the grid sampling scheme, and then applied the Fourier transform on the q-space data to estimate the underlying diffusion displacement pattern for further calculation of the ODF [15, 21, 31, 61, 65, 68–70, 72]. The sampling scheme of DSI produces a cluster of grid points distributed on a sphere in the q-space. Each grid point corresponds to a specific value of diffusion sensitivity (b-value) and direction, and the b-value increases incrementally from 0 to a maximal b-value (b-max). When all the diffusion-encoding samples in the q-space have been collected, the Fourier transform (FT) is performed to obtain the PDF as the diffusion-encoding samples S(q) and their corresponding PDFs constitute a Fourier pair [10]. In sum, DSI measures the ODF by acquiring hundreds of diffusion-weighted images (DWI) with different diffusion-encoding gradients, each with different strength and direction [5, 21]. In this manner, it is possible to infer the number of fiber-compartments and their relative amplitude as well as spatial orientations by reconstructing the ODF local maxima. It enables DSI to resolve kissing or crossing or bending fiber orientations at single voxel-based level, as shown in Fig. 2.1.
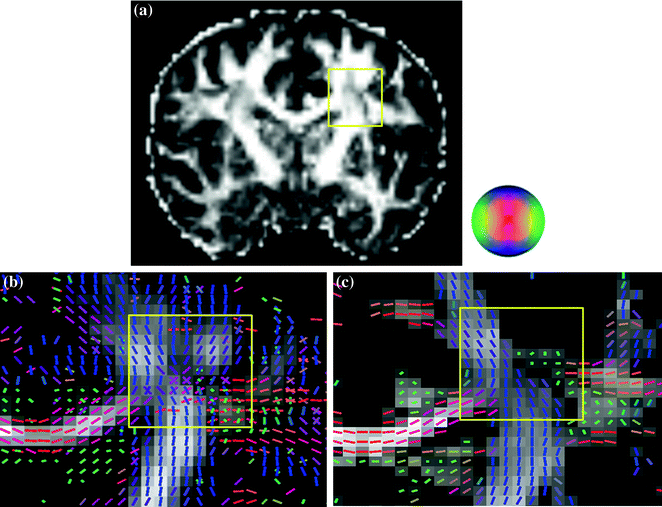
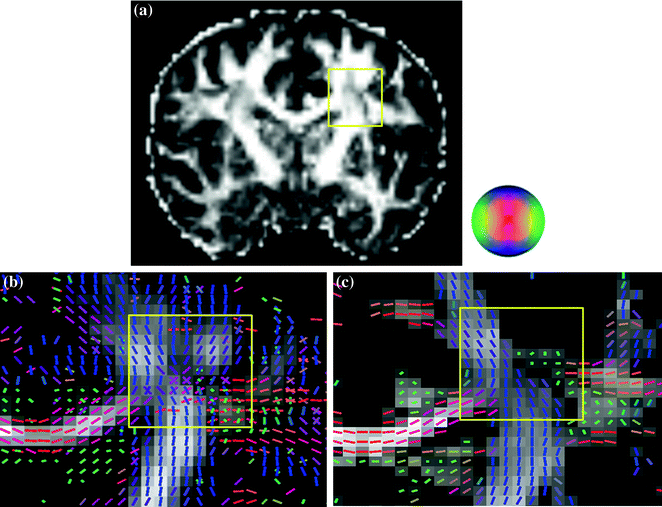
Fig. 2.1
Comparison on the fiber orientations of the centrum semiovale resolved by DTI and DSI on the same subject. b Using DSI, fibers coursing towards the corpus callosum (red) intersect with vertically oriented corona radiata fibers (blue) and rostro-caudally oriented long association fibers (green). In contrast to using DTI (c), only one fiber orientation is seen within one voxel. a DTI-derived FA map. The area within yellow boxes of each image a, b, c is the centrum semiovale. The directions of the ODF or SDF are pseudo-colored: red in the left–right direction, green in the anterior-posterior direction, and blue in the axial superior-inferior direction, illustrated by the color ball. The gray background represents the calculated values of GFA (b) and FA (c), respectively
Bear in mind, though, some precautions should be carefully exercised when implementing these two q-space imaging methods to derive diffusion ODF. One caveat of the QBI method is that the acquired diffusion MR signal is in fact contributed by the diffusion displacements in all directions, not just the displacements perpendicular to the diffusion gradient vector. Consequently the q-ball ODF can only be deemed as good approximation since it doesn’t consider all diffusion displacements in the three-dimensional space [62]. On the other hand, DSI is able to characterize the diffusion probability density function by applying the Fourier transform to the MR signals in the q-space; however, it still relies on numerical estimation to calculate the ODF. The mathematical estimation often encounters the truncation artifacts in the Fourier transform, as entails additional treatment like a Hanning filter smoothing on the PDF [22, 31].
Generalized-sampling imaging (GQI): Recently the spin distribution function (SDF) has been defined to quantitatively describe a distribution of the spins undergoing diffusion on the voxel basis , which is unlike the diffusion ODF representing a probability distribution of the diffusion displacement [72]. This relation leads to an imaging method named generalized q-sampling imaging (GQI) which is readily applicable to a wide range of q-space datasets including those acquired by the shell or grid sampling schemes. Taking a closer look at the reconstruction equations of GQI and DSI, they share the same theoretical basis. Hence GQI and DSI reconstruction could result in similar diffusion patterns. Note that the reconstruction process of GQI does not require deconvolution procedures and the SDF values can be compared across voxels [72].
2.4 Diffusion MR Tractography
Tractography hasbeen developed to improve the depiction of data from diffusion imaging of the brain and aid the image interpretation [8, 67]. The primary purpose of tractography is to clarify the orientational architecture of nerve fibers by integrating pathways of maximum diffusion coherence. The computational algorithms normally track the diffusion maximum from voxel to voxel in such a way of simulating the fibers growing across the brain. The fibers offered by tractography are often accepted to represent individual axons or nerve fibers, but they are more accurately viewed as lines of dominant diffusion that follow or parallel the local diffusion maxima [43]. This distinction is vital because, given with certain imaging resolution and signal-to-noise level, lines of maximum diffusion coherence may differ from the axonal architecture in some brains [42]. For example, DTI provides a Gaussian approximation of the actual displacement distribution, and since the representation of that distribution is restricted to variations of an ellipsoid, this method creates various biases in the resulting tractography [27]. Also the tractography results depend on the tracking algorithm used. Deterministic fiber tracking from DTI uses the principal direction of diffusion to integrate trajectories over the image but ignores the fact that fiber orientation is often undetermined in the diffusion tensor imaging data [42].
The fact is that the connectivity maps obtained with tractography vary according to the diffusion imaging modality used to obtain the diffusion datasets. Hagmann and colleagues investigated statistical fiber tracking methods based on consideration of the tensor as a probability distribution of fiber orientation [23]. The application of fiber tractography to data such as those obtained with DSI or QBI results in the depiction of a large set of fiber tracts with a more complex geometry. Such greater complexity obtained with this method is due to the consideration of numerous intersections between fibers that can be resolved or differentiated. In such sense, DSI tractography overcomes some above biases and allows more realistic mapping of connectivity [21, 31, 69, 70]. Figure 2.2 presents typical examples of six major white matter tracts reconstructed by DSI.
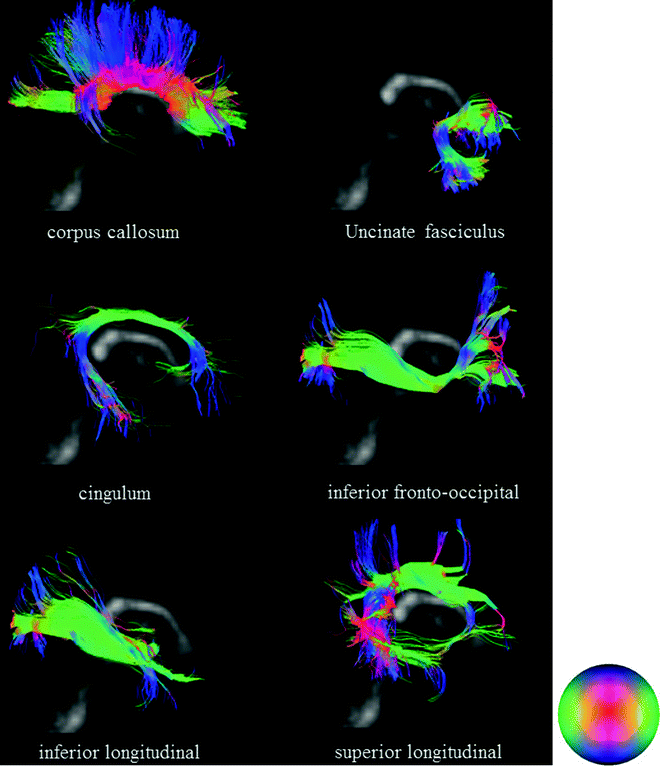
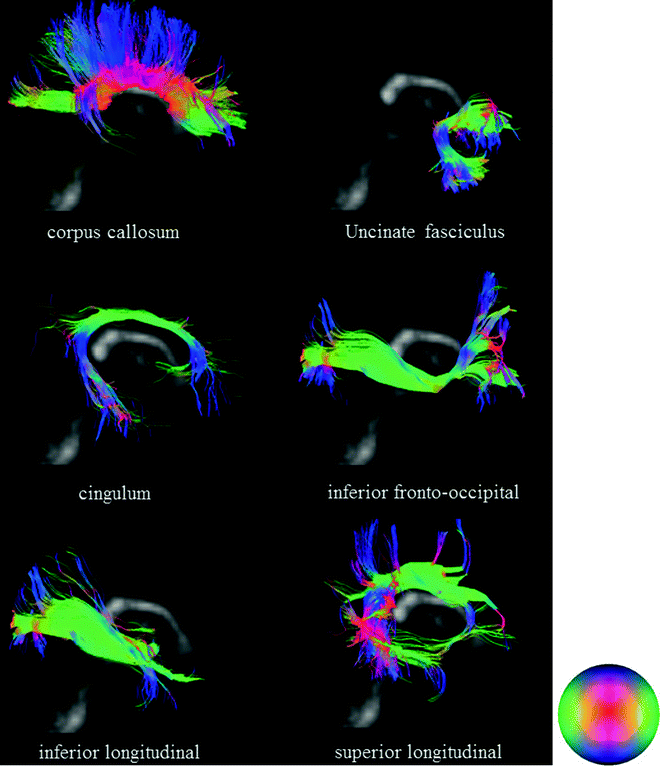
Fig. 2.2
Six classic white matter tracts obtained with DSI tractography (overlay on sagittal views of GFA maps)
Nonetheless, tractography provides more interesting and valuable information for depicting the human neuroanatomy in vivo.
2.5 Procedure of Data Analysis
Here we used the DSI image dataset collected by Functional Brain Imaging Platform (FBIP) at Institute of Neuroscience, Chinese Academy of Sciences, Shanghai, to demonstrate the procedure widely-used in the analysis of the diffusion MRI data. It will lend the support to those who are interested in applying these neuroimaging techniques to solve those clinically-driven problems during diagnostics and therapy of psychiatric disorders . A schematic workflow diagram of diffusion MRI processing is illustrated in Fig. 2.3.
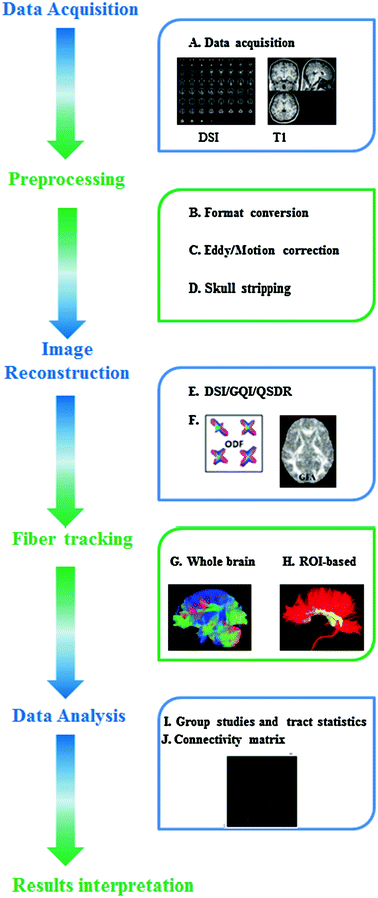
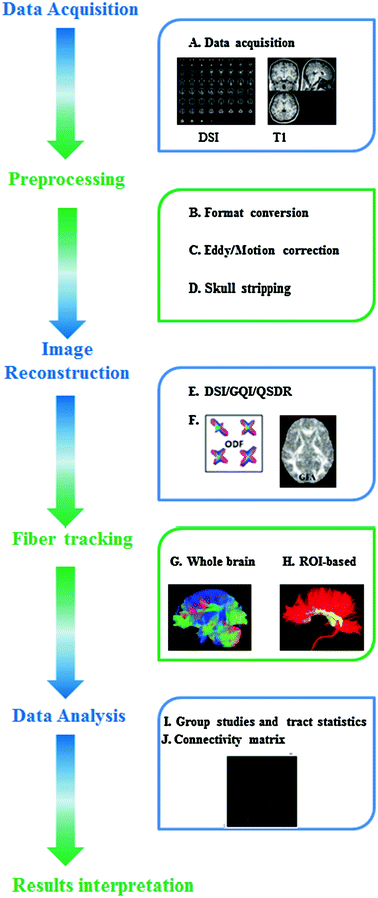
Fig. 2.3
A schematic diagram of typical DSI data-processing workflow. Step 1: Data acquisition (A). Step 2: Preprocessing includes the conversion of original dataformat (B), eddy and motion correction (C), and skull stripping (D). Step 3: Image reconstruction. Some commonly-used reconstruction approaches are listed here like DSI, GQI or QSDR (E). The ODFs or GFA are calculated for further analysis (F). Step 4: Fiber tracking. Either the whole-brain (G) or ROI-based fiber tracking (H) can be chosen for distinct research objectives. Step 5: Data analysis. Statistical comparison of fiber tracts can be conducted at group-level (I and J) and the network-level analysis can be used to extract more features from the structural connectivity matrix
The detailed imaging protocol will be described in other places and summarized shortly here. T1-weighted structural MRI images were acquired on a 3T MR imaging system (Magnetom Trio; Siemens, Erlangen, Germany) using a 12-channel phased array head coil (TR = 2,300 ms, TE = 3 ms, TI = 1,000 ms, flip angle = 9°, FOV = 256 × 256 mm2, voxel size = 1.0 × 1.0 × 1.0 mm3, 176 slices, no slice gap). Earplugs were used, and movement was minimized by stabilizing the head with cushions. Diffusion spectrum imaging was acquired using a twice-refocused spin-echo EPI pulse sequence [55]. The diffusion-encoding scheme used in this study followed the framework of DSI in which diffusion-weighted images were acquired with diffusion gradients of different b values corresponding to the grid points filled within a sphere in the 3D diffusion-encoding space (q-space) [68]. TR = 9,500 ms, TE = 152 ms, flip angle = 90°, FOV = 80 × 80 mm2, voxel size = 2.4 × 2.4 × 2.4 mm3, b-max = 7,000 s/mm−2. To reduce the scan time, we only recorded half-sphere DSI data [20, 21]. Specifically, the DSI data were acquired with 128 diffusion-encoding directions corresponding to grid points filled in the half sphere of the q-space. Acquisition time for the half-scheme acquisition was 21 min. To correct for image distortion resulting from magnetic susceptibility, field maps were acquired using a GRE sequence with two TEs (acquisition time, 91 s, TR/TE = 500/3.38 and 5.84 ms). The matrix size and FOV of the field maps were the same as those used in the DSI dataset.
The half-sphere data were extrapolated to the other half of the sphere based on the symmetry property of the data in the q-space, and the eight corners of the cube that were unsampled were zero-filled. The susceptibility-induced distortion on each DSI image was first corrected using the acquired field maps [24], and then subjected to the motion correction and eddy current compensation using the software based on FSL (http://www.fmrib.ox.ac.uk/fsl). The following DSI data reconstruction was conducted through using the generalized q-sampling imaging approach available in the software of DSI Studio (http://dsi-studio.labsolver.org) [15]. The ODFs were reconstructed to 162 discrete sampling directions (corresponding to the vertices of a 4-fold regularly tessellated icosahedron projected onto the sphere). The diffusion deconvolution was then applied to the diffusion ODFs generated from GQI to increase the angular resolution of the resolved fiber. Having obtained the ODF, the GFA (similar to FA) was derived to quantify the directionality of the diffusion on a scale from zero (when the diffusion was totally random) to one (when the diffusion was along one direction only). The formula of deriving the value of GFA is defined as the ratio of the standard deviation of ODF and its root mean square [64]. Like FA, GFA has been used to infer microstructure integrity of the white matter fiber tracts.
In addition to subject-specific reconstruction approach, we also reconstructed the DSI data in MNI-152 space (a standard space introduced by the International Consortium for Brain Mapping, http://www.bic.mni.mcgill.ca/ServicesAtlases/ICBM152NLin2009) using q-space diffeomorphic reconstruction (QSDR) [15], which provides a direct approach to analyze the group difference and also facilitates the comparison using fiber tracking. QSDR is the generalization of GQI that allows users to construct ODFs in any given template space (e.g. MNI space). DSI Studio first calculates the quantitative anisotropy (QA) mapping and then normalizes it to the NTU-90 QAmap. The NTU-90 atlas is the average of the normalized subject data in the MNI-152 space.
For the fiber-tracking datasets, all fiber tracking was performed using the DSI Studio. In most of clinical studies, an ODF-streamlined region of interest (ROI)-based approach are frequently chosen to discern the difference between the patient and control groups [15]. Depending on various kinds of research objectives, there are several ways available to define the ROIs of the subjects. In QSDR reconstruction approach, we used the normalized ROIs from the JHU White Matter tractography atlas provided by DSI studio [67]; In GQI reconstruction approach, we used the selected ROIs from the JHU atlas and warped it to the subject T1-weighted image space by linear registration. Tracts were generated using an ODF-streamline version of the FACT deterministic tractography algorithm [8, 15], and left and right hemispheres were investigated separately. If an ODF had more than one peak orientation, the initial direction was the primary fiber from the resolved orientations. The advantage of “primary” is the stableness and consistency of the results. Trilinear interpolation was used to estimate the propagation direction. Fiber progression continued with a certain step size like 1 mm (half the spacing for QSDR reconstruction approach), minimum fiber length like 40 mm, and turning angle threshold (usually set at 40°–70°). To smooth each track, the next moving directional estimate of each voxel was weighted by a combination of the previous incoming direction and the nearest fiber orientation. Once tracked, all streamlines were saved in the TrackVis file format. Segmentation of the fiber tracts was performed with TrackVis software (http://trackvis.org).
2.6 Diffusion MRI Applications in Psychiatric Disorders
Diffusion MRI has been becoming an indispensable tool to investigate a variety of psychiatric disorders such as schizophrenia, major depressive disorder , eating disorders, attention deficit disorder and addictions and so on. It has shed insightful light on our understanding of neural connectivity and how abnormalities in connectivity may contribute to the pathogenesis of psychiatric illnesses. This section will concisely summarize recent applications on those psychiatric diseases with DTI/DSI techniques, specifically restricting our attention to the reward-circuitry based brain disorders.
Obsessive-Compulsive Disorder (OCD): Alterations in the WM tracts within the classic cortico-striato-thalamo-cortical (CSTC) circuitry, which has long been implicated in the pathogenesis of OCD [39], were always observed in the literature with DTI method. There have been reported that alterations of FA were observed in many brain regions of OCD patients, such as the anterior cingulate [12, 60], internal capsule [12, 73], white matter in the area superolateral to the right caudate [73], corpus callosum [57, 73], and the right inferior parietal and medial frontal regions [36], the bilateral semiovalcenter extending to the subinsular white matter [45]. However, the conclusions drawn from these current studies have been inconsistent. For example, findings in the FA of the anterior cingulate were particularly mysterious, showing higher values [12], lower values [60], or no changes [36, 45, 73] in OCD patients in comparison to normal subjects.
Findings concerning the anterior thalamic radiations (ATR) seem to be inconsistent as well. Cannistraro et al. [12] reported an increased FA value in the left anterior limb of the internalcapsule, whereas Yoo et al. [73] found increases in FA in the superolateral area of the right caudate that were penetrated by the ATR. These divergent observations by the DTI could be caused by the notoriously complicated structure of the brain network , in which the presence of crossing fibers has been robustly demonstrated by the DSI-based tractography in both nonhuman primate and human [21, 58, 69]. Therefore, Chiu and his co-workers applied the GFA value to describe the microstructural integrity of the white matter tracts [15]. They found significantly lower mean GFA in the right ATR and the left the anterior segment of cingulum bundles in OCD subjects compared to the ATRs and cingulum bundles of normal controls. In addition to the GFA measure, asymmetry of the mean GFA between the left and right tracts was also calculated [15], which might provide a parsimonious explanation to the above discrepancy observed in different reports. Notwithstanding the new evidence, it requires further research to fully elucidate those apparent contradictory results. Besides that, more widespread WM abnormalities like the microstructural alterations in the fronto-basal pathways targeting the orbitofrontal cortex and the anterior cingulate cortex have been proposed to be involved in OCD [39]. Menzies and colleagues reported that the anatomical connectivity between lateral frontal and parietal regions was altered as well as microstructural abnormalities in intra-hemispheric bundles linking distinctive areas of the prefrontal cortex to posterior parietal and occipital association cortices [36].
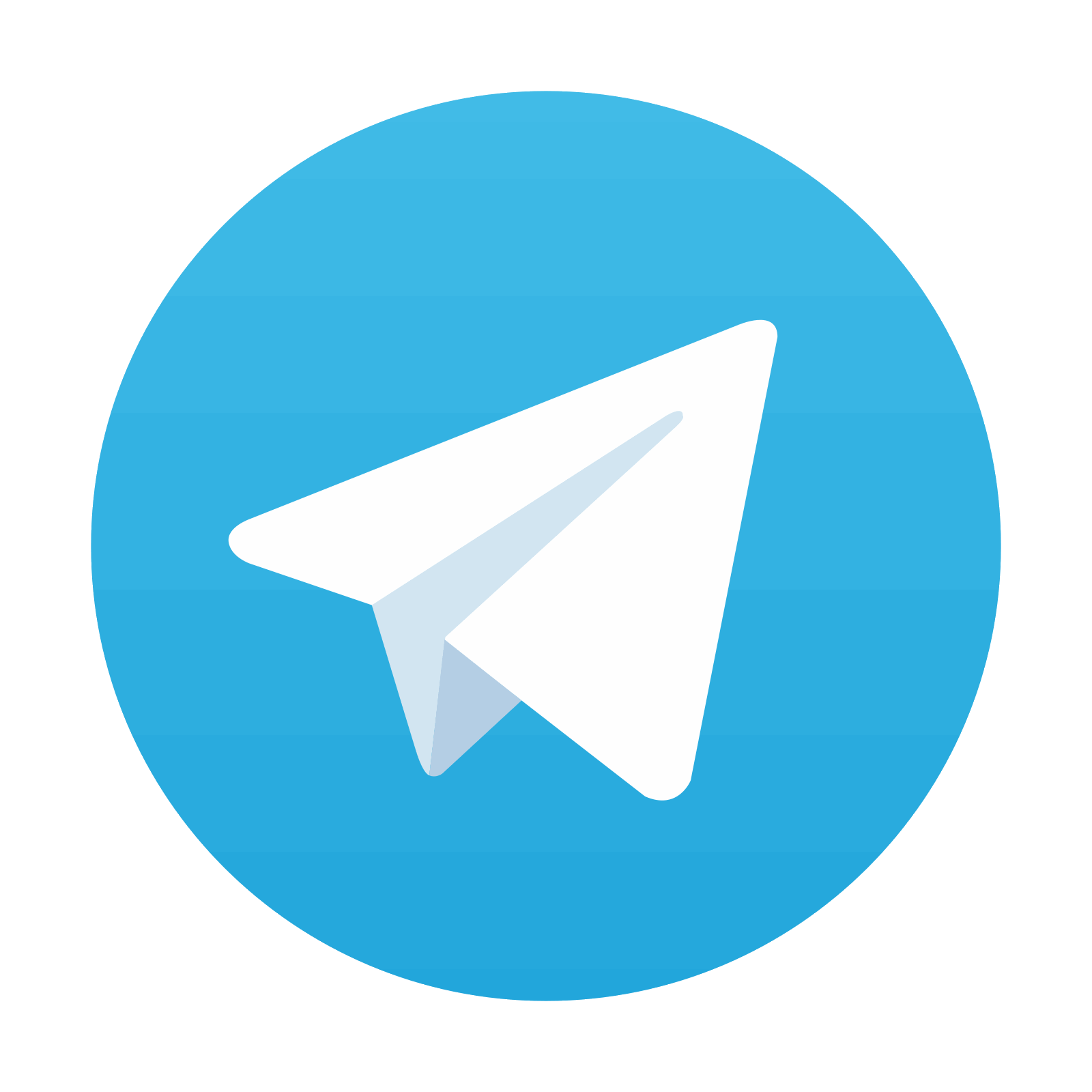
Stay updated, free articles. Join our Telegram channel

Full access? Get Clinical Tree
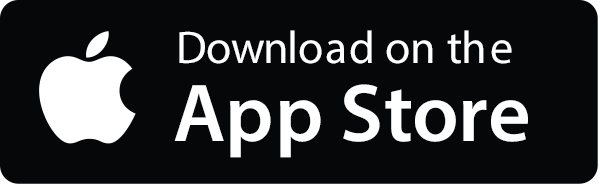
