Until a few years ago, there was little interest in expensive or speculative improvements to any of these four components. The primary market for BCI systems was research groups. Very few patients, or their caregivers, purchased BCIs for home use, mainly because expert assistance was needed to identify, assemble, install, configure, use, and maintain the BCI system. Therefore, the number of BCI systems purchased per year was too small to foster broad adoption, and there was limited funding for academic research.
Due to the rapidly growing research community interested in BCI technology, including contributions to all four BCI components from many different disciplines and regions, BCIs have become increasingly practical for broader user groups. New applications, reduced prices, improved robustness in noisy environments, and improved usability have all made BCIs more appealing. Although no fundamentally new signal acquisition systems have been developed—most BCIs still rely on the electroencephalogram (EEG) recorded from electrodes on the head—active and dry electrodes have made EEG recording much more practical and convenient [9–12]. Active and dry electrodes also entail improved signal processing, with chips on each electrode that amplify the signal when it is recorded and thus reduce noise. Many new BCI applications are emerging or improving, such as BCIs to control orthoses, virtual environments, smart homes, mobile robots, and games such as World of Warcraft [6, 7, 13–16]. These applications are especially important for opening new market opportunities, since they provide new opportunities for end users to use BCIs for the applications they need or want. New and/or improved operating protocols such as intendiX® and public domain software have made BCIs more accessible to users without a technical background [17]. The following section provides an overview of the three major kinds of BCIs: SMR-, P300-, and SSVEP-based BCIs.
3.1.1 Types of Noninvasive BCIs
BCIs are generally categorized according to the mental activities that users must perform and the corresponding neural activity. Brain activity can be assessed by various functional imaging methods like functional magnetic resonance imaging, functional near-infrared spectroscopy, positron emission tomography, and magnetoencephalography or by more invasive methods like electrocorticography or single neural cell recordings and others. Most BCIs rely on the electroencephalogram (EEG). EEG is safe, portable, and relatively convenient and inexpensive, making it the neuroimaging tool of choice for research groups and different consumers.
Many early BCI systems used slow cortical potentials [19], which rely on EEG changes that users can learn to control with months of training. These BCIs are no longer widely used because of the extensive training requirement and because other BCIs are faster, more accurate, and offer a larger vocabulary.
BCI systems based on induced oscillations generally use motor imagery (MI) strategies to generate event-related de-/synchronization (ERD/ERS) in the alpha and beta frequency ranges of the EEG. These changes in sensorimotor rhythms (SMRs) can be detected by a BCI and used for control. SMRs and other components related to imagining common mental tasks like navigation, singing, or arithmetic [2, 3]. BCIs based on SMR changes have been developed to control cursors, navigate through games and virtual environments, and control devices like wheelchairs, mobile robots, and orthoses [3, 6, 14, 20, 21]. These BCIs typically allow control over one or two axes of movement or allow a few specific decisions. However, the highest information transfer rates are reached with only two decisions, beyond which the accuracy falls dramatically.
A P300-based BCI system relies on the fact that an infrequent event that the user silently counts induces a P300 component in the EEG. This P300 is a positive deflection in the EEG signal that develops around 300 ms after the event. Such systems are well suited for direct selection tasks such as spelling or choosing numbers, because they can allow the user to choose from a large number of choices (that is, a large vocabulary) without moving a cursor toward a target or performing other intermediate steps. P300 BCIs have been developed to allow spelling in different languages, including English, Chinese, and Japanese [9–11, 22–25].
Steady-state visually evoked potentials or SSVEPs can allow a wide number of choices, limited by the number of distinct frequency responses that can be analyzed in the EEG. Lights that flicker in the range of around 8–30 Hz induce brain oscillations of the same flickering frequency. These oscillations are larger if people pay attention to one of the stimuli, producing a corresponding increase in brain oscillations that a BCI can detect. Applications have included spelling and control of orthoses and virtual avatars [6, 9, 13, 26, 27]. Both the P300 and SSVEP approaches require an external stimulus to elicit specific patterns of brain activity and are therefore called synchronous BCIs. These BCIs rely on EEG patterns that the brain produces without specific training, and most people can use them within a few minutes. In contrast, BCIs based on SMRs often require training times of several minutes to weeks, and SCP BCIs take even longer. SMR and SCP BCIs do not need an external stimulation source and can be controlled asynchronously [3, 19, 28, 29], although these systems typically rely on visual stimuli to present instructions, stimuli, and feedback.
New BCI user groups could change the way that these different BCI approaches are used and encourage studies with new mental tasks and neural signals. BCI technology is gaining attention for new directions such as stroke rehabilitation, functional brain mapping, coma assessment, and other applications that could greatly increase the variety in the stakeholders interested in BCIs ([2, 15, 29–33]. The confluence of Information and Communication Technology (ICT) techniques (brain/neuronal–computer interfaces, affective computing, virtual reality, ambient intelligence) and neuropsychology allows new directions with BCI technologies. Emerging BCI systems are often integrated within an advanced software environment that could improve quality of life of people by providing not only means for communication and control but by helping doctors and patients conduct more effective therapy and learn more about disabilities and treatment opportunities.
Still, the principal focus of many BCI research teams is to provide user/patient groups with very special needs with a new opportunity for communication and control. BCIs for spelling and basic communication have become more practical. The first commercial BCI system for home use, intendiX®, has been purchased and used by people without technical backgrounds, who used intendiX® to provide needed communication for patients. Recently, BCI systems have been designed for novel control applications for simple games and entertainment. The Screen Overlay Control Interface (SOCI) is designed to allow people to control a wide variety of applications with a BCI and has been validated with more advanced and mainstream games such as World of Warcraft and Angry Birds. Overall, there has been major progress in BCI research and development recently, as new user groups synergize with cheaper, easier, more flexible, and more robust BCIs.
3.1.2 BCI Illiteracy: An Ongoing Challenge
Amongst all the attention to new methods, applications, and user groups, one problem that has been present throughout the history of BCI research remains a concern today. Some people are unable to use some BCIs. Even with training, clear instructions, advanced and numerous electrodes, and cutting-edge electronics and signal processing, some BCI systems are unable to effectively distinguish between the brain states that users might use for control. Users might think about moving the left hand versus right hand or focus on specific stimuli, but the resulting EEG does not contain reliable differences between different conditions. This phenomenon has been dubbed “BCI illiteracy” [24]. Some people have found this term objectionable because it implies that the fault lies with the user and not the system designer. Other terms have been suggested or used such as “proficiency,” “inability,” or “inefficiency” [5, 28]. These terms refer to the same general phenomenon as “illiteracy,” a term we use in this chapter because it is most widely adopted in the literature.
Defining illiteracy, and comparing performance across studies, has been challenging because researchers use different criteria to distinguish between good performers and bad performers. Early work stated that 70 % accuracy was effective for a two-choice paradigm, while lower accuracy results would be acceptable with more choices [1, 35]. Other work treated 60 % as a control threshold for a two-choice BCI. More recent work has shown that the threshold for effective communication should incorporate other factors than the number of choices, such as the total number of trials [36, 37]. If a user only sends a short message, then a higher accuracy threshold may be needed.
Furthermore, direct comparisons of speed and accuracy are meaningless with some BCIs. For some purposes, including motor rehabilitation, any accuracy above chance level could be helpful. Asynchronous BCIs, defined below, cannot always be measured via speed and accuracy and may use other measures such as task completion time or error rate. Within this chapter, we focus our discussion on noninvasive BCIs that are intended for communication and control, rely on synchronous protocols, and are well suited to assessment of speed, accuracy, and bit rate. Fortunately, many BCIs meet these criteria.
Each of the following three sections discusses BCI illiteracy within one of the three major BCI approaches (ERD, P300, SSVEP). Each section discusses how each of these BCIs works, extending the introduction above, and then reviews studies that assess literacy across many users. Each section will describe the first published study that aimed at assessing BCI literacy within each of these three approaches and then newer work with improved methods and performance. The chapter concludes with emerging directions and general discussion.
3.2 How Many People Can Use a Motor Imagery BCI?
BCIs based on ERD/ERS (also called SMR BCIs) have many appealing features. For example, they usually require subjects to imagine movements, which can be very intuitive, natural control signals for navigation and many others (such as moving a wheelchair or an avatar). Users might imagine moving the left hand, right hand, or feet to move to the left, right, or forward. ERD BCIs also do not require external stimuli to generate the brain activity needed for control, although some external stimulation is necessary in any BCI to provide feedback.
However, ERD BCIs may require training subjects to attain adequate control and find a suitable type of imagery (such as playing tennis or weight lifting) and maintain attention on this imagery, which may be distracting or tiring. Their overall information transfer rate is slower than most P300 and SSVEP BCIs. ERD BCIs also may encounter greater problems with “illiteracy” than other BCIs ([20, 27, 28, 38, 39).
3.2.1 The First Study of ERD BCI Literacy
A very early study evaluated the accuracy of 99 BCI users (mean age 38 ± 22.4 years) controlling a two-class MI-based BCI [20]. Data were recorded at a local fair over a period of 2 months. Subjects were first prepared for BCI recording, which took about 10 min. The subjects sat in a comfortable armchair 150 cm in front of a computer screen and were asked to avoid unnecessary movement and keep both arms and their feet relaxed. Each subject had to perform two BCI runs separated by a short break—a training run followed by an application run. Each of these runs consisted of 20 trials of left-hand movements and 20 trials of foot movements, and the order of these 40 movements was determined randomly.
Each run started with the presentation of a fixation cross in the center of the screen (see Figs. 3.2 and 3.3). After 2 s, a warning stimulus was given in the form of a “beep.” After 3 s, an arrow (cue stimulus) pointing to the left or right was shown for 1.25 s. The subjects were instructed to imagine a right-hand movement or a both-feet movement until the end of the trial, depending on the direction of the arrow. In runs with feedback, the EEG patterns were detected and classified online throughout the session. For 3.75 s after the arrow disappeared, between second 4.25 and second 8 after arrow onset, the classification result was used to control the length of a horizontal bar that extended to the left or right. This horizontal bar changed in real time based on the classification result. After each trial, there was a random delay of 0.5–2.0 s before the next trial began. Hence, each binary decision required 8.5–10 s.
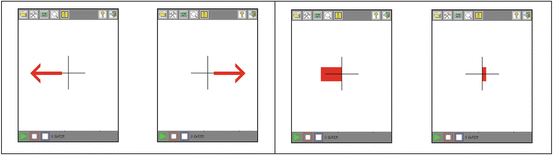
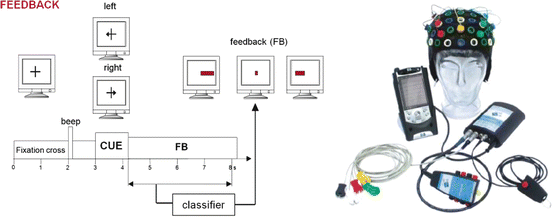
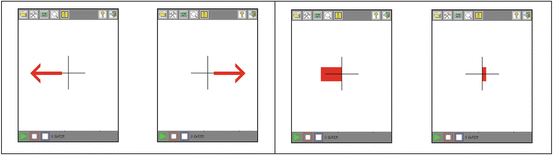
Fig. 3.2
These two panels show the display that the subjects viewed on a Pocket PC. The left panel is the display during the training phase. Red arrows indicate that the subject should imagine moving the left hand (left image) or both feet (right image). The right panel is the display during the application phase. The direction of the bar indicates the classification result: the left hand (left image) or both feet (right image). The length represents classification reliability
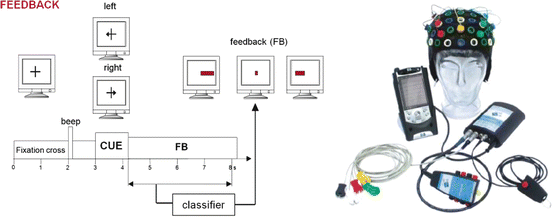
Fig. 3.3
The left panel shows the display along with the events occurring within each trial. The right panel is the hardware used
The EEG was recorded with gold electrodes from two bipolar channels over the right-hand and foot representation areas (2.5 cm anterior and posterior to electrode positions C3 and Cz of the international 10–20 electrode system), as shown in Fig. 3.4 (left side). Data were collected in a public environment [see Fig. 3.4 (right side)]. The EEG signals were amplified and band-pass filtered between 0.5 and 30 Hz and sampled at 128 Hz. For the analysis of the EEG patterns, (1) an adaptive autoregressive (AAR) model based on recursive-least-squares (RLS) algorithm (first month) and (2) band power (BP) estimation (second month) were applied. For BP estimation, the average power in the alpha (10–12 Hz) and beta (16–20 Hz) band at each electrode position was estimated. A total of 128 samples were averaged, yielding an estimation of the band power for a 1 s interval. These band power values were log transformed. In both cases, linear discriminant analysis (LDA) was used for the classification of the parameters. The classification result indicates the class to which the input belongs by the sign of the result. The magnitude of the result indicates the confidence in correct classification.
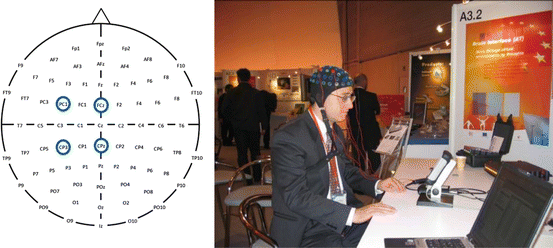
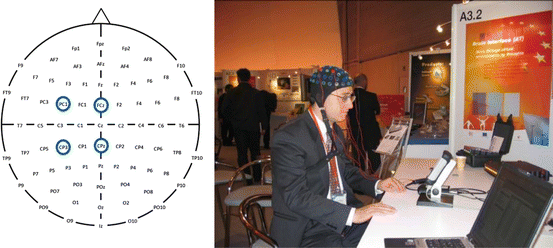
Fig. 3.4
In the left panel, the locations of the two bipolar electrodes are circled in blue. The right panel is a photograph of one of the subjects using this BCI system
Data from the first run were classified either based on AAR parameters or on BP values. The time point that yielded the minimal average classification error based on a 10 × 10 fold cross validation of a linear discrimination was identified, and the AAR and band power coefficients of this classification time point were used to set up the LDA classifier for the application run.
In about 20 % of the subjects, the two brain states were distinguished with an accuracy of greater than 80 % after only 20–30 min of training, as shown in Table 3.1. Furthermore, 70 % of the sessions were classified with accuracies between 60 and 80 %, and a marginal discrimination between brain states was possible in only 6.7 %.
Accuracy (%) | RLS (N = 76) | BP (N = 117) | RLS + BP (N = 193) |
---|---|---|---|
90–100 | 6.6 | 6.0 | 6.2 |
80–89 | 10.5 | 14.5 | 13.0 |
70–79 | 30.3 | 33.3 | 32.1 |
60–69 | 40.8 | 42.7 | 42.0 |
50–59 | 11.8 | 3.5 | 6.7 |
Total | 100 | 100 | 100 |
The results of this initial study with 99 people suggested that at least half of the healthy population could use a BCI based on motor imagery and that a high accuracy of above 90 % is possible for a few people. However, results also showed that certain subjects cannot achieve reasonable BCI control at all, at least for this kind of BCI. Performance might be improved by additional neuro-/biofeedback training, or subjects have to switch to another type of BCI such as a P300- or SSVEP-based approach.
3.2.2 The 64-Channel Sensorimotor BCI Study
As discussed in the previous section, some subjects could not attain reasonably high BCI performance with SMR-based BCIs. Comparing the performance and accuracies in recently published articles with P300- or SSVEP-based BCI approaches, discussed below, SMR-based approaches normally require more training, have lower accuracy, and have higher illiteracy. This might partly occur because, for practical reasons, only a few electrode positions are typically used with many applications. This study investigated how many people could control a motor imagery-based BCI with common spatial patterns (CSPs) using 64 EEG channels.
This multichannel study used a very similar experimental paradigm and EEG feature extraction approach as described in the two-channel study above. The multichannel study instead used a CSP approach, which requires more channels, to identify EEG data features based on spatiotemporal data preprocessing that can best be discriminated in the two-class BCI [40]. Furthermore, this newer study improves prior work in terms of user comfort and sensor mounting speed by using active electrodes, which do not require time-consuming procedures to abrade the scalp before mounting the electrode cap.
CSP is a method of developing a set of spatial filters that are designed to minimize the variance of one class while maximizing the variance of the other class. Given N channels of EEG, the CSP method provides an N × N projection matrix. This matrix is a set of subject-dependent spatial patterns, which reflect the specific activation of cortical areas during left- and right-hand movement imaginations. For most practical applications, only a small subset of the discriminating filters are needed. The two first spatial filters contribute most to the variance of class one data, and the last two spatial filters contribute most to the variance of class two data. With the projection matrix W, the decomposition of one trial of motor imagery is described by
where X is the channel x time EEG data matrix, the columns of W −1 are a set of CSPs and can be considered as time-invariant EEG source distributions, and Z is the transformed time series.

Due to the definition of W, the variance for a left-hand movement imagination is largest in the first row of Z and smallest in the last row of Z. The variance of a right-hand imagination is largest in the last row of Z and smallest in the first row of Z. The variances from the newly defined time series Z are extracted, similar to the band power feature extraction step from raw EEG. Unlike the extraction of band power features in the alpha and beta bands, no band-pass filtering of these bands is performed for CSPs. As discussed above, it is sufficient to extract the first two and the last two rows of Z, which reduces the dimensionality and hence the computational effort needed [41]. Data from a training session were utilized to construct the transformation matrix W. In the application phase, the corresponding four spatially filtered time series were normalized, log transformed, and finally classified by LDA.
This CSP approach, along with other signal processing, was set up through a rapid prototyping environment called g.BCIsys (g.tec medical engineering GmbH). Figure 3.5 presents the signal flow through the MATLAB Simulink model. Data were recorded at 64 scalp positions according to the international 10/10 system and sampled at 256 Hz. Active EEG electrodes (g.LADYbird, g.tec medical engineering GmbH) were used to increase data quality and user comfort. The multichannel g.HIamp biosignal amplifier (g.tec medical engineering GmbH, Austria) allowed impedance control of the active electrodes, and electrode impedances were kept below 30 kΩ. EEG was digitized by a 24 bit high-precision ADC, which fosters oversampling to increase the signal-to-noise ratio. Data were then band-pass filtered between 8 and 30 Hz before applying the transformation matrix W. Then, the variance of the resulting four time series was calculated within a time window of a length of 1, 1.5, or 2 s, respectively. These features were normalized, log transformed, and finally classified with the LDA. The classification result drives the BCI Paradigm feedback block that presents the classified MI to the user. The Simulink model also stores the EEG data with trigger information in MATLAB format and allows researchers to visualize the data for data quality inspection.
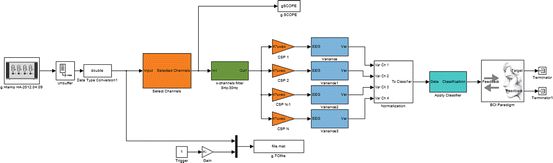
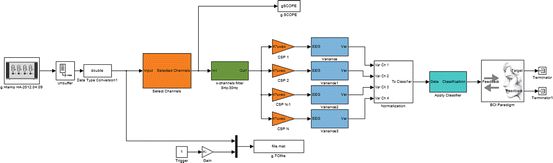
Fig. 3.5
The Simulink model. The spatial patterns approach is applied to 64 band-pass-filtered EEG channels. The resulting four channels of variance are normalized before classification. Finally, the feedback block presents the classified motor imagery to the user
A total of 20 healthy users (mean age 23.5 years, 15 male) participated in the multichannel study [42]. Users were recruited from the Johannes Kepler University Linz and the University of Applied Sciences Upper Austria. All subjects were new to BCIs. The electrode preparation took about 10 min. Users then performed one BCI session, lasting in total about 90 min. Each session consisted of seven runs, and each run included 20 trials for left-hand movement imageries and 20 trials for right-hand movement imageries in a randomized order. The timing of the experimental paradigm was identical to the two-channel study from 2003 (see Fig. 3.3).
The first run (run 1) was performed without providing any feedback. With the data from this run, a first set of spatial filters (CSP1) and a classifier (WV1) was computed. Before that, data were visually inspected, and trials with artifacts were removed. With this first set of spatial patterns and classifier, another four runs (run 2, run 3, run 4, and run 5) were performed while giving online feedback to the user. The merged data from these four runs (run 2345) were used to set up a second set of spatial filters (CSP2) and a classifier (WV2) that used more trials and was thus more accurate. We also compared whether a 1, 1.5, or 2 s variance window gives better results. Again, artifacts were manually rejected from the data before continuing with the calculation of CSP2 and WV2. Finally, to test the online accuracy during the feedback sessions, two more runs (run 6 and run 7) were conducted.
The results in Table 3.2 show that nearly all people can control an MI-based BCI system with CSPs and 64 EEG electrodes. One person achieved a perfect accuracy of 100 %, 19 persons were above 60 %, and only one person was below 60 %. Only one person did not achieve accuracy above 59 % within the training time of about 60 min, although five people had only marginal control between 60 and 70 %. The grand average accuracy was 80.7 %.
Table 3.2
This table summarizes the classification results of the CSP study
Accuracy (%) | Number of sessions | Percentage of sessions |
---|---|---|
100 | 1 | 5 |
90–99 | 5 | 25 |
80–89 | 5 | 25 |
70–79 | 3 | 15 |
60–69 | 5 | 25 |
50–59 | 1 | 5 |
Total | 20 | 100 |
The newer study with 64 channels and CSP clearly outperformed the two-channel implementation. Importantly, the CSP accuracies were calculated in real time and are therefore independent from the training data and were not cross-validated like done in the two-channel study. The CSP method would not be as effective with only a few electrodes. Ramoser and colleagues used a 56-channel montage and showed that performance declines below 18 electrodes [43]. CSPs require electrodes over the complete sensorimotor cortex to capture the important information. The two-channel study tried to minimize the number of channels, and therefore only two bipolar EEG derivations over the right-hand and feet motor regions were used. This bipolar derivation was similar for every subject and was not subject specific and adapted. In contrast, the CSP filter calculates an individual filter for each person that weights each electrode according to its importance. Figure 3.6 presents four examples of the most important spatial patterns derived by the CSP filter.
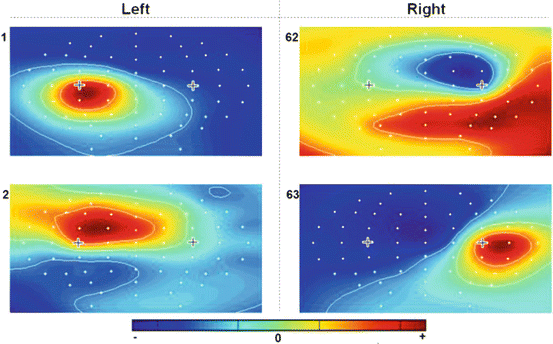
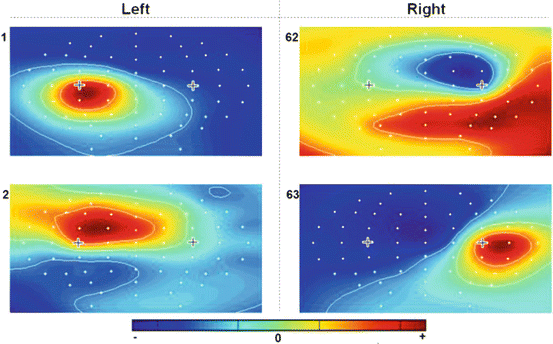
Fig. 3.6
Examples of the four most important common spatial patterns associated with left-hand imagination (two left images) and right-hand imagination (two right images) recorded with 64 channels. Clear, distinct patterns have been extracted for each of the two classes, which yields the basis for subsequent classification
In both MI studies, the population that could not control the BCI above 60 % accuracy was comparably low (5 % for CSP; 6.7 % for two-channel study). This outcome suggests that a certain percentage of users do not generate any classifiable ERD with motor imagery, no matter how many electrodes are used. Therefore, using more electrodes and improved signal processing may reduce illiteracy in ERD BCIs, but not eliminate it [28, 39]. The CSP study also entailed more training, which may have also improved performance. Additional training could improve performance further and might help illiterate subjects find mental strategies that lead to better control. Further studies would be needed to study these questions in more detail.
In this study, using active electrodes reduced preparation time and improved comfort relative to a 64-channel study with passive electrodes. The 2003 study required about the same preparation time (10 min) with two bipolar electrodes, since these were passive electrodes. Active electrodes can also improve performance because they are more resistant to noise. Some patients are often near numerous electronic devices, and other people may want to use BCIs reliably in real-world settings.
3.2.3 The Multichannel BCI for Stroke Rehabilitation
Recently, the idea of utilizing MI for neurological rehabilitation became popular. The idea is to use the BCI not to replace lost motor function, but to improve motor functions in patients. Mentally rehearsing movements (that is, performing MI) could be used as an effective therapy in stroke rehabilitation even if no feedback about the performance is given to the user. MI may be a method to overcome learned nonuse in chronic stroke patients and could also be practiced by patients with poor motor performance, which otherwise excludes four out of five patients from active movement therapies.
There are different advantages of using MI along with a BCI and online feedback, relative to MI alone: (1) the feedback helps and motivates the patient to perform accurate MI, (2) the therapist gets feedback about the performance of MI and can track changes over time, and (3) real-time feedback may increase Hebbian plasticity, which is likely to increase cortical activity. To test this approach, Ang et al. compared rehabilitation success across 54 hemiparetic stroke patients who received either standard robotic rehabilitation or rehabilitation with an MI-BCI and robotic feedback [44]. They showed that significant gains in Fugl-Meyer assessment scores were observed in both groups at post-rehabilitation and 2-month post-rehabilitation, but no significant differences were observed between groups. Furthermore, they proved that hemiparetic stroke patients can operate EEG-based MI-BCI and that EEG-based MI-BCI with robotic feedback neurorehabilitation is effective in restoring upper extremity motor function after stroke.
These results encouraged us to develop an MI-based brain–computer interface (BCI) that can help stroke patients control different feedback devices. The BCI was connected either to an upper limb rehabilitation robot (Amadeo, Tyromotion GmbH, Austria) or a virtual reality (VR) system (gVRsys, g.tec medical engineering GmbH, Austria). Both the VR system and the rehabilitation robot provide online feedback to the user about the detected MI (see Fig. 3.7). A total of 11 post-stroke patients and a control group of 11 healthy people took part in experiments so far. We present initial results from four healthy users performing the experiment with sensory feedback with the rehabilitation robot, followed by results from 11 healthy and 11 post-stroke patients using the VR system.
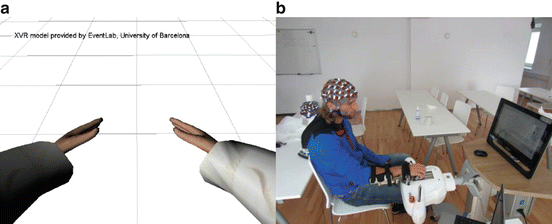
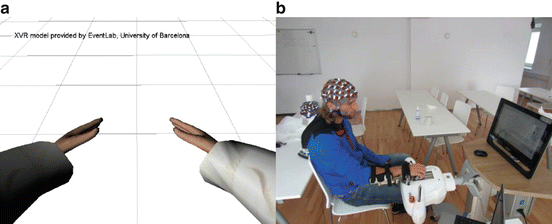
Fig. 3.7
The two feedback paradigms. Panel A is the VR paradigm, and panel B is the robotic feedback paradigm
3.2.3.1 The Stroke Rehabilitation BCI with Robotic Feedback
The BCI experiments were set up with g.BCIsys, as shown in the Simulink model in Fig. 3.8. The data were recorded over 64 positions distributed over the cortex and sampled at 256 Hz. Active EEG electrodes (g.LADYbird) were used to make the preparation procedure faster and easier and to increase data quality. A g.HIamp biosignal amplifier (g.tec medical engineering GmbH, Austria) was used for data recording. The unit has 256 ADCs with 24 bit precision and performs oversampling to increase the signal-to-noise ratio. Before applying the spatial filters, the EEG data were converted to double precision and band-pass filtered between 8 and 30 Hz. The variance from spatially filtered data was then calculated within a time window of 1.5 s length. These features were normalized, log transformed, and classified with the LDA. The LDA classification result drives the BCI Paradigm feedback block. This block controls the paradigm timing and sends the feedback commands to the external feedback device, either the rehabilitation robot or the VR system. The block ReceiveGRIPS_Signals tracks the actions of the rehabilitation robot and saves this data in synchrony with the EEG data for off-line analysis.
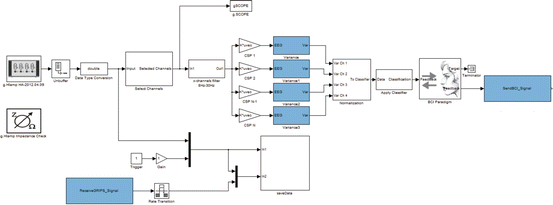
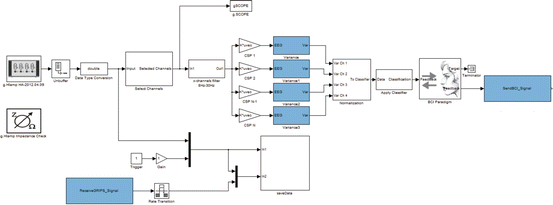
Fig. 3.8
The Simulink model. The spatial patterns are applied to 64 band-pass-filtered EEG channels. The resulting four channels of variance are normalized before classification. Finally, the BCI Paradigm block sends the feedback to the SendBCI_Signal block, which communicates to the VR or the rehabilitation robot. The block called ReceiveGRIPS_Signal receives the position of the single fingers of the rehabilitation robot to save them in synchrony with the EEG data
One experimental run lasted about 6 min and contained 40 randomized commands of either left-hand or right-hand MI. The timing of the experiment was exactly the same as for the two-channel study (see Fig. 3.3). Four healthy subjects (mean age 24 ± 5.2 years, two left-handed, two right-handed) participated in the tests with the rehabilitation robot Amadeo (see Fig. 3.7b). The Amadeo is a mechatronic finger rehabilitation device that allows each individual finger, including the thumb, to move independently and separately. The positions, as well as the forces of each finger, were measured constantly during the paradigm and saved with the EEG data, allowing detailed off-line analysis. One experimental paradigm consisted of four runs. With the data from the first three training runs, a specific classifier for detecting the MI was generated. No feedback was presented during the training runs. In the following run, this classifier was tested and the online error rate was calculated with the novel run. The robot gave feedback to only one hand. In a real rehabilitation session, this would be the affected side. For the healthy subjects, we selected the dominant hand to receive robotic feedback. The cue was given via a red arrow, pointing either to the left side or the right side of a computer screen. If the cue pointed to the side that was not fixed in the rehabilitation robot, the subject was asked to perform a real (not imagined) full flexion and extension of his/her fingers. If the cue pointed to the other hand, the subject was asked to instead imagine the same movement. When the correct MI was detected, the robot provided feedback by performing a flexion and extension of the five fingers. Within one trial, only one full flexion and extension was done. If no correct MI was detected during the feedback phase of the trial, then the robot performed no movement.
Table 3.3 presents the results from the four healthy subjects with robotic feedback. Notably, one subject displayed particularly poor performance, and the other three attained good control. The accuracy level is averaged over 40 trials.
Table 3.3
Accuracy rates of the healthy users participating in the robotic feedback paradigm
Session # | Accuracy (%) |
---|---|
1 | 92.50 |
2 | 95.00 |
3 | 68.70 |
4 | 90.00 |
Mean accuracy | 86.55 |
SD | 13.97 |
3.2.3.2 The Stroke Rehabilitation BCI with VR Feedback
A separate study explored the VR implementation of the MI-BCI for stroke rehabilitation. Eleven post-stroke patients (mean age 67.5 ± 10.3 years) and 11 healthy subjects (mean age 22.3 ± 4.2 years) participated in the tests with the VR system shown in Fig. 3.7. The measurements with stroke patients were performed at the Krzeszowice Rehabilitation Center, Poland. The measurements with the healthy users occurred at Technologies OG, Austria.
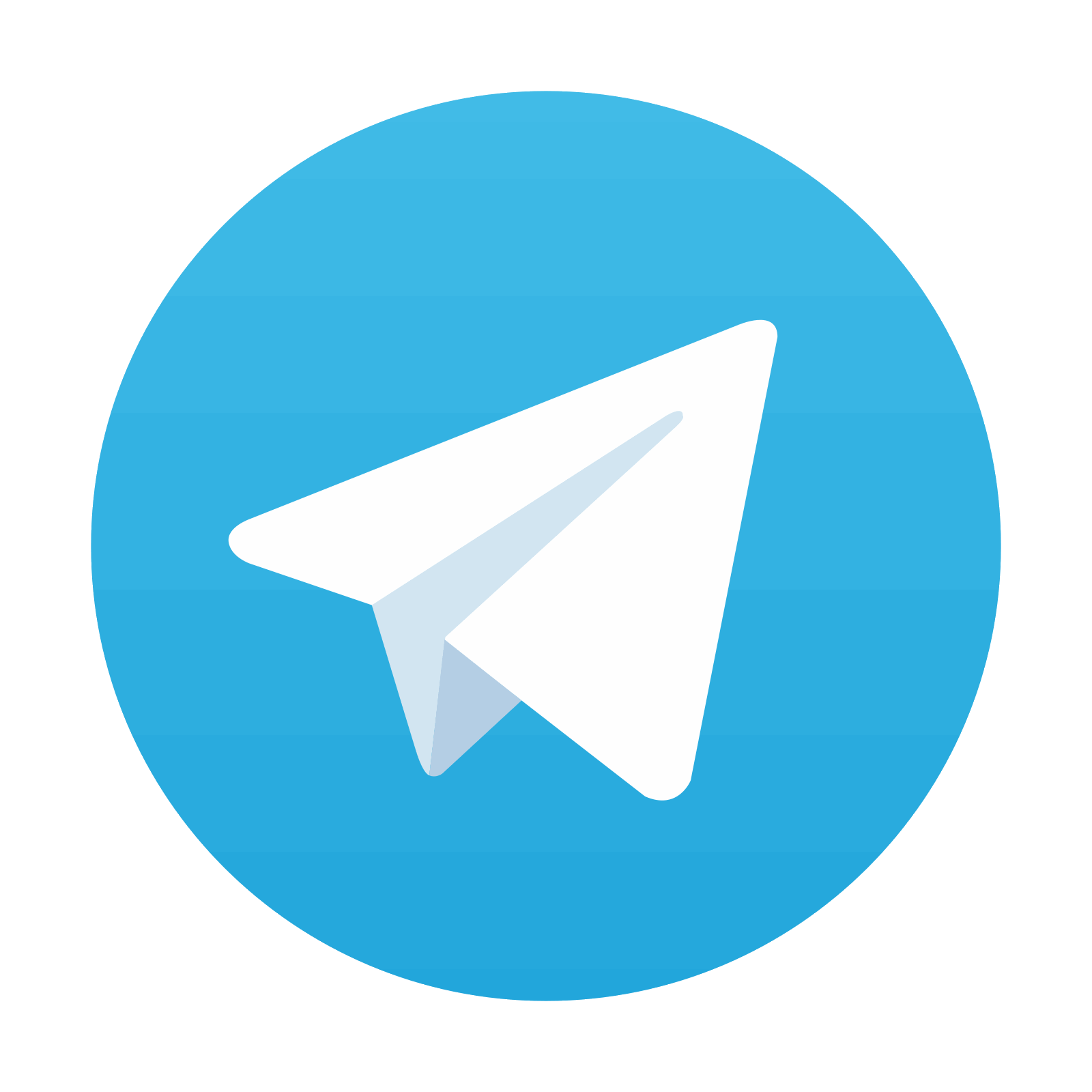
Stay updated, free articles. Join our Telegram channel

Full access? Get Clinical Tree
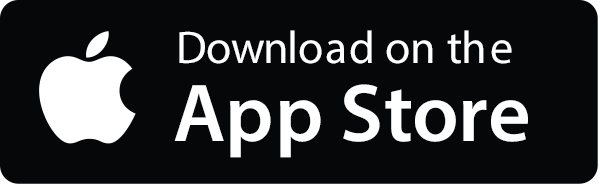
