Abstract
The Computational Neurosurgery Outcomes Center at Brigham and Women’s Hospital focuses on new knowledge creation and value-based innovation with big data, predictive analytics, patient-reported outcomes, and national databases. Work with artificial intelligence, including machine learning for structured data such as online medical records and natural language processing for unstructured data such as clinic notes, allows for analysis of high-velocity, high-volume, and high-complexity neurosurgical data. Use of mobile-based actively collected patient reported outcomes has been paralleled by the development of digital phenotyping and passive data collection. The study of surgical outcomes has evolved tremendously since the work of Ernest Amory Codman and big data, predictive analytics, and passively collected patient reported outcomes are the next frontier for outcomes research.
Keywords
Big data, Artificial intelligence, Machine learning, Natural language processing, Digital phenotyping, Patient reported outcomes, National databases
Introduction
Computational Neurosurgery Outcomes Center
Dr. Timothy R. Smith and Dr. William B. Gormley founded Computational Neurosurgery Outcomes Center (CNOC) at Brigham and Women’s Hospital and Harvard Medical School in 2015. The purpose of this center is to centralize disparate administrative, clinical, and financial data sources and detailed outcomes tracking and reporting in order to both internally increase quality improvement guidelines for high-value care delivery at Partners Healthcare and to externally synthesize big data to inform best practices and protocols for the international neurosurgical community.
Four major categories of new knowledge creation and value-based innovation at CNOC are
- 1.
Hospital-based process improvements.
- 2.
Implantable and wearable technology outcomes-data acquisition and sharing.
- 3.
Outcome prediction based on artificial intelligence.
- 4.
Improved access to specialty care via telemedicine.
Four examples of these potential value-based techniques include patient discharge optimization with automated medication reconciliation to help prevent readmission, the use of implantable and wearable technology to capture objective measures of patient function, the application of machine learning via natural language processing (NLP) and predictive model development to predict outcomes, and the expansion of telemedicine to increase access to care.
This chapter will focus on the role of CNOC in applying big data and predictive analytics to improve outcomes by reducing complications.
Big Data and Predictive Analytics
Big data can be understood as the combination of the three V’s: volume (quantity of data), velocity (rate of collection), variety (types of data collected). Neurosurgical data are characterized by enormous volume, rapid velocity, and multidimensional variety. This highly complex data stream requires adequately powered analytical tools. Artificial intelligence fits the bill with the predictive analytic techniques of machine learning and NLP.
Machine learning is the creation of meaningful insights without explicit rules-based instruction. Machine learning deals with structured data, such as online medical record or large national database spreadsheets of patient demographics, procedures, billing, and outcomes. In practice, this means algorithms that are able to ingest large quantities of data, learn general principles and relationships from these data, and then apply these principles and relationships to predict future outcomes. For example, a machine-learning algorithm can learn what principles dictate discharge to rehabilitation or discharge to home from a dataset of spine surgery patients. This algorithm can then predict the likelihood of a new patient being referred to rehabilitation versus discharged home. For example, Asadi et al. used machine learning to analyze clinical presentation, imaging, procedural details, complications, and outcomes for 199 patients undergoing 659 endovascular procedures for brain arteriovenous malformation and found that the algorithm was able to predict 30-day survival with greater than 95% accuracy.
Senders et al. conducted a systematic review of machine learning in neurosurgery and found ML algorithms used as “prediction models for survival, recurrence, symptom improvement, and adverse events in patients undergoing surgery for epilepsy, brain tumor, spinal lesions, neurovascular disease, movement disorders, traumatic brain injury and hydrocephalus.” Furthermore, the authors compared the prediction performance of machine learning and logistic regression models and found that machine learning models “performed significantly better and showed a median absolute improvement and area under the curve of 15% and 0.06, respectively.”
Senders et al. also conducted a systematic review to identify studies that evaluated machine learning models as clinical decision-making support systems for neurosurgical treatment. After screening 6402 relevant citations, they identified 221 studies with full-text screening that used “machine learning to assist surgical treatment of patient with epilepsy, brain tumors, spinal lesions, neurovascular pathology, Parkinson’s disease, traumatic brain injury, and hydrocephalus.” In particular, machine learning was used for surgical planning, intraoperative guidance, neurophysiological monitoring, and neurosurgical outcomes prediction.
NLP deals with unstructured data such as free text of clinic notes, pathology reports, or radiology reads. NLP can parse through unstructured data, remove nonmeaningful data, recursively manipulate the remaining data, and produce insights specific to the task on hand. For example, a clinical research study may be interested in determining patient quality of life after brain tumor resection by analyzing postoperative clinic notes. NLP can sift through thousands of clinic notes with ease and classify each note as documenting quality of life improvement, worsening, or no change. Other applications include rapidly processing radiology reads to stratify patients into operative or nonoperative candidates. Cohen et al. used NLP to analyze clinic notes from 6343 pediatric surgery epilepsy candidates. The authors then found that the algorithm was able to better predict surgical candidates from a new sample of 62 patients than a manual chart review by two pediatric epileptologists.
Similarly, Senders et al. conducted a systematic review of natural (human) intelligence versus artificial intelligence in neurosurgery and identified 23 studies in which artificial intelligence algorithms were used for diagnosis, presurgical planning, or outcome prediction. In these 23 studies, P -values were available for comparison on 50 outcome measures for human versus artificial intelligence. Of these 50 outcome measures, in 29 (58%) artificial intelligence models significantly outperformed clinical experts. In 18 outcome measures (36%), artificial intelligence models performed equivalent to clinical experts. Additionally, in four other studies that compared performance of clinicians aided by artificial intelligence to clinicians alone, all studies showed improved performance with artificial intelligence aid.
Patient-Reported Outcomes
An emerging area for big data in healthcare is patient-reported outcomes. Patient-reported outcomes in neurosurgery have conventionally focused on actively collected measures such as the Oswestry disability index (ODI), the Short Form 36, and the Visual Analog Scale (VAS), among others. Despite incredible advances in computing in every other industry, clinical encounters continue to require patients to fill out paper forms. Kim et al. studied the utility of the mobile device-based system (personal tablet computers) for collecting ODI and VAS scores in an outpatient spine clinic. They assessed the responses of 96 patients, of whom approximately 50% were over the age of 50, and found that 91.7% were satisfied with the mobile device system, 74% supported the use of the mobile system, 89.6% found the surveys to help physicians better understand the patients’ conditions, and 69.8% felt the time to understanding as a result of the surveys was decreased. At CNOC, Cote et al. similarly studied patient reported outcome measures in the neurosurgical clinic by administering an iPad survey (PROMIS10) related to physical and mental health pre- and postoperatively. Of 349 patients (630 procedures with predominantly fusions or laminectomies) enrolled from August 2014 to 2016, the initial PROMIS10 survey response rate was 83.1% with a median follow-up of 4.8 months.
The Onnela Lab at the Harvard TH Chan School of Public Health created Beiwe, a research platform including a study portal for researchers and a smartphone application for patients. Beiwe is securely encrypted and compliant with the Health Insurance Portability and Accountability Act (HIPAA). Torous et al. define digital phenotyping as the “moment-by-moment quantification of the individual-level phenotype in-situ using data from smartphones and other personal digital devices.” In this seminal work, they classify active data as “data that requires active participation from the subject for its generation, such as surveys and audio samples.” Passive data, on the other hand, are “data that are generated without any direct involvement from the subject, such as GPS traces.” Passive data able to be gathered by the application from patients’ smartphones include GPS traces of the patient’s activity, accelerometer readings, duration of phone calls, number of text messages, and unique WiFi networks interacted with over the course of a day. After Beiwe is downloaded on patients’ smartphones, the application passively collects multiple data streams and uploads the data to a central server where the information can be assessed by researchers. Active data gathered by the application includes customizable surveys and audio recordings by patients. Researchers can customize the questions in the administered surveys and the timing of surveys and they can track which surveys are completed and not completed by patients.
At present, Torous et al. are using Beiwe in a study of 20 patients with schizophrenia. The goals of the study are to collect active data in the form of self-reported symptom surveys based on psychiatric batteries, such as the Positive and Negative Symptom Syndrome Scale, and passive data including up to 1 million passively collected data points such as GPS, accelerometer, voice samples, call logs, text logs, and phone use data. The outcome measures for the study are “(1) adherence to and acceptability of the smartphone application (2) comparison of the smartphone application data to clinician collected metrics (3) sensitivity of the smartphone application in predicting symptom change through individual data streams (surveys, GPS, accelerometer, voice, call logs, text logs, screen data) and in combination.”
Work at CNOC by Cote et al. has applied digital phenotyping and the Beiwe smartphone application to the clinical symptoms and disease severity of neurosurgical patients. Cote et al. collected 1562 person-days of data from 44 patients, mean age 51.9 years, and found a daily survey response rate of 51.2%. Pain scores of participants were significantly associated with mobility, as measured by GPS sensors—“reporting significant pain (5–7) [compared to no pain] was associated with an average decrease of 0.52” locations visited in the past 24 h.
Apple Inc. (Cupertino, California) also launched its own smartphone-based application, ResearchKit, for clinical studies in 2015. ResearchKit allows researchers to develop informed consent forms, precreated surveys, and active tasks gathering information from the iPhone’s accelerometer, gyroscope, screen, and microphone. Bot et al. are using Apple’s ResearchKit iPhone app interface in the mPower study to observe Parkinson’s disease symptom severity and response to medication. In all, 9520 patients consented to the study, agreed to share data with the biomedical community, and were queried with baseline demographic data acquisition surveys, surveys for Parkinson’s disease assessment, such as the Parkinson Disease Questionnaire 8 (PDQ-8) and the Movement Disorder Society Universal Parkinson Disease Rating Scale (MDS-UPDRS). In addition, the application included assessments of patients’ memory using a spatial memory task, a tapping activity to measure dexterity and speed, a voice activity requiring patients to speak into the microphone, and a walking activity to monitor patient gait and balance over a 30-s period. Although many patients did not complete all of the tasks, 898 participants contributed data on at least 5 separate days over the first 6 months of the study.
There are multiple levels of innovation that need to be addressed in order to fully understand the impact of applications such as Beiwe and ResearchKit on patient-reported outcomes in neurosurgery. At present, patient-reported outcomes are collected actively by patients responding to surveys on paper during office visits. The first step demonstrated successfully by Kim et al. is to move the paper surveys into mobile devices. The next step is to move beyond conducting these active surveys only during office visits. At best, office-based surveys are a representative snapshot of the patient’s current condition. At worst, they are a random assessment, wholly unrepresentative of the lived experience of our patients. Using mobile device-based systems to administer active surveys throughout the course of the patient’s pre- and postoperative periods can provide a much more detailed understanding of time-dependent fluctuations in patients’ well-being. A huge unmet clinical needs for physicians is monitoring of time-dependent patient functioning in the nonclinical setting. Smartphone-enabled actively collected surveys will revolutionize the way patients can communicate suffering, recovery, and well-being. Finally, the most disruptive element of smartphone-based applications such as Beiwe and ResearchKit is the acquisition of passive data. In addition to collecting time-dependent actively reported information about patients’ well-being, these smartphone-based applications can curate patterns of patient mobility, societal functionality, and satisfaction before and after surgery without requiring patients to do anything more than carry around their smartphones.
National Databases
Another frontier for big data and predictive analytics in health care are national databases. In 2013, Mayo Clinic collaborated with Optum, a subsidiary of UnitedHealth Group, to form Optum Labs. At its inception, Optum Labs included claims and clinical data from “multiple health plans and healthcare providers for over 150 million people, covering 10 years or more of patient experience.” In February 2016, International Business Machines (IBM) Watson Health (Armonk, NY) created the Watson Health Cloud by acquiring Truven Health Analytics for 2.6 billion dollars, thereby aggregating records for approximately 300 million patient lives. Truven Health Analytics includes the MarketScan Research Databases, cost, claims, quality, and outcomes data for over 200 million unique patients since 1995. In addition to these massive commercial databases of patient clinical and financial information, there are multiple public sector aggregations of patient data including the Agency for Healthcare Research and Quality databases (such as National Inpatient Sample, State Inpatient Database), the American College of Surgeons databases (National Surgical Quality Improvement Program), and the Center for Medicare and Medicaid Services data sets, among others. Big data in healthcare, as such, are now available not only at the institutional level but also at the regional and national levels. Furthermore, the formation of huge claims-based data has been paralleled by the rise of specialty specific registries. For example, the National Neurosurgery Quality and Outcomes Database (N2QOD) was created by the American Association of Neurological Surgeons in 2012 to “systematically measure and aggregate surgical safety and 1-year postoperative outcomes data.” By creating and curating these huge repositories of patient data, health care has already immersed itself in the future of predictive analytics. Predictive analytics applied to these huge stores of data will bring benefits not only at the level of the individual patient (personalized medicine) but also to the health system as a whole by reducing avoidable errors and improving value-based care delivery.
Summary
The study of clinical outcomes has existed in surgery since the work of Ernest Amory Codman at Massachusetts General Hospital in the 1900s ; however, the field truly blossomed with the 2000 Institute of Medicine report “to err is human,” the emphasis on value-based care delivery by Porter and others, and regulatory changes tying compensation to outcomes. While the patient safety movement has matured since the beginning of the 21st century, patient-reported outcomes have only just begun to grow. Additionally, most of the work with patient-reported outcomes has focused on actively collected data, for example, requiring patients to fill out self-report quality of life surveys. Work with passively collected patient outcomes is the next frontier in outcomes research. All of these developments have laid an incredible foundation for predictive analytics to improve the care we deliver at the level of the individual and at the level of the nation. Our mission and work at the Computational Neurosurgery Outcomes Center is precisely aligned with this goal.
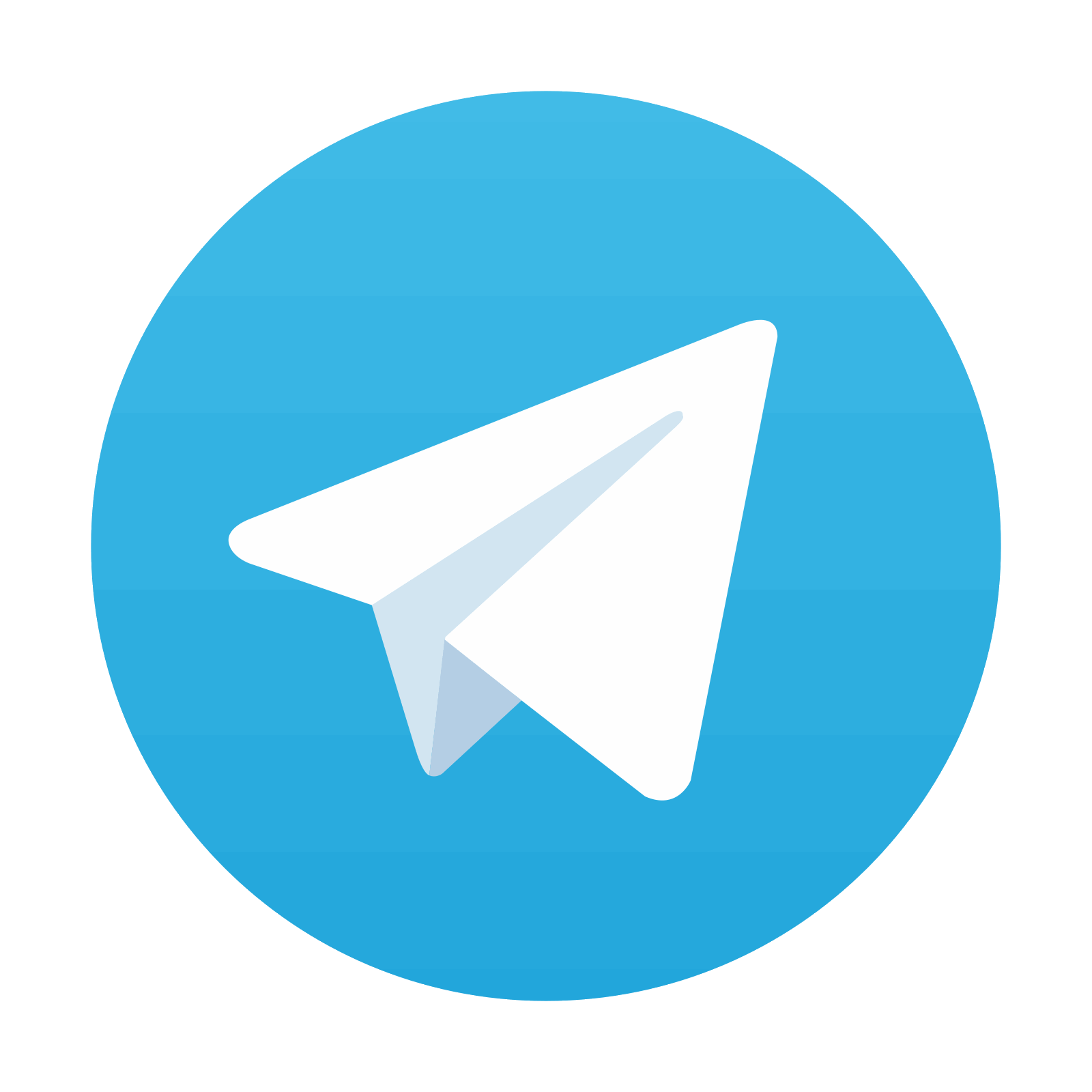
Stay updated, free articles. Join our Telegram channel

Full access? Get Clinical Tree
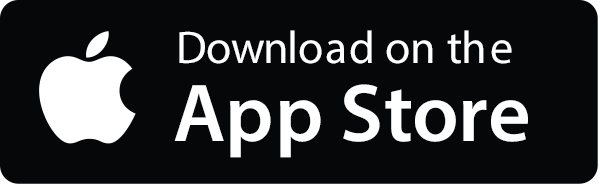
