Fig. 7.1
Two concepts of intermediate phenotype approach. Susceptibility genes for neuropsychiatric disorders do not directly encode for the clinical syndrome/behaviors. The abnormal behaviors observed in neuropsychiatric disorders are produced by intermediate steps that occur between genes and syndrome/behaviors. Intermediate steps such as cell activity and neural circuits underlie the syndrome/behaviors of neuropsychiatric disorders. Intermediate phenotype is likely associated with a more basic and proximal etiological process rather than pathogenesis of disease itself. (a) From genes to behavior. One concept is that intermediate phenotype approach is a tool to discover risk genes for neuropsychiatric disorders. (b) From behavior to genes. The other concept of the approach is a strategy to characterize neural systems affected by risk gene variants for elucidating the neural systems impaired in neuropsychiatric disorders
7.2 Intermediate Phenotype for Neuropsychiatric Disorders (Neurocognition, Neuroimaging, and Neurophysiology)
Intermediate phenotypes in medicine other than neuropsychiatric disorders, include lipid levels and risk of coronary heart disease (Castelli et al. 1992), sodium homeostasis and risk of hypertension (O’Connor et al. 2000), and body mass index and risk of diabetes (Walters et al. 2010b). The paradigm of an intermediate phenotype for neuropsychiatric disorders first emerged in 2001 (Egan et al. 2001). This study reported that a functional variation in the catechol-O-methyltransferase (COMT) genotype (Val158Met) was reported to be associated with altered prefrontal activity during a working memory task. The COMT is a major mammalian enzyme involved in the metabolic degradation of catecholamines; this activity is higher in the Val158 protein than in the Met158 protein. The Val158 genotype was associated with lower executive performance, less efficient physiological response in the prefrontal cortex, and a risk of schizophrenia (Egan et al. 2001). Since then, hundreds of articles related to intermediate phenotypes have been published, and several candidates for intermediate phenotypes of neuropsychiatric disorders have been identified in these articles. The intermediate phenotypes for neuropsychiatric disorders are roughly classified into: neurocognition, neuroimaging, neurophysiology, and others.
7.3 Previous and Recent Studies of Intermediate Phenotypes
We will focus on our previous and recent studies of schizophrenia and neurocognitive, neuroimaging, and neurophysiological intermediate phenotypes as examples, but this approach can also be extended to other neuropsychiatric disorders and neurodegenerative diseases.
7.3.1 Neurocognitive Intermediate Phenotypes
Neuropsychological studies of schizophrenia support the assumption that deficits in general cognitive ability, and more discrete aspects of neurocognition such as attention, memory and executive functions, are heritable, stable over the course of the illness, present in probands as well as in their healthy relatives and are differentially linked to the risk of schizophrenia (Burdick et al. 2009). Therefore, neurocognitive dysfunctions represent good potential candidates for intermediate phenotypes in studies of neuropsychiatric disorders, particularly schizophrenia. IQ, assessed by the Wechsler Adult Intelligence Scale (WAIS), perseverative errors and the number of categories achieved in Wisconsin Card Sorting Tests (WCST) to assess executive function, and the performance index (d’) of Continuous Performance Tests (CPT) used to assess attentional function, are all prominently used as neurocognitive intermediate phenotypes for schizophrenia, because their effect sizes are large (Allen et al. 2009; Burdick et al. 2009).
Using a candidate gene approach, and based on the following criteria: (1) strength of evidence for association with schizophrenia based on sample size and number of replications; (2) linkage to a gene locus associated with schizophrenia; (3) biological plausibility based on evidence of altered function and expression in vivo or in vitro; (4) evidence of altered expression in schizophrenic brain, based on measures of mRNA or protein, or relative expression of isoforms or alleles (Preston and Weinberger 2005), several candidate genes have been highlighted: COMT (22q11); DTNBP1 (6p22); NRG1 (8p12-21); RGS4 (1q21-22); GRM3 (7q21-22); DISCI (1q42); and G72(DAOA) (13q32-34). Firstly, we present associations between these genes and neurocognitive intermediate phenotypes. As described above, the association between COMT and schizophrenia using intermediate phenotypes, has already been investigated in this field. The study reported that the COMT genotype was related to perseverative errors in the WCST (Egan et al. 2001). They found that the low-activity Met158 allele carrier of COMT had a higher cognitive performance, and that the Val158 allele is significantly transmitted to schizophrenic offspring. These data suggested that the COMT Val158 allele impairs prefrontal cognition and physiology, and thereby slightly increases the risk of schizophrenia. Subsequent study found that high-activity Val158 allele homozygous had the poorest performance of working memory on n-back tasks (Goldberg et al. 2003). Siblings and patients with schizophrenia performed significantly worse than controls and the allelic effects on performance were found to be similar across groups. These findings were supported by other studies, but not all studies. In addition to the perseverative errors of WCST, the COMT polymorphism was associated with other neurocognitive intermediate phenotypes assessed by a Trail Making task, verbal fluency, verbal recall, IQ score and n-back task accuracy. However, a meta-analysis of reported associations between the COMT Val158Met and measures of memory and executive function, found no association between the COMT genotype and the majority of phenotypes, except for IQ score (Barnett et al. 2008).
The dystrobrevin binding protein 1 (DTNBP1) gene is linked to a risk for schizophrenia, and several studies, including ours have reported that several SNPs and haplotypes in the DTNBP1 gene influence general cognitive ability and memory function, in both schizophrenic patients and healthy control subjects (Hashimoto et al. 2009, 2010a). A meta-analysis supports two SNPs (rs1018381 and rs2619522) in DTNBP1 modestly influencing general cognitive ability (Zhang et al. 2010). Like these genes, several associations of other genes detected by candidate gene approaches with neurocognitive intermediate phenotypes, have been reported in this decade (Rose and Donohoe 2013): NRG1 and verbal fluency and attention; RGS4 and verbal fluency; DISC1 and memory function; G72(DAOA) and episode memory, working memory, verbal fluency and attention. Meta-analyses of the reported associations will be performed in the future. We have reported association studies between other genes that are implicated in schizophrenia and neurocognitive intermediate phenotypes (Hashimoto et al. 2007, 2013; Ohi et al. 2013b): PACAP and memory performance, KCNH2 and IQ, attention/vigilance and working memory and AKT1 and attention.
The GWAS of schizophrenia have identified several risk variants located in the ZNF804A, NRGN and TCF4 genes, MIR137 and MHC region, as a widely-used benchmark for genome-wide significance (p < 5.0 × 10−8) (Stefansson et al. 2009; O’Donovan et al. 2008; Ripke et al. 2011). The number of variants detected by GWAS is rapidly increasing (Consortium 2011; Ripke et al. 2013). The biological functions of many of these genes are unknown. Therefore, an intermediate phenotype approach has been used as a tool to investigate the biological function of these genes. The SNP rs1344706 in the ZNF804A gene was associated with schizophrenia in the first GWAS (O’Donovan et al. 2008); the biological function of this gene was unknown at that time. Subsequently, although several studies, including ours, have been reported (Fernandes et al. 2014; Stefanis et al. 2013; Walters et al. 2010a; Balog et al. 2011; Hashimoto et al. 2010b; Voineskos et al. 2011; Chen et al. 2012), the results were inconsistent. The rs1344706 variant was associated with a range of cognitive phenotypes, attention, memory, working memory and executive functions in some studies (Walters et al. 2010a; Balog et al. 2011; Hashimoto et al. 2010b; Voineskos et al. 2011; Chen et al. 2012), but other studies have reported no effect on the same phenotypes in other samples (Fernandes et al. 2014; Stefanis et al. 2013). Moreover, directions of reported association are also inconsistent. Some studies reported that the ZNF804A risk A allele was associated with better neurocognitive performance in patients with schizophrenia (Walters et al. 2010a; Chen et al. 2012), while others reported that the risk A allele was associated with worse performance (Balog et al. 2011; Hashimoto et al. 2010b; Voineskos et al. 2011; Chen et al. 2012). The rs12807809 located approximately 3 kb upstream of the NRGN, is a genetic variant detected by a second GWAS for schizophrenia (Stefansson et al. 2009). Neurogrannin (NRGN) plays an important role in the Ca2+–CaM signaling pathway, including the post-synaptic activation of CaM-dependent protein kinase II (CaMKII) by CaM, which is associated with strengthened N-methyl-d-aspartate (NMDA) receptor signaling (Li et al. 1999). Although several studies have investigated the association between the SNP and neurocognitive phenotypes (Donohoe et al. 2011b; Krug et al. 2013; Ohi et al. 2013c; Walters et al. 2013), the associations were not found to be significant. We found an association between IQ and NRGN diplotype (a combination of haplotypes) but not with SNP in patients with schizophrenia (Ohi et al. 2013c). The TCF4 rs9960767 was also detected by a second GWAS of schizophrenia. Based on evidence that Tcf4 transgenic mice display profound deficits in sensorimotor gating and contextual and cued fear conditioning (Brzozka et al. 2010), and that severe dysfunction of TCF4 causes Pitt–Hopkins syndrome with expression of mental retardation, microcephaly, facial dysmorphisms, and intermittent hyperventilation (Walters et al. 2010b), three studies have investigated influences of rs9960767 on neurocognitive intermediate phenotypes of schizophrenia (Lennertz et al. 2011b; Albanna et al. 2014; Lennertz et al. 2011a). Lennertz et al. reported an influence of the rs9960767 variant on verbal memory (Lennertz et al. 2011b). Contrary to the expectation that the schizophrenia-associated allele of the TCF4 gene would lead to impaired verbal memory, they found that the carriers of the disease-associated allele showed better neurocognitive performance, in patients with schizophrenia. Lennertz et al. did not detect any significant association between rs9960767 and other comprehensive neuropsychological tests in their other study (Lennertz et al. 2011a). Albanna et al. reported the risk allele of the SNP was related to worse performance in Reasoning/Problem-Solving (Albanna et al. 2014). One marker in the MHC region, rs6904071, was associated with delayed episodic memory (Walters et al. 2013).
7.3.2 Neuroimaging-based Intermediate Phenotypes
Structural and functional neuroimaging has yielded intermediate phenotypes for neuropsychiatric disorders, because a number of MRI studies have revealed whole brain and region-specific differences between patients and controls. Similar to neurocognitive intermediate phenotypes, many studies have showed evidence that neuroimaging data were suitable for intermediate phenotypes (Birnbaum and Weinberger 2013; Allen et al. 2009). As studies for functional neuroimaging have discussed elsewhere (Birnbaum and Weinberger 2013), we present only structural neuroimaging intermediate phenotypes. Voxel-based-Morphometry (VBM) and FreeSurfer techniques are mainly used to investigate gray matter abnormalities in patients with neuropsychiatric disorders compared with controls, while diffusion tensor imaging (DTI) technique is used to investigate white matter abnormalities.
As described above, we present in the order of genes detected by candidate gene and GWAS approaches. Many neuroimaging studies of COMT using structural magnetic resonance imaging (MRI) have reported significant associations between the Val158Met variant and brain structures (Ira et al. 2013; Ohnishi et al. 2006), although some studies have failed to replicate these findings (Barnes et al. 2009; Wang et al. 2013). A recent systematic review showed that smaller temporal and frontal brain areas were associated with the COMT Val allele in patients with schizophrenia, and their relatives (Ira et al. 2013). Several studies have found that the SNPs or/and schizophrenia-risk haplotypes in the DTNBP1 were associated with different brain regions such as the anterior cingulate cortex, hippocampal, prefrontal, occipital, and total brain volumes, but one study failed to find this association (Donohoe et al. 2010; Dutt et al. 2009; Narr et al. 2009; Trost et al. 2013; Tognin et al. 2011). Several associations between other genes and brain structures have been reported (Rose and Donohoe 2013; Buckholtz et al. 2007), although the associated regions were inconsistent, and the associated subjects were different (only in patients, controls or both subjects); RGS4 and decreased gray matter volumes in dorsolateral prefrontal cortex, thalamus and superior temporal gyrus, and white matter volumes in ventral prefrontal region; NRG1 and total gray matter, total white matter, lateral ventricle, frontotemporal and hippocampal volumes, and white matter density in the interior capsule; GRM3 and white matter integrity of cortico-cerebellar-thalamic-cortical circuit (Mounce et al. 2014); DISC1 and several gray matter volumes in the prefrontal cortex, superior frontal gyrus, hippocampus, insular cortex, anterior cingulate cortex and supramarginal gyrus, and fractional anisotropy in prefrontal white matter volume (Hashimoto et al. 2006); G72(DAOA) and frontal volume and cortical thickness in middle temporal, inferior parietal, and lateral occipital cortices. We have also reported associations between other genes and neuroimaging-based intermediate phenotypes; PACAP and hippocampal volume; VAV3 and superior and middle temporal gyri; AKT1 and inferior parietal lobule (Ohi et al. 2013b; Hashimoto et al. 2007; Aleksic et al. 2013).
Compared with other intermediate phenotypes, many of the associations between genetic variants detected by a GWAS approach and neuroimaging-based intermediate phenotypes have been reported. Several studies have used neuroimaging-based intermediate phenotypes to examine whether the SNP rs1344706 in the ZNF804A detected by the first GWAS, impacts upon brain structure, including gray matter and white matter volumes. However, the reported associations were inconsistent in either regions or subjects, as follows: Lencz et al. first reported that the risk allele homozygotes had larger total white matter volumes, and reduced gray matter volumes in several regions, including the angular gyrus, parahippocampal gyrus, posterior cingulate, and medial orbitofrontal gyrus/gyrus rectus, among healthy subjects when controlling for white matter volumes (Lencz et al. 2010). Voineskos et al. showed that individuals homozygous for the risk variant, demonstrated reduced cortical gray matter thickness in the superior temporal gyrus and the anterior and posterior cingulate cortices in healthy individuals (Voineskos et al. 2011). On the other hand, Donohoe et al. demonstrated that the risk allele when homozygous had relatively larger gray matter volumes, particularly hippocampal volumes, in patients with schizophrenia, but not in controls (Donohoe et al. 2011a). Wassink et al. showed this SNP influenced white matter volume in patients with schizophrenia and controls in opposite directions (Wassink et al. 2012). Wei et al. indicated that the risk allele carriers presented higher white matter density in the schizophrenia patients, and lower white matter density in healthy controls (Wei et al. 2012). There were some associations between the risk allele and lower fractional anisotropy in several regions (Kuswanto et al. 2012; Ikuta et al. 2013). On the other hand, there were also reports of no effect of the SNP on white matter integrity (Sprooten et al. 2012; Wei et al. 2013; Fernandes et al. 2014; Voineskos et al. 2011), on gray matter volumes (Wassink et al. 2012), on cortical thickness (Bergmann et al. 2013) or on any brain volumes (Cousijn et al. 2012).
The rs12807809 SNP around NRGN is also associated with several brain regions. Ohi et al. first reported the association of rs12807809 with the gray matter volume of the anterior cingulate cortex in patients with schizophrenia, but not in controls (Ohi et al. 2012). In addition, it was reported that the risk T-allele homozygotes had a thinner left entorhinal cortex in patients with schizophrenia (Thong et al. 2013). Two other studies showed an association between this SNP of NRGN and cortical thickness, but there are also opposite directions of the association (Rose et al. 2012; Walton et al. 2013). The MIR137 risk genotype was associated with reduced white matter integrity throughout the brain, as well as smaller hippocampi and larger lateral ventricles (Lett et al. 2013) in patients with schizophrenia. However, a study showed no association between this SNP and white matter microstructure (Kelly et al. 2014). One marker in the MHC region, rs6904071, was associated with hippocampal volume (Walters et al. 2013). A second stage GWAS in schizophrenia was conducted using the first stage GWAS to increase the sample size. This second stage study found additional risk genes, such as the CNNM2, PCGEM1, TRIM26, CSMD1, MMP16, NT5C2 and CCDC68 (Consortium 2011). The risk variant rs7914558 in the CNNM2 gene was associated with the volume of the bilateral inferior frontal gyri, and no significant association between other risk genes and gray matter morphology was observed (Ohi et al. 2013a). However, another research group showed increased gray matter volume in the temporal pole and the anterior cingulate cortex, in subjects with a risk allele in the CNNM2 (Rose et al. 2013a). The same group also reported no association between the risk SNP in CSMD1 and gray matter volume (Rose et al. 2013b).
7.3.3 Neurophysiological Intermediate Phenotypes
Neurophysiological examinations have the advantages of being easy to undertake and of using simple non-language-related stimulation, regardless of age, sex, race and language, as well as several neurophysiological deficits in schizophrenia which frequently occur before the onset of the psychotic symptoms has been reported. These neurophysiological candidate intermediate phenotypes in schizophrenia, include abnormalities in prepulse inhibition (PPI), smooth pursuit eye movements, auditory P50 event-related potential (ERP), P300, mismatch negativity (MMN) and prefrontal activation using near-infrared spectroscopy (NIRS) (Turetsky et al. 2007). Evidence suggests that many of these neurophysiological deficits are distinct from each other. They are stable, mostly independent of symptom state and medications, and are also observed in healthy relatives. These candidates have relatively high heritabilities (Greenwood et al. 2007; Sakakibara et al. 2014; Hall et al. 2006).
As described above, we present in the order of candidate gene approach-based genes and GWAS-based genes. Compared with neurocognitive and neuroimaging-based intermediate phenotypes, the number of reports using neurophysiological intermediate phenotypes is small. The COMT Met158 carriers affect elevated PPI levels in healthy subjects (Roussos et al. 2008) as well as in schizophrenia patients (Quednow et al. 2010), increased prefrontal activation during the verbal fluency task in patients with schizophrenia (Takizawa et al. 2009), and lower P50 deficits (Lu et al. 2007). On the other hand, the results using eye movements were inconsistent; COMT Met158 allele has an alleviating effect on eye movement disturbances in patients (Rybakowski et al. 2002) and in controls (Thaker et al. 2004), while the Met158 carrier is associated with worse performance on the antisaccade task both in patients (Thaker et al. 2004), and in controls (Haraldsson et al. 2010). Reported associations between other genes detected by candidate gene approaches and neurophysiological phenotypes are as follows (Rose and Donohoe 2013); NRG1 and P300 latency; RGS4 and eye movements (Kattoulas et al. 2012); GRM3 and MMN (Kawakubo et al. 2011); DISC1 and P300 latency (Blackwood et al. 2001). We have also reported some associations between other genes and neurophysiological intermediate phenotypes; RELA and PPI; SIGMAR1 and prefrontal activation; (Ohi et al. 2011; Hashimoto et al. 2011). There were a few studies investigating association of GWAS-based genes and neurophysiological intermediate phenotypes: ZNF804A and P300 (O’Donoghue et al. 2014; Del Re et al. 2014); TCF4 and PPI (Quednow et al. 2011) and P3 amplitude (Hall et al. 2014); MIR137 and P300 (Decoster et al. 2012).
7.3.4 Other Intermediate Phenotypes
As the concept of the intermediate phenotype expands, new candidate intermediate phenotypes are arising, such as gene expression in post-mortem brain and lymphoblast, personality traits using Temperament and Character Inventory (TCI), Neuroticism-Extraversion-Openness Personality Inventory (NEO-PI), and Schizotypal Personality Questionnaire (SPQ). However, as the conditions for intermediate phenotypes, such as heritability, stability over time, or showing increased expression in the unaffected relatives of probands, may be attributed to chance, the results obtained using these intermediate phenotype approaches should be interpreted carefully.
7.4 Future Development of the Intermediate Phenotype Approach
Overall, the numerous effects of the risk genetic variants in genes identified by both candidate gene and GWAS approaches on several intermediated phenotypes have been reported, but the reported associations have not been consistent. This inconsistency may be due to a small number of subjects in the association studies between genes and intermediate phenotypes, when compared with those in genetic association studies between genes and diseases. In addition, this inconsistency may also be due to differences in the methods of measurement (a different type of test) and the tested subjects (only patients, controls or both). Therefore, further replications of these studies, using larger sample sizes and a common methodology, and subsequent meta-analysis of reported associations, are necessary to lead to reliable conclusions.
To control confounding factors (e.g. medications and duration of illness), intermediate phenotypes studies were conducted using only healthy subjects. However, using only healthy subjects may overlook more characteristic associations observed in patients. In our opinion, studies using both patients and controls are important to investigate associations between genes and intermediate phenotypes. In addition, studies using unaffected relatives of patients with neuropsychiatric disorders, may also be a valid approach because they carry risk genes for the disorder without the confounding factors relating to the state of the disorder.
Following a genome-wide linkage study of intermediate phenotypes; e.g. eye movement (3p14), spatial processing (2p25 and 16q23), PPI (5p15), verbal memory (8q24), attention (10q26) and facial memory (10q26 and 12p12) (Greenwood et al. 2013), several GWAS using intermediate phenotypes as a phenotype have recently been published. These studies sought new genetic variations to explain the risk for neuropsychiatric disorders, as well as their underlying mechanisms of neurocognition, neuroimaging, and neurophysiology. In particular, GWAS on neuroimaging-based intermediate phenotypes have frequently been undertaken. In the early stages of GWAS on brain morphology, the Alzheimer’s Disease Neuroimaging Initiative (ADNI) carried out a GWAS of brain structures in fewer than 1000 subjects, including healthy controls and individuals with both mild cognitive impairment or Alzheimer’s disease. This research group used several brain phenotypes and methods of analysis (Shen et al. 2010; Stein et al. 2010a, b). Although extensive efforts using structural phenotypes and different strategies were made, these studies failed to identify new genetic variants with any genome-wide significance. Within the last few years, several large-scale collaborative consortiums, such as the Enhancing NeuroImaging Genetics through Meta-Analysis (ENIGMA) Consortium, have been established to overcome the problem of sample size (Thompson et al. 2014; Psaty et al. 2009; Schumann et al. 2010). The ENIGMA Consortium is a collaborative network of researchers working together on a range of large-scale studies that integrate data from 70 institutions worldwide. The ENIGMA studies have analyzed neuroimaging data from more than 12,000 subjects. ENIGMA’s first project was a GWAS to identify common genomic variants associated with hippocampal or intracranial volume (Stein et al. 2012). The intergenic variant rs7294919, located at 12q24.22, was associated with hippocampal volume, and rs10784502, located within HMGA2 at 12q14.3, was associated with intracranial volume. Researchers continue to explore genetic associations with subcortical volumes (ENIGMA2) and white matter microstructure (ENIGMA-DTI). They are also focused on understanding the impact of schizophrenia, bipolar illness, major depression and attention deficit/hyperactivity disorder (ADHD) on the brain. On the other hand, Hass et al. performed a GWAS of hippocampal volume using 328 subjects, including both healthy controls and schizophrenic patients, but no SNP was found to reach genome-wide significance (Hass et al. 2013). This failure may have been due to the small sample size employed.
Although several GWAS which investigated neurocognitive phenotypes impaired in schizophrenia, such as general cognitive ability, executive function, processing speed, and verbal fluency, have been conducted in other cohorts that did not include patients with schizophrenia (Davies et al. 2011; Cox et al. 2014; Luciano et al. 2011; Lencz et al. 2014; Benyamin et al. 2014), these studies reported that no single variant was found to be associated with any of the neurocognitive phenotypes at the level of genome-wide significance. To increase sample size and to identify any genetic variants related to neurocognitive intermediate phenotypes, the Cognitive Genomics consorTium (COGENT) – an international consortium of nine teams of researchers across seven countries – was founded, mirroring the ENIGMA neuroimaging consortium. On the other hand, there are few GWAS focusing on neurophysiological phenotypes in patients with schizophrenia or other neuropsychiatric disorders. GWAS on P300 did not find any genome-wide significant variant in approximately 1000 unrelated individuals drawn from a study of alcohol dependence (Zlojutro et al. 2010), while GWAS on MMN found a nominal genome-wide significant variant on chromosome 4q32.1 (p = 5.14E-8) in a small number of dyslexic children (Roeske et al. 2011). These failures may be due to the small effect size of each genetic variant on the phenotypes. To detect a genome-wide significant variant using GWAS of intermediate phenotypes, a larger sample size would be required because the level of significance in GWAS is very strict (<5.0E-8). Researchers in the field should, therefore, form a large consortium, such as ENIGMA and COGENT, to increase sample sizes and thereby detect the signals. This also highlights the importance of large-scale studies of intermediate phenotypes – both within and between categories – of neuropsychiatric disorders, such as the Consortium on the Genetics of Schizophrenia (COGS) and the Bipolar Schizophrenia Network on Intermediate Phenotypes (B-SNIP). An advantage of these studies is that they address the question of whether risk variants related to intermediate phenotypes are specific to schizophrenia or generalized to neuropsychiatric disorders across various conditions, including schizophrenia or bipolar disorder.
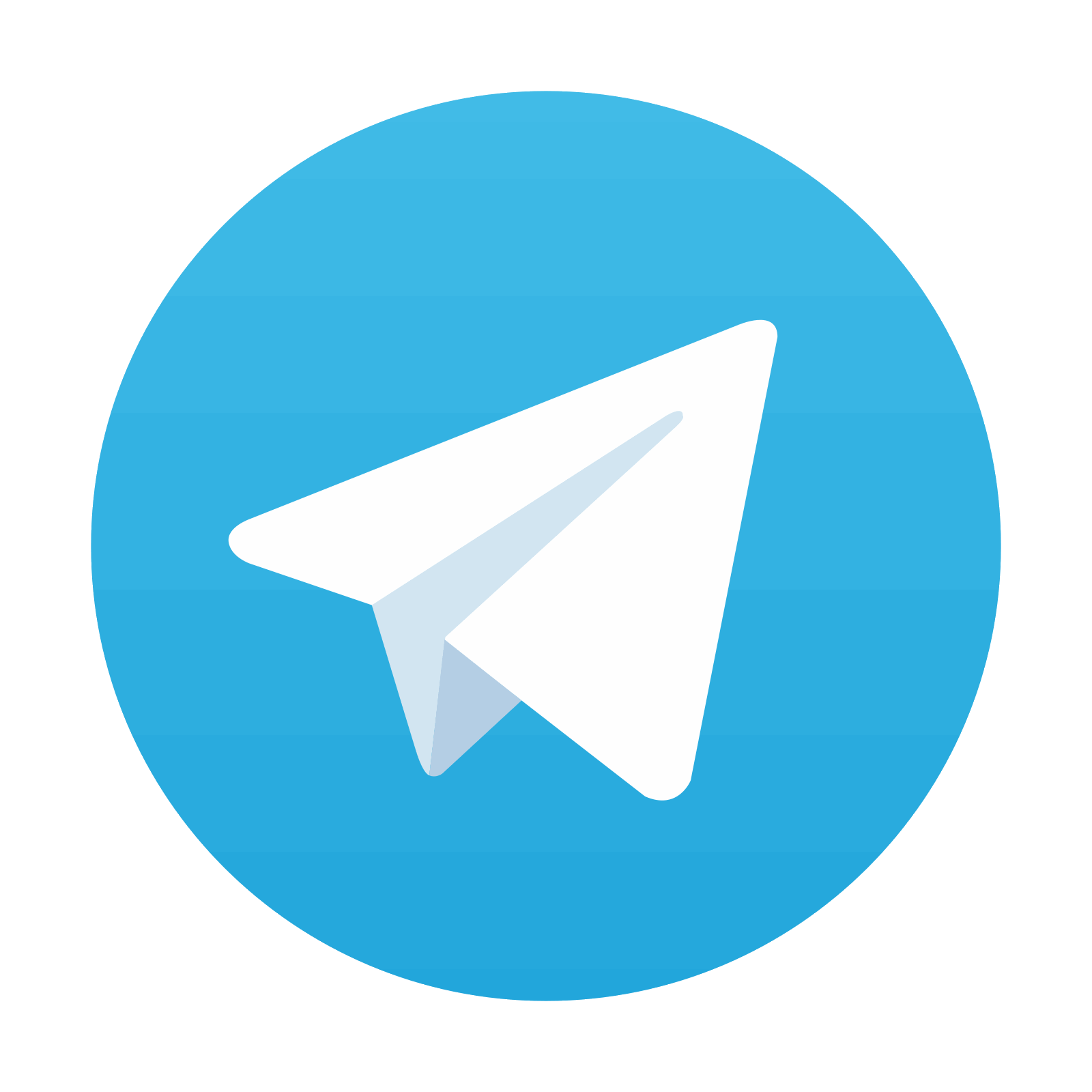
Stay updated, free articles. Join our Telegram channel

Full access? Get Clinical Tree
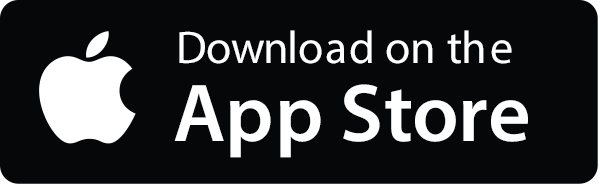
