Diagnostic testing is used to evaluate symptoms or abnormal physical examination findings. Testing should be ordered to assess the severity or stability of a medical problem or to evaluate an abnormal symptom or sign detected during a medical interview and examination. Routine testing or repeated testing of asymptomatic individuals with previously normal test results does not accurately predict which patients will develop complications but it does increase resource use and healthcare costs.
Screening for conditions associated with long-term morbidity or mortality
Identifying objective signs of improvement or progression of chronic disease
Monitoring levels of potentially toxic medications in the preoperative period (e.g., warfarin, digoxin)
Detecting drug-induced organ damage
Confirming the accuracy of previously obtained abnormal results
Pursuing a differential diagnosis of acute complaints, such as dyspnea or chest pain
Testing asymptomatic or low-risk patients
Repeating normal tests
Ordering screening tests with poor sensitivity
Ordering nonspecific batteries of tests that are unlikely to benefit patients
The term case finding refers to routine ordering of tests to detect unsuspected disease. Case finding generally does not significantly improve morbidity or mortality and is not an appropriate rationale for hospital testing.
Case confirming refers to testing patients for suspected disease or screening asymptomatic patients for diseases of public health importance.
CASE 11-1
You start your morning shift and have two patients handed over by the night shift. Both patients had chest pain during the night and negative troponins and EKGs.
Patient #1: Kelly is a 35-year-old morbidly obese woman with no pertinent past medical history admitted for intractable headaches. A review of systems is positive for burning, nonradiating substernal pain, which is worse at night and is unrelated to exertion. The burning sensation is not associated with nausea or diaphoresis and generally resolves on its own after several hours. Kelly is a non-smoker and has no contributory family history. Her serum total cholesterol level is 130 mg/dL. The pain during previous night was consistent with this past history.
Patient #2: Jack is an obese 65-year old man admitted for stroke. Jack has arterial hypertension, type 2 diabetes mellitus, dyslipidemia, and smokes cigarettes. He reports having occasional substernal chest pressure associated with dyspnea when he walks “faster than usual.” He developed chest pain last night while he was trying to get out of the bed and go to the bathroom.
You are considering referring each patient to the cardiology service for further testing. You speak to the cardiologist on the phone and inquire whether testing would be mandated in each case. She tells you that it depends on pretest probabilities because exercise stress testing is an imperfect test and has a sensitivity of 90% and a specificity of 80%. She tells you that there are a number of clinical decision rules that may be used in this case to calculate the pretest probability.
Pretest probability is the prevalence of the condition for which the test is being considered; that is, the percentage of patients with specific risk factors who have the disease at one given time. It may be defined as the clinician’s assessment of the probability that a patient has a particular condition or disorder before performing any testing.
A patient who has risk factors for a given disease based upon history, symptoms, physical findings, or demographic factors would have a higher pretest probability of having that disease than a patient with an absence of risk factors for that same disease. Pretest probability is derived from the clinician’s judgment about the prevalence of a disorder in the specific setting in which the patient is seen based on experience and ability to recognize patients and diseases that have similar clinical presentations.2,3,5
This clinical experience is coupled with knowledge gleaned from clinical research that has quantified the predictive ability of specific clinical signs and symptoms. Pretest probability may vary considerably for patients seen in different geographic regions or between primary, secondary, and tertiary care settings.
In considering diagnostic testing in the hospital setting, the clinician should first utilize knowledge of the patient’s risk factors and other information to determine the pretest probability for a given abnormality.2,3,5
If a disease is prevalent among individuals with risk factors similar to the patient, it is likely the patient has that disease. Under these circumstances, abnormal or “positive” test results may confirm the diagnosis but could also delay treatment of a serious condition. Normal or “negative” test results have the potential under these circumstances to be erroneous.
In contrast, if a disease is highly unlikely among individuals who are similar to the patient, then it is unlikely that the patient has that disease. In this case, diagnostic testing is usually unwarranted if there is a more likely diagnosis. In this situation, abnormal test results are likely falsely positive and could lead to unnecessary, and potentially hazardous, additional testing.
For both of these patients we are concerned about the possibility of coronary artery disease (CAD) and we are considering whether or not further cardiac testing is needed. We can refine our estimates of the pretest probability that each patient’s chest pain is due to obstructive coronary artery disease by considering:
How prevalent is heart disease among 65-year-old men versus 35-year-old women in the United States?
How likely is coronary disease in patients who smoke and have hypertension, diabetes, and hyperlipidemia? (Adding other comorbidities to the clinical context alters the pretest probability and, in the case of Jack, increases it significantly.)
How likely is CAD in patients experiencing nonexertional chest pain as described above? (Signs and symptoms also impact pretest probability. In the case of Kelly, her chest discomfort is atypical of obstructive coronary artery disease and does not increase her pretest probability above other patients with her demographics. For Jack, his angina is concerning for myocardial ischemia and would increase his pretest probability of coronary disease significantly.)
Clinical decision rules are instruments developed around specific case scenarios that combine key portions of the history, physical examination, and tests in order to inform estimates of pretest likelihood of a diagnosis or clinical outcome.6 A large number of commercially developed computer programs are now in widespread use that have compiled these resources, and increasingly, physicians are utilizing these to guide diagnostic and therapeutic decision making. Caution must be exercised, however, as the validity of clinical decision rules varies (some are based on expert consensus, single multivariate models, point scores, or a series of multivariate analyses) and the applicability to an individual patient may vary.
What are some examples of major clinical decision rules useful for the evaluation of hospitalized patients?
ABCD2 Score—assists in assessing stroke risk after a transient ischemic attack; Josephson SA, et al. Stroke. 2008;39(11):3096–3098.
APACHE II Score—assesses severity of illness for patients in intensive care unit settings; Knaus WA, Draper EA, Wagner DP, Zimmerman. APACHE II: a severity of disease classification system. Crit Care Med. 1985;13;818–829.
Atrial Fibrillation CHA(2)DS(2)—Vasc Score for Stroke Risk; Lip GY, Halperin JL. Improving stroke risk stratification in atrial fibrillation. Am J Med. 2010; 123(6):484–488.
CAGE Alcohol Abuse Questionnaire—identifies patients at high risk for alcohol abuse; Ewing JA. Detecting alcoholism. The CAGE questionnaire. JAMA. 1984;252(14):1905–1907.
Child-Pugh Classification—assists in evaluating the severity of chronic liver disease; grades correlate with 1 and 2-year survival; Desmet VJ. Hematology. 1994;19(6):1513–1520.
Duke Criteria—assist in establishing diagnosis of infective endocarditis; Durack, et al. Am J Med. 1994;96(3):200–209.
HAS-BLED Bleeding Risk for Atrial Fibrillation—assesses bleeding risk in patients with atrial fibrillation at 1-year follow-up; Pisters R, Lane DA, et al. A novel user-friendly score (HAS_BLED) to assess 1-year risk of major bleeding in patients with atrial fibrillation: the Euro Heart Survey. Chest. 2010;138(5):1093–1100.
Mini-Mental State Examination—assesses likelihood of significant cognitive impairment; Folstein MF, Folstein SE, McHugh PR. Mini-mental state. J Psychiatric Res.1975;12:189–198.
Modified Early Warning Score—identifies patients at early risk of deterioration based upon changes in vital sign parameters leading to emergent transfer; Reinei K. Eur J. Anesthesia. 2012;29(3):152–157.
STOP BANG Screening Tool—Screening tool for obstructive sleep apnea; Chung F. Anesthesiology. 2008;108(5):812–821.
Survive Sepsis Screening Tool (UK Sepsis Trust; Dr. JR Richardson, Dr R Daniels; sepsis.app.support@champagnewebs.com)
Well’s Criteria for Pulmonary Embolism (Wells PS, Anderson DR, Rodger M, Ginsberg JS, Kearon C, Gent M. Derivation of a simple clinical model to categorize patients’ probability of pulmonary embolism: increasing the model utility with the SimpliRED d-dimer. Thromb Haemost. 2000;83:418)
Risk scores derived from clinical decision rules can help evaluate the appropriateness of testing. For example, consider our scenarios above. We can make use of The Framingham Risk Score System to estimate the 10-year probability of cardiovascular disease based on the patient’s risk factors, including age, sex, blood pressure, lipid profile (or body mass index), smoking history, systolic blood pressure, history of diabetes, and the use of antihypertensive medications. An online calculator of 10-year cardiovascular risk is available at http://www.framinghamheartstudy.org/risk-functions/cardiovascular-disease/10-year-risk.php. The pretest probability of heart disease is 0.7% for a population of women with normal blood pressure, normal lipids, and no history of diabetes versus 88.5% for a population of older men with several cardiovascular disease risk factors (Table 11-1).
Estimation of 10-year Cardiovascular Risk Based Upon Clinical Risk Factors
Risk Factor | Kelly | Jack |
---|---|---|
Gender | Female | Male |
Age (years) | 35 | 65 |
Systolic BP (mmHg) | 110 | 130 |
Total cholesterol (mg/dL) | 130 | 280 |
HDL cholesterol(mg/dL) | 60 | 30 |
Antihypertensive treatment | No | Yes |
Smoking | No | Yes |
Diabetes | No | Yes |
10-year risk of MI, stroke, congestive heart failure (%) | 0.7 | 88.5 |
From these data, we see persuasive indications that the diagnostic yield for ischemic heart disease testing in Kelly will likely be quite low (Table 11-1).
The pretest likelihood of heart disease with Kelly’s risk factors and presentation is extremely low. Kelly is a very young woman with no significant risk factors for heart disease. Her pain is not likely caused by ischemic heart disease. Esophageal reflux is a more likely diagnosis. Is a stress test necessary or helpful for this patient?
In contrast, Jack is an older man with several risk factors for cardiovascular disease. Based on published cardiovascular disease risk factors, the likelihood that Jack has cardiovascular disease would remain high even if he had a normal/negative stress test.
Pretest probability is crucial when deciding whether or not patients require diagnostic testing. Pretest probability is influenced by the prevalence of a disease and by the patient’s individual risk factors. Using pretest probability, clinicians can reserve testing for situations where it is clearly indicated and likely to impact management.
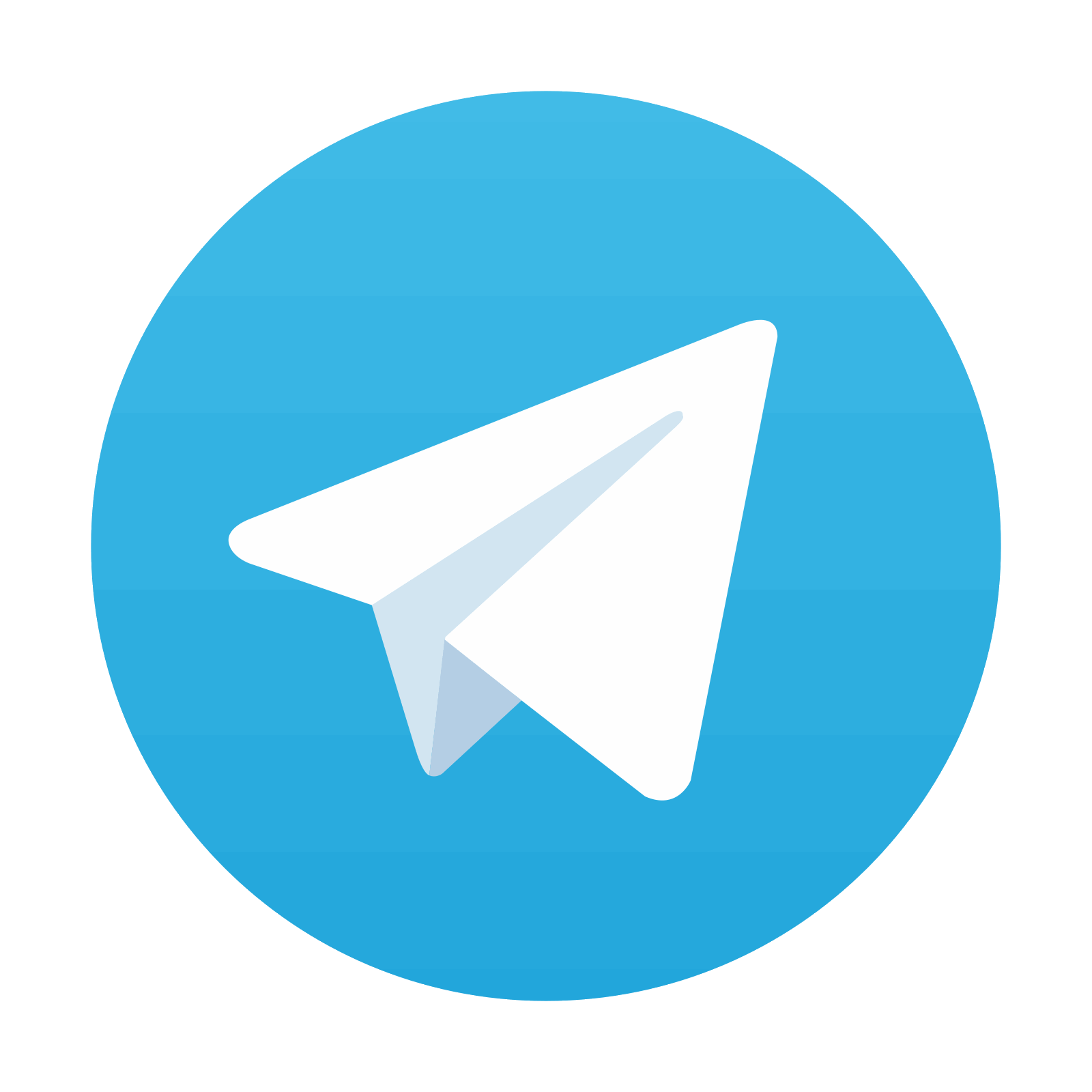
Stay updated, free articles. Join our Telegram channel

Full access? Get Clinical Tree
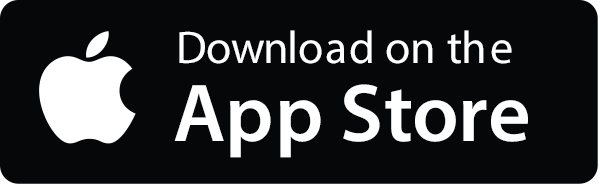
