(1)
Cognitive Function Clinic Walton Centre for Neurology and Neurosurgery, Liverpool, UK
Abstract
This chapter examines the statistical methodology of diagnostic test accuracy studies, emphasizing the various measures of discrimination and comparative measures which may be used to define the outcome of such studies, most based on the construction of a 2 × 2 table.
Keywords
DementiaDiagnostic test accuracy studiesMethodsStatisticsSensitivity and specificityLikelihood ratiosDemographic parameters in diagnostic test accuracy studies may require descriptive statistics (e.g., median age of population undergoing the diagnostic test; Sect. 4.1.2.1) whereas diagnostic parameters involving probabilities require inferential statistics. Unlike aetiologic research, where it is important to examine possible confounders (and hence use logistic regression as part of the analysis), diagnostic testing is generally independent of causal interpretation (Knottnerus and Muris 2002:56), and hence logistic regression may be reasonably eschewed, since it is difficult to implement in clinical practice.
Many of the issues and methods described briefly in this section are covered at greater length in texts devoted to statistical methods in diagnostic medicine (e.g., Zhou et al. 2011). It should be emphasized that what follows is a clinician’s heuristic approach to pragmatic statistics, not a statistician’s systematic approach to statistical theory.
3.1 Significance Tests: Null Hypothesis Testing
One possible way to examine the utility of a diagnostic test is to compare the mean test scores in groups with and without the target diagnosis, and compute whether the difference reaches statistical significance. Such significance testing is based upon rejection or acceptance of the null hypothesis. This may also be applied to proportions (e.g., the null hypothesis that the proportion of patients with a positive family history of dementia was the same in cognitively impaired and non-impaired groups, which was not rejected; Larner 2013a) as well as to mean test scores (e.g., the null hypothesis that test scores were not different between the demented and non-demented groups).
Continuous variables may be analysed using Student’s t test, assuming that the data come from a normal (Poisson) distribution and that the variability within the groups is the same. If data are not normal (i.e., skewed or asymmetrical), or if there is variability across groups, then non-parametric (“distribution-free”) methods may be used, such as the Mann–Whitney U test. Categorical differences may be analysed with the Chi-squared (χ 2) test or for proportions using the Z test. Significance threshold is usually set at a p value < 0.05; p values > 0.05 but <0.1 may be said to show a trend.
These aggregate data are of little use for individual patient diagnosis but are sometimes useful in the initial evaluation of diagnostic tests (for example in studies answering phase I and II questions: Sackett and Haynes 2002; Sect. 1.3.1) to ascertain how well they differentiate between disease and non-disease states (some examples are given in Sect. 4.3.1 and Table 4.2). Generally, measures of discrimination based on the 2 × 2 table are preferred in the analysis of diagnostic test accuracy studies.
3.2 The 2 × 2 Table; Table of Confusion; Confusion Matrix
Binary test results (above and below a chosen cutoff, threshold, or dichotomisation point; Sect. 2.2.3) may be cross-classified with the binary reference standard (disease present or absent; Sect. 2.2.1) in a 2 × 2 data table, also sometimes known as a table of confusion or a confusion matrix (Fig. 3.1). Hence every case assessed becomes classified as a true positive (TP; a), a false positive (FP; b), a false negative (FN; c), or a true negative (TN; d).
The dichotomisation of test results/scores in this way is useful for clinical and statistical interpretation, since a number of parameters of diagnostic test accuracy, known as measures of discrimination (Knottnerus and van Weel 2002; Sect. 3.3), may be derived from a 2 × 2 table, and hence this approach is often adopted. Suitable calculators for these parameters also exist (e.g., www.clinicalutility.co.uk). However, in the context of tests of cognitive function, it should be noted that studying cognition as a continuous variable affords greater statistical power (Altman and Royston 2006).
Moreover, it should be recognised that the symptoms and pathology of dementia disorders occur on a spectrum, and that the binary diagnostic categories are retained for their clinical utility.
Besides any theoretical objection(s), dichotomizing the data into a standard 2 × 2 table may prove difficult in practice. Patients may sometimes not be tested with either the index test or the reference standard, or these values may be lost or indeterminate. To accommodate these imperfections of real world practice, some authors advocate the use of a 3 × 3 table (Sappenfield et al. 1981; Sackett and Haynes 2002:31) or a 2 × 3 table (Schuetz et al. 2012), rather than the standard 2 × 2 table. Certainly an “intention to diagnose” and/or “intention to screen” approach, analogous to the “intention to treat” approach in clinical therapeutic trials, should be adopted in pragmatic diagnostic accuracy studies to avoid patient exclusion and loss of data which might bias results. Dichotomization may be inappropriate when the object of tests is something other than identifying simply the presence or absence of disease (i.e., the polytomous nature of tests). If there are more than two possible test outcomes (e.g., when considering outcomes, as with phase IV questions; Sect. 1.3.1.3) the table may need to expand, (e.g., to a 2 × 4 table; Burch et al. 2012).
3.3 Measures of Discrimination
Many measures of discrimination may be derived by analysis of a 2 × 2 table (Habbema et al. 2002; Qizilbash 2002), each with their own advantages and shortcomings for characterizing the findings of diagnostic test accuracy studies, some of which are discussed here.
Broadly one may distinguish between measures used to estimate the probability of disease in individuals (e.g., sensitivity, specificity, likelihood ratios) and measures used for global assessment of test discriminatory power but which cannot be used to estimate the probability of disease in individuals (e.g., diagnostic odds ratio, area under the receiver operating characteristic curve).
Understanding the results of diagnostic tests in terms of probabilities is a longstanding (Casscells et al. 1978) and persistent problem (Manrai et al. 2014) for clinicians. In particular, presenting probabilities as percentages, although a standard practice, can lead to confusion (Bodemer et al. 2014). For this reason, percentages have been eschewed in this discussion in favour of decimal fractions.
3.3.1 Accuracy; Error Rate; Net Reclassification Improvement (NRI)
Overall test accuracy, or correct classification accuracy, is given by the sum of true positives (a) and true negatives (d) divided by the total number of patients tested (Fig. 3.1):
This is sometimes stated as the number of individuals correctly classified by the test (e.g., Brown et al. 2014). There is some evidence that optimal test accuracy of cognitive screening instruments correlates with time of test administration (i.e., longer tests are more accurate for diagnosis; Larner 2015a).

Overall test accuracy is dependent on disease prevalence, as for predictive values (Sect. 3.3.3). Thus a test which is deemed accurate in one population may be deemed inaccurate in another population with different disease prevalence.
Overall test accuracy is seldom used in clinical practice, sensitivity and specificity being generally preferred. Optimal overall test accuracy according to the above formula may be used to define test cutoffs since this represents the optimal correct classification, although there are objections to the use of this methodology (see Sect. 2.2.3).
Overall test inaccuracy, or error rate, is given by the sum of false positives (b) and false negatives (c) divided by the total number of patients tested:
This parameter is seldom used in clinical practice, although perhaps should be (Larner 2015a).

Net reclassification improvement or net reclassification index (NRI) may be used to quantify test performance, expressed simply as the change in proportion of individuals correctly classified (e.g., as dementia or not dementia) on the basis of an investigation that is added to the existing diagnostic information (Pencina et al. 2008). Most simply this may be calculated as the difference between prior (pretest) probability of diagnosis (or prevalence of disease: Sect. 2.1.1.2) and posterior probability or test accuracy (Richard et al. 2013). NRI is claimed to be intuitive and easy to use (Richard et al. 2013).
Another measure of diagnostic test accuracy, not to be confused with overall test accuracy or correct classification accuracy, is the area under the receiver operating characteristic (ROC) curve (Sect. 3.3.7).
3.3.2 Sensitivity and Specificity; Youden Index
Yerushalmy (1947) introduced the terms sensitivity and specificity to promote understanding of the utility of diagnostic tests (Altman and Bland 1994a).
Sensitivity is a measure of the correct identification of true positives (a):
This is sometimes stated as the number of cases correctly identified by the test (e.g., Brown et al. 2009, 2014; Hancock and Larner 2011), or as true positive rate (TPR) or positive per cent agreement.

Specificity is a measure of the correct identification of true negatives (d):
This is sometimes stated as true negative rate (TNR) or negative per cent agreement.

There is always a balance or trade-off to be struck between test sensitivity and specificity, in other words they are inversely related according to the choice of test cutoff point (see Table 2.1 left hand two columns for an illustration of this trade-off between sensitivity and specificity). Choosing test cutoffs, the process of calibration (Sect. 2.2.3), will be determined, at least in part, by the needs of the particular clinical situation.
Despite their value as measures of diagnostic accuracy, sensitivity and specificity are of no use in estimating the probability of disease in individual patients (Akobeng 2007a).
If the aim of the test is to identify as many cases or true positives (e.g., of dementia) as possible (i.e., case finding, rule-in: Mitchell and Malladi 2010a, b), tests of high sensitivity but low specificity might be used, accepting that such a policy will inevitably result in many false positives (b) or “overcalls”. The false positive rate (FPR) is a measure of the probability of the absence of disease in the presence of an abnormal test, or a measure of the incorrect identification of positives, defined as:
Thus, as test sensitivity increases and specificity decreases, false positives will increase; as sensitivity falls and specificity increases, false positives will decrease. For a test with perfect specificity, there will be no false positives.

Alternatively, if the aim of the test is to exclude as many normals (i.e., disease free individuals) as possible (i.e., screening, rule-out: Mitchell and Malladi 2010a, b), tests of high specificity but low sensitivity might be used. This will minimise false positives (b) but accept more false negatives (c). The false negative rate (FNR) is a measure of the probability of the presence of disease in the presence of a normal test, or a measure of the incorrect identification of negatives, defined as:
Thus, as test specificity increases and sensitivity decreases, false negatives will increase; as specificity falls and sensitivity increases, false negatives will decrease. For a test with perfect sensitivity, there will be no false negatives.

Table 3.1 illustrates this trade-off between sensitivity and FNR, and between specificity and FPR, using data from several pragmatic diagnostic test accuracy studies of cognitive screening instruments performed under relatively uniform conditions (setting, operationalization of reference standard).
Table 3.1
Summary of sensitivities, false positive rates (FPR), specificities, and false negative rates (FNR) for different cognitive screening instruments at optimal cutoffs (defined by maximal test accuracy) for the diagnosis of dementia as defined in pragmatic diagnostic test accuracy studies
Sensitivity | FPR | Specificity | FNR | Reference | |
---|---|---|---|---|---|
MMSE | 0.70 | 0.11 | 0.89 | 0.30 | Larner (2013b) |
MMP | 0.51 | 0.03 | 0.97 | 0.49 | Larner (2012a) |
Codex | 0.84 | 0.18 | 0.82 | 0.16 | Larner (2013c) |
ACE | 0.85 | 0.17 | 0.83 | 0.15 | Larner (2007a) |
ACE-R | 0.87 | 0.09 | 0.91 | 0.13 | Larner (2013b) |
M-ACE | 0.46 | 0.07 | 0.93 | 0.54 | Larner (2015b) |
6CIT | 0.88 | 0.22 | 0.78 | 0.12 | Abdel-Aziz and Larner (2015) |
DemTect | 0.85 | 0.28 | 0.72 | 0.15 | Larner (2007b) |
MoCA | 0.63 | 0.05 | 0.95 | 0.37 | Larner (2012b) |
TYM | 0.73 | 0.12 | 0.88 | 0.27 | Hancock and Larner (2011) |
AD8 | 0.97 | 0.83 | 0.17 | 0.03 | Larner (2015c) |
False inflation of test sensitivity and specificity may occur if patients with a negative index test are not subjected to the reference standard (e.g., if it is invasive, risky, expensive), with their consequent categorisation as true negatives (d; Fig. 3.1) when they are actually false negatives (c). This verification bias (Sect. 1.4.2.2) will falsely inflate the value of d, the numerator of specificity, and reduce the value of c, which contributes to the denominator of sensitivity.
Clinicians may prefer tests with high sensitivity, whereas researchers may prefer high specificity (Tate 2010:250). In other words, when looking for cognitive impairment clinicians may be prepared to accept false positives which inevitably come with highly sensitive (poorly specific) tests, in preference to tests with high specificity and hence with false negatives (i.e., missed diagnoses). In contrast, research criteria may adopt a principle of high specificity. For example, in the IWG-2 diagnostic criteria for Alzheimer’s disease, Dubois et al. (2014:616) state that “(f)or research purposes – clinical trials, validation of new biomarkers, or follow-up of patient cohorts – the general approach is to recommend that cutoff points are selected within given target populations that have high specificity for an early AD diagnosis, potentially at the expense of lower sensitivity”. Whether such rigorous research criteria can be applied in clinical practice is moot: evidence of in vivo pathophysiological biomarkers, either CSF changes in Abeta and tau or positive amyloid PET imaging, is a requirement for AD diagnosis in these criteria, but these investigations are not universally available and, since they are costly, may not become so. Since all tests are likely to have some false positives and/or false negatives, it may be better to regard them as “screening” rather than diagnostic tests, indicating those patients who need further investigation and/or clinical follow-up to establish diagnosis (delayed verification).
Disease prevalence in the setting in which the diagnostic test is deployed may also influence decisions about where test cutoffs should be set and hence test sensitivity and specificity. If the target diagnosis has a low prevalence (e.g., dementia in community-based settings), test cutoffs maximising sensitivity may be desirable, minimising false negatives and tolerating false positives. Conversely in a high prevalence setting (e.g., dementia in a specialist memory clinic), test cutoffs maximising specificity may be desirable, minimising false positives and tolerating false negatives.
Either strategy has potential costs for patients: to be labelled with a disorder which is not in fact present may cause unnecessary anxiety and lead to unnecessary treatment and lifestyle changes; to be falsely reassured that disease is not present may delay the onset of appropriate treatment. These difficult arguments are also of relevance in considering how “costworthy” screening tests are (Ashford 2008), and in performing weighted comparison (Sect. 3.4.4) which requires some definition of how many false positives a true positive diagnosis is worth.
Recommendations on the desirable sensitivity and specificity of diagnostic tests have not, to the author’s knowledge, been published. The World Health Organization (WHO) disease screening criteria require, inter alia, that there should be a suitable test or examination to detect the disease with “reasonable sensitivity and specificity” (Wilson and Jungner 1968; Moorhouse 2009). Sensitivity and specificity no less than 0.8 (or 80 %) has been deemed appropriate and desirable for biomarkers of Alzheimer’s disease (The Ronald and Nancy Reagan Research Institute of the Alzheimer’s Association and the National Institute on Aging Working Group 1998).
Both STARD (Bossuyt et al. 2003) and STARDdem (Noel-Storr et al. 2014) guidelines recommend that “sensitivity and specificity” be amongst the keywords of papers reporting diagnostic test accuracy studies.
In addition to sensitivity and specificity, reporting the actual numbers of true positive, false positive, false negative, and true negative cases is, in the author’s view, to be recommended in diagnostic test accuracy studies, not least in order to facilitate meta-analysis of such studies.
Since there is a trade-off between sensitivity and specificity (Table 2.1, left hand two columns; Table 3.2, left hand two columns), what is often most important for the characterization of a diagnostic test is the combination of both sensitivity and specificity. Indeed it has been argued that “neither sensitivity nor specificity is a measure of test performance on its own. It is the combination that matters” (Habbema et al. 2002:117). But can the two parameters be meaningfully combined, and if so how? Various methods have been used. Youden (1950) defined the Youden index (Y), or Youden J statistic, defined as:
The Youden index has achieved some breadth of usage. Optimal test cutoffs (Sect. 2.2.3) may be defined using the highest Youden index, maximising sensitivity and specificity based on ROC curve analysis (Table 2.1, right hand column; Sect. 3.3.7). Other studies have simply quoted the sum of sensitivity and specificity (e.g., McCrea 2008; Hancock and Larner 2009a:190), whilst others cite a diagnostic efficiency defined as a “weighed summation of sensitivity and specificity” (Devigili et al. 2008).
Table 3.2
Data from a pragmatic diagnostic accuracy study of the Patient Health Questionnaire-9 (PHQ-9; Kroenke et al. 2001) for the diagnosis of dementia showing test sensitivity, specificity, and positive and negative likelihood ratios at different cutoff values ranging from best specificity to best (perfect) sensitivity
PHQ-9 cutoff | Sensitivity | Specificity | Positive Likelihood Ratio (LR+) | Negative Likelihood Ratio (LR−) |
---|---|---|---|---|
1 | 0.39 | 0.77 | 1.65 | 0.80 |
2 | 0.47 | 0.69 | 1.50 | 0.77 |
3 | 0.53 | 0.56 | 1.21 | 0.83 |
4 | 0.63 | 0.50 | 1.27 | 0.73 |
5 | 0.63 | 0.47 | 1.19 | 0.78 |
6 | 0.71 | 0.45 | 1.31 | 0.63 |
7 | 0.78 | 0.45 | 1.42 | 0.50 |
8 | 0.86 | 0.44 | 1.52 | 0.32 |
9 | 0.86 | 0.44 | 1.52 | 0.32 |
10 | 0.88 | 0.41 | 1.48 | 0.30 |
11 | 0.88 | 0.39 | 1.44 | 0.31 |
12 | 0.90 | 0.38 | 1.44 | 0.27 |
13 | 0.90 | 0.28 | 1.25 | 0.36 |
14 | 0.92 | 0.27 | 1.25 | 0.31 |
15 | 0.92 | 0.23 | 1.20 | 0.35 |
16 | 0.92 | 0.22 | 1.18 | 0.37 |
17 | 0.92 | 0.17 | 1.11 | 0.47 |
18 | 0.96 | 0.16 | 1.14 | 0.26 |
19 | 0.96 | 0.14 | 1.12 | 0.29 |
20 | 0.96 | 0.11 | 1.08 | 0.37 |
21 | 0.98 | 0.09 | 1.08 | 0.22 |
22 | 1.00 | 0.08 | 1.08 | 0 |

The heterogeneity of clinical populations imposes potentially serious limitations on the utility of sensitivity and specificity measures, since very different values of these measures may be found in different patient subgroups within the sampled population (Moons et al. 1997a).
3.3.3 Predictive Values; Predictive Summary Index
Predictive values give the probability that a test will give the correct diagnosis, information not available from the sensitivity and specificity (Altman and Bland 1994b; Akobeng 2007a).
Positive predictive value (PPV) is the probability of disease in a patient with a positive test:
PPV is of value in answering the question of how useful or meaningful a positive test result is (Forsyth 2003:i11). PPV is also a measure of posttest probability (Sackett and Haynes 2002:29) which may be compared to pretest probability or prevalence (Sects. 2.1.1.2 and 4.1.2.3). Posttest probability should not be confused with sensitivity (Sect. 3.3.2) or posterior probability (Sect. 3.3.1).

Negative predictive value (NPV) is the probability of the absence of disease in a patient with a negative test:
PPV and NPV may be combined using the predictive summary index (PSI; Youden 1950), defined as:
PPV and NPV are influenced by the prevalence of the target disease in the population undergoing testing (as a simple example will show: Box 3.1). As a consequence of this fact, tests which are very sensitive and specific (and hence accurate) may yet have a very low PPV if the condition being sought is uncommon in the population being tested (e.g., Woolf and Kamerow 1990:2452).


Box 3.1: Dependence of PPV on Disease Prevalence
Q. If a test to detect a disease whose prevalence is 1 in 1,000 (0.1 %) of the community population has a sensitivity of 0.95, what is the probability that a person found to have a positive result actually has the disease?
A. If sensitivity is 0.95 (and by extension false negative rate is 0.05), then in a population of, say, 100,000, there will be 100 cases (=1 in 1,000) of which 95 are detected by the test (true positives; a) and 5 are not (false negatives; c). Of the (100,000–100) = 99,900 non cases, one would anticipate that 5 % (=4,995) would test false positive (b), leaving (99,900–4,995) = 94,905 true negatives (d). Hence, the positive predictive value of the test is a/(a + b) = 95/95 + 4,995 = 95/5,090 = 0.019, or approximately 1 in 50.
Alternatively it could be argued that if 1,000 people are tested, because of the known prevalence of 1 in 1,000, one person has disease, but because of the 5 % false negative rate of the test, then 50 people will test positive, hence the chance of someone with a positive test actually having disease is 1/50 = 0.02.
Q. If the same test with the same sensitivity is then applied in an outpatient clinic population where the disease prevalence is 200 in 1,000 (20 %), what is the probability that a person found to have a positive result actually has the disease?
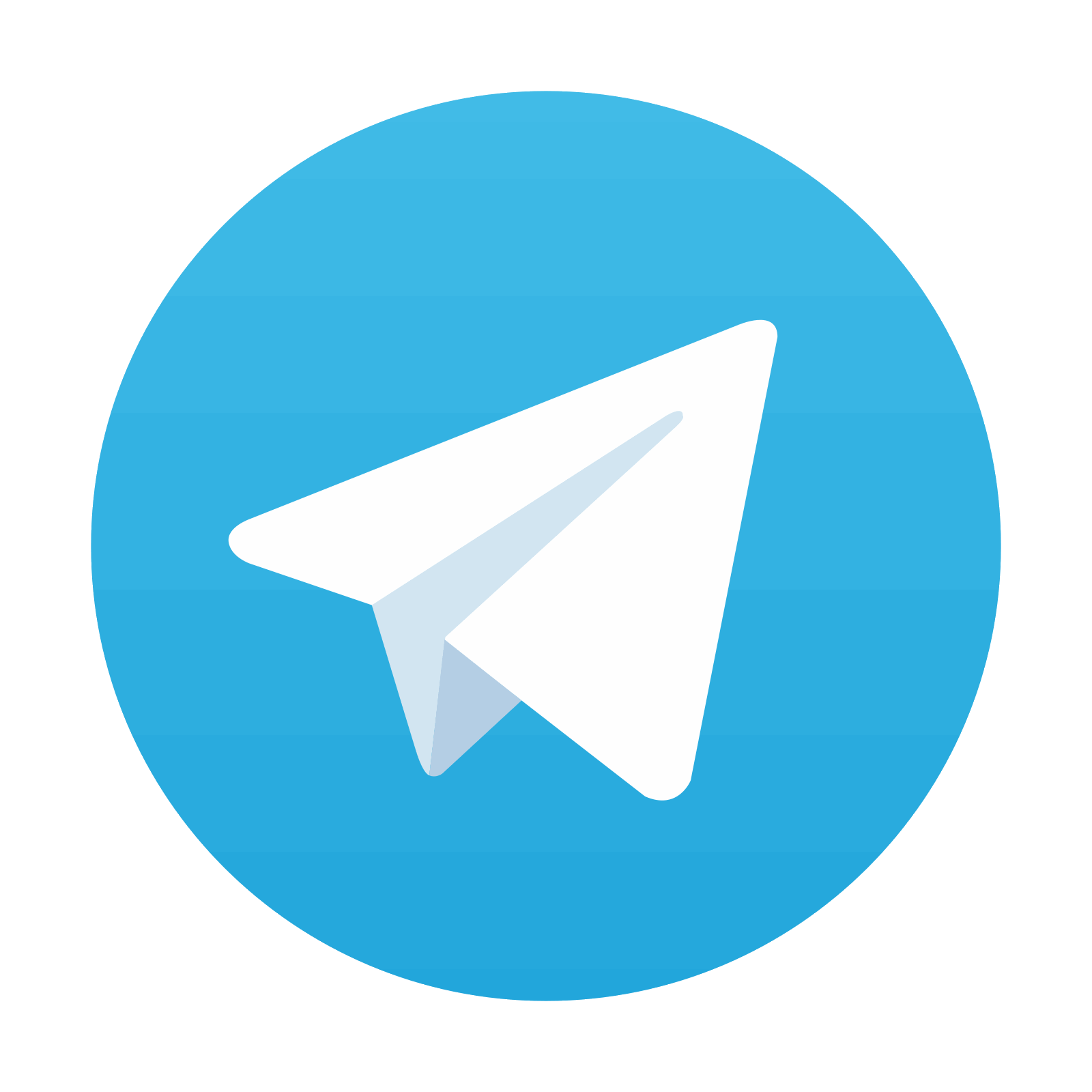
Stay updated, free articles. Join our Telegram channel

Full access? Get Clinical Tree
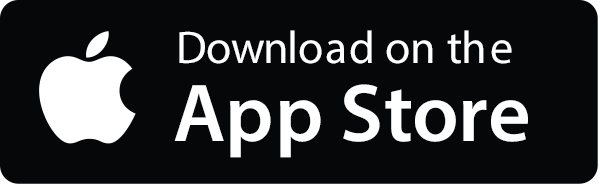

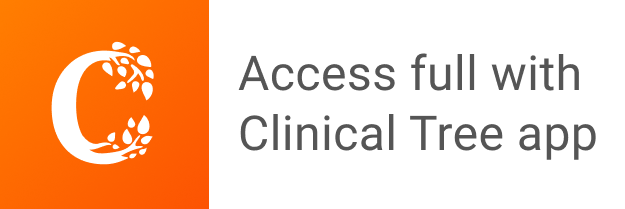