© Springer International Publishing Switzerland 2015
Florian Deisenhammer, Finn Sellebjerg, Charlotte E Teunissen and Hayrettin Tumani (eds.)Cerebrospinal Fluid in Clinical Neurology10.1007/978-3-319-01225-4_1313. Methods for Biomarker Analysis
(1)
Laboratory of Neuroimmunology, IRCCS, National Institute of Neurology “C. Mondino”, Pavia, Italy
(2)
Proteome Biochemistry, San Raffaele Scientific Institute, Milan, Italy
(3)
Division of Neuroscience, Institute of Experimental Neurology, San Raffaele Scientific Institute, Milan, Italy
Abstract
Neuroinflammatory disorders such as multiple sclerosis (MS) encountered a therapeutic revolution in the last 20 years and have raised hope also for neurodegenerative diseases affecting especially the elderly population in western countries. Biomarkers, especially those from the field of neuroimaging, were decisive in accelerating drug development and optimization of treatments. Neuroimaging, however, may be not sufficient in the case of neurodegeneration, where structural alterations accumulate at a very low rate over several years. To capture this slow evolution, biomarkers in the cerebrospinal fluid appear as a very plausible candidate. We will review here, in general terms, the state of the art in the field biomarkers in the CSF with a special focus on the application of “omics” platforms.
13.1 Introduction
The availability of reliable biomarkers is a cornerstone of the up-to-date medicine. However, it may happen that new biomarkers prove weak, whereas old, consistent ones are downgrading. For instance, the analysis of the cerebrospinal fluid (CSF) has considerably lost relevance in the revised diagnostic criteria for multiple sclerosis (Polman et al. 2011). For decennia, oligoclonal IgG bands in the CSF have been one major supporting diagnostic criterion for multiple sclerosis (Freedman et al. 2005), and their down qualification has raised a certain debate (Siritho and Freedman 2009; Galea et al. 2011). This appears paradoxical if one considers that huge investments have been made by the academy but especially by the industry to find new biomarkers in the CSF in the last few years (over 3,000 papers published in the last 5 years). This great effort has yielded an enormous list of candidate biomarkers for neurological disorders, of which only few have reached the bedside. The difficulty to translate this enormous amount of data into useful tools for the clinical neurologist is due to several problems. The first is the relatively poor methodological quality of most studies. The general impression is that in several cases, the isolated finding of the involvement of a certain pathway during disease, lacking mechanistic insight, is reported by authors as a potential biomarker, to pretend a more focused aim to a study with limited ambition. Secondly, pre-analytical errors, which account for the most part of the analytical errors (Plebani et al. 2014) and affect many different aspects (comparable sample selection in multicentric studies, time to processing, sample storage, etc.), are rarely considered. The third most common error is that many studies are designed to answer a clinically irrelevant question (such as distinguishing established AD from healthy donors), rarely addressing the real diagnostic dilemma (e.g., comparing the many different conditions leading to mild cognitive decline). This approach is preliminary and useful for the selection of candidate biomarkers, requiring validation studies on appropriate case series.
On the other hand, the development of technologies able to perform unbiased analysis of nucleic acids, proteins, lipids, and metabolites has provided powerful tools to identify potential biomarkers in the CSF, and these technologies have been applied and are currently applied to this kind of investigations. As for other biological fluids, the enormous amount of data generated by these technologies represents a challenge that needs complex statistical and bioinformatics analyses. The general concept, in fact, is that for most multifactorial diseases, to find a pattern or signature, possibly of different molecular nature, will be more likely than the golden bullet, the perfect biomarker. Thus, new approaches such as neural networks and machine learning are increasingly used for data mining.
This field of investigation on the CSF topic has already changed the way we approach cognitive decline and the differential diagnosis of Alzheimer’s disease (AD). In this disease, lumbar puncture was rarely performed (and mostly by mistake), while today, CSF biomarkers, such as low beta-amyloid 1–42, elevated protein tau, and phosphorylated protein tau, are routinely used also in prodromal AD (Ritchie et al. 2014) to help diagnosis or to confirm diagnosis in later stages. Despite the final validation of these CSF biomarkers, especially in combination with other markers such as neuroimaging pictures and psychological tests (Filippi et al. 2013), is still missing (Ferreira et al. 2014), they are cost-effective in identifying AD among MCI patients (Nazco et al. 2014). CSF biomarkers in AD are also aimed at capturing phenomena that associate with underlying pathogenetic mechanisms, such as neuroinflammation (Alcolea et al. 2014) and structural damage (Fortea et al. 2014), and, possibly, at monitoring disease progression in clinical trials (Dumurgier et al. 2014). CSF biomarkers have been linked to cognitive performance also during aging (Li et al. 2014), thus becoming, in perspective, a monitoring that will be proposed to the general elderly population to predict cognitive decline. We can only expect that extensive evaluation of currently used biomarkers in MCI/AD patients over the next years, with accumulation of further experience, and possibly the identification of new biomarkers will most likely yield more accurate diagnostic and prognostic information in the future (Lista et al. 2014).
While CSF beta-amyloid 1–42, protein tau, and phosphorylated protein tau are routinely tested in most neurology departments for the differential diagnosis between AD and other forms of dementia, the field is moving forward also in other neurodegenerative disorders. In Parkinson’s disease (PD), for example, the evaluation of AD biomarkers appears to be useful to predict the occurrence of associated dementia (Alves et al. 2014; Siderowf and Logroscino 2014), rather than to discriminate PD with cognitive onset from AD (Vranová et al. 2014). In PD, investigations on several CSF biomarkers, including synuclein, are ongoing but are far from being conclusive or clinically validated (Parnetti et al. 2014; Malek et al. 2014).
In several other neurological disorders such as infections (Fraisier et al. 2014; Cassol et al. 2014), spinal cord injury (Pouw et al. 2014), amyotrophic lateral sclerosis (Tortelli et al. 2014), and stroke (Hjalmarsson et al. 2014), investigations are actually in progress, revealing the enormous interest in finding new ways to stratify neurological disorders, especially in view of forthcoming clinical trials. A very interesting field, characterized by a reappreciation of possible organic causes, is psychiatry. As a result of such reappreciation, CSF biomarkers are, of course, matter of intense investigation. While a neuroimmunological pathophysiology is evident in the multifaceted psychiatric manifestations of limbic encephalitis, characterized by the presence in the CSF of specific antibodies, such as anti-NMDA-R, anti-VGKC, anti-glycine, etc., in mood disorders, organic causes are less evident. Depression, bipolar disorder, and other major psychoses have been linked to altered innate immunity (Bergink et al. 2014). Major depression seems currently the most promising disease to be characterized by CSF abnormalities: protein profiles (Woods et al. 2014), choroidal gene expression (Turner et al. 2014), neurofilaments (Jakobsson et al. 2014), and cytokines (Kern et al. 2014) are all examples of potential CSF biomarkers that have been investigated and proposed as biomarkers.
The development of more potent technological platforms, the identifications in relatively recent years of new entities in molecular and cellular biology, such as miRNA and microvesicles, both promising categories of biomarkers, increase the expectation that in the near future, clinicians will have refined tools to diagnose neurological and psychiatric disorders, foresee disease evolution, plan therapy, and monitor outcomes. But, as stated above, the field is invaded by relatively low-quality literature, and the issue that only a very limited number of apparently promising biomarkers undergo proper clinical validation still remains. Is this shortcoming unavoidable? Not necessarily. Over a decennium ago, this same concern resulted in the development, during a consensus meeting, of a checklist and a generic flow diagram for studies of diagnostic accuracy called the STARD initiative (Bossuyt et al. 2003). Over the years, this checklist has been published in numerous medicine journals and has been implemented by many editors. Nevertheless, a minority of biomarker studies, even among those cited in the present chapter, do adhere to these guidelines. Implementation of these guidelines is mandatory, in our opinion, to strengthen studies and focus investments on solid results. Further, clinical validation of biomarkers needs large cohorts that are difficult to put together in single centers, not mentioning that the challenge of the slight differences in terms of clinical classification, processing, and storage of samples constitutes a necessary test for biomarkers that aim at being employed in daily clinical practice. To this respect, it is noteworthy to mention the publication of a consensus protocol for the collection and storage of CSF aimed at minimizing preclinical errors, standardize procedures, and allowing, therefore, biomarker validation studies on large number of relatively homogeneous samples (Tumani et al. 2009; Teunissen et al. 2009). In the following paragraphs, we will discuss the main findings and the major technical difficulties in the CSF biomarkers research that exploits various approaches such as proteomic analysis, gene expression studies, and characterization of miRNA.
13.2 Proteomics Analysis of CSF
Different methodological approaches can be used for the analysis of the CSF’s protein content. The most frequently used techniques are addressed to perform the total differential protein expression analysis comparing two or more experimental conditions or group of samples. Quantitative proteomics measures the relative or absolute protein abundance or specific post-translation modifications (van Gool and Hendrickson 2012; Craft et al. 2013) and is nowadays principally based on mass spectrometry approaches, even though classical gel-based analyses (namely, 2-dimensional electrophoresis and 2-dimensional differential fluorescence gel electrophoresis) are still largely used. Gel-based methodologies are limited in the number and in the specific type of protein that can be resolved, while the tandem mass spectrometry approaches coupled with liquid chromatography (LC-MS/MS) are able to resolve without limitation thousands of proteins (Yuan and Desiderio 2005a; Huang et al. 2007b). The relative quantitation of protein expression is typically reported as a fold change and can be obtained by label-based or label-free methods, while absolute quantitation can be determined by addition in the samples of internal stable-isotopic standards (see Craft et al. (2013) for a detailed methodological review).
Using these approaches, CSF studies have been conducted in several neurological diseases including neurodegenerative diseases, in particular in Alzheimer (see Liu et al. (2014) for review) (Lista et al. 2014), in psychosis (Huang et al. 2007a), multiple sclerosis (Stoop et al. 2010b; Lourenco et al. 2011), amyotrophic lateral sclerosis (Conti et al. 2008), brain injury (Conti et al. 2004), peripheral neuropathies (Conti et al. 2005), etc. (see Craft et al. (2013) for a review). However, the biological implications and the relevance of the proposed biomarker of many of the published studies remain to be clearly established.
In addition to the analysis of differential protein expression level, some interesting results have been obtained, investigating specific post-translation modifications promoted by the pathological conditions that can be used as putative source of biomarker discovery. In fact, in several neurological diseases characterized by the presence of neuroinflammation or oxidative stress conditions, like in the case of some neurodegenerative disease, oxidative modifications of the CSF protein have been investigated as acidification of the protein isoelectric point (Olivieri et al. 2011) or as increase in protein carbonylation (Olivieri et al. 2011; Iannaccone et al. 2013), protein nitration (Castegna et al. 2003), protein thiol group modifications (S-cysteinylation, S-glutathionylation, S-nitrosylation) (Nakamura et al. 2013; Poulsen et al. 2014), and protein deamidation (Barbariga et al. 2014) the latest reflecting accelerated protein aging under pathological conditions. Furthermore, the protein phosphorylation, the most common post-translational modification for the reversible regulation of protein function, has also been investigated (Yuan and Desiderio 2003).
An alternative proteomics approach for CSF investigation might be the serological proteome analysis (SERPA) (De Monte et al. 2008; Privitera et al. 2013) that exploits the presence of autoantibodies in some neurological disease like multiple sclerosis (Menon et al. 2011). In the SERPA approach, the autoantibodies present in the CSF of the patients are used to screen the proteome of the patient resolved by 2-dimensional electrophoresis in order to identify putative autoantigen/s. The presence and the titer of specific autoantibodies might be considered itself a putative biomarker.
Regardless of the type of the methodological approaches used, the biological and chemical features of the CSF made necessary the introduction in the experimental pipeline of sample preprocessing steps to improve the quality of the results. For example, for the gel-based approaches, the high salt concentration (>150 mM) and the relative low protein concentration (0.2–0.7 mg/ml) require the introduction of desalting and protein concentration steps that can be performed by ultrafiltration, dialysis, and protein precipitation (Yuan and Desiderio 2005a). Another technical problem for CSF proteins is the wide dynamic range (several orders of magnitude) of some specific protein concentration that causes difficulties in the detection of low-abundant proteins. Therefore, very abundant proteins like albumin, immunoglobulin, transthyretin, etc. can be eliminated by affinity chromatography removal or by CSF sample pre-fractionation (Yuan and Desiderio 2005a). Protein pre-fractionation can be achieved by standard biochemical methods that exploit different biochemical features of the protein like hydrophobicity, size, charge, etc. (Zhang et al. 2005; Yuan and Desiderio 2005a). The most popular techniques used for CSF protein fractionation are the reversed-phase solid-phase extraction (Yuan and Desiderio 2005b) and liquid- or semi-solid-phase isoelectric focusing that allow protein separation on a solid pH gradient and a protein recovery in liquid phase. These kinds of approaches allow the separation and concentration of low-abundant proteins, but generate for each CSF sample a large number of fractions that must be analyzed.
Some other warning rises for specific post-translation modification analysis like in the case of oxidative modifications; in fact, the risk of artifactual modification does exist due to the ex vivo protein oxidation fostered by the experimental conditions or by sample storage conditions (Olivieri et al. 2011; Poulsen et al. 2014). In our experience, we avoid experimental oxidation by storing the sample and performing the experiments under nitrogen-conditioned atmosphere (Olivieri et al. 2011; Barbariga et al. 2014). In the case of protein deamidation, for example, it is mandatory to set up a different protocol for tryptic digestion necessary for mass spectrometry analysis because the standard conditions used (ammonium bicarbonate buffer at pH 8.5, at 37 °C) are the ideal conditions to promote very strong in vitro deamidation (Barbariga et al. 2014). Thus, for every kind of analysis, methods for simple, robust, and reproducible inhibition of artificial modifications should be developed.
Other general critical points that must be taken into consideration are the risk of CSF contamination by blood proteins that may occur during sample collection, the interindividual variation of the specific protein concentration (Stoop et al. 2010a; Perrin et al. 2013), and the age-related changes in protein relative abundance (Zhang et al. 2005) that might dramatically affect the outcome of the analyses.
In conclusion, the proteomics approaches may offer very interesting input for CSF biomarker discovery; however, in order to transform this vast experimental effort in meaningful information, there is still a need for standardization of methods and experimental design.
13.3 mRNA in the CSF
Gene expression studies are possible in the CSF only if examined mRNA is cell associated or if it is protected from degradation, i.e., it is packaged in extracellular membrane vesicles (EMVs). In the last years, EMVs and their content have gained attention as potential biomarkers also in clinical neurosciences (Borgiani et al. 2012; Rao et al. 2013). There are two main populations of EMVs, exosomes (50–80 nm in diameter, of intracellular origin) and shed vesicles or ectosomes (200–2,000 nm in diameter, budding from plasma membrane). For biomarker studies, this difference is not only relevant for biological reasons, but because, despite a certain loss, exosomes survive freeze and thawing, shed vesicles/ectosomes don’t. In practical terms, archival CSF samples, stored frozen, are inadequate for shed vesicles/ectosomes analysis. Further, processing and storage of CSF determine what kind of molecules, formerly packaged into EMVs, are detectable in cell-free CSF. Nevertheless, the content, especially RNA species of EMVs, is considered a very promising biomarker (Ratajczak et al. 2006; Valadi et al. 2007; Deregibus et al. 2007; Skog et al. 2008; Hong et al. 2009; Choi et al. 2013a, b). To date, around 50 high-throughput extracellular vesicles (EVs) transcriptomic studies based on microarray and next-generation sequencing have been published (Choi et al. 2013a). However, these studies are controversial. It has been described in several different systems that mRNAs and miRNAs are packaged into EVs. Several studies have reported that specific mRNA is enriched into EVs by means of sorting a specific mRNA present in the cell cytoplasm (Valadi et al. 2007; Deregibus et al. 2007; Palanisamy et al. 2010). Also, Hong and Skog have demonstrated that the mRNA content of EVs reflects the cellular mRNA of the cell of origin. In contrast, they demonstrated that EVs and their original cells have a similar number of mRNA, and some vesicular RNAs were relatively enriched (Skog et al. 2008; Hong et al. 2009; Choi et al. 2013a).
Discordant results emerging among these transcriptomic studies have been affected by non-vesicular RNAs, degraded RNAs, or bovine serum-derived EVs contamination. To avoid this contamination, EVs purification requires critical steps such as RNase digestion and differential centrifugation EVs isolation using swinging bucket rotor (Choi et al. 2013a; Cvjetkovic et al. 2014). Thus, EVs isolation protocols affect the yield and purity of EVs RNA.
We have previously described that microvesicles (MVs) of myeloid origin in the cerebrospinal fluid (CSF) are a marker of microglia/macrophage activation (Verderio et al. 2012). Starting with the hypothesis that MVs content reflects processes ongoing in pathologically relevant cell types and may be a useful biomarker in several pathological processes, we performed in vitro and in vivo mRNA studies in MVs from classically M1- and alternatively M2-activated macrophages. Because the number of myeloid MVs in the CSF does not allow collecting enough material for transcriptome analysis, we performed mRNA studies in murine macrophages.
In accordance to previous reports (Valadi et al. 2007; Skog et al. 2008; Pegtel et al. 2011; Chen et al. 2012), our microarray data indicate that MVs content is not the mere sampling of mRNAs present in the cell cytoplasm: a specific set of transcripts are enriched in MVs as compared to parental cells. Furthermore, there is certain number of transcripts that are packaged into MVs regardless of activation state and type. Both M1 and M2 activation, however, induce the expression and sorting into MVs of specific transcripts. We validated by real-time RT-PCR typical M1 and M2 markers, identified as enriched in MVs by the microarray analysis. To perform RT-PCR assays, we had to overcome the lack of a validated housekeeping gene for MVs. Using bioinformatic tools and assessing stability and expression levels, we identified PSAP, a known marker of extracellular membrane vesicles (Shan 2011), as a reliable housekeeping gene for myeloid MVs (Garzetti et al. 2013). All these studies have demonstrated that mRNAs associated to MVs reflect the functional phenotype of parental cells. Thus, for neurological disorders characterized by microglial activation, the idea will be to exploit these data to identify biomarkers able to measure microglia activation and activation type.
13.4 miRNAs in the CSF
Among noncoding RNAs, miRNAs are short RNA molecules that, through regulation of translation of messenger RNAs into proteins, control all cellular processes, including cell cycle, differentiation, functional phenotype, etc. (Cech and Steitz 2014). The discovery that miRNAs can be detected in biological fluids has made the obvious candidates as biomarkers. In biological fluids, miRNAs are more stable than expected, because they are complexed with proteins or encapsulated in extracellular membrane vesicles (EMVs). Their detection, upon purification of total RNA or using techniques specific for short RNA species, is performed using RT-PCR, deep sequencing, or microarrays. Advantages and pitfalls of these approaches are not the topic of this chapter. The experience accumulated in the field of oncology, which has pioneered the use of miRNAs as biomarkers, can be useful to evaluate promises and pitfalls of this research topic (Jarry et al. 2014).
Most of the studies in the CSF deal with the search for miRNAs able to identify AD patients. The design of all reports is of little clinical relevance, comparing already diagnosed, frankly demented AD patients either with healthy donors or with nondemented patients affected by other neurological disorders. Mild cognitive decline and different types of dementia, whose differential diagnosis represents the real challenge for the clinical neurologist, are never investigated, as also a possible prognostic value. Reproducibility of results is also disappointing. Out of 60 miRNAs identified in one small study (10 demented AD patients vs. 10 nondemented controls) (Cogswell et al. 2008), none was shared by the others, although some miRNAs were found significantly altered at least in two studies. The only miRNA found altered in three reports, hsa-miR-146a, was detected as decreased in two studies (Kiko et al. 2013; Müller et al. 2014) and elevated in another (Alexandrov et al. 2012). The only study trying to validate a single miRNA in two independent cohorts identified a decreased miRNA that has not been identified in the other investigations (Frigerio et al. 2013). Patient selection and stratification, preclinical errors, technical issues, all may have contributed to contradictory results. Despite short RNAs are the most abundant RNA species in the CSF (Pogue et al. 2014), still their purification and analysis using different techniques may lead to incoherent results. Most of the studies use commercial kits designed for small RNA species for extraction and RT-PCR, either candidate miRNAs or cards encompassing assays for most known miRNAs. Quantification using microarrays for miRNA is certainly less reliable and may explain some contradiction in reports. The most robust approach, deep sequencing, is rarely used also because of relatively high costs as compared to RT-PCR and microarrays, although costs should be calculated also taking in account reliability of results.
The situation, both for patient selection and for technical hurdles, is slightly better in the only other CNS pathology where more than one report is available, namely, brain tumors. Three reports agree that hsa-miR21 is elevated in the CSF of patients with glioblastoma in comparison with brain metastasis (Baraniskin et al. 2011b; Teplyuk et al. 2012; Akers et al. 2013); however, the same miRNA is elevated also in the main CNS brain tumor that goes in differential diagnosis with glioblastoma, primitive B-cell lymphoma (Baraniskin et al. 2011a).
Pioneering, preliminary studies are available in other neurological disorders. In multiple sclerosis (MS), Hagikia and colleagues preliminarily identified miRNAs able to differentiate MS patients from those affected by other neurological disorders and MS patients with different disease courses (Haghikia et al. 2012). After the identification of miRNA binding TDP-43, a gene involved in familial amyotrophic lateral sclerosis (ALS), Freischmidt and coworkers measured five of them as being dysregulated also in the CSF of sporadic ALS (Freischmidt et al. 2013). A pilot study has identified that a certain number of miRNAs have been found in the CSF associated to HIV infection and to HIV encephalitis (Pacifici et al. 2013). Finally, miRNAs specific to psychoses have been identified in the CSF of a very small cohort of psychiatric patients in comparison with healthy donors using microarrays (Gallego et al. 2012).
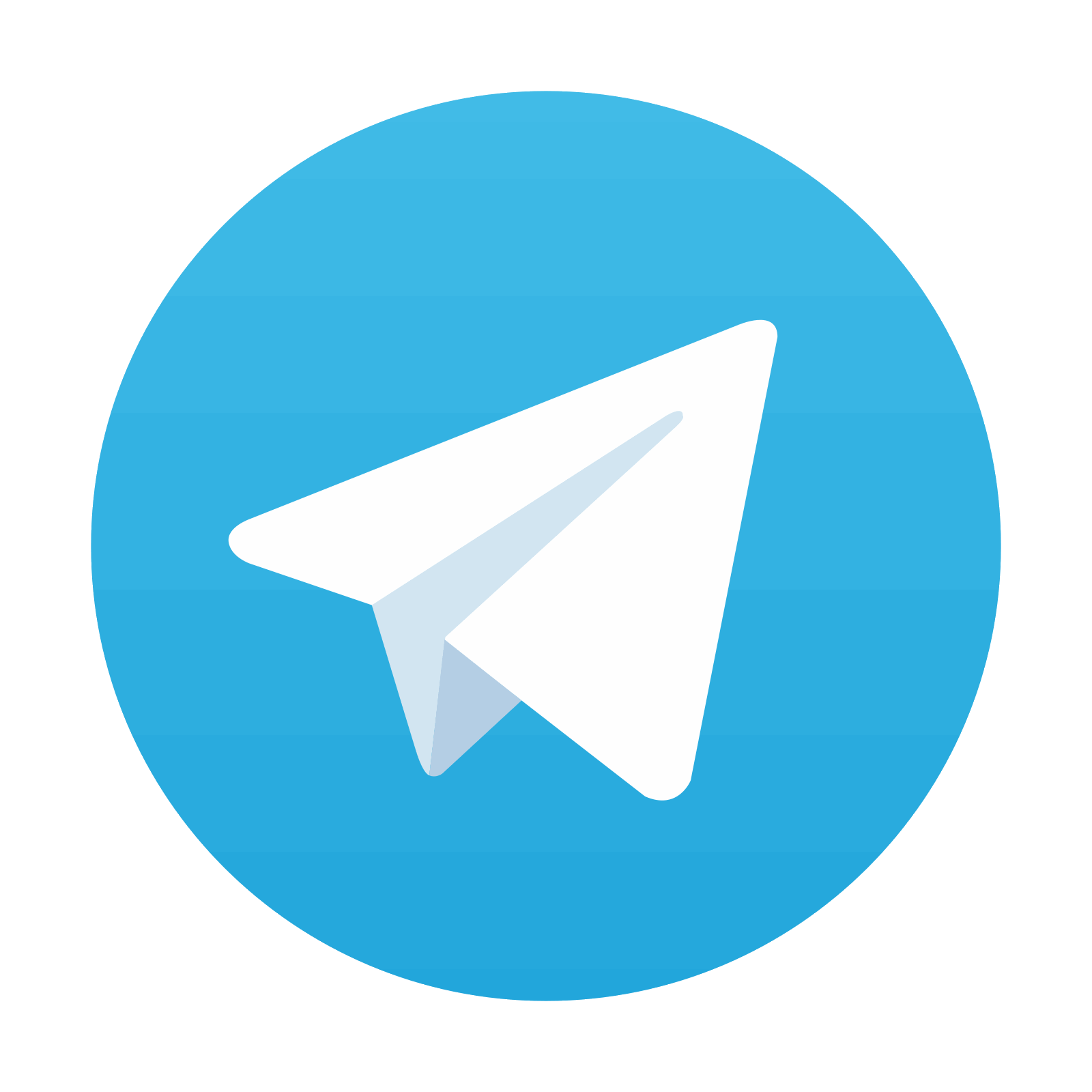
Stay updated, free articles. Join our Telegram channel

Full access? Get Clinical Tree
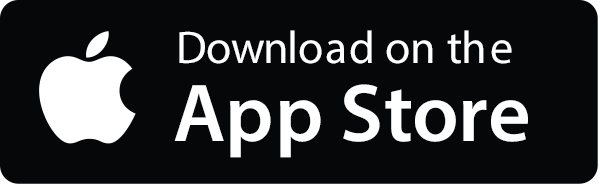
