Fig. 6.1
Genome, Transcriptome, Proteome, Metabolome and Lipidome (from http://en.wikipedia.org/wiki/Lipidomics). Transcriptome refers to the collection of all genes expressed in a given tissue at a given time. Similarly, a proteome is a collection of all proteins produced by an organism at a given time. Finally, proteins exert their action in specific biological pathways (e.g. as enzymes or receptors). A metabolome is a collection of thousands of biological molecules used (as substrate) and created (as product) by such pathways. The main classes of these molecules are amino acids, sugars and lipids, the latter constituting a lipidome
6.1 Transcriptomics
Although all somatic cells contain the same genes, different cells express only a subset of these genes at any given time. As discussed in the previous chapter, there are several genetic and epigenetic mechanisms that regulate gene expression, thereby creating a vast variability across individuals in the level of a particular mRNA in a given tissue. This variability can be captured—at a genome-wide level—using RNA microarrays or next-generation sequencing of RNA (RNA-Seq1). But given the limited access to brain tissue, how can we use transcriptomics in population-based studies of the human brain? With certain limitations, there are at least four possibilities.
First, we can use transcriptomics when evaluating the functional consequences of a genetic variation on gene expression in non-brain tissues. Is a SNP discovered, for example, in a GWAS of a particular brain phenotype also associated with variations in gene expression in the same cohort? As we have learned in Chap. 5, this would be more likely if the SNP is non-synonymous and/or located in the promoter region of the gene in question.2 Therefore, it would be helpful to know whether individuals who differ in this particular genotype also differ in the level of gene expression. This can be checked, provided that investigators collected and stored mononuclear leucocytes (lymphocytes and monocytes) and calculated the number of different blood cells per unit of whole blood; 1 ml of blood should contain ~4 × 106 leucocytes (white blood cells) of which ~1 × 106 are mononuclear leucocytes. If yes, one can measure the amount of RNA (of the gene of interest) in monocytes and normalize this value by the number of monocytes per unit of volume. Thus, if we find a difference in the level of gene expression as a function of a SNP, we can infer that a genetic variation present at this genomic location (or one that is in linkage disequilibrium) has a potential to influence the transcription of DNA coded there. Of course, we do not know whether this is the case in other tissues, including the brain.
We can use a similar approach in other situations. For example, let us assume that we have uncovered an association between a known functional polymorphism—say, resulting in val66met in the BDNF protein (Text Box 4.4)—and a particular brain phenotype in one group of individuals but not in another. While there might be many possible explanations for such a group-by-genotype interaction, one of them could be that BDNF is less expressed in the group in which we did observed the BDNF effect, thus making the genotype less relevant. Such a scenario may be worth exploring, especially if one suspects environment-induced changes in DNA methylation or histone modification (see Chap. 5).
Second, a number of efforts are underway to construct transcriptomes in the human brain. For example, Kang et al. (2011) reported a number of global sex- and age-related differences in gene expressions assessed with RNA microarrays across 1,340 tissue samples, which were obtained from 16+ brain regions in 57 individuals, from embryos and foetuses, through children and adolescents to adults. The Allen Institute for Brain Science has released the first version of the Human Brain Atlas, containing relative values of gene expression for over a thousand distinct brain regions assessed with RNA microarray in a mere three individuals. These values are plotted in an atlas aligned with the MNI152 target brain, thus allowing 3D localization of each sample in the standardized sterotaxic space used in the majority of structural and functional imaging studies (Fig. 6.2). The two examples illustrate the enormity of constructing a representative transcriptome of the human brain. On the one hand (Kang et al. 2011), we have transcriptomic data obtained in a handful of brain regions in a number of individuals who differ widely in age (from 4 post-conception weeks to 60 years). On the other hand (The Allen Human Brain Atlas), we have transcriptomic data in over 1,000 brain locations mapped in sterotaxic space in three adult brains. The future will show whether such efforts can be expanded to provide a robust (probabilistic) representation of gene expression in the human brain during key developmental periods.
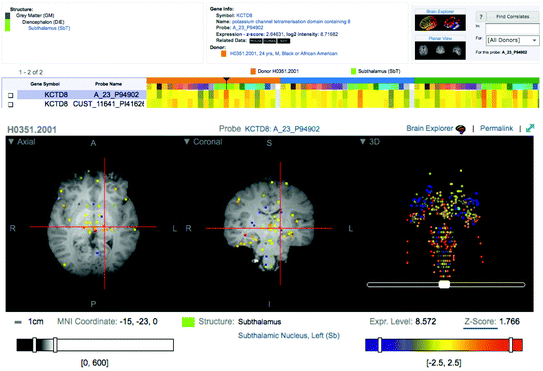
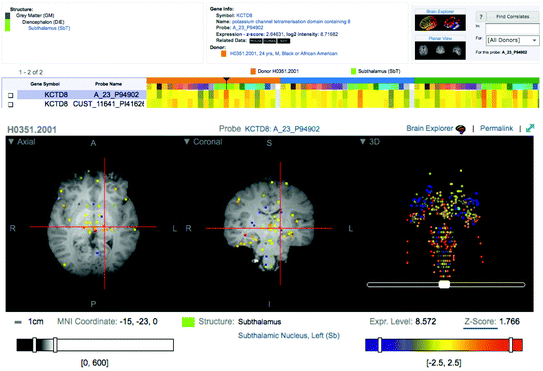
Fig. 6.2
The Allen Human Brain Atlas (KCTD8 example)
Third, we can use transcriptomes deposited in existing gene-expression databases to evaluate co-expression networks in the relevant tissues (e.g. Gillis et al. 2010; Gillis and Pavlides 2011). This type of analysis relies on evaluating profiles of co-expression between the target gene—revealed, for example, by a GWAS—and all other genes included in RNA microarrays collected across hundreds of experiments carried out in different laboratories. In this way, one can reveal biological pathways relevant for the trait in question and, in turn, explore the possible associations between the particular phenotype and any DNA variations in genes co-expressed with the target gene. This approach is also helpful in situations when the gene revealed by a GWAS does not have a well-established function; identifying the gene’s co-expression network provides additional information about the possible biological pathways relevant for a given genotype–phenotype relationship (Text Box 6.1.).
Text Box 6.1. Co-expression networks
We found that genetic variations in the KCTD8 locus are associated with the total brain volume in adolescent girls (Paus et al. 2012). Given that the function of this gene is largely unknown, we used the co-expression approach to identify biological pathways in which this gene might play a role. By interrogating over 600 microarrays of gene expression in foetal-mice tissue, we found that genes co-expressed with KCTD8 include those playing a role in potassium ion transport (KCNC3), development (e.g. NRG3, NRCAM) and neurotransmission (e.g. Slc6a13, Slc7a10, Slc6a3). We also analysed a small set of microarrays obtained in four human foetal brains (Johnson et al. 2009) and found that genes co-expressed with KCTD8 include those linked to brain-related gene ontologies, such as “neuron fate commitment,” “neural crest cell migration” and “neurotransmitter metabolic process” (Paus et al. 2012; http://www.chibi.ubc.ca/Paus). These two examples illustrate how co-expression analyses carried out with RNA data acquired from relevant tissues may facilitate the interpretation of novel findings obtained in population-based studies.
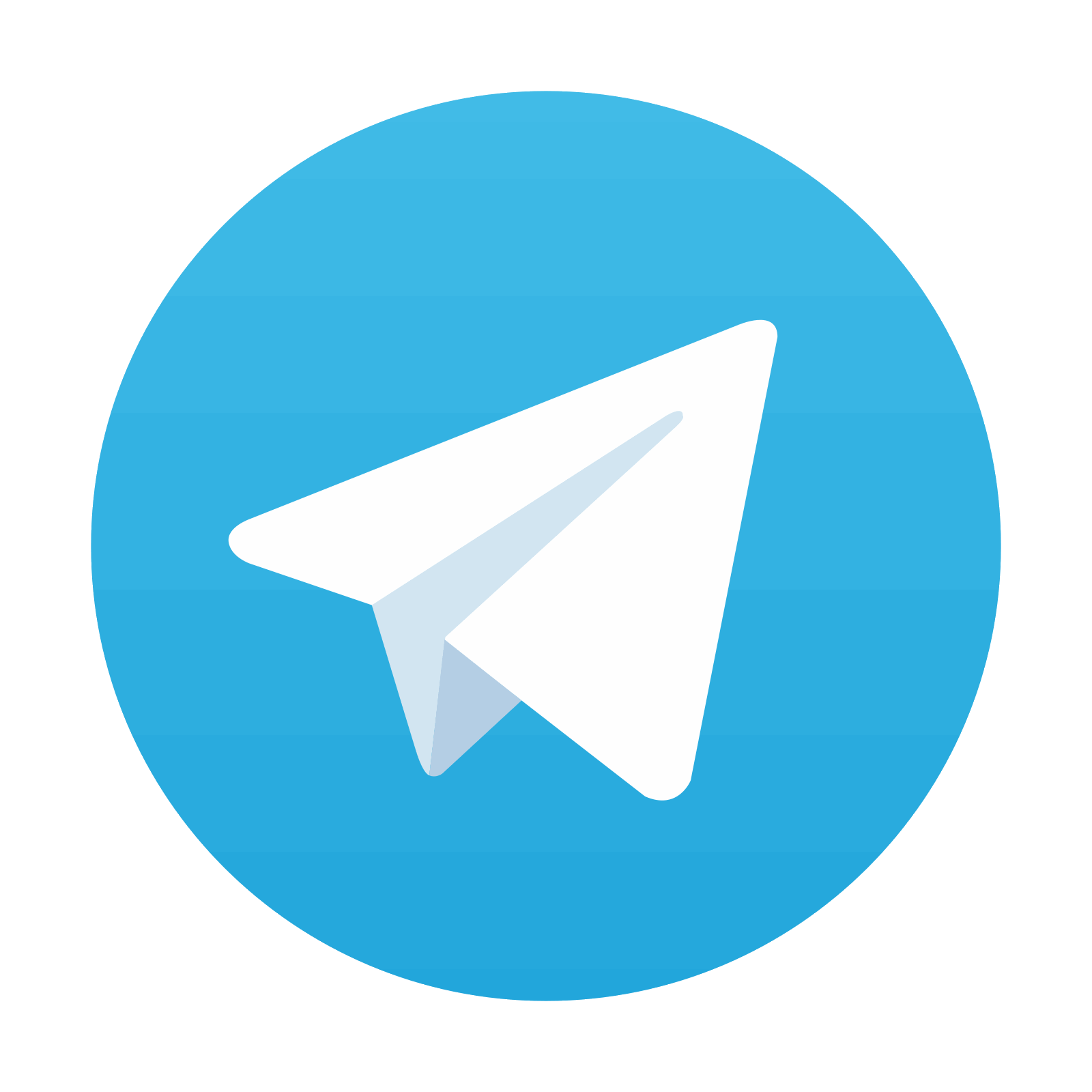
Stay updated, free articles. Join our Telegram channel

Full access? Get Clinical Tree
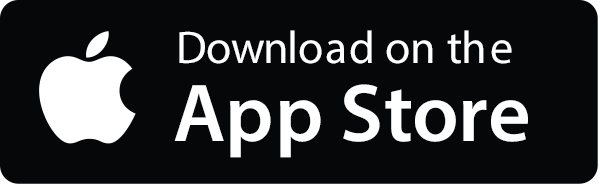
