Fig. 7.1
Shen et al. showed how infants and toddlers who were subsequently diagnosed with ASD had greater total cerebral volumes (after removing non brain tissue) and increased rates of growth, as compared to children with other developmental delays or with typical development. (Reproduced with permission from Brain. Shen et al. 2013)
Recently, a systematic review and re-analysis of head circumference and volumetric data suggests that biases in the selection of control populations may be falsely inflating differences in patients with ASD. The authors propose that had previous studies selected control samples from local communities, as well as accounted for known head circumference and brain volume confounders such as weight, ethnicity and social economic status, results would have been much less pronounced regarding early brain overgrowth in this disorder (Raznahan et al. 2013).
That being said, a recent publication sought to determine whether early neuroimaging biomarkers in infants at high risk for ASD might be predictive of a subsequent diagnosis. Shen et al. followed 55 infants (33 of whom were considered high risk given that they had a sibling with ASD and 22 of whom were considered low risk) with serial MRI scans between 6 and 24 months of age. They found that infants who were subsequently diagnosed with ASD had significantly greater extra axial fluid volumes (cerebrospinal fluid in the subarachnoid spaces), greater total brain volume (see Fig. 7.1), larger head circumferences, and larger lateral ventricle volumes. A ratio of extra-axial fluid volume to brain volume at 12–15 months of age that was greater than 0.14 was predictive of a subsequent diagnosis of ASD with 78 % sensitivity and 79 % specificity. These findings have yet to be replicated, but the authors suggest that structural MRI monitoring in high risks infants merits further investigation (Shen et al. 2013).
7.2 Voxel Based Morphometry
As the resolution of MRI imaging technology continued to improve, computerized data-analysis software was also developed, which permitted more precise quantification of imaging data. Voxel based morphometry (VBM) is an approach which measures the specific grey matter content of each volumetric pixel (voxel), thereby permitting automated delineation of grey and white matter. Simultaneously, images can be ‘warped’ onto a common template, in order to more precisely enumerate the differences between cases and control data sets (Hernandez-Garcia and Buschkuehl 2012).
This technology has allowed for a more exact quantification of whole brain and regional volumes, removing the need for manual mapping. In addition, specific subregions can be investigated in greater detail, or the whole brain can be surveyed, in terms of grey matter density by voxel cluster. Alternatively, strategies to correlate structural MRI data from VBM with social and cognitive function have also been employed (Ecker et al. 2012).
There is significant heterogeneity in the literature regarding findings. Differences in image filtering techniques, corrections applied for motion artifacts, statistical thresholds given multiple comparisons, selection of brain templates, as well as demographic and clinical differences in patient samples have complicated the interpretation of study results. Several meta-analyses (Duerden et al. 2012; Nickl-Jockschat et al. 2012; Via et al. 2011; Yu et al. 2011; Cauda et al. 2011) have attempted to synthesize findings, but even results across meta-analyses are variable (see Table 7.1). Two meta-analytic approaches in VBM include the activation likelihood estimate (ALE) (Laird et al. 2005) and the signed-differential mapping (SDM) methods. Both ALE and SDM combine data from published MRI coordinates to generate probability maps identifying grey and white matter clusters where findings converge across studies.
Table 7.1
Areas containing grey and white matter clusters of increased (+) or decreased (−) volume/ density across voxel based morphometry meta-analyses in patients with ASD vs. controls. (L/R: left or right side, ALE: activation likelihood estimate, SDM: signed-differential mapping, ASP: Asperger’s syndrome). Note: − R/L indicates an area of decreased volume/density of grey matter in this region on both the left and right side. − R/L and + R/L indicates subregions within the specified region that are both increased and decreased on both sides
(Duerden et al. 2012) | (Nickl-Jockschat et al. 2012) | (Cauda et al. 2011) | (Via et al. 2011) | (Yu et al. 2011) | |||
---|---|---|---|---|---|---|---|
Method | ALE | ALE | ALE | SDM | ALE | ||
Groups | Children | Adults | n/a | n/a | n/a | ASD | ASP |
Frontal Lobe | |||||||
Precentral gyrus | − R/L | − L | − L | ||||
Medial Frontal gyrus | − R/L | − R | − L | + R | − R | ||
Superior frontal gyrus | − R + R | − R/L + R | − L − R | ||||
Middle frontal gyrus | − R/L | + R/L | + L | + R | |||
Inferior frontal gyrus | − L | − L | + L | ||||
Temporal Lobe | |||||||
Superior temporal gyrus | − R/L + R/L | − R | |||||
Middle temporal gyrus | − R/L + R/L | − R + R | − R | + R/L − R | + L | + R | |
Inferior temporal gyrus | − R + L | ||||||
Fusiform gyrus | − R/L + L | + R | − L + R | + R | |||
Parietal Lobe | |||||||
Superior Parietal Lobule | − L | − R | |||||
Inferior Parietal Lobule | − L + R | − R/L | − R | + R | |||
Precuneus | − R/L + R | − R | + R | + R − R | − R/L | + R | − R |
Primary Sensory Cortex | − L | − R | + R | ||||
Occipital Lobe | |||||||
Temporal Occipital Regions | + R/L | ||||||
Middle Occipital Region | − L+ R | − R | − L | ||||
Inferior Occipital Region | + R | ||||||
Cuneus | − R/L | ||||||
Lingual Gyrus | + L | + R | + L | + L | |||
Insular cortex | |||||||
Any location | − R/L + R | + R − R | + R | ||||
Cerebellum | |||||||
Any location | − R/L + R | − R/L + R/L | + R/L | − R/L + R | − R | ||
Cerebellar Vermis | − R/L | + R | |||||
Cerebellar Tonsil | − R/L | ||||||
Subcortical Structures | |||||||
Putamen | − L + L | − R/L | − R/L | − R | |||
Caudate | + R | + R head − R tail | + R/L | ||||
Thalamus | − R/L | ||||||
Limbic System and Associated Structures | |||||||
Hippocampus | − L | − R | − R | − R/L | |||
Hippocampal Gyrus | − R/L | + L | + R | + R | |||
Uncus | − R | + R | |||||
Amygdala | − R | − L | − R | − R | − R/L | ||
Anterior Cingulate | − R + R | − R + L | + R | + R/L | |||
Posterior Cingulate | + R | − L | + R |
Areas that seem to emerge most consistently in these meta-analyses include volume loss in the putamen (Duerden et al. 2012; Nickl-Jockschat et al. 2012; Yu et al. 2011), volume gains in the caudate (Duerden et al. 2012; Yu et al. 2011) and volume loss in the hippocampus and amygdala (Nickl-Jockschat et al. 2012; Via et al. 2011; Yu et al. 2011). Significant differences also emerge in the prefrontal cortex (see Table 7.1). These findings must be interpreted with the consideration that structural brain changes in ASD may in part be due to comorbid psychiatric symptoms (Juranek et al. 2006), as well as concomitant treatment with psychotropic medications (Chakos et al. 1994). These and other confounding factors cannot be controlled for across meta-analyses. The functional implications of these areas will be discussed in more detail in Sect. 7.6 regarding regions of interest in ASD.
In addition to identifying regions of interests, the meta-analytic approaches described above have helped to elucidate age related differences in this condition. Findings span many heterogeneous regions, but similar to volumetric data, differences seem more pronounced and more numerous in the childhood/adolescent samples compared to adult samples (Duerden et al. 2012; Nickl-Jockschat et al. 2012).
Data from VBM analyses have also helped to inform the decision to condense previous diagnostic categories (i.e. autistic disorder, PDD-NOS, and Asperger’s syndrome) into a common autism spectrum disorder in the DSM-5 , given the absence of discrete biologically based disease categories (Via et al. 2011).
7.3 Cortical Thickness Mapping
In cortical thickness mapping, the technology from voxel based morphometry is applied specifically to the cerebral cortex , to permit more precise anatomic analysis of this area. Similar to whole brain VBM, in cortical thickness analysis, a computer is programmed to extract the cortical grey matter from underlying structures, and this data is transposed onto a common surface template. In this way, the cortical grey matter can be further quantified in terms of cortical thickness, and cortical surface area. These measurements are of interest in ASD, as they are thought to represent separate embryological processes, which may inform a more specific etiology in neurodevelopmental disorders (Lui et al. 2011). Cortical surface area, for example, is thought to reflect neural stem cell proliferation and migration into the ventricular zone, which occurs early in embryogenesis (Rakic 2009). Cortical thickness, however, fluctuates throughout the lifespan, and is representative of a dynamic process involving cell layering, axonal myelination, synaptic growth and pruning (Shaw et al. 2008). It is important to note that typical cortical development involves a process of gradually increasing thickness during early childhood, which reaches a maximum prior to the onset of puberty, followed by gradual thinning which extends into early adult life (Shaw et al. 2008). Neuroimaging findings suggestive of altered developmental trajectories would be consistent with the conceptual understanding that ASD is a neurodevelopmental disorder.
Similar to the heterogeneity in VBM data, the literature with respect to cortical thickness in ASD is again inconsistent, and seems to vary significantly depending on the age of the study population. Early trials in a small group of children (mean age of 10 years) with ASD compared to controls identified increased cortical thickness, particularly in the temporal lobe, despite comparable brain volumes overall (Hardan et al. 2006). Follow up longitudinal MRI data by the same research revealed that patients with ASD had greater decreases in cortical thickness over time, which correlated with severity of symptomatology. The small sample size, however, resulted in marginal statistical significance once multiple comparisons were accounted for. Despite this, hypotheses regarding exaggerated cortical thinning, excessive elimination of synapses or impaired arborization were put forward from this data (Hardan et al. 2009).
Follow up investigations looking at cortical thickness in ASD have aimed not only to quantify specific differences in larger sample sizes, but also to document the magnitude and direction of aberrant cortical growth rates, taking into account the important effects of age.
Looking at broad age range (10–60 years), Raznahan et al. (2010) examined cross sectional imaging data in males with ASD vs. controls. Contrary to earlier findings, individuals with ASD were found to have smaller cortical volumes and thickness than controls at younger ages. Additionally, the rate of cortical thinning with age was attenuated for affected individuals, particularly in the temporal regions. Surface area did not change with time in either group (Raznahan et al. 2010) (see Fig. 7.2). Scheel et al. (2011) found similar results looking cross-sectionally at patients with ‘high functioning’ autism between ages 20–55 (Scheel et al. 2011). Along these lines, Doyle-Thomas et al. (2013) found attenuated cortical thinning in patients with ASD across a wide age ranges as well (7–39 years) (Doyle-Thomas et al. 2013). Recently, Ecker et al. published a report on cortical thickness, volume and surface area in men with ASD ages 18–42 years. They identified many non-overlapping areas of both increased and decreased cortical thickness and surface area in patients with ASD compared to controls, particularly in frontal and temporal regions, although age correlates were not provided (Ecker et al. 2013a).


Fig. 7.2
Warmer colours on brain maps identify areas where age-by-group interactions were significant. Graphs show scatter plots of cortical thickness by age (10–60 years) in these specific regions (dotted line: ASD). (Reproduced with permission from Cerebral Cortex. Raznahan et al. 2010)
On the other hand, analysis of cortical thickness data focusing on more narrow age ranges, and including a greater proportion of younger participants, seems to have yielded findings more consistent with those initially described by Hardan et al. (i.e. exaggerated thinning). Wallace et al. found that males with ASD ages 12–23 years, had thinner cortices overall, and then showed increased rates of cortical thinning during adolescence, with differences again most pronounced in the temporal regions (Wallace et al. 2010). Mak-Fan et al. (2012) looked at cross sectional data in children ages 6–15 years, and identified increased grey matter volume, cortical thickness and cortical surface area in younger children compared to controls, that then also underwent exaggerated thinning, surpassing controls on all measures between ages 10–12 years. Age effects were most pronounced in specific regions, including the medial precuneus and the left inferior frontal gyrus (Mak-Fan et al. 2012b).
In toddlers with ASD, cortical volume and surface area, (but not thickness) were found to be increased compared to controls at 2 years of age. The rate of cortical growth on longitudinal imaging between ages 2–5 years did not differ between groups, however, generating further questions regarding the specific chronology of aberrant neurodevelopment (Hazlett et al. 2011).
To summarize, a few studies suggest cortical overgrowth at very young ages; at age 2 this is marked by increased cortical volume and surface area, but potentially not thickness. School age children seem to display increased cortical thickness that is subsequently met with cortical dysmaturation throughout childhood and adolescence, suggestive mostly of exaggerated and then perhaps attenuated cortical thinning, although the specific magnitude and direction of this change varies depending on the study. The temporal lobe in particular is an area where rate of growth is most altered. Upcoming large scale, prospective neuroimaging trials may help to clarify some of this heterogeneity.
7.4 Surface Based Morphometry
In addition to cortical thickness and cortical surface area, the specific pattern of gyrification in the cortex can also be quantified with mathematical indices. The gyrification index (GI) for example, is the ratio of the total length of the folded cortical surface (extending into each sulcus) divided by the outer cortical surface length (see Fig. 7.3). The rationale for studying these indices is supported in part by early literature where visible structural abnormalities were noted in the gyri of a small group of patients with ASD. Also, as described above with respect to cortical thickness and surface area, specific patterns of gyrification may also be reflective of altered neurodevelopmental processes, or underlying differences in brain cytoarchitecture. For example, variations in neuron layers, cell density, and thalamocortical connections are noted on histology comparing tissue from sulci vs. gyri. Similarly, sulci formation in early life is hypothesized to be driven by tensile pull on areas with greater connectivity to distant regions [summarized in (White et al. 2003)]. Accordingly, altered functional connectivity may yield measurable structural changes in cortical morphology.


Fig. 7.3
The gyrification index was calculated by comparing the ratio of the inner cortical contour to the outer contour, on a coronal imaging slide. (Reproduced from Psychiatry Research. Hardan et al. 2004)
Early in the MRI literature, Piven et al. (1990) found significant structural abnormalities in the cortices of several adolescent males with ASD compared to controls. Of 13 patients, 5 displayed noteable polymicrogyria (multiple small cortical folds), and 2 had macrogyria (large cortical folds), as observed by radiologists blinded to diagnosis (Piven et al. 1990). Control participants, on the other hand had typically appearing brains. With enlargement of sample sizes, the more striking structural abnormalities in the brains of patients with ASD were for the most part washed out, necessitating a more precise mathematical approach to quantify specific differences.
Hardan et al. (2004) for example, found preliminary evidence suggesting increased gyrification in the frontal lobes of adolescent but not adult patients with ASD compared to controls, as measured by statistical comparison of GI. Patterns emerged suggesting changes in cortical folding with age in affected patients but not in controls (Hardan et al. 2004). In a population of patients with high functioning autism, increased cortical folding was noted in the frontal lobe (Jou et al. 2010). More recently, high-resolution analysis on 3T scanners described increased cortical folding in the occipital and parietal regions of the brain in patients with ASD compared to controls, but that cortical surface area was comparable between the two groups. Both groups showed a decline in gyrification overtime (Wallace et al. 2013).
7.5 Structural Regions of Interest
Many investigators have sought to identify specific regions of interest (ROI), which may be structurally altered in ASD and serve as a disease biomarker. A variety of investigative approaches have been employed. Early trials were labor intensive, requiring manual tracing and measurement of specific regions. Since the advent of VBM, broad surveys of the brain, as conducted in the studies described in Sect. 7.4, allowed for simultaneous comparison across dozens of regions of interest or thousands of specific voxels, aiming to identify areas associated with the greatest differences between patient and control samples. Alternatively, the a priori specification of a hypothesis regarding structural differences in a particular region of interest has also been used, to allow for conservation of statistical power. Additionally, complex statistical approaches using mathematical models and machine learning algorithms have recently been developed to combine the predictive power of both methods.
Dozens of ROI have been implicated through VBM data (summarized in Table 7.1), but even across meta-analyses, the locations of proposed voxel clusters are variable, and show increases and decreases in grey matter density and volume in similar regions. Meta-analytic approaches reveal some agreement regarding volume loss in the putamen (Duerden et al. 2012; Nickl-Jockschat et al. 2012; Yu et al. 2011) and amygdala (Nickl-Jockschat et al. 2012; Via et al. 2011; Yu et al. 2011), and volume gains in the caudate (Duerden et al. 2012; Stanfield et al. 2008; Yu et al. 2011). Throughout the medical literature, the neuro-anatomical locations most often cited in relation to ASD include the cingulate cortex, limbic system, prefrontal cortex , temporal and parietal lobe structures, cerebellum and basal ganglia (see Fig. 7.4) (Cauda et al. 2011; Amaral et al. 2008). These regions will be discussed along with possible functional implications as they pertain to social communication deficits and repetitive behaviors. Regions of interest within white matter tracts will be discussed separately in subsequent sections.


Fig. 7.4
Summary of neuro-anatomical locations implicated in ASD, and functional correlates of these structures. (Reproduced with permission from Trends Neurosci. Amaral et al. 2008)
7.5.1 Cerebellum
Some of the earliest MRI data in ASD noted structural differences in the ceberelli of patients with ASD, including hypoplasia of the cerebellar vermis (Courchesne et al. 1988) . Some findings seemed to be accounted for primarily by variability in IQ (Piven et al. 1992). Subsequent trials, however, repeatedly observed slight volume reductions in several cerebellar vermi, in keeping with reduced numbers of Purkinje cells noted in this region on post mortem tissue analysis [see (Fatemi et al. 2012) for a review of cellular pathology]. Hyperplasia of the cerebellar vermi has also been observed in ASD, however, leading some to propose a bimodal distribution of both hypo and hyperplasia in this region (Courchesne et al. 1994). An overall pattern of slightly increased cerebellar volumes have been noted as well, which are proportionate to the overall larger cerebral volumes (Stanfield et al. 2008). Some studies, however, have found no overall differences or alternatively volume reductions, with age effects perhaps playing a role (Amaral et al. 2008; Scott et al. 2009).
The cerebellum is classically understood to be important in sensorimotor processing and balance; deficits in its function would be in keeping with subtle motor impairments noted in patients with ASD (Gowen and Hamilton 2013). A growing consensus in the literature implicates the cerebellum’s role in emotion, memory, attention, language and cognitive circuits, given that it is highly connected to many cortical regions and networks (Strick et al. 2009). Patients with cerebellar agenesis for example, display not only ataxia, but cognitive and affective symptoms as well (Schmahmann 2004). Along these lines, several studies have correlated structural differences in the cerebellum of patients with ASD to language impairment (Hodge et al. 2010) and social function (Catani et al. 2008).
7.5.2 Anterior Cingulate Cortex
The anterior cingulate cortex is a highly connected part of the frontal lobe, with functions related to autonomic regulation as well as social/ emotional processing, reward anticipation and error monitoring (Pfeifer and Peake 2012). On structural neuroimaging, increases (Doyle-Thomas et al. 2013; Jiao et al. 2010) and decreases (Ecker et al. 2013a; Greimel et al. 2013b; Hadjikhani et al. 2006) in volume and thickness of the anterior cingulate have been shown. Age effects have also been described in the region, (Doyle-Thomas et al. 2013; Scheel et al. 2011), which may account for some heterogeneity in the literature. Greater thickness in the anterior cingulate has been associated with poorer social outcomes in ASD (Doyle-Thomas et al. 2013). With functional neuroimaging , altered connectivity between the anterior cingulate, frontal lobe and striatal structures in ASD was associated with impaired response to social rewards and in impaired social communication (Delmonte et al. 2013). Similarly, deficits in structural connectivity in the anterior cingulate have been hypothesized to contribute to repetitive and rigid behaviors, given dysfunction in the error monitoring mechanism (Thakkar et al. 2008).
7.5.3 Amygdala
There is evidence of altered growth tragectories involving the limbic system, particularly centered on the amygdala in patients with ASD. Meta-analysis across VBM data suggest volume loss overall (see Table 7.1), however more recent data including younger age groups describe evidence of volume overgrowth (Bellani et al. 2013b). The amygdala is understood to play an important role in threat monitoring, memory formation and emotional reaction formation, with connections extending to many deep structures such as the thalami, hypothalami and brain stem nuclei, as well as cortical structures in the prefrontal and temporal regions (Catani et al. 2013). Interpretation of the literature with respect to the amygdala’s role in ASD is complicated by findings that comorbid anxiety can result in structural changes in this region as well (Juranek et al. 2006).
The contributions of the amygdala to social cognition and behavior continue to be elucidated (Bellani et al. 2013b). There is some evidence that structural changes in this region may influence the formation of appropriate eye contact and gaze (Barnea-Goraly et al. 2014; Kliemann et al. 2012). Larger amygdala volumes correlated with greater social communication impairment in children with ASD across several studies (Munson et al. 2006; Schumann et al. 2009; Kim et al. 2010).
7.5.4 Basal Ganglia
The basal ganglia consist of a group of deep brain structures including the striatum (caudate nucleus and putamen), as well as the globus pallidus, substantia nigra, subthalamic nucleus and the nucleus accumbens. The basal ganglia are classically understood to play a central role in voluntary motor control, but are also involved in procedural learning, reward processing and some social- emotional circuitry. Findings regarding structural changes in the basal ganglia of patients with ASD must be interpreted in light of possible confounders including neuroleptic medication use, which has been shown to independently increase striatal volumes (Chakos et al. 1994).
Increased volume and rate of growth of the caudate has been described in ASD (Langen et al. 2013), even after controlling for neuroleptic treatment. Volume loss in the putamen is shown across VBM meta-analyses in adults (see Table 7.1), but volume gains in this area have also been described in younger populations (Hua et al. 2013). From a functional perspective, enlargement of the caudate has been associated with repetitive behavior, rigidity and self-injury (Langen et al. 2007; Qiu et al. 2010; Wolff et al. 2013; Hollander et al. 2005; Langen et al. 2013). Changes in the shape of this structure have also been correlated with social communication deficits (Qiu et al. 2010).
The nucleus accumbens is another structure sometimes considered part of the basal ganglia system. It is important for perception and experience of reward (Kohls et al. 2013). Impaired connectivity / activation of the nucleus accumbens in fronto-striatal circuitry may explain impaired processing of social rewards and punishment in patients with ASD (Delmonte et al. 2013; Delmonte et al. 2012).
7.5.5 Frontal, Temporal and Parietal Regions
The prefrontal cortex lies in the most anterior part of the brain; it is involved in cognition, attention, language as well as social and executive function. Sections within the prefrontal cortex are described anatomically, including the dorsolateral, ventromedial, frontopolar and orbitofrontal regions, for example. The prefrontal cortex is connected to temporal and parietal regions through long-range association fibers, together making up what has been described in the ASD literature as the “social brain network.” Reduced volume and decreased activation in frontal and temporal regions (e.g. inferior frontal gyrus and middle temporal gyrus) have been associated with social communication impairment (Yamasaki et al. 2010), and impaired processing of facial expressions (Bastiaansen et al. 2011) in patients with autism, respectively. Age effects may play a role in interpreting finding in this region, however. In children with autism, for example, a significantly greater number of neurons have been observed in the prefrontal region on post mortem tissue analysis, in keeping with overall greater brain volume and weight in younger patients with ASD (Courchesne et al. 2011b).
The temporal lobe is important for language development and comprehension. It also houses the fusiform face area along its medial side, which has been well described to be an important structure involved in processing facial cues. Young children subsequently diagnosed with ASD show early deficits in activity in the temporal lobe in response to language cues (Eyler et al. 2012). Increased volume in the fusiform gyrus correlated with impaired face processing in adults with ASD (Dziobek et al. 2010). The fusiform gyrus (as well as several other frontal and temporal structures) also showed weaker activity on fMRI in patients with ASD while looking at dynamic and static faces (Sato et al. 2012). Lastly, hypoactivation of the fusiform gyrus in ASD correlated with decreased gaze fixation on the eyes in pictures of faces (Dalton et al. 2005).
Hypotheses in ASD regarding deficits in ‘theory of mind’ and ‘mirror neuron systems’ also tend to be associated with frontal, temporal and parietal structures. Theory of mind, for example, is a developmental milestone whereby a child learns to distinguish his/her own thoughts and perceptions from those of others. Theory of mind has been demonstrated to be delayed or impaired in children with ASD, and is hypothesized to involve structures in the prefrontal cortex and the temporo-parietal junction (Kana et al. 2014). Similarly, the ‘mirror neuron system’ represents a group of neurons that activate not only when a participant performs an action, but also when that action is observed being performed by another. The mirror neuron system is thought to play an important role in learning, language development and theory of mind, and perhaps empathy, and therefore has been implicated in ASD, as well as in other neuropsychiatric conditions. The inferior frontal gyrus and the superior parietal lobule are structures most associated with the mirror neuron system (Hamilton 2013). Reduced activity or structural thinning in these regions have correlated with social communication deficits in ASD as well (Enticott et al. 2012; Hadjikhani et al. 2006).
7.6 White Matter: Volumetric Analysis and Diffusion Tensor Imaging
Earlier studies looking at white matter volume in ASD described a pattern of white matter overgrowth in young children but not adolescents compared to typically developing peers (Courchesne et al. 2004). Subsequent meta-analysis across VBM studies looking at white matter specifically described no difference overall in white matter volume, and no difference between child/adolescent and adult age groups, however data did not include very young children (< 6 years). Some regional differences were noted, with increased white matter volume in the right arcuate fasciculus, the left uncinate and inferior fronto-occipital fasciculus, as well as volume losses in the cingulum and corpus callosum (Radua et al. 2011).
Beyond volumetric analysis, diffusion tensor imaging (DTI) is an approach permitting a more detailed analysis of the white matter microstructure of patients with neurodevelopmental disorders such as ASD. DTI uses MRI technology combined with vector algebra, to describe the magnitude and direction of diffusion of water molecules within each individual voxel in the brain. Differences in diffusivity patterns are represented by several specific parameters, and are thought to reflect subcellular structural changes. It is important to understand these measurements in order to interpret the DTI literature in ASD.
Axial diffusivity (AD), for example, quantifies diffusion of water molecules occurring parallel to white matter fibers. Increased AD has been found in diseases involving axonal degeneration; in autism, AD is thought to reflect both the integrity and density of axons . Radial diffusivity (RD) is a measurement of diffusion traveling perpendicular to the white matter fibers. RD is increased in demyelinating diseases, and serves as a surrogate measure of white matter myelination across neurodevelopment. Mean diffusivity (MD) (also known as the apparent diffusion coefficient, ADC) summarizes the total average diffusion occurring in a region, in the absence of any directional gradient (Alexander et al. 2007; see Fig. 7.5).


Fig. 7.5
Top panels show diffusion vectors. MD = (λ1 + λ2 + λ3)/3, RD = λ2 + λ3)/2, AD = λ1, and
Bottom panel shows sample MRI images selecting for specific diffusion measurements. (Reproduced with permission from Autism Research. Travers et al. 2012)

A summary vector, termed “fractional anisotropy” (FA), describes the overall spherical shape of the other vectors combined. For example, an area where water molecules move freely in all directions would be described as “isotropic.” An isotropic solution would yield in a three-dimensional diffusion vector that appears perfectly spherical, represented by an FA value close to zero. On the other hand, areas where diffusion is restricted in certain directions (e.g. in a white matter tract) are considered “anisotropic.” The resulting three-dimensional vector would be more ellipsoid shaped, represented by an FA value closer to 1.
In neuroimaging of white matter tracts, higher FA is thought to be a sensitive but not specific marker of white matter integrity, including axonal myelination, fiber diameter, and the integrity of cell membranes (Kubicki et al. 2007). In typically developing individuals, FA increases and MD decreases with age, as white matter gradually matures (Faria et al. 2010). The specific pathological correlates of DTI in ASD remain to be fully elucidated, however. From mouse models, induction of demyelination resulted in decreased FA and increased RD, while reduced axonal caliber correlated with lower AD values (Harsan et al. 2006). In mouse models of autism, impaired sociability of the BALB/CJ was associated with lower FA and higher MD on DTI. On pathology, the BALB/CJ mouse had more scattered, less densely packed and more poorly myelinated white matter fibers. The authors attributed higher MD values to the reduced axonal density in affected mice, while more poorly myelinated white matter fibers were thought to result in lower FA values (Kumar et al. 2012).
Dozens of articles have been published looking at DTI in patients with ASD compared to controls across age groups. Across the whole brain, most studies observe reduced FA and decreased MD [reviewed by (Travers et al. 2012)]. A recent systematic review and meta-analysis combines data from 14 DTI studies using regions of interest methods (with mean ages of participants between 3 and 32 years). Their results highlight some consistency in findings and are compared to volumetric data in Table 7.2. Fractional anisotropy seems to be lower in corpus callosum, left uncinate fasciculus and left superior longitudinal fasciculus in affected patients vs. controls, suggestive of reduced white matter integrity and possibly reduced myelination in these regions. Fewer studies have published data on MD, but significant increases emerge in the corpus callosum and the superior longitudinal fasciculi as well, which may suggest reduced axonal density of the fibers in these tracts (Aoki et al. 2013).
Table 7.2
Comparison of meta-analyses of white matter volume and diffusion tensor imaging findings by regions in ASD
Meta-analysis on white matter volume | Meta-analysis on DTI | |
---|---|---|
Study | (Radua et al. 2011) | (Aoki et al. 2013) |
Mean age of patient group | 21.4 | 15.2 |
Whole brain white matter | ND | – |
Corpus Callosum | ↓ | ↓ FA ↑ MD |
Superior Longitudinal Fasciculus | – | ↓ FA (L) ↑ MD |
Arcuate Fasciculus | ↑ | – |
Inferior Longitudinal Fasciulus | – | ND FA |
Inferior Fronto-occipital Fasciculus | ↑ | ND FA |
Cingulum | ↓ | ND FA |
Uncinate Fasciulus | ↑ | ↓ FA (L) ND MD |
These findings may have functional implications related to the core features of ASD. The superior longitudinal fasciculus, for example, is a large white matter bundle coursing in a ‘front to back’ direction across the brain, relaying information between the frontal, temporal, parietal and occipital lobes. It is divided into several segments, which help to coordinate and relay information needed for movement, spatial navigation, occulomotor function, and importantly, language. Increased MD in the superior longitudinal fasciculus correlated with severity of language impairment in affected children and adolescents (Nagae et al. 2012), and lower FA scores in this region correlated with greater communication impairment on the ADOS and ADI-R (Poustka et al. 2012).
The uncinate fasciculus is an association fiber, which connects structures in the prefrontal cortex (specifically the orbital frontal cortex) with anterior temporal lobe structures as well as the amygdala. These regions are thought to be important for emotional regulation and processing, decision-making, and reward valuation and anticipation. In a study of behavioral interventions, increased adherence to therapy and improved clinical outcomes correlated with higher FA values in the uncinate fasciculus (Pardini et al. 2012). In infants, higher FA in the uncinate fasciculus at 6 months of age was predictive of greater social communication at 9 months of age (Elison et al. 2013).
The corpus callosum is the largest white matter structure in the brain, connecting the left and right cerebral hemispheres. It is involved in many cerebral functions including integration of sensory and motor information, as well as many higher cognitive tasks such as problem solving, executive function, planning, language, and social cognition and communication (Bellani et al. 2013a). Congenital absence of a corpus callosum (called ‘agenesis of the corpus callosum’) is a condition often associated with developmental delay and motor impairment, although at times can be asymptomatic as well. A screening survey on children with agenesis of the corpus callosum found that 45 % were over the cut-off for autism spectrum disorder (Lau et al. 2013).
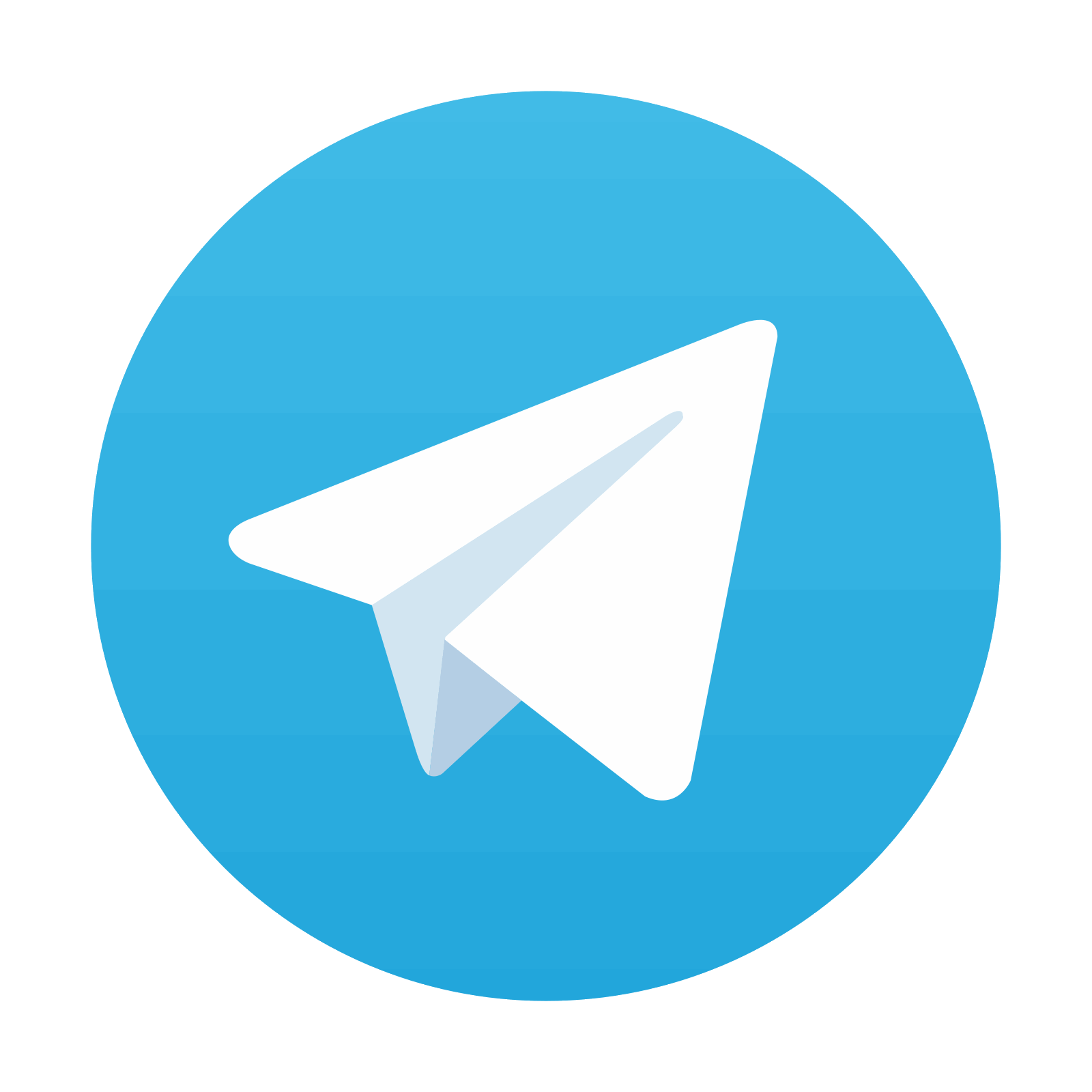
Stay updated, free articles. Join our Telegram channel

Full access? Get Clinical Tree
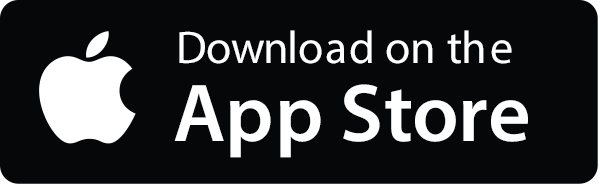
