Fig. 10.1
Healthy and total (healthy + unhealthy) life expectancy at birth in England, 1994–1999. Adapted from the Office for National Statistics licensed under the Open Government Licence v.1.0. From Bajekal (2005)
Given the demographic shifts occurring in developed countries, increasing healthy life expectancy is one of the critical elements of their healthcare systems. Healthy life expectancy provides a strong impetus for change, from a “reactive” to a “proactive” kind of medicine (see below). While twentieth century medicine was about finding causes and treatments, twenty-first century medicine looks for mechanistic pathways and preventive strategies.
What are the most common conditions associated with lower healthy life expectancy? In developed countries, the leading causes of disability—expressed as years lived with a disability—are neuropsychiatric conditions (41.9 %), cardiovascular conditions (6.7 %), respiratory (6.9 %) and musculoskeletal (7.6 %) diseases, malignant neoplasms (2.4 %) and diabetes mellitus (2.3 %) (Mathers et al. 2004). Among neuropsychiatric conditions, the most significant causes of disability are depression (15 %), alcohol-use disorders (6.8 %) and Alzheimer’s and other dementias (4.2 %) (Mathers et al. 2004). The number of years lived with disability (YLD) is rising, not only due to the increase in total life expectancy but also due to the increasing prevalence of diseases associated with a sedentary lifestyle (e.g. Kohl et al. 2012; Tremblay et al. 2011); moderate levels of physical activity (brisk walking daily) can increase life expectancy by as much as 4 years (Moore et al. 2012). Many of the major causes of disabilities listed above fall into this category.
Let us now consider the following common disorders of the brain and body: depression, addictions, dementias and what is known as metabolic syndrome (high blood pressure, impaired fasting glucose or insulin resistance, dyslipidemia, central obesity). The total number of YLD due to these disorders accounts for as much as 38.9 % of all YLD from all causes (Mathers et al. 2004). This is due to their prevalence (Table 10.1), tendency to emerge early in life (adolescence) and chronic course. Furthermore, living with these disorders is associated with a significant loss of the quality of life. This can be estimated by calculating preference scores for a given health state, which are typically expressed on a scale ranging from 1 (ideal health) to 0 (equivalent to being dead). For example, in a sample drawn from general population, living with moderate or severe depression is associated with preference scores of 0.7 and 0.5, respectively (Pyne et al. 2009). Not surprisingly, the economic cost of living with these disorders is staggering (Table 10.1). Depression alone ranks among major causes of disability worldwide—the second largest in industrialized countries (Lopez et al. 2006). In Canada, one estimate of the annual cost of depression, addictions and dementias combined is $50 billion.
Table 10.1
Prevalence, the number of healthy life years lost in the Americas due to a given condition and the annual cost of common chronic disorders in Canada
Disease | Prevalence | Health years lost—Americas | Annual cost (CDN)—Canada | ||
---|---|---|---|---|---|
Adolescents (12–19 years) | Middle aged (20–64 years) | Aged (65+ years) | |||
Depression | 6.4 | 5.0 | 1.9 | 10,764,000 | $1.4 billion |
Addiction | $39.8 billion | ||||
Alcohol | 7.0 | 2.0 | Unavailable | 4,830,000 | $14.6 billion |
Illegal drugs | 2.7 | 0.5 | Unavailable | 2,433,000 | $8.2 billion |
Dementia | Unavailable | Unavailable | 8.0 | 2,209,000 | $15 billion |
Obesity | 19.7 | 18.0 | 19.1 | Unavailable | $4.3 billion |
Hypertension | 0.6 | 12.8 | 48.9 | 1,105,000 | $880 million |
Diabetes | Unavailable | 4.2 | 18.1 | 4,095,000 | $5.6 billion |
Stroke | Unavailable | 1.3 | 8.5 | 3,988,000 | $3.6 billion |
Acute myocardial infarction | Unavailable | 1.7 | 10.2 | 6,523,000 | $3.0 billion |
Cancer | 0.06 | 1.0 | 6.2 | 11,461,000 | $14.2 billion |
Furthermore, as we pointed out in Sect. 8.3.2, common disorders of the body, such as obesity, diabetes, hypertension and dyslipidemia, are associated with impaired brain health. Overall, increasing with age, there is significant co-morbidity across the brain and body disorders (Fig. 10.2). Thus, common psychiatric co-morbidities of depression are anxiety disorders and substance (alcohol) abuse (e.g. Schuckit 2006; Krishnan 2005). Some consider depression to be one of the risk factors for dementia (Tsuno and Homma 2009). Among medical co-morbidities of depression, the most prevalent and/or clinically relevant are cardiovascular disease, obesity and diabetes. The mortality rate for patients with depression is twice that of the general population and is mainly due to increased cardiovascular mortality and suicides (Osby et al. 2001). Finally, dementia is more than twice as likely to occur in patients with metabolic syndrome (Vanhanen et al. 2006; see Sect. 8.3.2). This underlines the importance of an integrated brain–body approach when searching for risk profiles predicting brain health. Table 10.2 illustrates this point in terms of co-morbidities of bipolar disorders (Krishnan 2005).
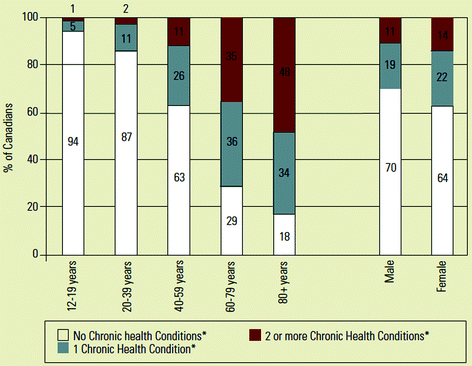
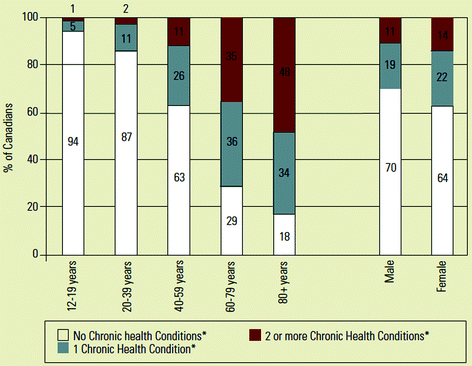
Fig. 10.2
Percentage of Canadians with none, one or two and more chronic health conditions (by age). *Select chronic health conditions include arthritic, cancer, chronic obstructive pulmonary disease, diabetes, heart disease, high blood pressure and mood disorders. Source: Statistics Canada (2005). From Broemeling et al. (2008).
Table 10.2
Lifetime co-morbidities of bipolar disorder with other disorders. PTSD post-traumatic stress disorder; OCD obsessive–compulsive disorder
Co-morbid condition | Mean rate of co-morbidity (%) | Percentage range across studies (%) |
---|---|---|
Any axis I disorder | 65 | 50–70 |
Substance use disorder | 56 | 34–60 |
Alcohol abuse | 49 | 30–69 |
Other drug abuse | 44 | 14–60 |
Anxiety disorder | 71 | 49–92 |
Social phobia | 47 | |
PSD | 39 | |
Panic disorder | 11 | 3–21 |
OCD | 10 | 2–21 |
Binge-eating disorder | 13 | |
Personality disorder | 36 | 29–38 |
Migraine | 28 | 15–40 |
Overweight | 58 | |
Obesity | 21 | |
Type 2 diabetes | 10 | |
Hypothyroidism | 9 |
10.2 Personalized Preventive Medicine
One size does not fit all. In the general population, the clinical manifestations (symptoms) and pathophysiology of the chronic disorders mentioned above are far from uniform. To start with, the prevalence rates of most of these disorders vary by sex (e.g. higher female-to-male ratio for depression [1.69] and Alzheimer’s dementia [1.99] and lower for alcohol-use disorders [0.24]; Mathers et al. 2004), age and ethnicity (Chiu et al. 2010). Pathways leading to these disorders are also likely to differ across different segments of the general population. For example, Chiu and colleagues reported a relatively low incidence of heart disease in Black Canadians, despite the high composite index of relevant risk (Chiu et al. 2010). This might indicate an uncoupling somewhere along the risk pathways or the existence of a resilience mechanism that counteracts the detrimental effects of these risk factors.
Another example of such an atypical profile is the virtual absence of obesity-associated hypertension in Pima Indians in Arizona, despite the presence of the typical association between type 2 diabetes and obesity in the same group (Hanson et al. 2002). In this case, the uncoupling may occur between fat cells (e.g. production of free fatty acids [FFA] and/or FFA-related signalling) and the autonomic nervous system. Persistent activation of the ANS is one pathophysiological mechanism leading to hypertension (Pausova 2006). Can detailed knowledge of an individual’s brain and body, gained through the approaches described here, be translated into ways of improving his/her health?
10.2.1 Personalized Medicine
Personalized medicine has emerged as a new paradigm that attempts to tailor treatment strategies to a specific patient (Hamburg and Collins 2010). In this context, biological markers (or biomarkers) are defined as any of an individual’s measured characteristics that may strongly indicate the suitability of a particular (drug) treatment for that individual (Anonymous 2001). Typically, biomarkers index the state of key pathogenic mechanisms underlying a given disease or an individual’s response to a specific treatment. But in the context of making long-term predictions, we need (preclinical) biomarkers unaffected by the full-blown diseases and its treatment (Text Box 10.1).
Text Box 10.1. Preclinical biomarkers
Biomarkers can index the state of key mechanistic pathways leading to a given disease. This can be achieved at different levels: (1) genomic level (e.g. a DNA variation associated with the low activity of an enzyme); (2) transcriptomic level (e.g. expression level [RNA] of this enzyme in the relevant tissue); (3) proteomic level (e.g. levels of this enzyme in blood or the relevant tissue); and (4) systems level (e.g. state of the organ/system impacted by the action of this enzyme, such as the structural and functional properties of vessels). Note that studying young- and middle-aged individuals in a preclinical stage of disorders is essential for identifying biomarkers (and mechanistic pathways) free of confounders associated with a full-blown (clinical stage) disease and its treatment.
At present, pharmacogenetics is perhaps the most promising example of personalized medicine. For example, the Food and Drug Administration in the United States approved several diagnostic tests to predict the effectiveness of specific anti-cancer drugs, based on the expression of particular genes in the tumour (Hambug and Collins 2010). Similarly, a dosage of an anti-coagulant drug (warfarin) is influenced by variants of the genes encoding specific metabolizing enzymes (CYP2C9, VKORC1), among European Americans but not African Americans (Limdi et al. 2008).
These examples illustrate the promise as well as the challenges of personalized medicine. A single biomarker is likely to have limited predictive value for an individual, especially when applied in the context of a complex disorder. For this reason, attention is now shifting towards “biomarker signatures/patterns” or “profiles of change” (Barker 2004; Scherer et al. 2010), again emphasizing the importance of high-dimensional and high-throughput phenomics. This topic is addressed below in the section on the risk/resilience profiling.
10.2.2 Preventive Medicine
Preventive medicine is likely to benefit from the same personalized approach as described for personalized medicine above. One of the challenges of implementing preventive medicine lies in the effectiveness of global public health initiatives aimed at reducing known risk factors for chronic disorders, such as metabolic syndrome. Despite clear benefits, a large segment of the general population fails to follow broad (i.e. generic) health recommendations. For example, the EPIC-Norfolk study, carried out in the United Kingdom between 1993 and 2006, revealed that only 21 % of men and 39 % of women engaged in all four of the healthy behaviours studied: being physically active, eating enough fruits and vegetables, avoiding excessive use of alcohol and not smoking (Khaw et al. 2008). These four factors carried a benefit of a fourfold reduction in the relative risk of mortality from all causes (including a fivefold reduction in cardiovascular mortality risks), compared with those who did not engage in those healthy behaviours at all. This is equivalent to adding 14 years of chronological age to an individual’s life expectancy (Khaw et al. 2008).
As a way of complementing global health promotion strategies (those applied to the general population), the healthcare system is beginning to focus its prevention strategies on individuals at risk of developing a disease (primary prevention) or on stopping a disease (or co-morbidity) in its early stages (secondary prevention). The main challenge of this approach is to identify at-risk individuals. As discussed extensively by the British epidemiologist Geoffrey Rose, a single biomarker is likely to identify individuals at risk of a disease only when it reaches extreme values; thus, only individuals at very high risk are identified (Rose 2008). But, by definition, this happens only in a small fraction of the general population. In the majority of individuals, the biomarker has as an intermediate value—making it difficult to decide with confidence whether or not a given individual is at risk of the disease in question. In terms of public health, this poses a conundrum (Text Box 10.2).
Text Box 10.2. Estimating risk using a single biomarker
Let us have a look at an example of glycated haemoglobin (HbA1C), a marker of glucose levels in blood in past months (and, in turn, diabetic state and its compensation), and its association with mortality (from Rose 2008). Rose notes that intermediate levels of HbA1C are associated with a relatively low relative risk (RR) of mortality (RR = 1.5), as compared to its high levels (RR = 3.5). On the other hand, because of the higher prevalence of intermediate (~24 %) versus high (~4 %) values in the general population, the estimated excess mortality rate associated with intermediate values is greater for intermediate versus high values of HbA1C (~33 vs. ~24 %, respectively) (Rose 2008). Thus, we cannot really say whether or not an individual with an intermediate level of HbA1C would become sick and die earlier, and yet, at a population level, these individuals contribute more to the overall mortality attributable to this phenotype than individuals with high levels.
As pointed out by Khaw and Marmot in their commentary on Rose’s Strategy of Preventive Medicine, “the majority of cases in the population occur not in the small numbers at very high risk but in the centre of the population distribution, where large numbers of people are exposed, albeit with only modest increases in risk” (Rose 2008, p. 7). Thus, one solution for personalized preventive medicine is to identify (the large number of) individuals at modest risk of developing a disease and offer them personalized strategies for risk reduction. But this cannot be achieved with a single biomarker. We suggest, instead, the development of risk/resilience profiling, based on multiple characteristics measured objectively in each individual, with state-of-the-art technologies operating at both a molecular and a system level. Clearly, one hopes that if we combine such high-fidelity information about the individual’s phenome with details about his/her genome and envirome, we will attain a level of accuracy to justify actions tailored to a particular individual.
10.3 Risk/Resilience Profiling
Profiling has been used in medicine from the very beginning. It is the specific combination of symptoms that leads to a correct diagnosis and any available treatment (McInnes and Semple 1994). For example, using data collected in the Framingham Heart Study, a 10-year risk of coronary heart disease (myocardial infarction and coronary death) can be calculated based on a number of (now) known risk factors, including age, total cholesterol, HDL cholesterol, systolic blood pressure, treatment for hypertension and cigarette smoking (Framingham Risk Scores; Anonymous 2002, 2010). Note, however, that this index fails to identify a great proportion of future coronary heart disease (Thompson et al. 2006).
A profile may indicate an optimal combination of physiological parameters associated with, for example, a long-term history of success in running a marathon (Maud et al. 1981). In general, the predictive value of a profile reflects the current state of pertinent knowledge. Conceptually, a profile serves a dual purpose: (1) a practical one (i.e. as a predictor) and (2) a theoretical one (i.e. as a model of the current state of knowledge). As explained below, phenotypic rather than genetic profiling may provide more power for building such predictive models. Nonetheless, we will begin by reviewing current concepts of genetic profiling—and their critiques—before turning our attention to the phenotypic profiling approach.
10.3.1 Genetic Profiling
Genetic profiling emerged as a possible tool for predicting an individual’s risk of complex diseases, using variations in his or her genome. Unlike monogenetic disorders—for example, Huntington’s disease (Fig. 10.3a) or phenylketonuria (Fig. 10.3b)—complex diseases are likely caused by host of factors: multiple genes (G1–G4 in Fig. 10.3c), various environmental influences (Fig. 10.3e) and, of course, combinations of the two (Fig. 10.3d, f).
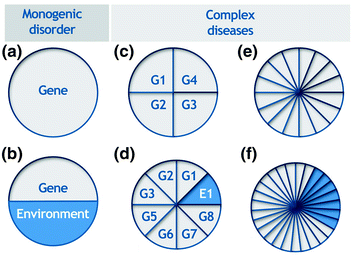
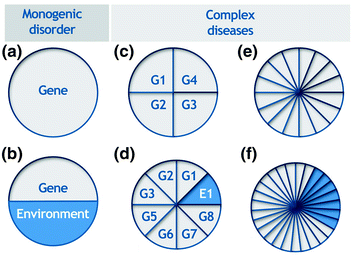
Fig. 10.3
Complete cause models or sufficient causes of disease development, G, gene; E, environment. From Janssens and van Duijn (2008)
Thus, “complex diseases result from the joint effects of multiple genetic and environmental causes, with each factor having only minor contribution to the occurrence of the disease” (Janssens and van Duijn 2008). Not surprisingly, we gain very little information by predicting the risk of such complex disorders using a single genetic variant (Vineis et al. 2001). This explains the shift towards simultaneous testing at multiple genetic loci, in other words, genetic profiling. But, as reviewed by Janssens and van Duijn (2008), genetic profiling for complex disorders has not been successful thus far; discriminative accuracy varies between 0.55 and 0.8 (0.5 = chance; 1.0 = perfect discrimination).
Even more important is the observation that genetic profiling adds very little, if anything, to the predictive value of clinical risk factors associated with complex disorders—including known biomarkers such as body mass index for type 2 diabetes (Janssens and van Duijn 2008). As concluded by these authors, “genetic testing may not improve the prediction of disease beyond classical risk factors or new biomarkers, if most of the genes involved in the disease play a role through these risk factors”. On the other hand, they underscore the heuristic value of genetic profiling for revealing new pathophysiological pathways underlying these disorders and, in turn, new opportunities for the development of novel interventions and prevention strategies (Janssens and van Duijn 2008). It should be pointed out that the above discussion is limited to profiling based on genetic (DNA) variations in the genome: genomic profiling may be a better term to use here. At present, it is unknown whether variations in the epigenome (epigenomic profiling) or in gene expression (transcriptomic profiling) may add unique predictive value, especially if assessed in relevant tissues.
10.3.2 Phenotypic Profiling
Phenotypic profiling—the measurement of the set of functional and structural characteristics at a specific point in an individual’s life—has the advantage of capturing the cumulative effects of both genetic and environmental factors.
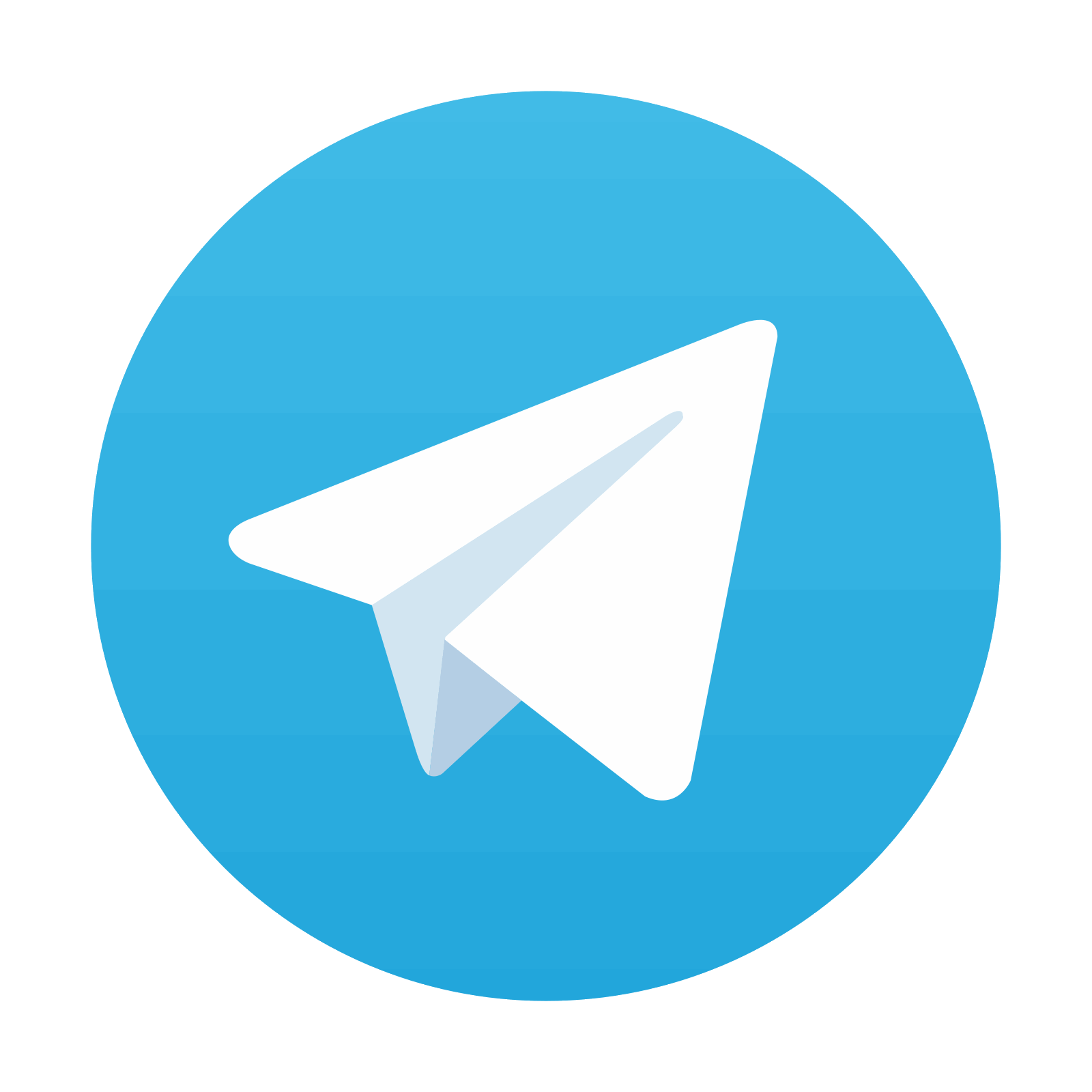
Stay updated, free articles. Join our Telegram channel

Full access? Get Clinical Tree
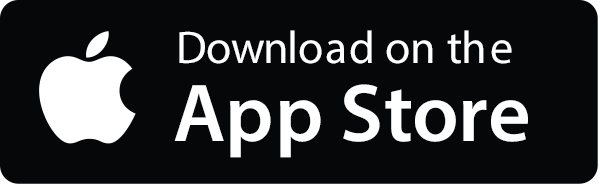
