Fig. 2.1
Diagram of a BMI system for environmental control and communication (modified from Kansaku [11])
We constructed a prototype of the BMI-based environmental control system. For the visual stimuli, we first prepared four panels with white/gray flicker matrices, one each for the desk light, primitive agent robot, television control, and Japanese alphabet (hiragana) spelling. We tested the system on both quadriplegic and able-bodied participants and reported that the system could be operated effortlessly [12].
One specific merit of the P300 speller algorithms is that we can easily translate the subject’s thoughts as a command preassigned to each icon. Therefore, our system can be used for various applications in environmental control and communication.
2.2.2 Effects of Visual Stimuli for P300-BMI
The white/gray flicker matrix has been used as a visual stimulus for the P300-BMI, but the white/gray flash stimuli might induce discomfort, particularly in subjects with a history of epilepsy. Parra et al. evaluated the safety of chromatic combinations for those with photosensitive epilepsy [13]. Five single-color stimuli (white, blue, red, yellow, and green) and four alternating-color stimuli (blue/red, red/green, green/blue, and blue/yellow with equal luminance) of four frequencies (10, 15, 20, and 30 Hz) were used as the visual stimuli. Under white stimulation, flickering stimuli with higher frequencies, especially those greater than 20 Hz, are potentially provocative. Under the alternating-color stimulation condition, as suggested by the Pokemon incidence [14], the 15-Hz blue/red flicker was the most provocative. Notably, the green/blue chromatic flicker emerged as the safest and evoked the lowest rates of EEG spikes. Accordingly, we investigated the effectiveness of green/blue flicker matrices as visual stimuli.
We evaluated the subjective feeling of comfort when using the green/blue chromatic flicker matrices (n = 9; 8 able-bodied and 1 spinal cord injury). Two panels with matrices (3 × 3 and 8 × 10) were intensified with two-color combinations (green/blue and white/gray); these were used for desk-light control and hiragana spelling. We used a visual analogue scale (range: 0–100 %) to evaluate the subjective feeling of comfort. The measured value for the green/blue (white/gray) flicker matrices was 79.3 % (49.0 %) in the desk-light control condition and 58.0 % (34.5 %) in the hiragana spelling condition. Significant differences between the two-color combinations were observed for each condition (p < 0.05) [15].
We further recruited able-bodied, untrained subjects to perform hiragana spelling using an 8 × 10 matrix with three types of intensification/rest flicker combinations (L, luminance; C, chromatic; LC, luminance and chromatic); both online and offline performances were evaluated (n = 10). The accuracy rate under the online LC condition was 80.6 %. Offline analysis showed that the LC condition was associated with significantly higher accuracy than was the L or C condition (p < 0.05) (Fig. 2.2). No significant difference was observed between the L and C conditions. The LC condition, which used the green/blue flicker matrix, was associated with better performances in the P300-BMI [16]. We showed that the green/blue flicker matrices were associated not only with a better subjective feeling of comfort but also with better performance.
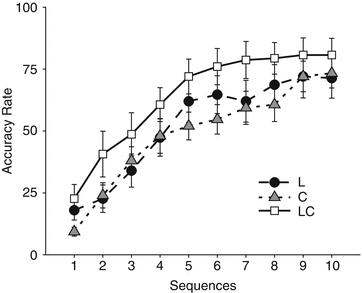
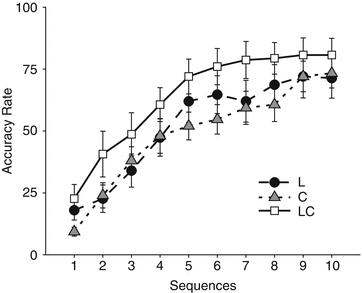
Fig. 2.2
Green/blue flicker matrix provided better performance in P300-BMI operation (modified from Takano et al. [16]). Mean performance curves at each condition for all ten sequences are shown. Mean performances in luminance (L), chromatic (C), and luminance and chromatic (LC) conditions are plotted by the broken line with black circles, the dotted line with gray triangles, and the solid line with white squares, respectively
To increase accuracy when operating the BMI system and to develop better classification methods [17–20], some studies have attempted to identify better, more efficient experimental settings by manipulating factors such as the matrix size and duration of intensification [21], channel set of the EEG [22], and flash pattern of the flicker matrix [23]. We proposed a method that combines luminance and chromatic information to increase the accuracy of performance using the P300-BMI, and this method can be applied along with those proposed in the aforementioned reports.
2.2.3 Neuronal Substrates Underlying the P300-BMI
To identify brain areas that were more enhanced in the green/blue flicker matrix than in the white/gray flicker matrix, we applied simultaneous EEG–functional magnetic resonance imaging (fMRI) recordings (n = 12). We did this because such recordings may highlight areas devoted to improved P300-BMI performance. The peak of the positive wave in the EEG data was detected under both conditions, and the peak amplitudes were larger at the parietal and occipital electrodes (particularly in the late components) under the green/blue condition than under the white/gray condition. fMRI data showed activation in the bilateral parietal and occipital cortices, and these areas, particularly those in the right hemisphere, were more activated under the green/blue condition than under the white/gray condition. The parietal and occipital regions more involved in the green/blue condition were part of the areas devoted to conventional P300s. These results suggest that the green/blue flicker matrix was useful for enhancing the so-called P300 responses [24].
We then applied magnetoencephalography (MEG) and aimed to map functional connectivities during the use of the P300-BMI using the two types of visual stimuli: green/blue and white/gray flicker matrices (n = 8). We used alphabet flickers (6 × 6) as the two types of visual stimuli. Whole-head 306-channel MEG data (Neuromag, Elekta) were collected during the experiment. The gradiometer data were segmented, and current sources were estimated with a narrowband scalar minimum-variance spatial filter [25, 26]. Anatomical MR images of each subject were used for coregistration and normalization. The mean imaginary coherence was computed in the alpha band (8–12 Hz) [27]. Significantly greater coherence was observed in the right posterior parietal cortex in the green/blue condition than in the white/gray condition. The larger functional connectivity observed in the green/blue condition may play a significant role in driving the P300-BMI [28].
2.2.4 Effects of Visual Stimuli for SSVEP
The SSVEP is another main approach in noninvasive BMIs; however, relatively low-frequency flickers have been used to elicit SSVEP signals, so these flickers are usually visible. If the frequencies used exceed the critical flicker frequency (CFF), these high-frequency flickers can be invisible. We aimed to prepare a new SSVEP-based BMI system using flickers with a higher frequency than the CFF. We prepared flickering devices with green and blue LEDs, and each color LED flickered alternately to elicit the SSVEP. We first evaluated the CFF in the participants (n = 6), and the mean CFF was 55.7 Hz (range: 51.2–60.3). We then evaluated the SSVEP amplitude from Oz while the participants fixated on an LED flicker with different frequencies of 30–70 Hz. The mean luminance was 500 cd/m2. We showed that the SSVEP was effectively induced by adjusting the luminance of green and blue color stimuli even if the frequency was higher than the CFF. We then applied the visible flickering stimuli (41, 43, and 45 Hz) and invisible flickering stimuli (61, 63, and 65 Hz) to elicit the SSVEP (n = 6). An EEG-triggered in-house robotic exoskeleton was used. The participants successfully controlled the equipment using both visible and invisible flickering stimuli (mean success rates of 85.6 and 82.0 %, respectively). The information transfer rate was >75.0 bit/min for each. Our new BMI system using high-frequency flickers of a higher frequency than the CFF was able to induce the SSVEP [29]. The green/blue color combination was a practical color combination not only for the P300-BMI but also for the SSVEP.
2.2.5 AR-BMI
These BMI systems can be used by persons with disabilities to support their daily activities. In this type of system, users rely on control panels that are pre-equipped; thus, each system is specialized for the user’s specific environment (e.g., his or her home). To expand the range of possible activities, it is desirable to develop a new system that can be readily used in new environments, such as hospitals. To make this possible, we added an AR feature to a P300-BMI. In this new system, we used a see-through head-mounted display (HMD) to create control panels with flicker visual stimuli to support the user in areas close to controllable devices. When the attached camera detected an AR marker, the position and orientation of the marker were calculated, and the control panel for the preassigned appliance was created by the AR system and superimposed onto the HMD. The participants were required to control system-compatible devices, and they successfully operated them without significant training (n = 15). Online performance with the HMD was not different from that using an LCD monitor. Posterior and lateral (right or left) channel selections contributed to the operation of the AR-BMI with both the HMD and LCD monitor. AR-BMI systems operated with a see-through HMD may be useful in building advanced intelligent environments [30].
A BMI study that used an immersive HMD and LCD monitor to provide visual stimuli showed no significant difference between the two technologies [31]. We applied both a see-through HMD and an LCD monitor to an AR-BMI system to further evaluate the effect of different types of visual stimuli, and in the online evaluation, the performance with the HMD was not different from that with the LCD monitor. The percent accuracy in this study ranged from 76 to 88 %; because the incidence of correct responses exceeded 70 %, the system is considered to have reached the level of practical use [22, 32].
We further proposed a new BMI system that permits the control of devices outside the user’s body. When the robot’s eyes detect an AR marker, the preassigned infrared appliance becomes controllable. The position and orientation of the AR marker were calculated from the images detected by the camera, and a control panel for the appliance was created by the AR system and superimposed onto the scene detected by the robot’s eyes (Fig. 2.3). To control our system using brain signals, we modified the P300 speller. The able-bodied participants were first required to make the robot move to a desk light in the robot’s environment (n = 10). Online performance was evaluated, and the mean accuracy for controlling the robot was 90.0 %. When the robot’ eyes detected the AR marker of the desk light, a flicker panel for controlling the appliance was displayed on the screen. The participants then had to use their brain signals to operate the light in the robot’s environment through the robot’s eyes. Online performance was evaluated, and the mean accuracy of light control was 80.7 %. By applying the AR technique with the BMI, we successfully showed that brain signals not only controlled an agent robot but also operated home electronics in the robot’s environment [33]. The user’s thoughts became reality through the robot’s eyes, enabling the augmentation of real environments outside the anatomy of the human body.
2.3 In-House Environmental Control System
2.3.1 System Development
Here, we list our studies of the basic characteristics of EEG-based environmental control and communication. Further, to apply these methods for clinical evaluation, we created an in-house environmental control system comprising hardware and software parts. For the hardware part, we constructed EEG amplifiers (24 bit, 1,024 Hz), EEG electrodes, EEG caps, and SSVEP devices. We implemented the P300, SSVEP, and sensorimotor rhythm for the system. The sensorimotor rhythm [34] and the SSVEP [35] can be used as a master switch, and the P300 and SSVEP can be used for communication or control of home electronics.
2.3.2 In-House EEG Electrodes
When applying EEG methodology, users must place EEG electrodes on the scalp each time, and the development of easy-to-use electrodes for clinical use is required. Therefore, we developed a conductive nonadhesive solid gel electrode for practical noninvasive BMIs. We performed basic material testing, including examining the volume resistivity, viscoelasticity, and moisture-retention properties of the solid gel. We then compared the performance of the solid gel, a conventional paste, and an in-house metal pin-based electrode using impedance measurements and P300-BMI testing. The solid gel was observed to be conductive (volume resistivity: 13.2 Ω cm) and soft (complex modulus: 105.4 kPa), and it remained wet for a prolonged period (>10 h) in a dry environment. Impedance measurements revealed that the impedance of the solid gel-based and conventional paste-based electrodes was superior to that of the pin-based electrode (Fig. 2.4a). The EEG measurement suggested that the signals obtained with the solid gel electrode were comparable with those obtained with the conventional paste-based electrode. Moreover, the P300-BMI study suggested that systems using the solid gel- or pin-based electrodes were effective. One of the advantages of the solid gel is that it does not require cleaning after use, whereas the conventional paste adheres to the hair, which requires washing. Furthermore, the solid gel electrode was not painful in contrast to the metal pin electrode [36].
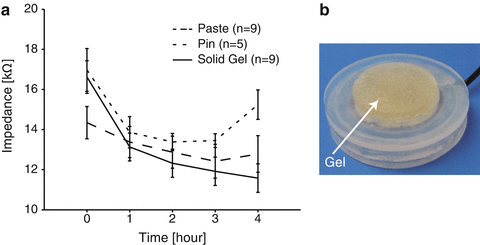
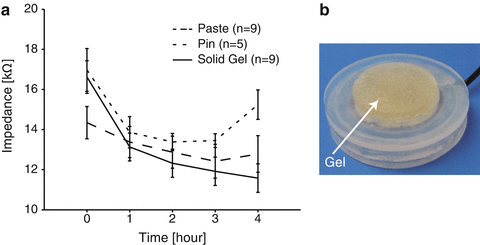
Fig. 2.4
(a) Time course of the electrode impedance of the solid gel-, metal pin-, and conventional paste-based electrodes mounted on the scalp (modified from Toyama et al. [36]). (b) Disposable electrode with the nonadhesive conductive gel
The solid gel electrode worked well for practical BMIs and could be useful for bedridden patients such as those with amyotrophic lateral sclerosis (ALS). For bedridden patients, we created the soft cap with electrode holders. We also developed disposable electrodes using the nonadhesive conductive solid gel for further easy use (Fig. 4b).
Moreover, these solid gel electrodes are potentially useful for other long-term EEG investigations such as sleep recordings and long-term monitoring of patients with epilepsy.
2.3.3 Easy-to-Use Clinical BMI System
To use the BMI system to support the daily activities of persons with disabilities, the system must be set for easy use. Therefore, we developed an easy-to-use BMI system. We developed peripheral devices, solid gel EEG electrodes, an external keypad, users’ manuals, and an online support platform. We prepared installation instruction manuals for BMI devices and EEG electrodes, including detailed step-by-step instructions and many photographs. The external keypad has seven buttons, and each button was assigned to a specific procedure, e.g., checking the EEG waveform or making a discriminant analysis template. The online support platform applied a remote desktop program and a 3G network, which enabled developers to check the BMI-based environmental control system, check EEG waves, update the programs, and fix any problems. The new easy-to-use BMI system was tested in a patient with ALS (male, age 61 years, ALSFRS-R = 0) by hospital staff members without the presence of developers. The patient was required to operate the P300-BMI. The hospital staff members successfully set up the BMI devices and EEG soft cap with electrodes, and the patient successfully controlled the P300-BMI (92 % accuracy). The online support platform also worked well. The easy-to-use BMI system worked well in clinical testing, which suggests that it can be used clinically [37].
2.3.4 Unified User Interface Ready for EEG and Other Biosignals
To date, patients with progressive diseases have been required to change various input devices (e.g., push button, eye gaze tracker, EMG sensor, piezo sensor, and BMI), and these patients must replace the user interface (UI) to fit the level of paresis whenever they change the input devices. We developed a unified UI that can be used for various input signals. We prepared a unified UI with icons in a tiled arrangement and a cursor. The icons provide various functions, such as character input, light control, changing television channels, emailing, and web browsing. Kinetic switches, eye gaze tracking, and SSVEP can be used to control the cursor. An external 1-ch signal stops a step-by-step sliding cursor to drive the target icon function. Alternatively, two or three signals result in up, down, left, or right movement of the cursor to drive the target icon function. The tiled icons can also be used as a flicker matrix for operating the P300-BMI. For character input, the UI provides direct and predictive input methods. The unified UI, prepared for EEG and other signals, worked well in our system [38].
2.3.5 Clinical Trials of BMI-Based Environmental Control System
2.3.5.1 Patients with Cervical Spinal Cord Injury
We evaluated the efficacy of the P300-BMI with the green/blue flicker matrices for individuals with cervical spinal cord injury (SCI). Ten individuals with cervical SCI (age 26–53 years, all male) and 10 age- and sex-matched able-bodied controls (age 27–52 years, all male) with no prior BMI experience were asked to input hiragana (Japanese alphabet) characters using the P300-BMI with two distinct types of visual stimuli (white/gray and green/blue) in an 8 × 10 flicker matrix. Both online and offline performance were evaluated. The mean online accuracy of the patients with SCI was 88.0 % for the white/gray and 90.7 % for the green/blue flicker matrices. The accuracy of the control subjects was 77.3 and 86.0 % for the white/gray and green/blue, respectively. There was a significant difference in online accuracy between the two types of flicker matrices. Patients with SCI performed with greater accuracy than did controls, but the main effect was not significant. Individuals with cervical SCI successfully controlled the P300-BMI, and the green/blue flicker matrices were associated with significantly greater accuracy than the white/gray matrices. The P300-BMI with the green/blue flicker matrices is effective for use not only in able-bodied subjects but also in individuals with cervical SCI [39].
2.3.5.2 Patients with ALS
We also evaluated the effect of visual stimuli in the P300-BMI for patients with ALS. We applied a newly developed region-based two-step P300 speller, which has a larger flashing area than the conventional visual array. Seven ALS patients and 7 age- and sex-matched able-bodied control subjects were required to input hiragana characters using our P300-BCI system. We prepared two types of input procedures, the conventional row/column (RC) speller and the two-step speller, and evaluated their online performance. The mean online accuracy of the ALS patients was 24 % for the RC condition and 55 % for the two-step condition. The accuracy of the control subjects was 71 and 83 % for the RC and two-step condition, respectively. Accuracy in ALS patients was significantly lower than that in the control subjects, and the new visual stimuli significantly increased accuracy of ALS patients. Using the new speller, two ALS patients showed an initial accuracy sufficient for practical use (>70 %). The other two ALS patients, who performed better in the first trial using the new speller, continued to experience the BCI system, and their mean accuracy increased to 92 %. The two-step procedure for the visual P300-BCI system provided significantly increased accuracy for ALS patients compared with a conventional RC speller [40].
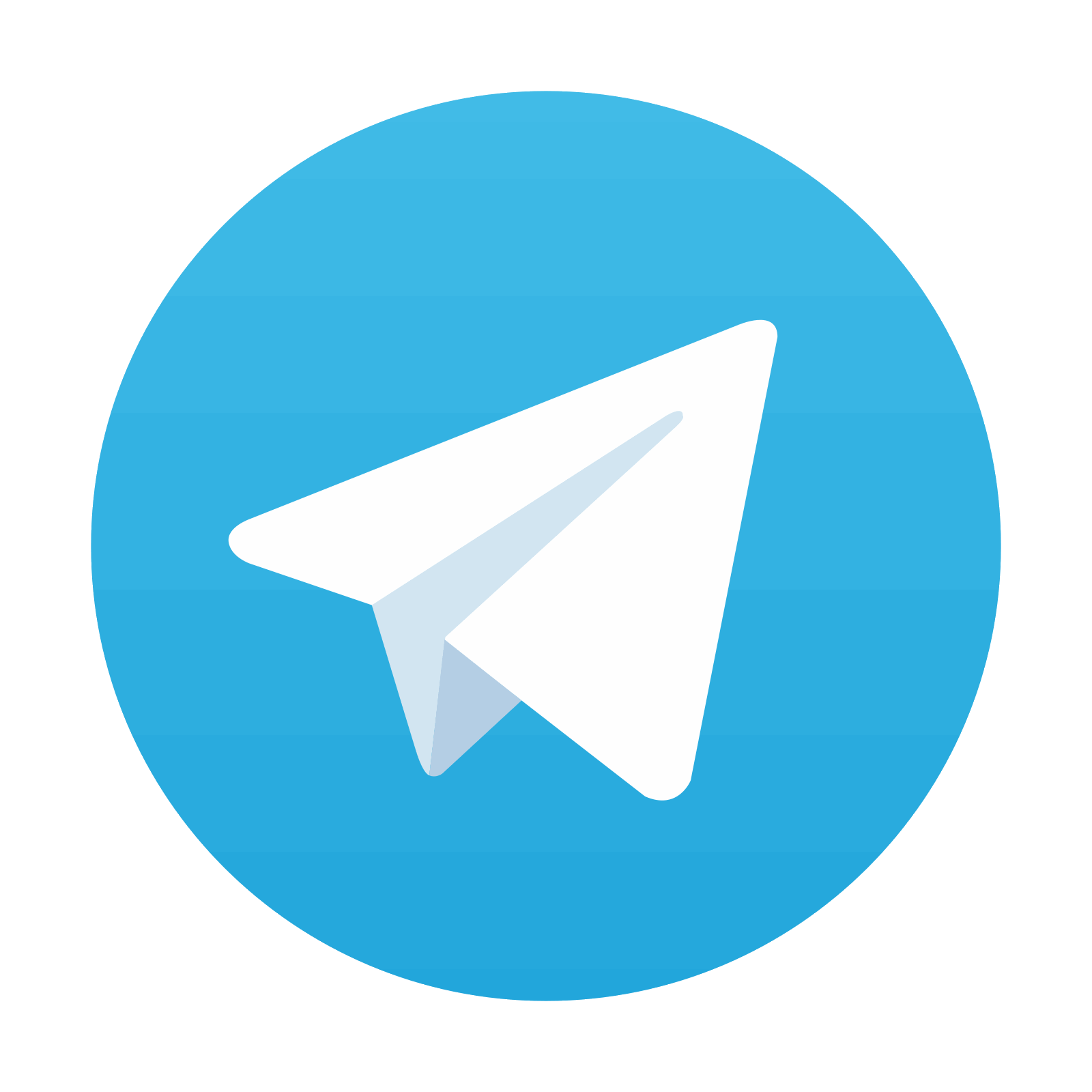
Stay updated, free articles. Join our Telegram channel

Full access? Get Clinical Tree
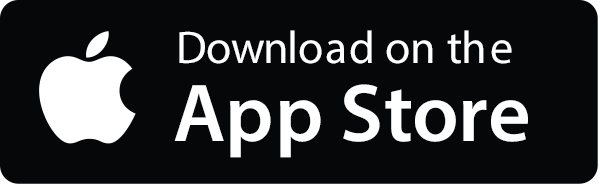
