3199 Quantitative EEG in the Pediatric Intensive Care Unit LEARNING OBJECTIVES • Provide a basic overview of the principles of quantitative EEG • Recognize common qEEG background and seizure patterns and distinguish these from artifact • Appreciate the strengths and limitations of qEEG in seizure detection • Understand the role of qEEG in the detection of cerebral ischemia Introduction Quantitative EEG (qEEG) is an emerging technology that has the potential to enhance the real-time cerebral monitoring of critically ill children. qEEG relies on mathematical principles and analytic techniques to decompose the raw EEG signal into its fundamental components (e.g., frequency and amplitude). The signal is then transformed and compressed, enabling the display of hours’ worth of qEEG data on a single screen. This, in turn, facilitates the rapid identification of seizures and EEG background changes. In this chapter, we examine the basic principles and clinical applications of qEEG. Basic Principles of qEEG Quantitative EEG uses mathematical principles and computational techniques to graphically display information derived from the raw EEG. These graphical displays are often referred to as “trends” and the use of qEEG is commonly called “trending.” qEEG trends can be incorporated into a vendor’s own EEG review software or displayed using a separate qEEG software package. Using qEEG trends alongside raw EEG review conveys several advantages. First, by compressing the time scale, it is possible to visualize long periods of EEG data on a single screen. Typically, one “screen” of raw EEG contains 10–15 s of information, while one screen of qEEG data can encompass hours, or even days, of recording. This time-compressed display is useful when rapidly screening for seizures as well as when evaluating background features (e.g., state change/variability, symmetry, and amplitude, among others). Second, a variety of different qEEG trends can be displayed simultaneously as a “panel,” which allows concurrent visualization of various aspects of the EEG tracing (Figure 9.1), and the choice of trends that make up a panel can be tailored to the clinical concern. Finally, trends can be displayed at the bedside and can be interpreted by nonneurophysiologists after a brief training,1–8 enabling real-time, near-continuous cerebral monitoring. Introduction to the Fourier Principle and Power Understanding quantitative EEG trends requires a familiarity with two basic principles: the Fourier principle and power. The Fourier principle states that any waveform can be broken down into component sine waves based on their frequency, allowing an analysis of each frequency band in the raw EEG. The Fourier principle tends to be most relevant in trends that display the EEG in terms of its frequency components. Power represents the area under the amplitude curve of each wave at a given frequency. This can be measured as absolute power (the power at a specific frequency) or relative power (the power at a specific frequency relative to the total power of a given epoch). The power at a given frequency can also be compared to other specific frequencies and calculated as a ratio (i.e., alpha to delta ratio [ADR]). Together, these two principles allow for the analysis of wave forms and their corresponding amplitudes at different frequencies (e.g., alpha, delta, beta, etc.), which may be altered by acute cerebral pathology. Figure 9.2 illustrates the Fourier principle and how this, along with the principle of power, is used to generate many of the most common trends used in critical care EEG monitoring. Quantitative EEG Trends Each trend focuses on a different EEG characteristic (Table 9.1). The most commonly used trends are based on time (e.g., amplitude-integrated EEG [aEEG], peak envelope, and suppression ratio) and frequency (e.g., compressed spectral array [CSA]/color density spectral array [CDSA]/fast Fourier transformation [FFT] spectrogram, rhythmicity spectrogram, power ratios, and asymmetry spectrogram). Time-based trends focus on how amplitude changes over time, while frequency-based trends examine the contribution of different frequencies over time. Time-Based Trends AMPLITUDE-INTEGRATED EEG (aEEG) The aEEG trend has been used extensively in the neonatal intensive care unit for both background assessment and seizure detection. This trend displays the amplitude of the filtered and rectified EEG signal as a function of time (Figure 9.3). The raw EEG signal is down-sampled to a rate of 64 samples/s and then filtered through a 60 Hz notch filter and an asymmetric bandpass filter. The asymmetric bandpass filter filters fluctuations in very slow (<2 Hz) and fast frequencies (>15–20 Hz). For frequencies between 2 and 15–20 Hz, the band-pass filter has a positive gradient to give equal weight to the lower amplitudes of higher frequency activity and the higher amplitudes of lower frequency activity.9 This filtered signal is then rectified (all values made positive), and output from 1 s epochs are plotted with time on the x-axis and amplitude on the y-axis, displayed as a linear scale from 0 to 10 µV and a logarithmic scale from 10 to 100 µV. The lower margin of the aEEG trace represents the minimum amplitude and the upper margin of the aEEG trace represents the maximum amplitude of the raw EEG signal over a discrete period of time (usually 10 or 15 s). A seizure typically appears on aEEG as an increase in the lower and sometimes upper margins of the trace (Figure 9.4). This is due to the fact that during a seizure, there is typically a loss of the mixed-frequency, lower-amplitude background activity as it is replaced by more monomorphic, higher-amplitude patterns. In addition to screening for seizures, amplitude-integrated EEG is also useful for assessing the EEG background, especially in neonates (see Chapter 8) (Figure 9.5). PEAK ENVELOPE (PE) The peak envelope trend displays the time-smoothed, band-passed filtered maximal amplitude as a function of time in order to provide a simplified depiction of the amplitude characteristics of the trace. The raw EEG is initially passed through an asymmetric band-pass filter (e.g., 2–20 Hz), then the maximum absolute amplitude (µV) for each one-second epoch is identified. A running average over a set epoch (often 10 s) is displayed on the y-axis with time on the x-axis. The left and right hemispheres may be displayed independently to evaluate for background asymmetry. The peak envelope trend 321can also be used to screen for seizures; focal seizures typically present with a unilateral increase in the peak envelope (Figure 9.6). SUPPRESSION RATIO The suppression ratio is a measure of the percentage of time during which the EEG is suppressed. This is displayed as a line with time on the x-axis and the suppression ratio on the y-axis, with a value ranging from 0% to 100%. qEEG algorithms typically define suppression as an amplitude of less than 5 μV, in contradistinction to the definition of suppression in the ACNS critical care EEG terminology, which defines suppression as an amplitude of less than 10 μV. The suppression ratio is typically used to monitor the degree of burst suppression and is not commonly used for seizure detection; however, increased suppression can often be seen in the post-ictal period (Figure 9.7). Frequency-Based Trends Frequency-based trends rely primarily on the Fourier principle described above. Mathematically, Fourier analysis is the process of decomposing a function into oscillatory components, sine waves in the case of EEG signals. This enables the analysis of the contribution of different frequency domains (e.g., delta, theta, alpha, beta, etc.) to the EEG signal as a whole. The area under the amplitude curve for any given frequency is the power. Frequency-based EEG trends display this calculated power in a variety of ways, as detailed below. POWER (COLOR) SPECTROGRAM The power spectrogram, also referred to as a compressed spectral array (CSA), color density spectral array (CDSA), or fast Fourier transformation (FFT) spectrogram, displays time on the x-axis, frequency on the y-axis, and power on the z-axis (Figure 9.8). The power is represented by warmer colors (yellows, reds, purples, and whites) typically representing higher power and cooler colors (blues and greens) representing lower power. Often, the power spectrogram is displayed independently for the left and right hemispheres as a means of evaluating symmetry. However, it is also possible to visualize the power spectrogram for a single channel, which can assist in the detection of focal seizures or other localized pathology. Seizures appear as an increase in power, usually at higher frequencies than the baseline background. There is typically a smooth, relatively gradual increase in power, corresponding to the incrementing onset of the seizure, followed by a smooth, relatively gradual decrease in power, corresponding to the decrementing offset of the seizure (Figure 9.9). This creates an arch or “flame” shape on the spectrogram. This signature can be subtle when a seizure is comprised of low-frequency discharges (Figure 9.10A) or when a seizure arises from an asymmetric background (Figure 9.10B). In addition to screening for seizures, the power spectrogram can be used to assess various features of the EEG background. For example, slowing will appear as an increase in power (warmer colors) at low frequencies, while a low-voltage background will appear as an absence of warm colors and a predominance of cool colors (Figure 9.11). RHYTHMICITY SPECTROGRAM This is a frequency-based trend similar to the power spectrogram. Time is displayed on the x-axis, frequency on the y-axis, and power on the z-axis as the density of blue coloration; dark blue represents high power while yellow represents low power (Figure 9.12). However, in contrast to the CDSA, the rhythmicity spectrogram only displays power in frequencies that display a “high degree” of rhythmicity. This trend is primarily used for seizure detection. As seizures tend to be highly rhythmic, they appear as areas of increased power. Seizure evolution is often well-visualized as an incrementing increase in power at progressively higher frequencies and/or a decrementing decrease in power at progressively lower frequencies (Figure 9.13). The rhythmicity spectrogram is proprietary and therefore, the mathematics used in its derivation are not published. RELATIVE ASYMMETRY INDEX AND SPECTROGRAM The relative asymmetry index and asymmetry spectrogram are similar to other frequency-based trends in that time is displayed on the x-axis and frequency on the y-axis. However, these trends incorporate an additional calculation that compares the power of homologous channels over the left and right hemispheres. Relative Asymmetry Index In the relative asymmetry index, if there is higher power on the left, the relative asymmetry index is negative, deflecting below a middle x-axis, while positivity implies greater power on the right (Figure 9.14). The values range between −50% and 50% but may be adjusted according to the properties of the EEG trace. Seizures appear as upward deflections if they occur over the right hemisphere and downward deflections if they occur over the left hemisphere. If there is a period of post-ictal suppression, the relative asymmetry index will appear as a deflection in the direction opposite to the deflection that occurred during the seizure; for example, a seizure over the left hemisphere will appear as a downward deflection of the trace while the ensuing period of post-ictal suppression will appear as an upward deflection, given that there is now relatively more power over the right than over the left hemisphere. The relative 322asymmetry index is primarily used to assess for background asymmetry. A symmetric background will appear as fluctuations around the middle x-axis. An asymmetric background will appear as a sustained deflection above or below the middle x-axis depending on which hemisphere has higher power. Asymmetry Spectrogram When displayed as a spectrogram, this trend uses the intensity of red or blue coloration to correspond to absolute power over the right or left hemisphere, respectively (Figure 9.15). Progressively darker shades of red and blue indicate greater degrees of asymmetry. A symmetric background appears as a mixture of lightly saturated colors, while an asymmetric background appears either red or blue with the darkness of the coloration corresponding to the degree of asymmetry (Figure 9.16). Focal seizures appear as an increase in either red or blue coloration depending on the location of the seizure. This may be followed by an increase in the opposite color due to post-ictal suppression causing a relative decrease in power in the same location from which the seizure arose. Like the relative asymmetry index, the asymmetry spectrogram is used to assess for background asymmetry, which, as noted above, appears as either dark red or blue, depending on which hemisphere has greater power. POWER RATIOS Delta/Alpha Ratio The delta/alpha ratio (DAR) is the ratio of power in the delta frequency band (1–4 Hz) to power in the alpha frequency band (8–13 Hz) (Figure 9.17). This is also commonly displayed as the alpha/delta ratio (ADR). Time is displayed on the x-axis and the power ratio is displayed on the y-axis. As opposed to the power spectrogram and asymmetry spectrogram, the DAR or ADR is displayed as a line graph and not a spectrogram, although the values are also based on Fourier domain analysis. Typically, this trend is used to evaluate for changes in background activity (Figure 9.18) and not to screen for seizures. The left and right hemispheres can be displayed independently to help visualize asymmetries. Trend Panels Each trend displays unique information that informs our understanding of a patient’s EEG. However, the trends, for the most part, are not meant to be used in isolation. They provide complimentary information and their full potential is realized when they are used together as a panel of trends, whether for assessing the EEG background (Figures 9.19, 9.20, and 9.21) or screening for seizures (Figures 9.22 and 9.23; see also below). Artifacts One of the greatest challenges in using quantitative EEG in the ICU is distinguishing artifacts from seizures and other abnormalities. One of the most straightforward ways to identify artifacts is by their abrupt onset and offset. While seizures, as noted above, typically have an incrementing onset and a decrementing offset that result in relatively smooth or gradual changes in amplitude or power, artifacts tend to occur abruptly and often have a ragged, rather than smooth appearance (Figure 9.24). However, despite this difference, it is easy to mistake artifact for seizure. Whenever a seizure or other abnormality is suspected based on a qEEG trend, the finding should be confirmed with the raw EEG. Additionally, qEEG software packages typically include artifact reduction algorithms for the removal or reduction of common artifacts, for example, muscle activity, eye movements, and 60 Hz artifact (Figure 9.25). Clinical Applications of qEEG Trends Seizure Detection The most common use of qEEG in the ICU is seizure detection. The goal of qEEG in this context is to increase the efficiency and accuracy of seizure identification and quantification by both neurophysiologists and bedside providers. Several different qEEG displays may be used to aid in seizure detection, with the power spectrogram, aEEG, and rhythmicity spectrogram being the most commonly used trends for this purpose. In keeping with the goal of improving the efficiency of seizure identification, one study found that qEEG-guided review of the raw EEG was able to shorten review times by 78%.10 Another study reported a time savings of 23.7% when qEEG was used to guide raw EEG review and 69% when qEEG was reviewed alone (although qEEG alone, without raw EEG review, resulted in a reduced sensitivity for seizure detection).11 In this study, a median of 19 min was required to review a 6-h epoch of raw EEG alone, a median of 14.5 min was required when qEEG was used to guide raw EEG review, and 6 min was required when qEEG was used alone. The ability to screen for seizures using various trends also has applications for EEG screening by non-neurophysiologists (Table 9.2). Numerous studies have assessed the ability of various types of adult providers, including neurology trainees, EEG technologists, critical care attendings and fellows, and critical care nurses, to identify seizures using qEEG trends in a simulated setting after a brief training.1,2,4,7 The majority of these studies used the CDSA with or without the aEEG. Sensitivity and specificity for seizure detection ranged from 0.66 to 0.92 and 0.38 to 0.95, respectively, with no consistent differences 323based on provider type. Three studies have also assessed the ability of providers in the pediatric intensive care unit to use the power spectrogram with or without the aEEG to screen for seizures in a simulated setting.3,5,8 In the pediatric ICU, critical care nurses, fellows, and attending physicians used a CDSA to evaluate for seizures following cardiac arrest; the sensitivity for seizure detection was 64%, 72%, and 78%, respectively.3 Another study of qEEG after cardiac arrest interpreted by pediatric critical care nurses, fellows, and attending physicians reported similar sensitivities and specificities and also incorporated aEEG review; in this study, addition of the aEEG to the CDSA did not improve sensitivity or specificity.8 The third study performed in the pediatric setting compared the ability of pediatric ICU fellows, nurses, neurophysiologists, and EEG technologists to identify seizures using a CDSA and aEEG.5 Sensitivities were similar using the aEEG for ICU fellows, nurses, and neurophysiologists (73–83%), but lower for EEG technologists (66.7%). Sensitivity was statistically similar for all providers when using the CDSA (73–88%) to screen for seizures. All of the studies described above incorporated training modules of modest duration in a simulated setting. While these studies demonstrated reasonably good performance for identifying seizures, from a practical standpoint, we may be able to increase the sensitivity in the real-world clinical setting if the bedside provider were to receive real-time training on a specific patient’s EEG. This approach was undertaken by Kang et al.6 Adult patients with nonconvulsive status epilepticus were identified and their seizure signature on aEEG and a rhythmicity spectrogram was identified. Their bedside nurse was then given brief bedside training on the use of quantitative EEG to screen for seizures, tailored to their patient’s seizure signature. The ability of the bedside nurses to identify seizures on subsequent 1-h EEG epochs was then analyzed. The sensitivity was 85.1% (95% CI, 71.1–93.1%) and the specificity was 89.8% (95% CI, 82.8–94.2%) for the detection of seizures for each 1-h block when compared to interpretation of the conventional EEG by two neurophysiologists. While tailoring the training to a specific patient’s EEG may be useful in identifying recurrent seizures in a patient with known nonconvulsive seizures, this approach would not allow for the identification of new-onset nonconvulsive status epilepticus by bedside providers. Of note, aEEG as a screening tool for the detection of seizures in neonates has been studied much more extensively in real-world settings and has been reported to have sensitivity as low as 38% for users with less than one year of experience with aEEG.12–15 325Seizure Detection Algorithms Seizure detection algorithms have been the subject of active investigation for several decades. Over forty years ago, Jean Gotman began experimenting with automated ictal and interictal detection and quantification.16,17 Since then, there have been a number of automated systems and machine learning algorithms developed to identify seizures.18–23 EEG trending software packages, including Persyst (Solana Beach, CA), Nihon Kohden (Irving, CA), Natus (Pleasanton, CA), and others, include proprietary seizure-detection algorithms that are frequently updated as technology improves. To date, however, all of these methods face the same challenges that Gotman described 40 years ago, including differentiating seizures from artifact and non-ictal rhythmicity. In studies performed in adults, automated seizure detectors tend to identify generalized seizures more accurately than focal seizures. For example, in a recent study of electrographic seizures in adults, an automated seizure detector identified at least one seizure in approximately half of the patients (53%) with seizures, including 70% of those with generalized seizures and 46% of those with focal seizures.24 Seizures in pediatric patients tend to be more heterogeneous than those seen in adults, including single-channel seizures in neonates and complicated multifocal epilepsies in older children, creating additional challenges for automating seizure detection. Ischemia Detection In addition to seizure identification, qEEG can be used for the real-time detection of evolving cerebral ischemia. The utility of qEEG in ischemia detection is based on the brain’s response to ischemic injury (Figure 9.25).25 The EEG signal is based on a summation of excitatory and inhibitory postsynaptic potentials generated primarily by pyramidal neurons. Slower frequency (delta and theta range) activity is generated primarily by cells in cortical layers 2–5, while faster frequencies (alpha and beta range) derive from cells in cortical layers 5 and 6.26 Pyramidal neurons in cortical layers 3, 5, and 6 are especially sensitive to ischemic injury. Hypoperfusion results in alterations of synaptic function with the level of cellular disruption dependent on the severity of ischemia.27 This change in synaptic function appears on EEG as a loss of faster frequency (alpha and beta range) activity followed by an increase in slower frequency (theta and delta range) activity, and the change in the EEG can be correlated with the rate of cerebral blood flow.28 The initial change in the EEG, the loss of faster (beta and alpha range) activity (Figure 9.26) is seen at a cerebral blood flow (CBF) of approximately 25–35 mL/100 g/min. At a CBF of 18–25 mL/100 g/min, there is an increase in 4–7 Hz theta activity, which is followed by an increase in 1–4 Hz delta activity as the CBF falls to 12–18 mL/100 g/min. At this point, the brain tissue may remain viable if the ischemia is reversed in a timely fashion. However, as the CBF continues to fall below 10 mL/100 g/min, the EEG becomes suppressed, indicating irreversible injury (Figure 9.27). These principles have been applied primarily to the detection of delayed cerebral ischemia (DCI) in adult patients with subarachnoid hemorrhage (SAH), and the American Clinical Neurophysiology Society (ACNS) recognizes the detection of ischemia as an indication for the use of cEEG in the ICU.29 Several qEEG patterns have been examined to determine which is the most sensitive for the detection of cerebral ischemia. Among these are: DAR; alpha/delta ratio; (delta+theta)/(alpha+beta) ratio (DTABR); alpha variability; relative power of delta, theta, alpha, and beta activity; and QSLOWING (ratio of summed power across 1.95–7.81 Hz versus summed power across 1.95–24.90 Hz). The alpha/delta ratio (ADR) is the most frequently used parameter for the detection of DCI. Rots et al. used a limited montage of seven electrodes and examined thirteen qEEG indices to predict cerebral ischemia in patients with aneurysmal SAH.30 By using a combination of ADR and alpha variability, the authors were able to detect EEG changes seven hours before the clinical diagnosis of DCI, which, in theory, could lead to earlier intervention and improved outcome. An “EEG alarm” based on decreasing alpha variability and ADR, new slowing, and new or worsening epileptiform activity has been used to prospectively predict DCI.31 A recent meta-analysis confirmed the utility of decreasing ADR as a predictor of DCI in aneurysmal SAH; in this meta-analysis, the ADR had a sensitivity of 83% and specificity of 74% for the detection of DCI.32 Not surprisingly, the inverse of the ADR, the delta/alpha ratio (DAR), has also been found to be a robust marker of acute ischemia. In general, a DAR below 1 is considered normal, while a value above 2 is likely to be abnormal. A cut-off threshold of 3.7 showed 100% sensitivity and 100% specificity for discriminating between patients with radiographically confirmed acute ischemic stroke and controls.33 The relationship between alpha and delta power has also been described in a case report as a useful indicator of successful reperfusion following administration of intravenous recombinant tissue plasminogen activator (r-tPA) for acute ischemic stroke.34 Although clinical applications of quantitative EEG for detection of ischemia have not been systematically studied in pediatrics, at the authors’ institution, it is routine to monitor patients undergoing encephalo-duro-arterio-synangiosis (EDAS) for surgical revascularization of moyamoya both intra-operatively and in the acute postoperative period with continuous EEG. While conventional EEG has been examined for use both in diagnosis and follow-up of moyamoya patients,35 we have found DAR to be a useful measure of postoperative cerebral perfusion and have used this to help determine mean arterial pressure (MAP) goals (Figure 9.28). At this time, it remains to be determined whether the relevant parameters for ischemia detection differ 326based on a patient’s age, as the youngest children have very little alpha activity at baseline. This may make parameters such as total power or theta to delta power more relevant than alpha to delta power.36 Prognostication In addition to providing unique insight into features of the EEG background, qEEG is being investigated for its ability to contribute to outcome prediction. Several studies in children have used raw EEG background features to aid in prognostication after cardiac arrest.37–40 A recent study applied mathematical modeling to qEEG data from pediatric cardiac arrest patients and found that a model incorporating specific qEEG features could be used to predict a favorable outcome with a positive predictive value of 0.79.41 Moreover, in infants with hypoxic-ischemic encephalopathy, lower total spectral power correlates with more severe injury on brain MRI, similar to a suppressed aEEG.42 Several advances have recently been made in qEEG-based prognostication in adults following cardiac arrest and other forms of anoxic brain injury. A novel Cerebral Recovery Index (CRI), based on five qEEG features (power, Shannon entropy, alpha-delta ratio, a measure of EEG continuity, and coherence in the delta band) at 24 h post-arrest, has been shown to predict poor outcome with 100% specificity.43 More recently, a background continuity index and a ratio of the amplitude of the bursts to the suppressed periods in a burst-suppression pattern at 12 h post-arrest predicted a poor outcome with 100% specificity.44 qEEG patterns with poor spectral variability have also been associated with poor outcome45 with a low false positive rate (15%).46 A number of additional studies have also used spectral power to predict outcomes following cardiac arrest,45 subarachnoid hemorrhage,47 and TBI.48 Frequency ratios also have prognostic value in assessing outcome after ischemic stroke. Using an awake EEG recording approximately 2 days following symptom onset, Finnigan et al. examined qEEG predictors of 30-day National Institutes of Health Stroke Scale (NIHSS) scores.49 They found that DAR, relative alpha power, and 48 h NIHSS score were significantly correlated with 30-day NIHSS, suggesting that these measures could aid in prognostication. Schleiger at al. evaluated the use of frontal DAR in predicting cognitive outcome after middle cerebral artery stroke.50 They reported that DAR calculated from electrodes F3, F7, F4, and F8 and global relative alpha power on an EEG done 62–101 h following symptom onset were significantly correlated with the functional independence measure and functional assessment measure (FIM-FAM) administered approximately 3.5 months later. Other Clinical Applications In addition to the above clinical applications, patterns may be seen on qEEG that are not readily appreciated on raw EEG. These patterns may be related to recurrent seizures, increased intracranial pressure, or other pathologic processes (Figure 9.29). Conclusion qEEG is an emerging tool in pediatric critical care that is complementary to raw EEG review and provides unique insight into the cerebral function of critically ill children. Future research is necessary for clinical application in children and should include collaboration with critical care providers, bioengineers, and computational neuroscientists so as to maximize the utility of this valuable technology. This chapter includes access to a video, Video 9.1 (Quantitative EEG), with real time EEG that illustrates concepts and patterns. Please follow the following url and click onto the show chapter supplementary tab to access the video: http://connect.springerpub.com/content/book/978-0-8261-4867-4/chapter/ch09 Disclaimer Figures are displayed using the QEEG software packages from Nihon Koden (Tokyo, Japan), Persyst (Solana Beach, CA) and Natus (Pleasanton, CA). The authors’ home institutions have site licenses for these products. The authors have no relationship with these companies and do not specifically endorse any one product. KEY POINTS • The fast Fourier transformation divides EEG into its component frequencies, allowing for the assessment of each over time • Power is the area under the curve of the amplitude of individual frequencies in the EEG and may be calculated as an absolute or relative value • qEEG is a sensitive tool for seizure detection • qEEG has a role beyond seizure detection, allowing for real-time assessment of EEG background, including acute changes that may be seen in ischemia Figures FIGURE 9.1. qEEG panel. Five hours of normal qEEG from a 13-year-old patient are displayed here. A panel can be created to incorporate various trends depending on the clinical scenario and may include seizure-probability and seizure-detection algorithms. The first trend is artifact intensity (A), which monitors eye and muscle movement. This can be helpful in evaluating state change and in identifying periods of the tracing that might be obscured by artifact. The next two trends are the rhythmicity spectrogram (B) and the color density spectral array (CDSA) (C). In these two trends, the left hemisphere is shown over the right. These are followed by the relative asymmetry spectrogram (D), the amplitude-integrated EEG (aEEG) (E), the suppression ratio (SR) (F), and alpha/delta ratio (ADR) which can also be displayed as delta alpha ratio (DAR) (G). For these trends, the left hemisphere is shown in blue and the right hemisphere is shown in red. Time is on the x–axis; in this panel, approximately 5 hours of data are displayed with vertical purple lines demarcating each 1-hour epoch. The y-axis is specific to each trend. The rhythmicity spectrogram, color density spectral array, and asymmetry spectrogram, which are frequency-based trends, display frequency along the y-axis, with power along the z-axis (*). The ADR or DAR is also a frequency-based trend; in this case, the y-axis represents the square root of the power ratio, which ranges from 0 to 2. The aEEG, in contrast, is a time-based trend with amplitude on the y-axis (0–10 μV as a linear scale and 10–100 μV as a logarithmic scale). The SR is also a time-based trend that displays percent suppression on the y-axis. Source: Image courtesy of Arnold Sansevere. FIGURE 9.2. Fourier principle and power. Schematic representation of the conversion of the raw EEG signal into a color density spectral array. Using the mathematical technique fast Fourier transform, raw EEG signals, consisting of fast (blue) and slow (red) frequencies from an arbitrary channel, are separated into their respective frequency components. The power, or area under the amplitude curve, is calculated for each frequency and can be represented graphically (blue and red bars) in the absence of the time domain. This graphical depiction demonstrates a higher power of the slow-frequency component (red tracing and bar) and a lower power of the fast-frequency component (blue tracing and bar). The power can be displayed over time as a color density spectral array with time on the x-axis and frequency on the y-axis. The power is displayed on the z-axis with warmer colors representing higher power and cooler colors lower power. FIGURE 9.3. Amplitude-integrated EEG (aEEG). The aEEG is a time-based trend with time displayed on the x-axis and amplitude on the y-axis. Amplitude is linear from 1 to 10 μV and logarithmic from 10 to 100 μV. The left (blue) and right (red) hemispheres are superimposed in this aEEG but can also be displayed separately. Source: Image courtesy of Arnold Sansevere. FIGURE 9.4. Seizures on aEEG. Seizures on aEEG manifest as an increase in the lower margin of the trace. There is also often a rise in the upper margin of the trace depending on the amplitude of the seizure. (A) In this trend, the left and right hemispheres are displayed independently, and the upper and lower margins of the trace on the left are denoted by blue arrows and on the right by red arrows. There are multiple seizures on theleft and right, a subset of which are highlighted by a *. A rise in both the upper (solid black arrows) and lower margins (dotted arrows) of the trace are seen during the seizures. No further seizures are seen after the time point denoted by the green arrow. (B) In this trend, the activity over left and right hemispheres is superimposed. There are multiple seizures over both hemispheres, a subset of which are highlighted by a *. The last 2 hours are enlarged in the inset shown above the main trend. The rise in the lower margin of the trace over both the left and right hemispheres is denoted by solid black arrows in the inset, while the rise in the upper margin is denoted by dotted black arrows. The baseline upper and lower margins of the trace are difficult to delineate given the presence of frequent seizures. Source: (A) Image courtesy of Dana Harrar; (B) Image courtesy of Arnold Sansevere. FIGURE 9.5. State change on aEEG. (A) Normal aEEG from a 1-day old term neonate monitored for characterization of events. This 5-hour panel shows the average aEEG with the right and left hemispheres overlapping (top trend) as well as the aEEG of the left (blue) and right (red) central-parietal regions. Sleep-wake cycling is seen as fluctuations in the amplitude and width of the aEEG tracing, corresponding to continuous and discontinuous (quiet sleep) portions of the tracing. (B) The trace in B is from a similar-aged neonate as in 9.5A but lacks the fluctuations seen in 9.5A, suggesting a paucity of sleep-wake cycling. FIGURE 9.6. Peak envelope trend. The envelope trend, like the aEEG, is a time-based trend. The average peak amplitude of the raw EEG over a 10–20 s epoch is plotted along the y-axis with time along the x-axis. This is in contrast to the aEEG, in which the minimum and maximum amplitude of the raw EEG are plotted over time. A seizure appears as an upward deflection of the peak envelope (black arrow). Here we see the upward deflection on the right (red) preceding the upward deflection on the left (blue), indicating that the seizure is arising from the right hemisphere. The data from the two hemispheres may be superimposed, as in this example, or displayed separately. The peak envelope for individual EEG channels of interest can also be displayed. Source: Image courtesy of Arnold Sansevere. FIGURE 9.7. Suppression ratio (SR). The suppression ratio, like the aEEG and peak envelope, is a time-based trend. Time is displayed on the x-axis with percent suppression on the y-axis. The left hemisphere is represented by a blue line and the right hemisphere by a red line. Zero percent suppression should be seen in a normal study. The tracing from an 18-year-old with epilepsy and hepatic failure is shown here. The SR shows a transient rise to approximately 50% during a post-ictal period (*). There is then a brief return to baseline (#) prior to a slow increase in the percent suppression that is initially more prominent over the right (red arrow) as compared to the left (blue arrow) hemisphere. Imaging showed cerebral edema. Source: Image courtesy of Arnold Sansevere. FIGURE 9.8. Color density spectral array. The CDSA, also referred to as the CSA (color spectral array) and FFT (Fast Fourier Transform) spectrogram, is a frequency-based trend displayed as time on the x-axis, frequency on the y-axis, and power on the z-axis with cooler colors representing lower power and warmer colors representing higher power. Source: Image courtesy of Arnold Sansevere. FIGURE 9.9. Seizures on color density spectral array. Seizures appear on the CDSA as an increase in warmer colors evolving from lower to higher frequencies. This may resemble a “flame.” When seizures are suspected, the trend should be compared to the raw EEG. The seizure signature on the CDSA is readily apparent (a subset of seizures marked by a *) and is highlighted on the panel to the right. The curved arrows highlight the gradual increase in warmer colors toward higher frequencies (upward arrow) followed by a gradual return to cooler colors (downward arrow). Note that the background appears symmetric, with the complement of warm and cool colors distributed equally over the left and right hemispheres (black brackets). Source: Image courtesy of Dana Harrar. FIGURE 9.10. Subtle seizures and background asymmetry on color density spectral array. (A) In contrast to FIGURE 9.9, in this panel, there is an asymmetry in the EEG background. The lower trend, representing the right hemisphere, has warmer colors (red, pink) at lower frequencies (solid black box), while the upper trend, representing the left hemisphere, has relatively cooler colors (green) at lower frequencies (dashed black box). This corresponds to higher amplitude waves at low frequencies over the right as compared to the left hemisphere. On the right half of the lower trend, very warm colors (white, purple) are seen recurring at low frequencies (vertical black arrows). When the raw EEG corresponding to one of these areas is reviewed, we see evolving low-frequency spikes (1 Hz) in the right central region (C4) (black arrow on raw EEG trace). The qEEG pattern is subtle and when such a pattern is seen, comparison should be made with the raw EEG for clarification. (B) The same trend as in 9.10A is displayed, this time highlighting the subtle flames seen toward the end of the trend (black arrows). The seizure on the far right of the CDSA (black box) is highlighted and enlarged in the inset above, allowing us to better visualize the subtle flame shape of the seizure. The raw EEG shows evolving 2 Hz spikes over the left (C3) and right (F4) hemispheres corresponding to the subtle flame in the box and inset. The channels that appear pale gray on the raw EEG have been minimized by artifact reduction. Source: Image courtesy of Arnold Sansevere. FIGURE 9.11. Low-voltage background on CDSA. A low-voltage background is seen on the CDSA as cool colors (low power) throughout. Source: Image courtesy of Arnold Sansevere. FIGURE 9.12. Rhythmicity spectrogram. Like the CDSA, the rhythmicity spectrogram is a frequency-based trend. Time is displayed on the x-axis, frequency on the y-axis, and power on the z-axis as the density of blue coloration; dark blue represents high power while yellow represents low power. In contrast to the CDSA, the rhythmicity spectrogram only displays power in frequencies that display a “high degree” of rhythmicity. Source: Image courtesy of Arnold Sansevere. FIGURE 9.13. Seizures on the rhythmicity spectrogram. The rhythmicity spectrogram is primarily used for seizure detection. As seizures tend to be highly rhythmic, they appear as areas of increased power. Seizure evolution is often well-visualized as an incrementing increase in power at progressively higher frequencies and/or a decrementing decrease in power at progressively lower frequencies. (A) A left-sided seizure is highlighted (black box) and enlarged in the inset on the right side of the page. The black arrow in the inset demarcates the gradual increase in power (dark color) from lower to higher frequencies as the seizure onsets. The rhythmicity spectrogram is primarily used for seizure detection. As seizures tend to be highly rhythmic, they appear as areas of increased power. Seizure evolution is often well-visualized as an incrementing increase in power at progressively higher frequencies and/or a decrementing decrease in power at progressively lower frequencies. (B) A continuation of the recording shown in 9.13A is shown. In this trend, more frequent seizures are seen. A right- > left-sided seizure is highlighted (black box) and enlarged in the inset on the right side of the page. The onset of the seizure is seen as an incrementing increase in power at progressively higher frequencies (left arrow), while the offset of the seizure is seen as a downslope of power from relatively higher to lower frequencies (right arrow). The rhythmicity spectrogram is primarily used for seizure detection. As seizures tend to be highly rhythmic, they appear as areas of increased power. Seizure evolution is often well-visualized as an incrementing increase in power at progressively higher frequencies and/or a decrementing decrease in power at progressively lower frequencies. (C) More subtle seizures than those seen in 9.13A, B are highlighted by the black arrows and enlarged in the inset above the main trend. As the seizure onsets, there is an incrementing increase in power at progressively higher frequencies. Source: Image courtesy of Arnold Sansevere. FIGURE 9.14. Relative asymmetry index. Like the CDSA and rhythmicity spectrogram, the relative asymmetry index is a frequency-based trend. Power between homologous regions is compared and displayed over time. Deflections below a center axis indicate higher power on the left, while upward deflections indicate higher power on the right. A symmetric background will appear as fluctuations around the middle axis. An asymmetric background will appear as a sustained deflection above or below the middle axis depending on which hemisphere has higher power. In contrast, the absolute asymmetry index (not shown) displays the absolute difference between homologous regions as a positive value. Source: Image courtesy of Arnold Sansevere. FIGURE 9.15. Asymmetry spectrogram. Like the CDSA, rhythmicity spectrogram, and relative asymmetry index, the asymmetry spectrogram is a frequency-based trend. Time is displayed on the x-axis, frequency from 1 to 18 Hz on the y-axis, and power on the z-axis. Higher power over the left hemisphere appears dark blue, while higher power over the right hemisphere appears dark red. This trend may be used to detect background asymmetries as well as seizures. Source: Image courtesy of Arnold Sansevere. FIGURE 9.16. Alternating hemiparesis on asymmetry spectrogram and relative asymmetry index. Asymmetry spectrogram showing waxing and waning asymmetry in a 9-year-old with alternating hemiparesis. The asymmetry spectrogram demonstrates recurring increases in power on the right at high frequencies and on the left at low frequencies (black brackets) that co-occurred with clinical episodes of right hemiparesis. This is also demonstrated on the relative asymmetry index as sustained deflections below the center axis at 1–5 Hz. Note that between episodes, overall power is higher over the left than over the right, but this is distributed across all frequencies. FIGURE 9.17. Power ratio: ADR and DAR. Like the CDSA, rhythmicity spectrogram, relative asymmetry index, and asymmetry spectrogram, the power ratio is a frequency-based trend. The ratio of the absolute power of alpha frequencies divided by the absolute power of delta frequencies is represented by the ADR, the inverse of which is displayed as the DAR. Time is displayed on the x-axis, while the y-axis represents the square root of the power ratio, with values ranging between 0 and 2. The ADR (or DAR) over the left hemisphere is represented by a blue line, while the right hemisphere is represented by a red line. In a normal symmetric tracing, as shown here, the red and blue lines are superimposed. A separation between the lines may indicate unilateral injury, either intraaxial or extraaxial. In early stages of ischemia, there is a decrease in alpha power, resulting in a decrease in the ADR (or an increase in the DAR). As ischemia worsens, there is an increase in delta power, resulting in a further decrease in the ADR (or increase in the DAR). Source: Image courtesy of Arnold Sansevere. FIGURE 9.18. ADR in acute ischemic stroke. The ADR from a 13-year-old female with laryngospasm and cardiac arrest after repair of an atlantoaxial dislocation is shown. After weaning of sedation and discontinuation of neuromuscular blockade, she was noted to have left-sided weakness. MRI showed a right-sided infarct. This was accompanied by a decrease in the ADR on the right (red arrows). cEEG confirmed right frontotemporal slowing. Source: Image courtesy of Arnold Sansevere. FIGURE 9.19. Trend panel showing normal awake, drowsy, and sleep features on qEEG. (A) Awake. (i) At the beginning of the recording, the patient is awake. An increase in artifact intensity can be seen in the muscle (green) and eye channels (blue and red). The EEG corresponding to the time of the vertical purple line on the qEEG panel shows eye blinks (red arrow) and muscle (blue arrow) most prominent in the temporal regions. (ii) There is an abrupt increase in power on the rhythmicity spectrogram and CDSA in the middle of the page (black circle). This is accompanied by an increase in the amplitude of the aEEG (black arrow). The corresponding raw EEG shows muscle artifact caused by chewing movements. (B) Drowsy. The muscle artifact and eye movement artifact begins to dissipate (black arrow), and there is an increase in cooler colors at lower frequencies (black circle). The corresponding EEG shows drowsy bursts (red bracket) with some preserved muscle artifact (black bracket). (C) Sleep. (i) Stage 1 sleep is shown and is marked by the presence of vertex waves (black bracket). The artifact intensity shows the early drop out of muscle, while the CDSA shows that there has not yet been a transition to higher power at lower frequencies. These features on the qEEG suggest an earlier stage of sleep. (ii) Stage 2 sleep is marked by sleep spindles (red arrows on the raw EEG), which are highlighted on both the rhythmicity spectrogram and CDSA by prominent activity at 14 Hz (black circles). (iii) In deeper stages of sleep, there is an increase in power in the delta range, seen as warmer colors on the CDSA (black box) and as increased rhythmicity at low frequency on the rhythmicity spectrogram (black box). Source: Image courtesy of Arnold Sansevere. FIGURE 9.20. Trend panel showing burst suppression. A 4-year-old male with congenital heart disease suffered a cardiac arrest requiring cannulation to ECMO. A burst-suppression pattern was seen on qEEG. The rhythmicity spectrogram shows rhythmic 1–10 Hz bursts of activity. On the CDSA, the bursts of activity appear as high power (warm colors) at low-to-medium frequencies, while the paucity of activity seen during the interburst intervals appears as periods of low power (cool colors). The aEEG shows the typical pattern for burst suppression, with brief periods of moderate to high amplitude activity alternating with periods of suppression. This pattern has been likened to a city skyline. The suppression ratio fluctuates between 80% and 100% suppression. Source: Image courtesy of Arnold Sansevere. FIGURE 9.21. Trend panel showing a low voltage tracing. A 22-month-old was cannulated to ECMO after heart transplant. At the start of the recording, the CDSA shows cooler colors over the right as compared to the left hemisphere, suggesting lower power over the right hemisphere. This is confirmed on the asymmetry spectrogram, which shows higher power over the left hemisphere, manifest as blue coloration. The aEEG also shows that the amplitude of the right hemisphere is lower amplitude than that of the left hemisphere. Over time, on the CDSA, there is a progressive loss of warmer colors (colored arrows), with the trend becoming dominated by cool colors, especially over the right hemisphere. This corresponds with the rise in the suppression ratio over the right more so than the left hemisphere and with the progressive decrease in amplitude on the aEEG, again seen more prominently on the right as compared to the left. The blue coloration of the asymmetry spectrogram also becomes darker as power decreases over the right hemisphere. Imaging showed right sided infarction and edema. Source: Image courtesy of Arnold Sansevere. FIGURE 9.22. Trend panels showing seizures. (A) A 4-year-old female with fever and meningismus presented with seizures and altered mental status. qEEG was notable for multiple seizures that were well-visualized on the rhythmicity spectrogram, CDSA, asymmetry spectrogram, and aEEG (black circles). These appear as increases in power at higher frequencies on the rhythmicity spectrogram and CDSA, as a relative increase in power over the right hemisphere when compared to the baseline on the asymmetry spectrogram, and as an increase in amplitude on the aEEG. The seizure is seen over both the right and left hemispheres. Of note, the background is asymmetric with higher power over the left hemisphere seen as warmer colors at low and medium frequencies on the CDSA; the higher power at low frequencies over the left as compared to the right hemisphere is highlighted by the black boxes (*). The higher power over the left hemisphere is seen as blue coloration on the asymmetry spectrogram. The amplitude is also higher over the left hemisphere as seen on the aEEG; the blue band, corresponding to activity over the left hemisphere, is higher in amplitude than the red band, corresponding to activity over the right hemisphere. (B) A 5-day-old male was cannulated to ECMO after undergoing truncus arteriosus repair. qEEG was notable for multifocal seizures comprised of evolving discharges of low frequency (2–3 Hz) and low amplitude (10 μV). At the beginning of the recording, these subtle seizures can be seen on the rhythmicity spectrogram (black arrow), CDSA (*), and aEEG (red arrows). The seizures become much more apparent as the recording progresses (black circle). By the end of the panel, there is more rhythmic activity at relatively higher frequencies (blue arrows) than seen during the early seizures. Of note, the background is discontinuous; this is best appreciated as the broad band of activity seen on the aEEG (black box). (C) A 10-month-old male presented with facial twitching and was found to have a right-sided intracranial hemorrhage. At the beginning of the recording, there are multiple seizures that are most prominent over the right hemisphere. The signature on CDSA is highlighted (black circle) and is associated with increased power at relatively higher frequencies on the right-sided rhythmicity spectrogram (black circle). The asymmetry spectrogram confirms a right-sided seizure; this appears as an increase in red coloration corresponding to higher power over the right hemisphere (thick black arrows). The prominence of the seizure over the right hemisphere is also apparent on the aEEG; the upper and lower margins of the right-sided aEEG trace (red) rise above the upper and lower margins of the left-sided aEEG trace (blue) (thin black arrow). These seizures are detected by the seizure detection and seizure probability algorithms shown on the top of the panel (black box). As the recording continues, a single left-sided seizure is seen toward the middle of the page. The signature on CDSA is highlighted (blue circle) and is associated with increased power at relatively higher frequencies on the left-sided rhythmicity spectrogram (blue circle). Now, the asymmetry spectrogram shows higher power on the left (blue arrows), and the upper and lower margins of the left-sided aEEG trace (blue) rise above the upper and lower margins of the right-sided aEEG trace (red) (thin blue arrow). Toward the end of the panel, there is seizure resolution (purple arrow). The asymmetry spectrogram shows higher power over the left hemisphere that is especially prominent in the delta range. This corresponds to post-ictal attenuation over the right hemisphere. (D) A term neonate with hypoxic ischemic encephalopathy had onset of frequent seizures as seen on the rhythmicity spectrogram, CDSA, asymmetry spectrogram, and aEEG (black circles). The top panel of the asymmetry spectrogram shows activity over the parasagittal regions while the bottom panel shows activity over the temporal regions. This helps localize the seizure to the temporal region as the asymmetry is seen most prominently over the temporal region (black circle). Seizures appear to resolve (purple arrow), and the background shows a burst-suppression pattern (*); however, subtle seizures continue to be seen on the aEEG and are highlighted by the black arrows. Source: Image courtesy of Arnold Sansevere. FIGURE 9.23. Trend panels showing status epilepticus. (A) Term neonate with refractory seizures. The CDSA is broken down by individual electrode pairs with the left-hemisphere channels labeled in blue and the right-hemisphere channels labeled in red. The CDSA seizure signature (red boxes) shows evolving increases in power at all frequencies on the CDSA. This is seen most prominently over the right hemisphere (Fp2-T4, T4-O2). The raw EEG confirms the presence of seizures maximal over the right centrotemporal region (C4/T6; T6 not shown on the CDSA). (B) A 13-month-old male with POLG1 presented with status epilepticus. This is best seen on the rhythmicity spectrogram, CDSA, and aEEG. There is high power at high frequencies on the left half of the rhythmicity spectrogram, corresponding to warm colors at high frequencies on the CDSA. The left half of the aEEG shows high amplitude activity over both the left and right hemispheres. Towards the latter half of the panel, the recording shifts to a low-voltage trace due to medication effect. The rhythmicity spectrogram shows a paucity of rhythmic activity (black arrow), the CDSA shows cooler colors at all frequencies (black arrow), the aEEG shows a drop in voltage (*), and the SR shows approximately 50% suppression over both hemispheres (**). (C) A 1-day-old born at 38 weeks with hypoxic ischemic encephalopathy treated with therapeutic hypothermia. Status epilepticus is seen towards the middle of the panel and is more prominent over the left as compared to the right hemisphere. (a) Rhythmicity spectrogram. During the seizure, there are darker colors at higher frequencies, and this is seen most prominently over the left hemisphere (black box). (b) CDSA. During the seizure, there is higher power (red/white) at higher frequencies over the left, more so than the right, hemisphere (black box). (c) Asymmetry spectrogram. There is higher power over the left hemisphere, manifest as a dark blue coloration of the spectrogram, during the seizure (black box). (d) Amplitude integrated EEG (aEEG). The amplitude is higher on the left, consistent with a seizure that is more prominent over the left as compared to the right hemisphere (black arrow). (e) Suppression ratio (SR). The * shows that after this prolonged seizure both hemispheres become approximately 50% suppressed. The blue line is above the red indicating that the left hemisphere is more suppressed than the right. Source: Image courtesy of Arnold Sansevere. FIGURE 9.24. Artifact. (A) Artifacts typically appear as a sudden increase in power at higher frequencies or in amplitude with no evolution. Artifacts often have a ragged, rather than smooth, appearance. This is in comparison to a seizure where increasing power evolves from lower to higher frequencies. In this figure, the black bracket labeled artifact shows a typical artifact signature displayed on the CDSA, aEEG, and corresponding raw EEG tracing. Common sources of artifacts in the ICU include electrode artifact, ventilator artifact, and patient movement. (B) Artifact reduction. In this term newborn with neonatal encephalopathy and persistent pulmonary hypertension, the raw EEG tracing (right) corresponds to the vertical purple line on the aEEG trace. This occurs during a high-amplitude deflection of the aEEG that resembles a seizure (black circles in upper panel). The raw cEEG shows significant myogenic artifact. Without artifact reduction enabled, myogenic artifact appreciated on the raw EEG resembles seizures on the aEEG. When artifact reduction is enabled on qEEG, the aEEG deflections are no longer seen (black circles in lower panel). Source: Image courtesy of Dana Harrar. FIGURE 9.25. Frequency and power as a function of cerebral blood flow. As cerebral blood flow decreases (x-axis), there is a concomitant decrease in fast frequency activity (y-axis) on EEG. On CDSA, this manifests as a decrease in warmer colors (less power) at faster frequencies. As cerebral blood flow continues to decrease, slower frequency activity (theta and delta) dominates the EEG. On CDSA, this appears as warmer colors (higher power) at slower frequencies. FIGURE 9.26. Trend panel showing early ischemia. A 6 year old male presented after a seizure and was found to have meningitis. (A) On the raw EEG, sleep spindles are seen over the right, more so than the left, hemisphere (black arrow). The paucity of spindles on the left manifests as a decrease in the ADR on the left due to the decrease in fast activity. This results in a split in the ADR between the left and right hemispheres (blue arrows). On the asymmetry spectrogram, there is higher power over the right hemisphere, particularly between 10 and 18 Hz (black circle), which is the expected frequency of sleep spindles (14 Hz). The background asymmetry is also seen on the relative asymmetry index (green plot in the lowest trend) as the majority of the activity is above the center axis, reflecting higher power over the right as compared to the left. This persistent asymmetry is also reflected in the absolute asymmetry index (yellow plot in the lowest trend), which shows a persistent asymmetry of approximately 40%. A 6-year-old male presented after a seizure and was found to have meningitis. (B) The same patient as in 9.26A is shown here. On the raw EEG, sleep spindles are again seen more prominently on the right (solid arrow) as compared to the left (dotted arrow). This spindle asymmetry can also be seen on the rhythmicity spectrogram. A signal is seen at approximately 14 Hz on the right (solid arrow) that is barely discernible on the left (dotted arrow). Repeat imaging showed sinous venous thrombosis and a corresponding left sided venous infarction. Source: Image courtesy of Arnold Sansevere. FIGURE 9.27. Trend panel showing an evolving infarction. (A) The first five hours of recording in a 3-month-old being monitored after cardiac arrest. Early in the recording, there is asymmetry with increased power in the right temporal region relative to the left temporal region (*), which improves after the first three hours of recording (black arrow). (B) Over the course of the next five hours, there is acute onset of marked asymmetry with decreased power over the left hemisphere, which appears on the asymmetry spectrogram as an increase in red coloration (black arrows). There is a corresponding increase in the DAR over the left hemisphere (blue) due to a loss of fast activity (black arrow). On the CDSA, there is a loss of warm colors on the left, corresponding to the decrease in power in this hemisphere (black box), while on the aEEG, there is a decrease in amplitude over the left hemisphere (black arrow). This raised concern for an acute ischemic event. (C) The patient’s CT scan showed a large area of hypodensity in the left middle cerebral artery territory (arrows). FIGURE 9.28. Trend panel showing monitoring after pial synangiosis. A five-hour panel from a six-year-old with moyamoya being monitored in the ICU immediately following right pial synangiosis is shown. Note the markedly asymmetric beta activity seen early in the study resulting from the residual effects of anesthesia (white *). The trends then return to baseline with a mild asymmetry that was present preoperatively. However, about 1.5 hours into the study, there is development of worsening asymmetry with voltage attenuation (black #) and a loss of power (white #) over the right hemisphere. This change was communicated to the ICU team, the mean arterial pressure was increased, and the EEG returned to baseline. FIGURE 9.29. Advanced trending and techniques. (A) A term neonate presented with abnormal movements of the right upper and lower extremity. CDSA was notable for a periodic increase in power in a low- to medium-frequency range (red line) preceding and admixed with seizures identified on raw EEG and CDSA (arrows). (B) qEEG may reveal periodic patterns not immediately apparent on raw EEG. A periodic increase in the delta/alpha ratio (arrow) is seen over the left hemisphere of a 3-year-old boy with a large left frontal intraparenchymal hemorrhage (arrows). This is followed by four seizures (#). Administration of hypertonic saline abolished the periodic increases in the DAR as well as the seizures. 371References 1.Dericioglu N, Yetim E, Bas DF, et al. Non-expert use of quantitative EEG displays for seizure identification in the adult neuro-intensive care unit. Epilepsy Res. 2015;109:48–56. 2.Swisher CB, White CR, Mace BE, et al. Diagnostic accuracy of electrographic seizure detection by neurophysiologists and non-neurophysiologists in the adult ICU using a panel of quantitative EEG trends. J Clin Neurophysiol. 2015;32:324–330. 3.Topjian AA, Fry M, Jawad AF, et al. Detection of electrographic seizures by critical care providers using color density spectral array after cardiac arrest is feasible. Pediatr Crit Care Med. 2015;16:461–467. 4.Amorim E, Williamson CA, Moura L, et al. Performance of spectrogram-based seizure identification of adult EEGs by critical care nurses and neurophysiologists. J Clin Neurophysiol. 2017;34:359–364. 5.Lalgudi Ganesan S, Stewart CP, Atenafu EG, et al. Seizure identification by critical care providers using quantitative electroencephalography. Crit Care Med. 2018;46:e1105–e1111. 6.Kang JH, Sherill GC, Sinha SR, Swisher CB. A trial of real-time electrographic seizure detection by neuro-ICU nurses using a panel of quantitative EEG trends. Neurocrit Care. 2019;31:312–320. 7.Williamson CA, Wahlster S, Shafi MM, Westover MB. Sensitivity of compressed spectral arrays for detecting seizures in acutely ill adults. Neurocrit Care. 2014;20:32–39. 8.Du Pont-Thibodeau G, Sanchez SM, Jawad AF, et al. Seizure detection by critical care providers using amplitude-integrated electroencephalography and color density spectral array in pediatric cardiac arrest patients. Pediatr Crit Care Med. 2017;18:363–369. 9.Prior P, Maynard D. Technical and practical aspects of monitoring cerebral function. In: Prior P, Maynard D, eds. Long-term Monitoring of EEG and Evoked Potentials, 1st ed. Elsevier; 1986. 10.Moura LM, Shafi MM, Ng M, et al. Spectrogram screening of adult EEGs is sensitive and efficient. Neurology. 2014;83:56–64. 11.Haider HA, Esteller R, Hahn CD, et al. Sensitivity of quantitative EEG for seizure identification in the intensive care unit. Neurology. 2016;87:935–944. 12.Wusthoff CJ, Shellhaas RA, Clancy RR. Limitations of single-channel EEG on the forehead for neonatal seizure detection. J Perinatol. 2009;29:237–242. 13.Rennie JM, Chorley G, Boylan GB, Pressler R, Nguyen Y, Hooper R. Non-expert use of the cerebral function monitor for neonatal seizure detection. Arch Dis Child Fetal Neonatal Ed. 2004;89:F37–40. 14.Bourez-Swart MD, van Rooij L, Rizzo C, et al. Detection of subclinical electroencephalographic seizure patterns with multichannel amplitude-integrated EEG in full-term neonates. Clin Neurophysiol. 2009;120:1916–1922. 15.Frenkel N, Friger M, Meledin I, et al. Neonatal seizure recognition—comparative study of continuous-amplitude integrated EEG versus short conventional EEG recordings. Clin Neurophysiol. 2011;122:1091–1097. 16.Gotman J, Gloor P. Automatic recognition and quantification of interictal epileptic activity in the human scalp EEG. Electroencephalogr Clin Neurophysiol. 1976;41:513–529. 17.Gotman J. Automatic recognition of epileptic seizures in the EEG. Electroencephalogr Clin Neurophysiol. 1982;54:530–540. 18.James CJ, Jones RD, Bones PJ, Carroll GJ. Detection of epileptiform discharges in the EEG by a hybrid system comprising mimetic, self-organized artificial neural network, and fuzzy logic stages. Clin Neurophysiol. 1999;110:2049–2063. 19.Winterhalder M, Schelter B, Maiwald T, et al. Spatio-temporal patient-individual assessment of synchronization changes for epileptic seizure prediction. Clin Neurophysiol. 2006;117:2399–2413. 20.Wilson SB, Scheuer ML, Emerson RG, Gabor AJ. Seizure detection: Evaluation of the Reveal algorithm. Clin Neurophysiol. 2004;115:2280–2291. 21.Kharbouch A, Shoeb A, Guttag J, Cash SS. An algorithm for seizure onset detection using intracranial EEG. Epilepsy Behav. 2011;22 Suppl 1:S29–35. 22.Ramgopal S, Thome-Souza S, Jackson M, et al. Seizure detection, seizure prediction, and closed-loop warning systems in epilepsy. Epilepsy Behav. 2014;37:291–307. 23.Sharmila A. Epilepsy detection from EEG signals: A review. J Med Eng Technol. 2018;42:368–380. 24.Gonzalez Otarula KA, Mikhaeil-Demo Y, Bachman EM, Balaguera P, Schuele S. Automated seizure detection accuracy for ambulatory EEG recordings. Neurology. 2019;92:e1540–e1546. 25.Foreman B, Claassen J. Quantitative EEG for the detection of brain ischemia. Crit Care. 2012;16:216. 26.Amzica F, Lopes da Silva FH. Cellular substrates of brain rhythms. In: Niedermeyer E, Schomer DL, Silva FHLd, eds. Niedermeyer’s Electroencephalography: Basic Principles, Clinical Applications, and Related Fields. 6th ed. Lippincott Williams & Wilkins; 2010:33–63. 27.Hofmeijer J, van Putten MJ. Ischemic cerebral damage: An appraisal of synaptic failure. Stroke. 2012;43:607–615. 28.Jordan KG. Emergency EEG and continuous EEG monitoring in acute ischemic stroke. J Clin Neurophysiol. 2004;21:341–352. 29.Herman ST, Abend NS, Bleck TP, et al. Consensus statement on continuous EEG in critically ill adults and children, part I: Indications. J Clin Neurophysiol. 2015;32:87–95. 30.Rots ML, van Putten MJ, Hoedemaekers CW, Horn J. Continuous EEG monitoring for early detection of delayed cerebral ischemia in subarachnoid hemorrhage: A pilot study. Neurocrit Care. 2016;24:207–216. 31.Rosenthal ES, Biswal S, Zafar SF, et al. Continuous electroencephalography predicts delayed cerebral ischemia after subarachnoid hemorrhage: A prospective study of diagnostic accuracy. Ann Neurol. 2018;83:958–969. 32.Yu Z, Wen D, Zheng J, et al. Predictive accuracy of alpha-delta ratio on quantitative electroencephalography for delayed cerebral ischemia in patients with aneurysmal subarachnoid hemorrhage: Meta-analysis. World Neurosurg. 2019;126:e510–e516. 33.Finnigan S, Wong A, Read S. Defining abnormal slow EEG activity in acute ischaemic stroke: Delta/alpha ratio as an optimal QEEG index. Clin Neurophysiol. 2016;127:1452–1459. 34.Finnigan SP, Rose SE, Chalk JB. Rapid EEG changes indicate reperfusion after tissue plasminogen activator injection in acute ischaemic stroke. Clin Neurophysiol. 2006;117:2338–2339. 35.Cho A, Chae JH, Kim HM, et al. Electroencephalography in pediatric moyamoya disease: Reappraisal of clinical value. Childs Nerv Syst. 2014;30:449–459. 36.Press CA, Morgan L, Mills M, et al. Spectral electroencephalogram analysis for the evaluation of encephalopathy grade in children with acute liver failure. Pediatr Crit Care Med. 2017;18:64–72. 37.Ostendorf AP, Hartman ME, Friess SH. Early electroencephalographic findings correlate with neurologic outcome in children following cardiac arrest. Pediatr Crit Care Med. 2016;17:667–676. 38.Abend NS, Xiao R, Kessler SK, Topjian AA. Stability of early EEG background patterns after pediatric cardiac arrest. J Clin Neurophysiol. 2018;35:246–250. 39.Ducharme-Crevier L, Press CA, Kurz JE, Mills MG, Goldstein JL, Wainwright MS. Early presence of sleep spindles on electroencephalography is associated with good outcome after pediatric cardiac arrest. Pediatr Crit Care Med. 2017;18:452–460. 40.Topjian AA, Sanchez SM, Shults J, Berg RA, Dlugos DJ, Abend NS. Early electroencephalographic background features predict outcomes in children resuscitated from cardiac arrest. Pediatr Crit Care Med. 2016;17:547–557. 41.Lee S, Zhao X, Davis KA, Topjian AA, Litt B, Abend NS. Quantitative EEG predicts outcomes in children after cardiac arrest. Neurology. 2019;92:e2329–e2338. 42.Jain SV, Zempel JM, Srinivasakumar P, Wallendorf M, Mathur A. Early EEG power predicts MRI injury in infants with hypoxic-ischemic encephalopathy. J Perinatol. 2017;37:541–546. 43.Tjepkema-Cloostermans MC, van Meulen FB, Meinsma G, van Putten MJ. A Cerebral Recovery Index (CRI) for early prognosis in patients after cardiac arrest. Crit Care. 2013;17:R252. 44.Ruijter BJ, Hofmeijer J, Tjepkema-Cloostermans MC, van Putten M. The prognostic value of discontinuous EEG patterns in postanoxic coma. Clin Neurophysiol. 2018;129:1534–1543. 45.Wiley SL, Razavi B, Krishnamohan P, et al. Quantitative EEG metrics differ between outcome groups and change over the first 72 h in comatose cardiac arrest patients. Neurocrit Care. 2018;28:51–59. 46.Efthymiou E, Renzel R, Baumann CR, Poryazova R, Imbach LL. Predictive value of EEG in postanoxic encephalopathy: A quantitative model-based approach. Resuscitation. 2017;119:27–32. 47.Gollwitzer S, Muller TM, Hopfengartner R, et al. Quantitative EEG after subarachnoid hemorrhage predicts long-term functional outcome. J Clin Neurophysiol. 2019;36:25–31. 48.Tolonen A, Sarkela MOK, Takala RSK, et al. Quantitative EEG parameters for prediction of outcome in severe traumatic brain injury: Development study. Clin EEG Neurosci. 2018;49:248–257. 49.Finnigan SP, Walsh M, Rose SE, Chalk JB. Quantitative EEG indices of sub-acute ischaemic stroke correlate with clinical outcomes. Clin Neurophysiol. 2007;118:2525–2532. 50.Schleiger E, Sheikh N, Rowland T, Wong A, Read S, Finnigan S. Frontal EEG delta/alpha ratio and screening for post-stroke cognitive deficits: The power of four electrodes. Int J Psychophysiol. 2014;94:19–24.372
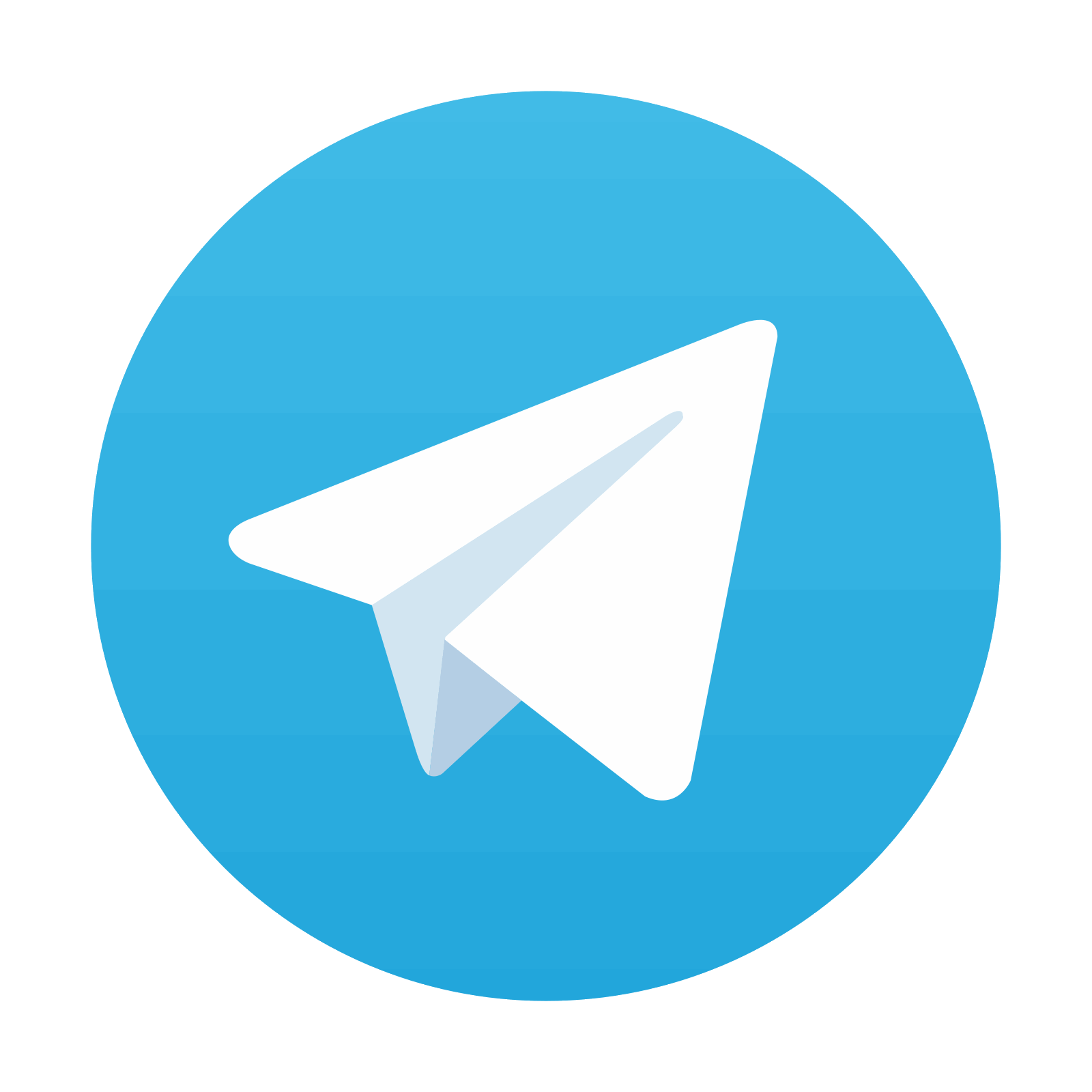
Stay updated, free articles. Join our Telegram channel

Full access? Get Clinical Tree
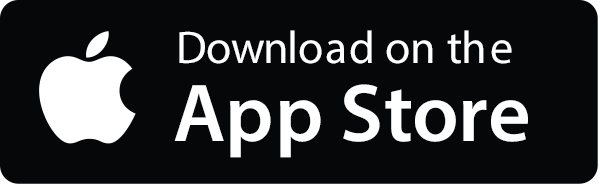
