Figure 16.1 Example problems (8 bars). Graphs were presented in blue and yellow, represented here by black and gray, respectively. (a) 2 × 2-way. Answers: smaller, greater. (b) Three-way. Answer: smaller. Adapted from Figure 1 in Halford, GS, Baker R, McCredden, JE and Bain, JD (2005) How many variables can humans process? Psychological Science, 16(1), 70–76.
(p.266)

Figure 16.2 Example problems (16 bars). Graphs were presented in blue and yellow, represented here by black and gray, respectively. (a) 2 × 3-way. Answers: greater, greater. (b) Four-way Answer: smaller. Adapted from Figure 2 in Halford, GS, Baker R, McCredden, JE and Bain, JD (2005) How many variables can humans process? Psychological Science, 16(1), 70–76.
Table 16.1 Number of participants answering neither, one, or both problems of each type correctly (n = 30)
Problem type | ||||
---|---|---|---|---|
Score | 2 × 2-way | 3-way | 2 × 3-way | 4-way |
Both correct | 30 | 26 | 23 | 13 |
One incorrect | 0 | 4 | 7 | 13 |
Both incorrect | 0 | 0 | 0 |
In the experiment, the verbal description of an interaction containing two possible directions for the interaction (greater or smaller) was presented at the top of the screen. A graph was then displayed below the text, initially using equal bar heights, while the participant read the verbal description and came to terms with how the descriptor variables mapped onto the corresponding bar labels in the graph. When the participants were ready, they pressed two keys which caused the graph to be transformed into a full figure, illustrating the interaction.
The experiment was designed to compare processing loads when task complexity increased, while holding memory load constant. Thus, two two-way interactions were compared with a three-way interaction (both using 8 bars) as shown in Figures 16.1a and b, and two three-way interactions were compared with a four-way interaction (both using 16 bars), as shown in Figures 16.2a and b. Each participant received two problems of each type for each of these two main comparisons. Solution times were recorded for the problem-solving phase (i.e., while the bars were unequal length). In addition, participants rated their confidence level for each answer.
Table 16.1 shows that as the complexity of the interaction increased, the number of participants answering one or more problems correctly decreased. Figure 16.3 shows that as task complexity increased, speed and confidence also both decreased, particularly between the 2 × 3-way and four-way problems. A supplementary experiment showed that performance on a five-way problem was no better than chance.
Overall, the results showed that a four-way interaction is difficult even for experienced adults to process without external aids. A soft limit to capacity to process information was indicated by the decline from three- to four-way interactions, with five-way being performed no better than chance. Our findings suggest that a structure defined on four variables is at the limit of human processing capacity, as a four-way interaction requires four variables to be integrated within a single concept. Representations based on four variables would coordinate well with visual and short-term memory capacities of four items, and are consistent with predictions from symbolic connectionist models, to be considered in a later section.
Neuroscience evidence for relational complexity
There is some evidence that specifically links relational complexity to processing in the frontal lobes. Waltz et al. (1999) contrasted two levels of relational complexity in participants with either prefrontal or anterior temporal lobe damage. The tasks used were transitive inference and modified Raven’s Progressive Matrices. In the one-relation condition, premises were presented in order, such as ‘Sam taller than Nate, Nate taller than Roy.’ This task can be segmented by processing one premise at a time, the order (Sam, Nate) being determined first, with Roy being concatenated to it, creating the ordered triple (Sam, Nate, Roy). This requires one binary relation to be (p.269)

Figure 16.3 Mean solution times and confidence ratings as a function of problem type. Adapted from Figure 3 in Halford GS, Baker R, McCredden JE and Bain JD (2005) How many variables can humans process? Psychological Science, 16(1), 70–76.
Waltz et al. (1999) found a double dissociation, so that prefrontal patients were impaired in processing complex relations, but were unimpaired in recall from semantic memory, whereas anterior temporal lobe damage was associated with impairment in semantic memory but not with impairment in relational processing. Furthermore, the groups did not differ in IQ, indicating that the effects reflected prefrontal involvement in processing complex relations, rather than a general factor of task difficulty.
Using a similar modification of Raven’s task to that used by Waltz et al. (1999), Christoff et al. (2001) observed greater activity in middle frontal gyrus (Brodmann area 10) for the two-relation condition than for the one or zero relation conditions, in a contrast analysis of fMRI (functional magnetic resonance imaging) data. Other studies (Kroger et al. 2002; Prabhakaran et al. 1997) found similarly increased activity in this and other prefrontal regions, as well as in the parietal lobes. Christoff and Gabrieli (2000) proposed a hierarchical model of the prefrontal cortex in relational processing, consisting of three functions: storage (ventrolateral); manipulation (dorsolateral); and evaluation (frontopolar). That is, processes in dorsolateral PFC operate on relations stored in ventrolateral PFC, and processes in frontopolar PFC evaluate the results of those operations.
(p.270) Further evidence for changes in cortical activity with changes in relational complexity has been found. Contrasts of activity during retention of binary (two roles) versus unary (one role) indexed lists revealed greater parietal and prefrontal activity for a variety of materials (Phillips and Niki 2002). Activity during the retention period for two binary lists was greater than for two unary lists for a region in the superior parietal lobule, dorsal to the occipitoparietal sulcus.
Developmental studies
The relational complexity metric has also been applied to a number of topics in cognitive development. Andrews and Halford (2002) conducted complexity analyses of tasks from six content domains: transitive inference, class inclusion, comprehension of relative clause sentences, cardinality, hypothesis testing and hierarchical classification. These analyses suggested that the tasks that cause difficulty for young children entail ternary relations, whereas the tasks mastered by young children are less complex. These complexity analyses were supported by a large-scale empirical study involving 241 children aged from 3 to 8 years. Children completed tasks in the six content domains, and relational complexity was manipulated in five of these. The items differed in complexity (binary- and ternary-relational) but were comparable in all other respects. Significant effects of relational complexity were observed in each domain. The ternary-relational items were more difficult, and were mastered later in development than the binary-relational items. Comparable ages of attainment were observed for tasks at the same complexity level in different domains. All tasks loaded on a single factor and factor scores were correlated with age (r = 0.80) fluid intelligence (r = 0.79) and working memory capacity (Listening span, r = 0.66). These results suggest that cognitive development is constrained by children’s capacity to process complex relations.
The relational complexity metric has been used to integrate findings from different paradigms and to resolve some long-standing paradoxes within the developmental literature. For example, category induction is attained by three years of age (Gelman and Markman 1987), when children can infer that a property attributed to one instance of a category is more likely to belong with other members of the same category than with members of a different category, independent of appearance (Deak and Bauer 1996; Gelman and Markman 1986, 1987; Gelman and O’Reilly 1988; Lopez et al. 1992). By contrast, children have difficulty making valid inferences about hierarchically structured categories until they are five years or older (Hodkin 1987; Inhelder and Piaget 1964; Winer 1980). For example, if they are shown a display depicting three bananas and five apples and are asked, ‘Are there more apples or more fruit?’, children under five or six years typically say, ‘More apples’. This apparent paradox has been resolved by relational complexity analysis. When both category induction and hierarchical classification were assessed using a common methodology (property inference tasks), the earlier development of category induction was shown to be due to its complexity being binary-relational, whereas hierarchical classification is ternary-relational. This was confirmed by the finding that hierarchical classification assessed using the property inference procedure corresponded in age of attainment to other ternary-relational tasks, transitivity and class inclusion assessed using the traditional procedure (Halford, Andrews and Jensen 2002).
The theory has generated many predictions beyond those that can be determined intuitively. Theory of mind provides an example. Theory of mind is typically assessed using false belief and appearance reality tasks, both of which are difficult for children younger than four to five years (Wellman et al. 2001). Relational complexity analyses indicated that these tasks are ternary-relational, therefore a structural complexity effect was predicted by Halford (1993). This has since been confirmed (Andrews et al. 2003). These authors devised binary-relational items that were closely matched in other respects to standard false belief and appearance-reality items. Mastery of these (p.271) binary-relational items was demonstrated by three-year-olds, whereas mastery of the ternary-relational items occurred later (four to five years).
Ternary-relational scores in the domains of transitivity, class inclusion, hierarchical classification and cardinality accounted for a high proportion of the variance in theory of mind scores, before and after controlling for age and performance on the binary-relational items in the same domains. Combined with the relational complexity effects, these results provide support for the role of relational complexity as a contributor to children’s theory of mind.
Predictions about children’s balance scale reasoning were also made ahead of data. It was predicted (Halford 1993) that two-year-olds would give correct judgments about a beam balance outcome when the decision could be based on weight information alone or distance information alone, because these are binary-relational. However children would not integrate weight and distance information until they could process ternary relations. These predictions were confirmed by Halford, Andrews, Dalton et al. (2002).
The above review demonstrates an accumulating body of research indicating that the capacity to process complex relations increases with age during childhood. Evidence obtained so far suggests that child cognitive development progresses through four levels of complexity. Unary relations are processed at a median age of one year. Children of this age appear to understand class membership, for example, dog (Fido). Binary relations, such as larger (elephant, mouse), are attained by about 1 ½–2 years of age. Ternary relations are processed at a median age of five years. Quaternary relations such as proportion (e.g., 2/4 = 4/8) emerge later, at around 11 years. That complexity effects are observed in a wide range of content domains indicates that the relational complexity metric is domain general in its application. There is also evidence for cross-domain consistency within individuals during early and middle childhood (Andrews and Halford 2002). The literature is consistent with an interpretation of the attainments of younger children, including some that have been regarded as precocious, as reflecting less complex processes than those of older children (Halford and Andrews 2006).
Working memory boundary conditions
The limitations of the central executive do not apply to all tasks, and it is essential to distinguish those cognitive processes that are subject to capacity limitations from those that are not. Lack of criteria for this can only lead to confusions and intractable issues. Three factors that distinguish cognitive tasks that are not capacity limited are automaticity, modularity and implicit processing. Automaticity develops through extended practice under constant mapping conditions (Hasher and Zacks 1979; Logan 1979; Logan 2005; Shiffrin and Schneider 1977) and is a well-known way of reducing processing loads. While constant mapping conditions of practice are maintained, tasks will be relatively free of capacity limitations, though the limitations will recur if mappings become varied.
Modular processes are also known not to impose processing loads on working memory. Modular processes are distinguished by the fact that they tend to be rapid, automatic, relatively unmodifiable, domain-specific and informationally encapsulated (Anderson 1992; Fodor 1983; Pylyshyn 1999). The distinction between implicit and explicit processes (Karmiloff-Smith 1992) is also relevant to identification of capacity limitations, because implicit processes tend to make low processing demands. They typically reflect a relatively early stage of development of acquisition of a concept, and are characterized by lack of strategic modifiability, so that performance tends to follow established or stereotyped procedures (Clark and Karmiloff-Smith 1993). The implicit–explicit distinction bears some correspondence to the distinction between associative and relational processes (Phillips et al. 1995).
(p.272) Symbolic processes and neural net representation of relations
We conceptualize the central executive as being specialized for processing relations, so we aim to demonstrate that relations can be represented in a neural network framework, along with processes for operating on the representation. There are two major approaches to neural net modeling of relational knowledge and, while there is some controversy, the common ground between the approaches provides useful insight into basic requirements for relational processing, and also provides possible explanations for capacity limitations in the central executive.
One approach to modeling relational knowledge uses role-filler bindings (Hummel and Holyoak 2003; Shastri and Ajjanagadde 1993). With this approach, loves (John, Mary) would be represented by binding John to the agent role of loves, and Mary to the patient role. This has limitations when multiple relational instances are to be represented (Halford et al. 1998, Sections 4.1.1.1 and 4.1.3; but see also Doumas and Hummel 2005).
The other major approach is to use symbol-argument bindings (Halford et al. 1998). That is, to represent loves (John, Mary), we bind the relation symbol loves simultaneously to John and to Mary. Structural alignment is used to maintain correspondence between instances, so if we have a second instance loves (Tom, Wendy), the two instances of loves are aligned, as are John and Tom, Mary and Wendy.
Bindings are implemented in two main ways. One way is based on synchronous activation (Hummel and Holyoak 2003; Shastri and Ajjanagadde 1993). Shastri and Ajjanagadde make units representing a role oscillate in phase (i.e., synchronously) with units representing the filler bound to that role, and out of phase with units representing other roles and fillers. This is a form of role-filler binding (see Figure 16.4).
Binding by simultaneous oscillation supports capacity limitations as follows, given that:
♦ a neural substrate is envisaged for this model
♦ biological neurons have a maximum rate at which they can oscillate (fire)
♦ cognition requires an (unspecified) minimum oscillation frequency, or cognitive responses become too slow, and
♦ oscillation frequencies must be sufficiently different, in order to be discriminable.
This means there must be a limited number of frequency ‘slots’ at which bound concepts can oscillate. This limits the number of concepts that can be represented simultaneously in a synchronous oscillation system.
The other approach to binding is based on a product operation, such as the tensor product, or outer product (Halford et al. 1998; Smolensky 1990) or circular convolution (Plate 1995). In the Structured Tensor Analogical Reasoning (STAR) model (Halford et al. 1998; Wilson et al. 2001; Wilson et al. 1995) the relation symbol and arguments are each represented by vectors, and the binding is represented by the outer product of these vectors. Thus the relational instance

Figure 16.4 Illustrating synchronous oscillation binding for the binary relation loves (John, Mary).
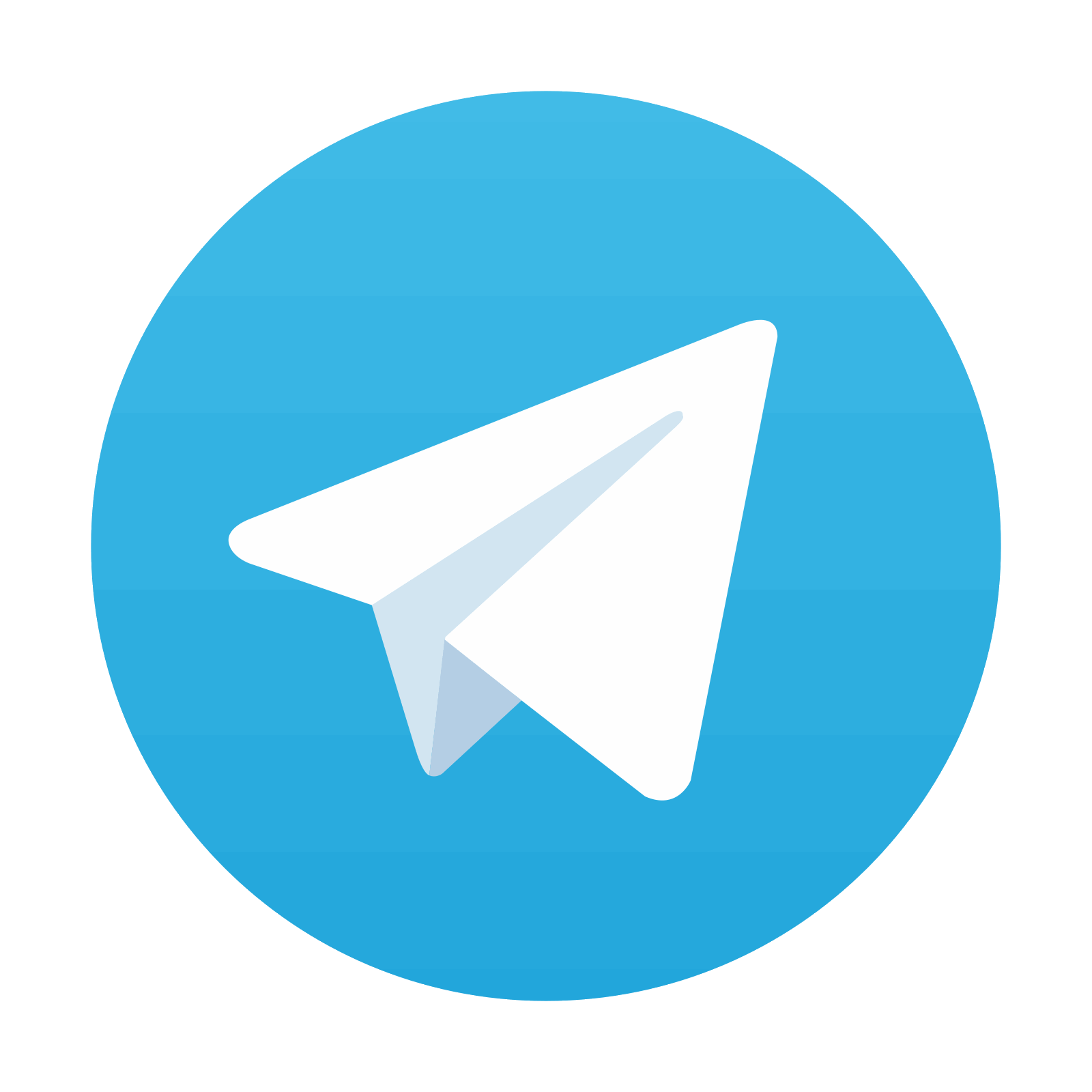
Stay updated, free articles. Join our Telegram channel

Full access? Get Clinical Tree
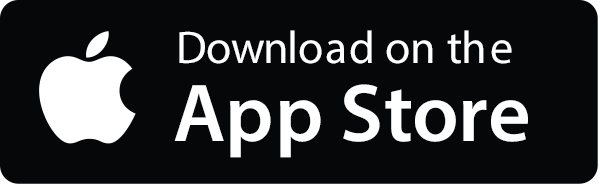

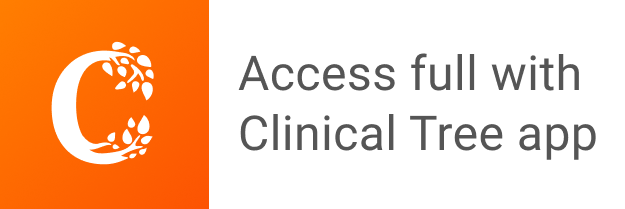