Scientific Foundations of the Field
INTRODUCTION
As we discussed in Chapter 1, the field of developmental psychopathology has diverse roots and origins. In this chapter, we discuss some aspects of the scientific basis of the field with an emphasis on research design, the role of evidence, and epidemiology.
Morgan et al. (2018) provide an excellent review of the scientific method and research approaches. As they note, research has the goals of increasing both knowledge and clinical competence. As a general rule, for research to be regarded of the highest quality it must be peer-reviewed in a scientific journal. This means that scientifically informed peers (other researchers and clinicians) have reviewed (typically in a double-blind fashion to preserve author and reviewer anonymity) a paper for a journal and the editor then accepts, rejects, or asks for revision of the paper. This process can be tedious and has its problems but is, at present, the best and most widely used approach to vetting new scientific information. The growing reliance on serious peer-reviewed research is a major advance since the first publication by Leo Kanner of a text book in the field (Kanner, 1935). We talk more about the role of research evidence subsequently, but it is important to understand that the scientific process does not end with one paper. To be regarded as evidence based, we require multiple papers (from different places and groups) that replicate and often extend a finding. In this way, science gradually and incrementally builds knowledge.
The field of child mental health faces several complications in doing research. In some ways, the most complex and challenging work is the more basic science in areas like genetics and neurobiology, but in other ways the most complicated is the actual work with children, their parents, and teachers. This underscores the problems of having at least three potential informants for any child during a study—that is, the child, the parents, and the teacher. Some measures, like blood pressure or galvanic skin response are less subject to the problem of differences in observations and data collected, but other measures, such as questionnaires filled out by different responders, can result in highly different views depending on the nature of the study. A similar issue relates to whether we ought to rely on observed behavior or on self-reports. For some purposes, either one or both may be needed. Depending on how the study is done (e.g., with or without a control group), it may be important to think about the impact of just being in a study itself on data obtained (Shapiro & Shapiro, 2000). A whole other set of issues arise relative to qualitative compared to quantitative research.
There is a large body of work on the analysis of quantitative data (Patton, 2002). Qualitative data are more subjective in nature, for example, the child’s perception of pain or of their feelings about a treatment approach (see Merriam & Tisbell, 2016). Qualitative data are typically gathered from interviews and observations, and investigators do not necessarily go on to try to apply rigorous statistical methods of analysis given the nature of the data obtained. It is important to emphasize that self-report data and ratings of behaviors or mental states by others (such as a parent rating their child’s anxiety) can be quantitative or qualitative in nature. Investigators working with quantitative data are more able to apply simple and some more sophisticated statistical analyses to help understand the meaning of the data they collect. Often, they will be attempting to prove some specific idea or hypothesis.
Research usually begins with a question about a problem or issue of some kind: for example, will exercise improve adolescent depression? A host of different measures and variables might be of relevance to a study looking to address this. These might include ratings of depressed mood as well as age, gender, social status, and ethnicity of the child and family. History of depression, rating scales of depression (from the child), and reports from parents and teachers can provide many additional variables for analysis.
Data analysis for quantitative researchers usually involves well-defined statistical methods, often providing a test of the null hypothesis (i.e., no true differences are observed). Qualitative researchers are more interested in examining their data for similarities or themes that might occur among participants on a particular topic. There is always a tension between how strictly one limits the subject group and how narrowly you define the problem. If you only study boys, for example, you cannot necessarily say much about girls. There is also a tension over how little or how much information you obtain. Variables can themselves be of various types, for example, they can be continuous like age or IQ, or they can be categorical like meeting criteria for the diagnosis of depression or not.
As researchers begin to design a study, they consider both independent and dependent variables. Independent variables have to do with things like disorder status (positive or negative) whereas dependent variables have to do with the things we measure and expect to change in response to the independent variables. Thus, the independent variables are presumed to involve antecedent or possible causes of effects that we can measure in the dependent variable(s) selected (Gliner et al., 2017). Variables can also be extraneous (i.e., things not of interest to the particular study). Morgan et al. (2018) provide an excellent summary of aspects of research variables and simple methods of data description and analyses. They note that there are a number of easy ways to visually depict (plot) data that can be highly informative. Depending on the nature of the distribution of the data, statistics like measures of central tendency may be very helpful, for example, average, median, and mode as well as measures of the variation within the data like the standard deviation. For some data, for example, categorical (present/absent, yes/no) or rank order data, for example, educational attainment levels, other kinds of statistical approaches apply (see Shadish et al., 2002).
Another set of issues has to do with different types of reliability and validity in research studies. There are several kinds of reliability: interrater reliability (agreement between two observations), test-retest reliability (how stable scores are over time), and internal consistency (how well items on a test “hang together” [i.e., correlate] with each other) as well as several others (Morgan et al., 2006). In child mental health, we have the problem of differences in reporting as well as stability of scores and changes over time. A test might work well for adults but not for children. In the past there were simple approaches to assessing reliability, for example, percent agreement scores or sometimes correlations but the field has become much more sophisticated with statistics used that take chance agreement levels into account. These more robust statistics provide us with better measures to assess the instruments used in work in child mental health (Morgan et al., 2006). As the field of developmental psychopathology matures, there is also continuing refinement of research tools. For example, there is now growing recognition and appreciation of the need to collect information from multiple sources and that each informant contributes a valuable perspective (De Los Reyes et al., 2015).
The notion of validity has to do with establishing that what we are measuring truly does capture what we intend it to: for example, does a dimensional assessment of parent-reported attention-deficit hyperactivity disorder symptoms really capture how the child behaves in the classroom? Again, there are various kinds of validity and various statistical approaches to its measurement (e.g., face validity, convergent validity, divergent validity). It is possible to have a test with good reliability that is not particularly valid, for example, picking a test on which the child cannot perform the tasks at all will result in highly reliable measures (child consistently scores very low) that do not tell us much about the child’s actual abilities.
Researchers try to use instruments that are well established and have high reliability and validity—and although never perfect, using these well-established measures helps ensure appropriate study design. As the measures are changed or extended in any way, for example, with a new population, different age, or different language, all these measurement issues have to be considered anew.
Quantitative research methods are of various types and can include randomized clinical trials as well as quasi-experimental descriptive and other experimental approaches (Merriam & Tisbell, 2016). The analysis of data from this kind of research tests relationships between variables and helps us answer research questions and address specific hypotheses: for example, drug X will significantly improve obsessive-compulsive disorder symptoms. For a study like this, a double-blind randomized placebo-controlled study would be the best because it provides appropriate guidance about inferring cause and effect. In a typical randomized controlled study, patients would be randomly assigned to one of two or more groups such that one group might receive the active drug (the medication of interest) while another group receives a placebo (a “sham” medication that is not expected to have any “real” effect). In this case, the independent variable is the group assignment (drug/placebo) whereas the dependent measure is the rating scale score measuring change in the severity of symptoms after treatment. Random assignment is critically important. Given ethical concerns, often at the end of a treatment trial, the “blind” will be broken, and, if effective, the drug may be offered to those who received the placebo. Other kinds of studies may be based on associations or descriptive work—these kinds of studies often precede more hypothesis-driven and rigorous randomized clinical trials. A whole set of preliminary studies would, for example, first be done in evaluating any drug before it ever reaches the stage for a rigorous placebo-controlled clinical trial (see Chapter 29). Of note, having either more than one randomized controlled clinical trial, or one large trial with multiple sites, is often very helpful in firmly establishing the evidence base of an intervention. Having a replication in a different site is a good way to ensure that the results obtained are substantive and not because of chance findings at one site.
There are several kinds of questions that can be addressed in research studies and several different approaches to study design. A hypothesis is essentially a research question about the relationship between two variables, for example, that our hypothetical drug X will improve functioning in patients with obsessive-compulsive disorder. Randomized experiments offer us the strongest evidence but quasi-experiments are also useful in giving us important information. As Morgan et al. (2018) discuss, there are many different experimental designs, some of which are much stronger than others. The choice of design raises important issues as researchers consider what their goals are and what specific questions they want to address (see Gliner et al., 2017 for discussion). Results of simple descriptive studies can inform research that adopts more rigorous controls for sources of potential bias. Depending on the nature of the design, some studies use within-subject scores or measures whereas others use between-subject measures (or sometimes both). The issues of research design are complex but very important in interpreting the results investigators obtain. Issues of sample selection and sample size are important. There are both type 1 and type 2 errors that can potentially be made. The significant values obtained in statistical analysis help protect us (partially) from making these errors. A type 1 error happens when we observe an apparent difference that really is not there, whereas a type 2 error occurs when we do not see a real difference that is there, because our study was poorly done or underpowered. The role of statistical power is an important one. For some observed effects, the strength of the effect is quite strong and even a
small sample would be likely to show a statistical result; in other studies, the effect itself may be important but hard to detect and larger samples may be needed for these studies. There are ways to estimate statistical power (Cohen, 1988) as well as effect size and these are frequently used as research projects are designed. There are also good methods for assessment of the strength of an association observed beyond statistical significance. It is important to realize that what might be a statistically significant result may have little or no clinical significance. This can be a real problem with very large studies where chance makes for fluke findings. Of course, yet another potential source of errors, particularly for correlational approaches, is the fact that even if things are correlated that does not mean they are causally connected. For example, road temperature and lemonade consumption both go up in summer and are strongly correlated but not causally connected (both having more to do with overall temperature). A whole body of work exists focused on helping evaluate the strengths of results obtained and comparing results across studies (meta-analyses). Meta-analytic studies summarize and synthesize results of a series of studies using quantitative measures (Lipsey & Wilson, 2000). A whole body of work in medicine and other fields has used meta-analyses to help us evaluate possible treatments and whether or not they are effective. Several independent groups now routinely conduct these studies to help establish what treatments work (see evidence-based treatments later in this chapter). These offer important insights into how important findings really are. Although meta-analyses are useful, it is important to realize that they have faced some criticisms. It can be argued that combining data from different studies, even if seemingly matching in certain parameters, can sometimes yield misleading results.
small sample would be likely to show a statistical result; in other studies, the effect itself may be important but hard to detect and larger samples may be needed for these studies. There are ways to estimate statistical power (Cohen, 1988) as well as effect size and these are frequently used as research projects are designed. There are also good methods for assessment of the strength of an association observed beyond statistical significance. It is important to realize that what might be a statistically significant result may have little or no clinical significance. This can be a real problem with very large studies where chance makes for fluke findings. Of course, yet another potential source of errors, particularly for correlational approaches, is the fact that even if things are correlated that does not mean they are causally connected. For example, road temperature and lemonade consumption both go up in summer and are strongly correlated but not causally connected (both having more to do with overall temperature). A whole body of work exists focused on helping evaluate the strengths of results obtained and comparing results across studies (meta-analyses). Meta-analytic studies summarize and synthesize results of a series of studies using quantitative measures (Lipsey & Wilson, 2000). A whole body of work in medicine and other fields has used meta-analyses to help us evaluate possible treatments and whether or not they are effective. Several independent groups now routinely conduct these studies to help establish what treatments work (see evidence-based treatments later in this chapter). These offer important insights into how important findings really are. Although meta-analyses are useful, it is important to realize that they have faced some criticisms. It can be argued that combining data from different studies, even if seemingly matching in certain parameters, can sometimes yield misleading results.
Meta-analyses usually end up producing estimates of effect sizes, that is, measures of how statistically strong the results are. These can be assessed in other studies as well. Various statistics can be used to assess the strength of an effect. Particularly in behavioral studies, a strong or large effect size can provide a good indication of clinical significance. For psychotherapy research, for example, to have at least a moderate or stronger effect size is desirable. Treatments that have small effect sizes are often not regarded as really supported by evidence-based reviews (see Cohen, 1988).
EVIDENCE-BASED PRACTICES AND TREATMENTS
Implementation of evidence-based treatments has been an important theme in child mental health—in medicine, psychology, education, and related areas (Hamilton, 2018). Most of the actual clinical work occurs either in private practice or larger clinical settings such as community mental health clinics. The transfer of knowledge about effective mental health treatments from research settings where these treatments are developed and tested to clinical settings where these treatments are delivered to children can be a challenging process. In this section, we address issues for implementation of evidence-based treatments.
As Hamilton (2018) points out, there are several somewhat overlapping terms used in describing similar concepts: evidence-based practice, evidence-based medicine, and evidence-based treatments/services. Not surprisingly, evidence-based medicine tends to be the term used by child psychiatrists and pediatricians and is very relevant to use of medications (see Chapter 20). The term evidence-based treatment is more common among psychologists and is often used relative to psychotherapy or psychosocial treatments referring to better use of empirical results to improve outcomes. Evidence-based services and evidence-based treatment are the terms more commonly used in interdisciplinary treatment settings. The more general term evidence-based practice encompasses all these uses and thus ranges from understanding the strength of evidence supporting a treatment to its implementation in the individual case. It is worth noting that in psychology in particular, there is often an emphasis on using clearly described and rigorously implemented psychosocial or behavioral approaches as part of evidence-based treatments. This is partly because of research requirements that treatments are well defined and can be replicated by others. Given the potential for disciplinebased terms to acquire highly specialized definitions within their respective disciplines, in clinical contexts, particularly interdisciplinary ones, the more general term evidence-based
practice is usually preferred. It is very apt given the general agreement of the interdisciplinary treatment team that the care of the individual child and family, in light of all the available evidence, is the goal of the treatment plan.
practice is usually preferred. It is very apt given the general agreement of the interdisciplinary treatment team that the care of the individual child and family, in light of all the available evidence, is the goal of the treatment plan.
One of the advantages of current digital technologies for all providers is ready access to information that evaluates treatment, and to treatment studies themselves. However, as the field of developmental psychopathology matures, new specialized treatments emerge and require use of very specific instruments or measures requiring significant training. This expertise and specialization can be more readily found in a university clinical research setting or even in a larger group practice setting but does become more challenging for the individual provider. A major increase in randomized controlled trials (RCTs) over the recent past is illustrated in Hamilton (2018). Similar increases have been seen in meta-analyses and systematic reviews that, along with RCTs, provide the fuel for making evidence-based recommendations.
There is a hierarchy of types of evidence with the strongest being RCTs and meta-analyses, to controlled studies, to case series, and finally to single cases and anecdotal clinical reports. The last category is not unimportant but clearly carries much less weight than the more scientifically informed studies. Case studies present their own complexities in that there is a tendency for only positive associations to be published (e.g., finding that syndrome X is associated with autism might be published but a paper that is titled syndrome X is not associated with autism is unlikely to see the light of day).
Sometimes, it is argued that rigorous research methods (Rutter et al., 1994) do not have applicability to something as individualized as psychotherapy, but, as Hamilton points out, evidence-based methods have been very effectively used to demonstrate positive effects and to help disentangle these effects (Hamilton, 2018). The importance and feasibility of conducting evidence-based practice in real-world settings have been illustrated in studies of psychotherapy with children and adolescents (Bickman et al., 1999; Weisz & Jensen, 1999). For example, data from an evidence-based system in Hawaii (the Hawaii’s Child and Adolescent Mental Health Division [CAMHD]) offers a useful case in point (Daleiden & Chorpita, 2005). As Hamilton (2018) notes, there is an important backstory to the CAMHD program, starting with a federal court decision mandating an effective system of care as the stimulus for establishing a more integrated mental health and special education approach with more access for youth to a wide range of services. The initial system response to the court’s decree included planning efforts and increases in service capacity, allowing more youth to access a wider variety of services, increased monitoring, and the agreement and commitment of all concerned to an awareness of evidence-based practices (Daleiden, 2004).
Two approaches are often used in understanding the overall benefits and risks of treatments and these can be used in child mental health as in other aspects of medicine. The number needed to treat refers to the number of individual cases you need to treat to prevent one additional bad outcome (e.g., suicide attempt, incarceration, or some other explicit indicator). This is usually expressed as a whole number and is typically done in studies with a control group. So, for example, in the treatment for adolescents with depression study, this number was 4 for the use of active medicine (fluoxetine) alone but 3 when you combined medication with cognitive behavior therapy (see Hamilton, 2018 for a detailed discussion). A different metric, the number needed to harm gives information on the average number of individuals who are exposed to some risk who are actually caused harm (as operationally defined over some period of time). Both numbers are usually given with a 95% confidence interval (see Hamilton, 2018 for a more extended discussion).
With the advent of evidence-based interventions and good search engines as well as free and ready access to the national library of medicine’s PubMed service, most clinicians have ready access to scientific information. As Hamilton (2018) reviews extensively, it is good to have some awareness of the potential pitfalls of literature searching in evaluating available evidence. For the university-affiliated clinician, scholarly resources are readily at hand. However, a simple internet search that a patient or family is often inclined to do is several steps below a search of information like that documented in PubMed (although there are some important cautionary notes even there) and can quickly lead to misguided information, particularly in the area of mental health. For example, at the time of this writing, typing
the word autism into Google gives about 200,000,000 hits whereas a search for autism in PubMed or some other scholarly base may show more like 40,000 peer-reviewed articles. As one can imagine, this staggering complexity is a problem for parents and youth searching for valid information. Sadly, going by the top 100 “greatest hits” is complex because a number of sites are shown that make baseless claims or ask for money to produce results (Reichow et al., 2014). As a general rule, websites that end in .edu or .gov provide potential consumers of mental health services the best information. Clinicians should be prepared these days to discuss with their patients the importance of evaluating the science behind any intervention.
the word autism into Google gives about 200,000,000 hits whereas a search for autism in PubMed or some other scholarly base may show more like 40,000 peer-reviewed articles. As one can imagine, this staggering complexity is a problem for parents and youth searching for valid information. Sadly, going by the top 100 “greatest hits” is complex because a number of sites are shown that make baseless claims or ask for money to produce results (Reichow et al., 2014). As a general rule, websites that end in .edu or .gov provide potential consumers of mental health services the best information. Clinicians should be prepared these days to discuss with their patients the importance of evaluating the science behind any intervention.
There are several additional important potential sources of information. The Cochrane collaboration is named in honor of the British epidemiologist Archie Cochrane, whose 1972 book Effectiveness and Efficiency: Random Reflections on Health Services was a landmark in the development of clinical trials research. Cochrane was very concerned with understanding what treatments were to be regarded as well established, inefficacious or unproven, or contraindicated using the scientific evidence provided in a clinical trial. The collaboration named in his honor conducts independent research assessments on various topics with rigorous guidelines for authors who engage in these reviews and the Cochrane Database of Systematic Reviews (CDSR) has become an outstanding online resource. Some of the most useful databases and clinical resources are summarized in Table 3.1.
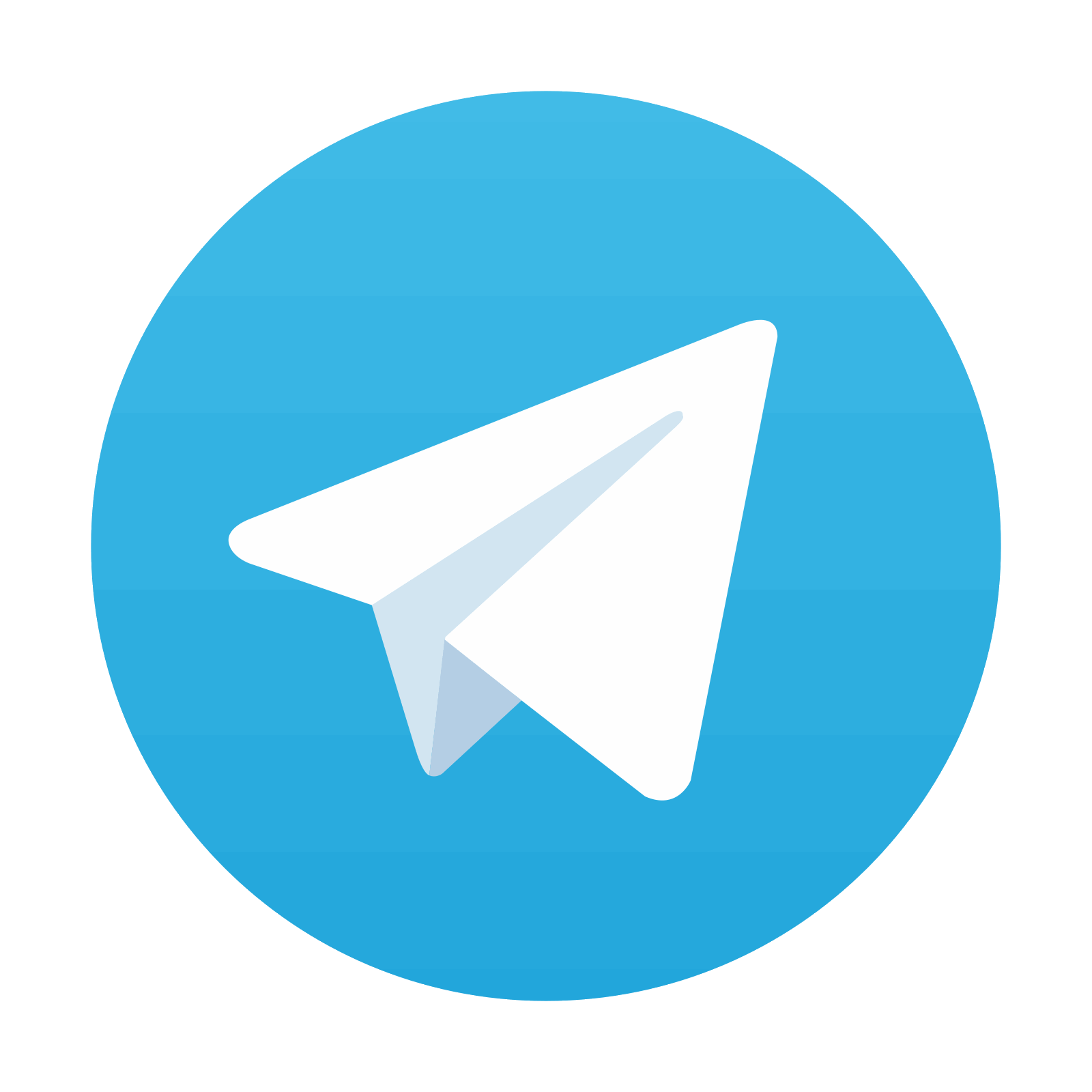
Stay updated, free articles. Join our Telegram channel

Full access? Get Clinical Tree
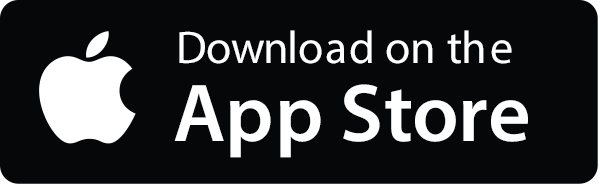

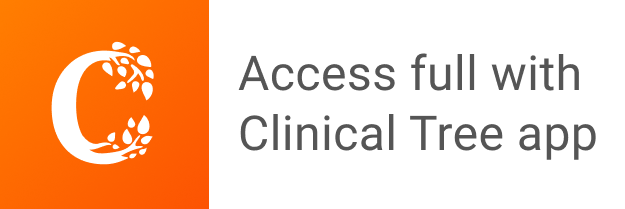