Epilepsy is characterized by intermittent, paroxysmal, hypersynchronous electrical activity that may remain localized and/or spread and severely disrupt the brain’s normal multitask and multiprocessing function. Epileptic seizures are the hallmarks of such activity. The ability to issue warnings in real time of impending seizures may lead to novel diagnostic tools and treatments for epilepsy. Applications may range from a warning to the patient to avert seizure-associated injuries, to automatic timely administration of an appropriate stimulus. Seizure prediction could become an integral part of the treatment of epilepsy through neuromodulation, especially in the new generation of closed-loop seizure control systems.
Epilepsy is considered a window to the brain’s function and is therefore an increasingly active, interdisciplinary field of research. The sacred or divine disease is among the most common disorders of the nervous system, second only to stroke and Alzheimer disease, and affects 1% to 2% of the world’s population. Although epilepsy occurs in all age groups, the highest incidences occur in infants and in the elderly. This high incidence of epilepsy stems from it having a large number of causes, including genetic abnormalities, developmental anomalies, and febrile convulsions, as well as brain insults such as craniofacial trauma, central nervous system infections, hypoxia, ischemia, and tumors.
The hallmarks of epilepsy are recurrent seizures and epileptic spikes. If seizures cannot be controlled, the patient experiences major limitations in family, social, educational, and vocational activities. These limitations have profound effects on the patient’s quality of life. Epileptic seizures and spikes are caused by the sudden development of pathologic, synchronous neuronal firing in the cerebrum and can be recorded by scalp, subdural, and intracranial electrodes. Seizures may begin locally in portions of the cerebral hemispheres (partial/focal seizures) with a single or multiple foci, or simultaneously in both cerebral hemispheres (generalized seizures). After a seizure’s onset, partial seizures may remain localized and cause mild cognitive, psychic, sensory, motor, or autonomic symptoms, or may spread (secondarily generalized) to cause altered consciousness, complex automatic behaviors, or bilateral tonic-clonic convulsions. Even though seizures typically run their course (seconds to minutes) and the brain subsequently recovers by itself, there are cases in which recovery is accomplished only through external intervention (ie, high doses of antiepileptic drugs), as in status epilepticus (SE), a life-threatening condition. The brain also does not recover by itself in the event of sudden unexplained death in epilepsy (SUDEP). SUDEP is a less common condition than SE, is seemingly unpredictable, and hence extremely difficult to monitor and provide for external intervention.
One of the most debilitating aspects of epilepsy is that seizures seem to occur without warning. Until recently, the general belief in the medical community was that epileptic seizures could not be anticipated. Seizures were assumed to occur abruptly and randomly over time. However, hypotheses on the mechanisms of ictogenesis and predictability of seizures had been postulated in the past based on reports from clinical practice (eg, existence of auras) and scientific intuition (eg, theory of reservoir). In the 1970s, attempts to show that seizures are predictable had also been undertaken via computer analysis of the electroencephalogram (EEG). Despite those early attempts, the results were not encouraging. Systematic and robust detection of a preictal period across seizures in the same patient, as well as across patients, remained elusive. The essential features of the brain’s transition to epileptic seizures were not captured, and a theoretic framework for seizure development that could lead to definition and subsequent detection of such preictal features was missing.
It was in the 1980s that new signal processing methodologies emerged, based on the mathematical theory of nonlinear dynamics and chaos for the study of spontaneous formation of organized spatial, temporal, or spatiotemporal patterns in physical, chemical, and biologic systems. These methodologies quantified the complexity and randomness of the signal from the perspective of invariants of nonlinear dynamics and represented a drastic departure from the signal processing techniques based on linear systems analysis (eg, Fourier analysis). Because the brain is inherently a nonlinear system, the general concept at that time was that seizures represented transitions of the epileptic brain from its normal, less ordered (chaotic) state to an abnormal, more ordered state and back to a normal state along the lines of chaos-to-order-to-chaos transitions. How this concept, when applied to the EEG in epilepsy, eventually changed some long-held beliefs about seizures and their dynamical causes is discussed later. Within this framework, systematic mathematical analysis of long-term EEG recordings that included seizures started in the mid-1980s at the University of Michigan (U of M), creating, at the time, the largest worldwide database of digitally stored peri-ictal EEG recordings with seizures. The existence of long-term preictal periods (order of minutes) was shown in 1988 by nonlinear dynamical analysis of EEGs recorded by subdural arrays from patients undergoing phase II monitoring of their seizures at the U of M Hospital’s Epilepsy Monitoring Unit (EMU).
Existence of a preictal period: seizure predictability
Among the important measures of the dynamics a linear or nonlinear system exhibits are the Lyapunov exponents that measure the average information flow (bits per second) the system produces along local eigendirections in its state space. Positive Lyapunov exponents denote generation of information, whereas negative exponents denote destruction of information. A chaotic nonlinear system possesses at least 1 positive Lyapunov exponent, and it is because of this feature that its behavior seems random, even if it is deterministic in nature. Methods for calculating these measures of dynamics from experimental data have been published.
The brain, being nonstationary, is never in a steady state in the strictly dynamical sense, at any location. We have shown that, in the case of a nonstationary system with transients like epileptic spikes, the use of the short-term maximum Lyapunov exponent (STL max ) constitutes a more accurate characterization of the rate of the average information flow than the traditional maximum Lyapunov (L max ) exponent. STL max is estimated from sequential EEG segments of 10 seconds in duration per recording site to create a set of STL max profiles with time. Analysis of scalp, subdural, or depth EEG from patients with focal (temporal and frontal lobe) epilepsy at the U of M, and subsequently at the University of Florida and Arizona State University, showed that the STL max profiles at brain sites systematically and progressively converge to similar values tens of minutes before a seizure and remain entrained up to the onset of the seizure. We have called this phenomenon preictal dynamical entrainment (convergence of measures of EEG dynamics long before a seizure onset), the involved brain sites critical sites, and the corresponding pairs of sites that interact in this dynamical sense critical pairs. The focal sites are typically part of the set of critical sites. Therefore, the following hypothesis was formed that directly relates to mechanisms of ictogenesis: the epileptic brain is dynamically entrained by the focal sites long before a seizure’s occurrence.
It was further hypothesized that the brain starts malfunctioning because of this loss of relative independence of processing of information at normal brain sites long before a seizure develops. Such a preictal entrainment is illustrated in Fig. 1 for a patient with focal epilepsy and EEG recorded by intracranial electrodes (see Fig. 1 A, B). The STL max profiles of critical sites with time are shown in Fig. 1 C. The convergence of STL max profiles of a pair of sites is quantified by the T-index, a statistical measure of the distance between the mean values of the respective time series. Small values of T-index denote small distances between the corresponding STL max profiles, and hence entrainment of dynamics. T-index values with time are estimated within a running window of 10 minutes in duration (60 STL max values) for a pair of STL max profiles. Pairs of sites that are dynamically entrained in the 10-minute period before a seizure are characterized as critical pairs. The average T-index profile illustrated in Fig. 1 D represents the average of all T-indices over time across the thus selected critical pairs of sites. From Fig. 1 D, it is clear that, if critical pairs of sites are selected (retrospectively) from the immediate preictal period of a seizure, a seizure is predictable. For example, in the seizure depicted in Fig. 1 B, a warning could have been issued about 1 hour before its onset (see Fig. 1 D), that is, when the average T-index crosses the statistical threshold T th = 2.662 (α = 0.01 significance level for convergence of STL max profiles) from above.

Other measures of dynamics, like phase, also exhibit dynamical entrainment in the preictal period, although later than STL max . In addition to patients, epileptic rats with chronic epilepsy also exhibit measurable preictal periods in the order of minutes. These results show that seizures are not abrupt transitions in and out of an abnormal ictal state, but instead they follow a dynamical transition that may evolve for minutes to hours. During this preictal dynamical transition, multiple regions of the brain progressively approach a similar dynamical state. Because such spatiotemporal transitions are progressive with time, it has been suggested that, in addition to preictal periods, seizure susceptibility periods could also be identified from analysis of corresponding interictal profiles of brain dynamics.
Resetting of brain dynamics at seizures
Seizures typically reset the preictal dynamical entrainment and lead to the disentrainment of dynamics of the focus from the rest of the brain. This important conjecture can be observed by comparing the average T-index values and their trend in the preictal period versus the postictal period. For example, after seizure’s onset (see Fig. 1 D), there is a trend toward disentrainment of the preictally entrained pairs of sites (T-index moves rapidly toward higher values above T th and toward ones it exhibited before the beginning of the preictal period). Fig. 1 C shows the same trend at the level of STL max profiles of individual brain sites. We have called this reversal of dynamics brain resetting at seizures and we have observed it in focal as well as generalized seizures, within and across patients. We also have observed it using other measures of brain dynamics. Furthermore, the observed dynamical resetting in patients with epilepsy is significantly specific to seizures at the α = 0.05 statistical significance level. Other groups have independently observed similarly reversal trends using classic methods of signal processing. This observation may reflect a passive mechanism (eg, high electrical activity during a seizure depletes critical neurotransmitters and thus deactivates critical neuroreceptors in the entrained neuronal network). An alternative explanation is an active mechanism, that is, seizure activity releases neuropeptides that may subsequently contribute to the temporary repair of a pathologic feedback network (discussed later) that allowed the dynamical entrainment to occur and to last for tens of minutes. Such an explanation is analogous to mechanisms attributed to seizures associated with electroconvulsive therapy (ECT).
Given the resetting of the brain’s pathological entrainment with the focus at seizures, and the recovery that follows, one would expect that seizures in SE fail to reset this pathology of brain dynamics. Indeed, we have observed this after a similar to the above dynamical analysis of the EEG from patients with SE in the emergency room (ER), intensive care unit (ICU), or the EMU. Successful antiepileptic drug (AED) administration, which interrupted SE seizures in rats and humans, resulted in the resetting of brain dynamics. Unsuccessful AED administration in humans and/or no AED administration in SE rats failed to reset the brain dynamics, with lethal consequences. Thus, resetting of dynamics could be used to monitor treatment of SE by AEDs, as well as for the evaluation of current AEDs, and possibly the design of new AEDs. Psychogenic nonepileptic seizures do not seem to reset the brain dynamics, and the phenomenon of resetting could thus be used as a tool for differential diagnosis between epileptic and psychogenic nonepileptic seizures.
Resetting of brain dynamics at seizures
Seizures typically reset the preictal dynamical entrainment and lead to the disentrainment of dynamics of the focus from the rest of the brain. This important conjecture can be observed by comparing the average T-index values and their trend in the preictal period versus the postictal period. For example, after seizure’s onset (see Fig. 1 D), there is a trend toward disentrainment of the preictally entrained pairs of sites (T-index moves rapidly toward higher values above T th and toward ones it exhibited before the beginning of the preictal period). Fig. 1 C shows the same trend at the level of STL max profiles of individual brain sites. We have called this reversal of dynamics brain resetting at seizures and we have observed it in focal as well as generalized seizures, within and across patients. We also have observed it using other measures of brain dynamics. Furthermore, the observed dynamical resetting in patients with epilepsy is significantly specific to seizures at the α = 0.05 statistical significance level. Other groups have independently observed similarly reversal trends using classic methods of signal processing. This observation may reflect a passive mechanism (eg, high electrical activity during a seizure depletes critical neurotransmitters and thus deactivates critical neuroreceptors in the entrained neuronal network). An alternative explanation is an active mechanism, that is, seizure activity releases neuropeptides that may subsequently contribute to the temporary repair of a pathologic feedback network (discussed later) that allowed the dynamical entrainment to occur and to last for tens of minutes. Such an explanation is analogous to mechanisms attributed to seizures associated with electroconvulsive therapy (ECT).
Given the resetting of the brain’s pathological entrainment with the focus at seizures, and the recovery that follows, one would expect that seizures in SE fail to reset this pathology of brain dynamics. Indeed, we have observed this after a similar to the above dynamical analysis of the EEG from patients with SE in the emergency room (ER), intensive care unit (ICU), or the EMU. Successful antiepileptic drug (AED) administration, which interrupted SE seizures in rats and humans, resulted in the resetting of brain dynamics. Unsuccessful AED administration in humans and/or no AED administration in SE rats failed to reset the brain dynamics, with lethal consequences. Thus, resetting of dynamics could be used to monitor treatment of SE by AEDs, as well as for the evaluation of current AEDs, and possibly the design of new AEDs. Psychogenic nonepileptic seizures do not seem to reset the brain dynamics, and the phenomenon of resetting could thus be used as a tool for differential diagnosis between epileptic and psychogenic nonepileptic seizures.
Seizure prediction
The ability to identify a mathematically defined preictal period via dynamical analysis of EEG and seizure predictability studies has constituted the basis for implementation of algorithms for prospective prediction of epileptic seizures. A major difficulty encountered in the attempt to prospectively predict an impending seizure is illustrated in Fig. 2 . In this figure, the average T-index profiles created from critical pairs of dynamically entrained sites in the 10 minute interval prior to 3 different time instants (1.45 hours, 0.57 hours, and 0 hours before seizure onset) are shown for a second typical seizure from the same patient as in Fig. 1 . It is evident that, if critical pairs are selected far away from that seizure (see Fig. 2 A), they may not be the relevant ones for prediction of this seizure. For example, critical pairs selected from 1.45 hours before seizure onset become disentrained before the seizure’s occurrence, whereas pairs selected from within the preictal period of this seizure are kept entrained until the seizure occurs to disentrain them. (The preictal period is defined and estimated from the average T-index profile of critical pairs selected in the 10 minute interval prior to 0 hours before seizure onset; see Fig. 2 C.) The participation of the epileptogenic focus in the critical sites in each case is illustrated in Fig. 3 . Visual inspection of Fig. 3 A, B shows that participation of the focus (right hippocampus in this patient) in the entrainment of normal sites becomes prominent as the seizure approaches. Thus, monitoring the T-index profile of the relevant critical pairs of sites is of paramount importance for prospective seizure prediction. The solution we have given to this problem is adaptive optimal estimation of critical sites (first-generation seizure prediction algorithms ) or pairs of sites (second-generation seizure prediction algorithms, independent from user input and without need for optimization from training datasets, that is, ready to run anytime on any patient without any a priori information ). In each case, predetermined rules are applied for adaptive selection of the critical sites or pairs of sites over time from present and past T-index values. These seizure prediction algorithms, applied to scalp and/or intracranial EEG from patients and/or animals with epilepsy, have achieved greater than 80% sensitivity (that is, more than 80% of seizures are predicted), fair specificity (false prediction rate of 0.12–0.17 per hour, that is, 1 false positive per 8 to 7 hours respectively) and average prediction time of more than 45 minutes per seizure (that is, issue of a warning for an impending seizure 45 minutes before its occurrence). Sensitivity and specificity did not depend on either seizure rate or the recording EEG modality.
Following our first findings on seizure predictability, there have been many investigations by other research groups worldwide in the last 20 years. References, chronologically cited herein from 1990 to 2010, indicate these investigations. A variety of methods have been used in analysis of short-term and long-term EEG: parametric (model based) or nonparametric (transform based), linear or nonlinear, reference dependent (eg, using baseline EEG segments from interictal periods) or independent, and at the microscopic (neuronal) or macroscopic (network) level. In general, these investigations have pointed to seizure predictability with varying degrees of success. Methods for statistical evaluation of their performance, regarding sensitivity and specificity on training and testing EEG datasets, also against periodic or pseudorandom warning time series, and the use of surrogate data to account for spurious values and noise in the EEG have been developed. However, on seizure prediction (prospective analysis) few investigations have been performed. Seizure prediction, which is what is needed in medical applications, is different from and more difficult to achieve than seizure predictability, as we have argued and shown earlier. A more recent investigation in seizure prediction was performed by Schulze-Bonhage’s group, a group that was very skeptical in the past with respect to feasibility of seizure prediction schemes. These investigators reported a maximum mean sensitivity of 43.2%, at a false seizure prediction rate of 0.15 per hour. Training on each patient’s EEG data was required before a prospective run of the algorithm on a patient. The interval at which a seizure was predicted ahead of time was not clearly reported but appeared to be in the range of 10 to 60 minutes. Although this performance is sensitivity-wise almost half of what we have reported in the past, even with our first-generation seizure prediction algorithms, it is an additional piece of independent evidence that seizures can not only be predictable (retrospective analysis) but predicted (prospective analysis) too.
Most recently, two other groups have attempted prospective seizure prediction using pattern recognition methodologies. A training stage per patient was required here too. The reported specificity values varied widely per patient while sensitivity to seizures was very high and appeared encouraging. However, in a closer inspection, both groups used the Freiburg database, that is, a database with fragmented, discontinuous EEG data (one 24 hour interictal period well separated from about 50 min preictal periods for a couple of seizures per patient). Even though this is an intermediate step towards seizure prediction, it may give a false picture of the capabilities of a seizure prediction algorithm, which has to work not only in real time but on line on continuous EEG data. This fact is shown by Freiburg group’s recent publication and the poor results they obtained about sensitivity using a fair specificity value when they decided to run their algorithms on long-term continuous EEG data.
Application of seizure prediction to seizure control
Incorporation of seizure prediction algorithms to neuromodulation schemes for abatement of seizures is one important area of application in the modern treatment of epilepsy (eg, via electromagnetic stimulation as in DBS, VNS, TDC and TMS, and via implantable cooling or in situ chemicals release/drug delivery systems). Our findings from analysis of long-term EEG dynamics in the domains of seizure predictability, prediction, and resetting, in animals and patients with various types of epileptic seizures and EEG recordings (scalp and intracranial), led us to formulate the following hypothesis about ictogenesis in terms of basic principles of control in adaptive linear or nonlinear dynamical systems: epileptic seizures may occur because of pathologic alterations in the global and/or local internal feedback loops in the brain, normally responsible for keeping the spatial correlations (interactions, synchronizations/entrainment) within strict limits with respect to time and space in order to support the brain’s fast multiprocessing and multitask function.
Using neuronal population models that are capable of exhibiting seizurelike behavior, we have shown that entrainment (disentrainment) of the populations’ STL max , with increased (decreased) coupling between populations, resembles the observed preictal dynamical entrainment (postictal disentrainment) of the STL max at critical sites in the epileptic brain. In agreement with burst phenomena in adaptive systems, seizures in these models occur if the existing (internal to the models) feedback loops are pathologic, in the sense that they lack the ability to compensate fast enough for excessive increases in the network coupling. This situation eventually leads to seizurelike transitions in those models. Motivated by these findings, we postulated the existence of an internal pathologic feedback action in the epileptic brain; subsequently, using a control-oriented approach, we developed a functional model for an external seizure controller. During periods of abnormally high synchrony (entrainment), the developed control scheme provides appropriate desynchronizing feedback to maintain normal synchronization levels between neural populations (homeostasis of dynamics). We have called this closed-loop feedback control view of epileptic seizures, and the developed seizure control strategies, feedback decoupling and have validated it on coupled chaotic oscillator models and biologically plausible neurophysiologic models.
Within the context of closed-loop, real-time, online control of seizures, seizures should be anticipated with good sensitivity and specificity from dynamical analysis of EEG, and effective external intervention is applied to change the pathologic brain dynamics (long-term entrainment) in a timely manner and prevent a seizure from occurring. To this end, we used our adaptive seizure prediction algorithm in the feedback branch of a closed-loop electrical stimulation scheme for seizure control. The lithium-pilocarpine (LP) model of acute SE in rats was chosen as the animal model for chronic epilepsy. Male Sprague-Dawley rats were used for the study. Three to 4 weeks after induced SE, rats were implanted with a 6 microwire monopolar electrodes targeted to 4 cortical and 2 hippocampal locations; 2 Teflon-coated tungsten bipolar twisted stimulating electrodes were implanted in the centromedial thalamic nucleus. Our second-generation seizure prediction program was used to predict, online and in real time, the developed seizures ( Fig. 4 ) and trigger an A-M Systems Model 2300 stimulator unit (Carlsborg, WA, USA) at seizure warnings. At a seizure warning, a train of square pulses was delivered in a charge-balanced bipolar cathodic fashion ( Fig. 5 ).
Results from this experiment are shown in Fig. 6 . The experiment consisted of weeks-long just-in-time (closed-loop) stimulation (phases A–D in Fig. 6 ) and periodic (open-loop) stimulation (phase E in Fig. 6 ). Weeks of dramatic reduction of seizures (see panel 3 in Fig. 6 ) followed just-in-time adaptive stimulation of the epileptogenic focus (left hippocampus; localized by analysis of the EEG by focus localization algorithms ) until resetting of dynamics is achieved (phase D in Fig. 6 ), whereas less reduction of seizures followed just-in-time stimulation of the thalamus (phases A and B in Fig. 6 ). No reduction of seizures compared with baseline followed open-loop periodic (period equal to the inverse of the mean of seizure rate in baseline) stimulation of the focus (phase E in Fig. 6 ). These results imply that closed-loop, focal stimulation schemes are superior to closed-loop, nonfocal schemes, which in turn are superior to open-loop, focal schemes. The demonstration of the importance of incorporating seizure prediction algorithms in closed-loop control schemes (just-in-time), as well as of the location of stimulation, for online seizure abatement was one goal of this experiment. The importance of stimulus form for seizure abatement, such that it ensures resetting of the entrainment of dynamics, was another goal (use of long stimuli until dynamics are reset resulted in further reduction of seizure rates; compare phases A vs B, and C vs D in Fig. 6 ). These results are in broad agreement and better than the ones we reported in Ref. with the epileptogenic focus identification and adaptive stimulation of the focus (hippocampus) versus thalamus contributing a lot to the observed drastic improvement in seizure abatement.
A look into the future
The results from investigations into seizure prediction in the last 25 years have established firm foundations for direct clinical applications to epilepsy. The concepts delineated in this article constitute the basis for a dynamical interpretation of ictogenesis and lead to long-term prediction of seizures tens of minutes before their clinical or electrographic onset, detection of seizure susceptibility periods, and the development of innovative treatments for epilepsy through timely interventions to control seizure occurrence (eg, via electrical stimulation and/or in situ drug delivery). We have shown that timing of intervention is critical for successful control of seizures, and that seizure prediction algorithms can now provide this important information online and in real time, making them an important component of future brain pacemakers for epilepsy.
Seizures seem to be the end result of a progressive recruitment of brain sites by the focus toward an abnormal synchronization of dynamics. The onset of such recruitment occurs on the order of minutes with respect to seizure prediction, on the order of hours and days with respect to seizure susceptibility. Resetting of this pathologic preictal dynamical recruitment occurs at seizures. In cases in which seizures are unable to reset this disorder of dynamics (eg, in SE), external intervention (eg, via AEDs) may be successful.
In light of the previous discussion, a dynamical systems perspective of the epileptic brain can also be formulated. Seizures could result from the inability of internal feedback mechanisms to provide timely compensation/regulation of coupling (entrainment) between brain sites, and hence seizure control may be achieved by preictally and/or interictal decoupling the pathologic sites via externally provided appropriate feedback. Such optimal feedback decoupling has been found to be a function of both the recorded EEGs and the coupling between respective brain sites. According to this hypothetical dynamical scheme of ictogenesis, the following series of events has been conjectured: (1) brain in spatiotemporal chaos; (2) internal or external stimulus enters the system and changes the spatial coupling between two or more brain sites; (3) spatial coupling produces spatial correlations, possibly storing the information about the stimulus and/or initiating action; (4) spatial correlations activate an internal compensating feedback mechanism; (5) compensation tries to remove (or assimilate) the developed spatial correlations; (6) the system returns to spatiotemporal chaos if step 5 is successful. In the normal brain, the correlations in the network must lie within a normal range and vary quickly in response to a stimulus, which implies that the internal feedback path should be well tuned and able to track changes of the coupling with time reasonably well. In the epileptic brain, step 5 involves pathologic (poorly tuned) internal feedback paths. The observed long-term dynamical entrainment before seizures can then be interpreted as an indicator of disorder in the internal feedback of the network. According to the theory of adaptive systems control, such disorders can exhibit large errors in the estimation of the coupling, and in turn cause local destabilization and bursting of the network (seizures). This rationale can also be applied to explain the mechanisms of reflex epilepsies, with external stimuli as inputs to the system causing enduring entrainment, and seizures following to reset it.
A global view of epilepsy and seizures in terms of systems dynamics and control theory constitutes an interdisciplinary avenue of research between engineering, neuroscience, and medicine, and has already shown that it can generate refreshing results and novel hypotheses. I strongly believe that it would benefit the epilepsy patient if this path continues to be pursued and developed further in the years to come.
Acknowledgments
I would like to acknowledge the support of this research by the National Institutes of Health ( NIH EB002089 BRP Grant on Brain Dynamics; NIH SBIR 1R43NS050931-01A1 ; NIH R21 NS061310-01A1 ), the Epilepsy Research Foundation of America and Ali Paris Fund for LKS Research, the National Science Foundation (grant no. 0,601,740 ), and the Arizona Science Foundation (Competitive Advantage Award grant CAA 0281-08 ).
The author has nothing to disclose.
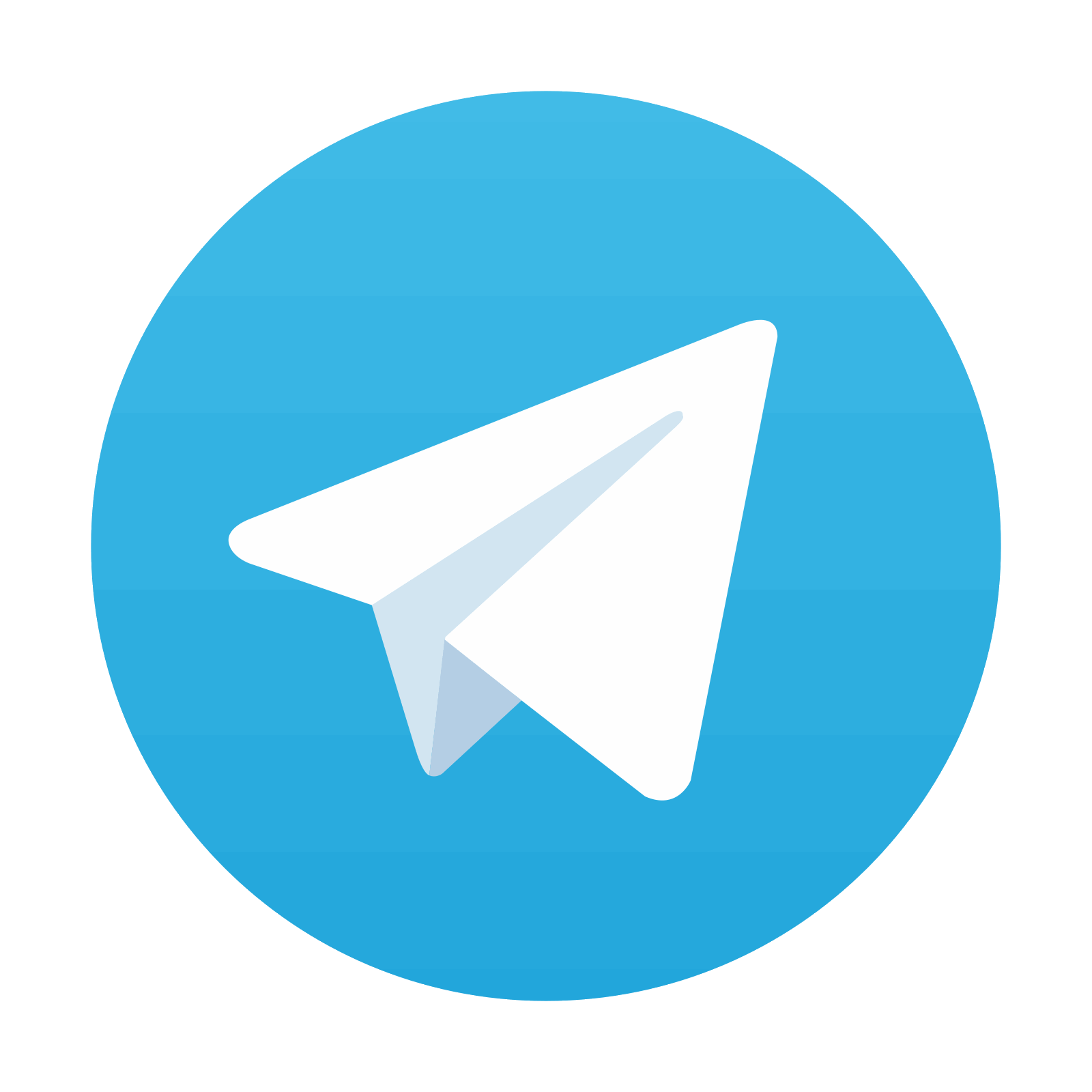
Stay updated, free articles. Join our Telegram channel

Full access? Get Clinical Tree
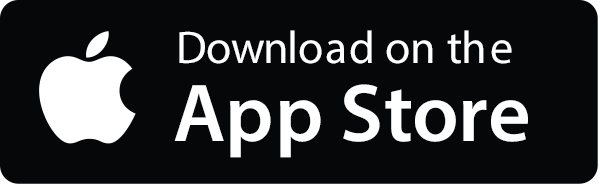
