Fig. 1
Hub-network: Intensively communicating top-level systems of separate representation hierarchies, and their proposed neural substrates in the brain. HC hippocampus; value – orbitofrontal cortex codes value of objects and action outcomes; context – retrosplenial cortex and precuneus are structures that represent concepts of spatial context for own behavior; object concepts – perirhinal cortex represents object concepts; action choice – medial pre – frontal cortex (mPFC) selects basic actions
The medial PFC selects basic actions (escape, fight, relax, consume, etc.) based on task-demand driven situation understanding provided by inputs about relevant current objects, their value, and the current spatial and behavioral context. The outputs of the mPFC to subcortical autonomous centers enable the adaptation of internal state of the body to the intended behavior. The hippocampus (HC) supports fast learning between any content including object-context-action-outcome. By activating such stored behavioral models we can construct a new scene with objects and persons, imagine an action and outcome [4]. This basic hub network is activated for any retrieval of self-related memory, as well as for planning and envisioning the future [5, 6]. Separate representation hierarchies dynamically linked at its top levels seem to be the key for that capability.
2.2 Rhythmic Control for Repetitive Composition of Dynamic Situation Model
For keeping track with the rapidly changing real world environment, the situation model must be updated with a sufficiently high frequency. Hence, to enable such a recurrent iteration of the construction process of the situation model, the length of the construction process must be limited. In the cortex, rhythmic modulation of excitability is ubiquitous and provides a natural control for iteration processes. Hippocampal nested theta and gamma rhythms may serve to link distributed subsystems of the different representational hierarchies (as outlined above) repetitively into a dynamic situation model according to the changing interaction situation. Any temporary stable composition can be learned with HC.
During slow-wave sleep so-called sharp-wave ripples (SW-R) transfer stored knowledge from HC to the hub network and the neocortex for consolidation [7]. However, the synchronizing SW-Rs during awake state seem to be involved in planning and decision making [8]. In the awake state a characteristic ripple-related sequence of events is observed starting with restriction of ongoing sensory inputs (extensive inhibition of subcortical structures), via activation of mPFC (action choice) and finally generation of SW-Rs in the hippocampus that synchronize respective sensory and motor areas for action realization [9].
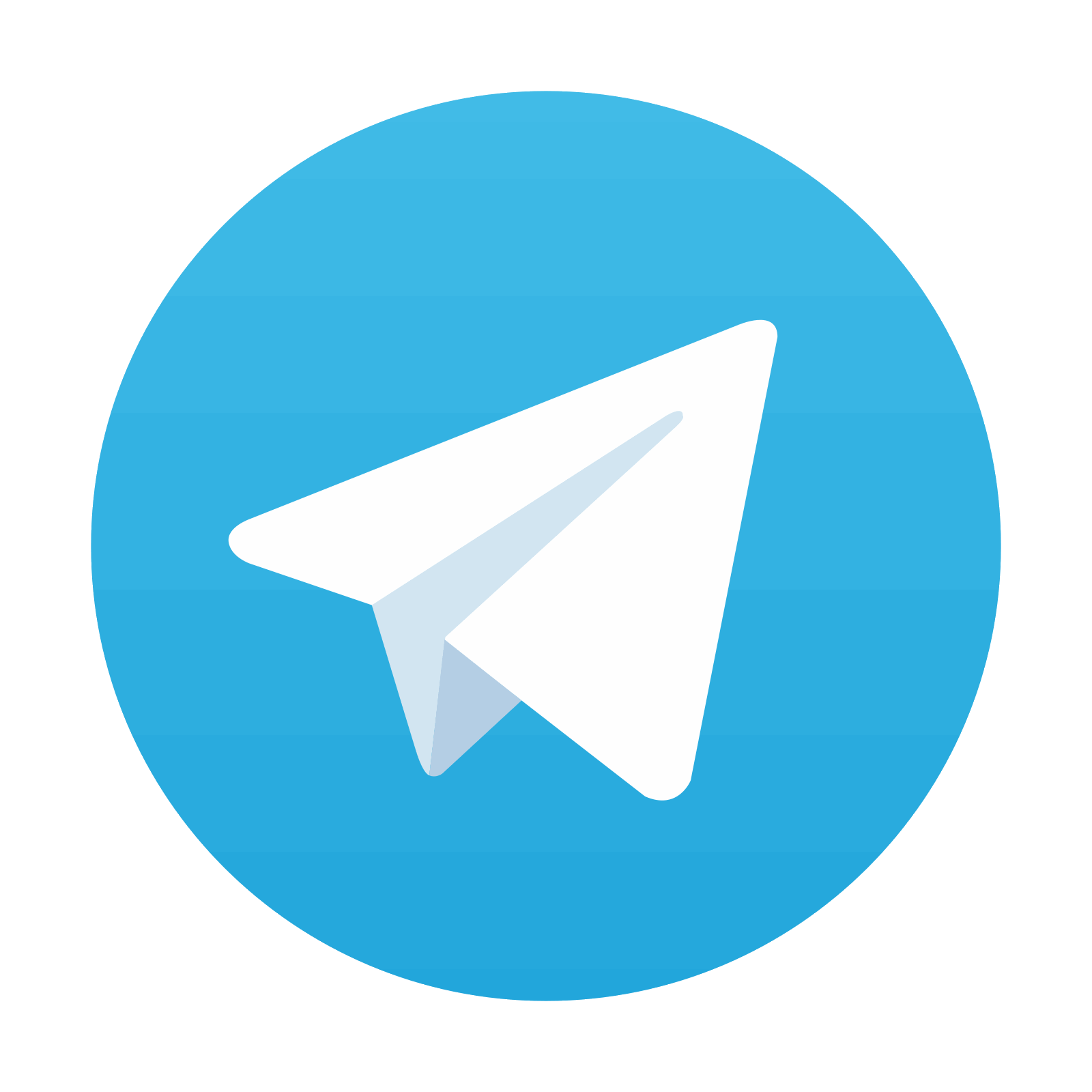
Stay updated, free articles. Join our Telegram channel

Full access? Get Clinical Tree
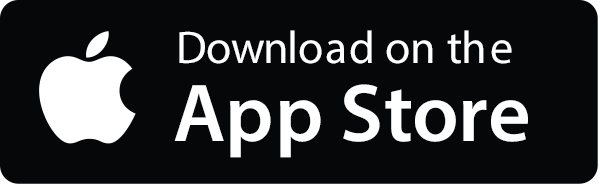
